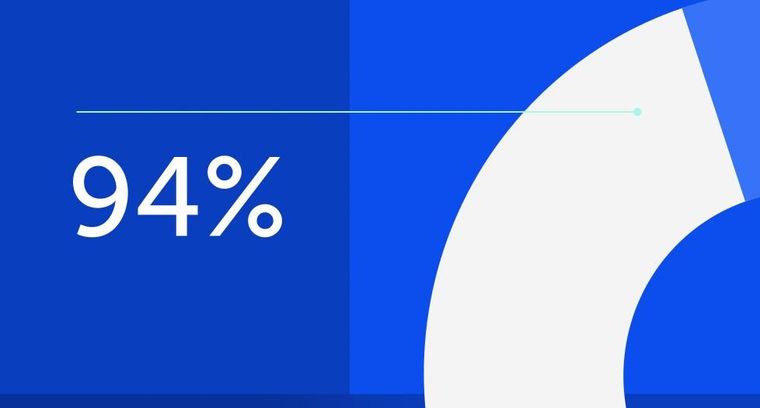
94% of researchers rate our articles as excellent or good
Learn more about the work of our research integrity team to safeguard the quality of each article we publish.
Find out more
ORIGINAL RESEARCH article
Front. Pharmacol., 04 January 2022
Sec. Ethnopharmacology
Volume 12 - 2021 | https://doi.org/10.3389/fphar.2021.808867
This article is part of the Research TopicTherapeutic Effects of Herbal Medicines: How Can We Best Investigate Bioactive Metabolites?View all 19 articles
San-Huang-Yi-Shen capsule (SHYS) has been used in the treatment of diabetic nephropathy (DN) in clinic. However, the mechanisms of SHYS on DN remain unknown. In this study, we used a high-fat diet (HFD) combined with streptozotocin (STZ) injection to establish a DN rat model. Next, we used 16S rRNA sequencing and untargeted metabolomics to study the potential mechanisms of SHYS on DN. Our results showed that SHYS treatment alleviated the body weight loss, hyperglycemia, proteinuria, pathological changes in kidney in DN rats. SHYS could also inhibite the oxidative stress and inflammatory response in kidney. 16S rRNA sequencing analysis showed that SHYS affected the beta diversity of gut microbiota community in DN model rats. SHYX could also decrease the Firmicutes to Bacteroidetes (F to B) ratio in phylum level. In genus level, SHYX treatment affected the relative abundances of Lactobacillus, Ruminococcaceae UCG-005, Allobaculum, Anaerovibrio, Bacteroides and Candidatus_Saccharimonas. Untargeted metabolomics analysis showed that SHYX treatment altered the serum metabolic profile in DN model rats through affecting the levels of guanidineacetic acid, L-kynurenine, prostaglandin F1α, threonine, creatine, acetylcholine and other 21 kind of metabolites. These metabolites are mainly involved in glycerophospholipid metabolism, tryptophan metabolism, alanine, aspartate and glutamate metabolism, arginine biosynthesis, tricarboxylic acid (TCA) cycle, tyrosine metabolism, arginine and proline metabolism, arginine and proline metabolism, phenylalanine, tyrosine and tryptophan biosynthesis, phenylalanine metabolism, and D-glutamine and D-glutamate metabolism pathways. Spearman correlation analysis showed that Lactobacillus, Candidatus_Saccharimonas, Ruminococcaceae UCG-005, Anaerovibrio, Bacteroides, and Christensenellaceae_R-7_group were closely correlated with most of physiological data and the differential metabolites following SHYS treatment. In conclusion, our study revealed multiple ameliorative effects of SHYS on DN including the alleviation of hyperglycemia and the improvement of renal function, pathological changes in kidney, oxidative stress, and the inflammatory response. The mechanism of SHYS on DN may be related to the improvement of gut microbiota which regulates arginine biosynthesis, TCA cycle, tyrosine metabolism, and arginine and proline metabolism.
Diabetic nephropathy (DN), major complications of diabetes, is a contributing factor in late-stage renal failure (Wang et al., 2016). The current treatment for DN includes blood glucose control and regulation of lipid metabolism using anti-hypertension medicines (Mann et al., 2010; Pazdro and Burgess, 2010; Tone et al., 2005; Jin et al., 2014). However, these conventional treatments do not completely prevent the progression of DN (Tuttle et al., 2018). The development of new therapeutic agents to improve renal function and halt the progression of DN has become an active area of investigation.
Traditional Chinese medicine (TCM) has contributed significantly to the treatment of DN. Numerous studies have shown that TCM has significant advantages for improving renal function, controlling blood glucose levels, and inhibiting the inflammatory response on DN (Zhong et al., 2015). A multicenter randomized controlled study showed that Liu-Wei-Di-Huang pill significantly reduces erythrocyte aldose reductase activity, β2-microglobulin expression, and urinary protein levels in diabetic patients (Song et al., 2004). Qi-Zhi-Jiang-Tang decoction also delays the progression of DN (Guo et al., 2014). Wu-Ling powder ameliorates the inflammatory response in a rat model of DN by inhibiting the NF-κB signaling pathway (Liu et al., 2009). Tong-Shen-Luo (TSL) significantly reduces the extracellular matrix in renal tissues in a rat model of DN (Wu et al., 2007). Finally, Xian-Zhen decoction decreases the accumulation of glycosylated products in renal tissues of rats with DN (Tang et al., 2005).
The human intestine contains a plethora of microorganisms that interact with each other and together maintain the metabolic-immune nervous system homeostasis of the body (Li et al., 2020). Disorders in gut microbiota contribute to the development of various diseases (Guarner, 2006). Patients with DN have an increased ratio of Firmicutes to Bacteroides in the gut compared with healthy individuals (Gong et al., 2021). These alterations in the microbiota are associated with various pathological changes associated with DN, abnormal hemodynamic inflammatory responses, and metabolic abnormalities (Grigor’eva, 2020; Magne et al., 2020; Macho-González et al., 2021; Zhang et al., 2021). Transplantation of germ-free mice with microbiota from mice with DN resulted in elevated levels of 24-h urine proteins (Cai et al., 2020). Furthermore, gut microbiota may be involved in the progression of DN by modulating metabolism (Li et al., 2020). The pathogenesis of DN may be elucidated by analyzing microbiota metabolism using 16S rRNA sequencing technology combined with untargeted metabolomics, This may lead to the development of new treatments for DN that regulate gut microbiota and host metabolism.
San-Huang-Yi-Shen capsule (SHYS) consists of Astragalus mongholicus Bunge, Panax quinquefolius L., Dioscorea oppositifolia L., Cornus officinalis Siebold and Zucc., Cuscuta chinensis Lam., Polygonatum sibiricum Redouté, Rehmannia glutinosa (Gaertn.) DC., Euryale ferox Salisb., Rosa laevigata Michx., Leonurus japonicus Houtt., Salvia miltiorrhiza Bunge, Conioselinum anthriscoides ‘Chuanxiong’, Atractylodes lancea (Thunb.) DC., Paeonia lactiflora Pall., and Gypsophila vaccaria (L.) Sm. And has been used in the treatment of many chronic renal diseases including DN, IgA nephropathy and chronic renal failure (Bian et al., 2011; Su et al., 2015b; Zhong et al., 2016). Clinical studies showed that SHYS could alleviate the proteinuria in DN patients (Su et al., 2015a). Animal study has also demonstrated the protective effects of SHYS on DN model rats (Chi et al., 2013). However, the mechanisms of SHYS on DN remain unknown. In this study, we used a high-fat diet (HFD) combined with streptozotocin (STZ) injection to establish a DN rat model. Next, we used 16S rRNA sequencing and untargeted metabolomics to study the potential mechanisms of SHYS on DN.
HFD (65.75% basal chow, 20% sucrose, 10% lard, 3% egg yolk powder, 1% cholesterol, 0.25% pig bile salt) was purchased from Beijing Sibeifu Bioscience Co., Ltd. (Beijing, China). Irbesartan was obtained from Sanofi Winthrop Industrie (France). STZ was purchased from Solarbio Biotechnology Co., Ltd. (Beijing, China). Creatinine (Cr), blood urea nitrogen (BUN), urine protein, superoxide dismutase (SOD), methane dicarboxylic aldehyde (MDA), and glutathione peroxidase (GSH-Px) assay kits test kits were obtained from Nanjing Jiancheng Biological Engineering Institute (Nanjing, China). Rat interleukin (IL)-1β, IL-6, tumor necrosis factor alpha (TNF-α) enzyme-linked immunosorbent assay (ELISA) kit was obtained from Multi Science Biotechnology Co., Ltd. (Hangzhou, China). Reference standards of ferulic acid, atractylodin, tanshinone, astragaloside, hyperoside, loganin, gallic acid, morroniside, allantoin, oleanic acid, ginsenoside Rb1, ginsenoside Re, ginsenoside Rg1, paeoniflorin, vaccarin, pyrrolidinium, leonurine, and catalpol were obtained from Sichuan Weikeqi Biological Technology Co., Ltd. (Sichuan, China).
SHYS was prepared from the pharmacy department of Cangzhou Hospital of Integrated Traditional Chinese and Western Medicine. Briefly, 15 g of Astragalus mongholicus Bunge (Batch number: 201126), 12 g of Panax quinquefolius L., 12 g of Dioscorea oppositifolia L. (Batch number: 201008), 12 g of Cornus officinalis Siebold and Zucc. (Batch number: 201126), 12 g of Cuscuta chinensis Lam. (Batch number: 200815), 12 g of Polygonatum sibiricum Redouté (Batch number: 200912), 15 g of Rehmannia glutinosa (Gaertn.) DC. (Batch number: 201126), 12 g of Euryale ferox Salisb. (Batch number: 201012), 12 g of Rosa laevigata Michx. (Batch number: 201126), 10 g of Leonurus japonicus Houtt. (Batch number: 200817), 12 g of Salvia miltiorrhiza Bunge (Batch number: 201102), 12 g of Conioselinum anthriscoides ‘Chuanxiong’ (Batch number: 200817), 10 g of Atractylodes lancea (Thunb.) DC. (Batch number: 201014), 10 g of Paeonia lactiflora Pall. (Batch number: 201012), and 6 g of Gypsophila vaccaria (L.) Sm. (Batch number: 201106) were weighed. All herbs were authenticated by pharmacist in the pharmacy department of Cangzhou Hospital of Integrated Traditional Chinese and Western Medicine. Then, these crude herbs were decocted, evaporated and made into capsuled prepapation according to the medical institution preparation standard in Hebei (approval number: Z20050795). The specification of SHYS was 0.45 g per capsule. The production licence of SHYS was shown in Supplementary Figure S1.
Quality control of SHYS was performed using Ultra performance liquid chromatography (UPLC; ACQUITY UPLC®, United States) coupled with Xevo G2 quadrupole-time of-flight (Q-TOF) mass spectrometer (MS; Waters Corp., Milford, MA, United States) systems. Briefly, the test solution was injected onto an ACQUITY UPLC BEH C18 Column (2.1 mm × 100 mm, 1.7 μm) held at 50°C. The flow rate was 0.3 ml/min and the injection volumn was 2 μl. Mobile phase A was 0.1% formic acid aqueous solution and mobile phase B was acetonitrile contained 0.1% formic acid. The mobile phase conditions were: 0 min, 5% B; 1 min, 10% B; 6 min 60% B; 6.5 min 100% B; 10 min 100% B; 10.1 min 5% B; 13 min 5% B.
A Q-TOF MS equipped with an electrospray ionization (ESI) source was used for both positive and negative ionization scan modes (m/z ranges from 50 to 1,200 Da). The scan time was 0.2 s. The capillary voltages were 3,000 V (positive mode) and 2,200 V (negative mode), respectively. The desolvation temperature was 350°C and the source temperature was 100°C. The sample cone voltage was 40 V and the extraction cone voltage was 4 V. The cone gas flow was 40 L/h and the desolvation gas flow was 800 L/h (both positive and negative modes).
Sixty male Sprague-Dawley (SD) rats (speciic pathogen free grade, 6–8 weeks old) weighing 200 ± 20 g, were obtained from the Beijing Huafukang Biotechnology Co., Ltd. [Production License No.: SCXK (Beijing) 2020-0016]. The animal were housed at 25 ± 2°C, a 12 h light-dark cycle, with free access to food and water. The study was approved by the Ethics Committee of Cangzhou Hospital of Integrated Traditional Chinese and Western Medicine.
DN model was induced using HFD and STZ injection as described previously (Shen et al., 2020). Briefly, rats were fed with HFD for 8 weeks. Subsequently, rats were injected intraperitoneally with 30 mg/kg of STZ (dissolved in 0.1 mol/L citric acid buffer, pH = 4.5). After STZ injection, rats were continued to be fed with HFD and blood glucose and 24-h urine protein levels after fasted for 12 h were investigated weekly. FBG levels of ≥12 mmol/L and urine protein levels of ≥20 mg/24 h were used as criteria for establishing the animal model of DN (Zhang et al., 2014), this occurred 2 weeks after STZ injection.
After 1 week of acclimatization, 10 rats were randomly selected as the Control group and provided a standard diet, whereas the remaining 50 rats received HFD and STZ injection to induce DN. After the animal model had been established, DN rats were randomly divided into the Model, Irbesartan, SHYS low-dose, SHYS middle-dose, and SHYS high-dose groups, with 10 rats in each group. Rats in Control and Model groups were gavaged with 2 ml of distilled water; Rats in Irbesartan were gavaged with 11.51 mg/kg irbesartan (Wang et al., 2018); while rats in SHYS low-dose, SHYS middle-dose and SHYS high-dose groups were gavaged with SHYS capsule doses of 0.41, 0.81, and 1.62 g/kg, respectively, once daily for 4 consecutive weeks. The dosage in SHYS middle-dose group in our study was based on a conversion of human to rat body surface area, while the low dose was half the middle dose and high dose was double the middle dose. Body weight and FBG were measured every 2 weeks after SHYS treatment (Figure 1).
After 4 weeks of SHYS intervention, 24 h urine samples were collected from all rats using metabolic cages. Then, rats were anesthetized with an intraperitoneal injection of 50 mg/kg pentobarbital sodium. Blood was collected from the inner canthus, and centrifuged at 3,000 rpm for 15 min to collect the serum. The levels of 24-h urine protein and serum creatine (Cr) and blood urea nitrogen (BUN) in each group of rats were determined using the test kits according to the manufacturer’s instructions (Nanjing Jiancheng Biological Engineering Institute).
The rats were sacrificed by cervical dislocation after blood sampling. The kidneys were quickly removed. 0.1 g of kidney tissues were weighed and immersed in 900 μl of normal saline and homogenized at 4°C. The supernatants were collected after centrifugation at 3,000 rpm for 15 min to obtain the renal tissue homogenate. Level of the total protein content in renal tissue homogenate was detected by BCA assay according to the manufacturer’s instructions (Nanjing Jiancheng Biological Engineering Institute). Besides, SOD and GSH-Px activities and MDA levels representing oxidative stress were measured according to the instructions described in the kit (Nanjing Jiancheng Biological Engineering Institute).
After 4 weeks of SHYS intervention, IL-6, IL-1β, and TNF-α levels in renal tissue homogenates from each group were measured by ELISA, which was performed based on the manufacturer’s instructions (Multi Science Biotechnology Co., Ltd.).
After 4 weeks of SHYS intervention, the renal tissues from each group were fixed in 10% formalin, washed with water for 20 min, dehydrated in gradient alcohol, cleared with xylene, embedded in paraffin. The tissues were cut into 5 μm thick strips, stained with hematoxylin and eosin (H and E), and subjected to Masson and Sirius Red staining. The relative collagenous fiber area was quantified using integrated optical density (IOD) with the Image-Pro Plus 6.0 software. The positive area (%) was calculated according to the following formula: IOD/sum area × 100%.
After 4 weeks of SHYS intervention, cecum contents were collected and weighed. Total genomic DNA was extracted using the CTAB/SDS method. The purity and concentration of DNA samples were assessed on a 1% agarose gel. The DNA was diluted to 1 ng/μl with sterile water.
The V3-V4 region of the 16S rRNA gene was amplified using 338 F (5'-ACTCCTACGGGAGGCAGCAG-3') and 806R (5'-GGACTACHVGGGTWTCTAAT-3') primers. PCR amplification included 10 ng of template DNA, 0.2 µm of forward and reverse primers, and 15 µl of Phusion® High-Fidelity PCR Master Mix (New England Biolabs). The reaction conditions were as follows: pre-denaturation at 98°C for 1 min, 15 cycles of denaturation at 95°C for 10 s, annealing at 50°C for 30 s and extension at 72°C for 30 s, and then holding at 72°C for 5 min and storage at 4°C. The PCR products were purified using the Qiagen Gel Extraction Kit (Qiagen, Germany). A 2% agarose gel was used for detection. Sequencing libraries were generated using the TruSeq® DNA PCR-Free Sample Preparation Kit (Illumina, United States) and library quality was assessed on a Qubit@ 2.0 Fluorometer (Thermo Scientific) and an Agilent Bioanalyzer 5400 system (Agilent, United States). The analysis of peak-heights in agarose gel electropherogram was shown in Supplementary Figure S2. Finally, the libraries were sequenced on the Illumina NovaSeq platform to obtain 250 bp of paired-end sequences.
The raw sequencing data were spliced using FLASH (Version 1.2.7, http://ccb.jhu.edu/software/FLASH/). After quality control, the effective tags were obtained. The tags were clustered using Uparse software (Version 7.0.1001, http://drive5.com/uparse/) at 97% similarity to obtain operational taxonomic units (OTUs) (Edgar, 2013). The SILVA reference database (Version 138, http://www.arb-silva.de/) based on the Mothur the algorithm, was used to annotate the OTUs with taxonomic information (Quast et al., 2012). Multiple sequence comparisons were conducted using MUSCLE software (Version 3.8.31, http://www.drive5.com/muscle/) (Edgar, 2004). The abundance of OTUs was normalized using the sequence number corresponding to the sample with the smallest sequence. Αlpha-diversity indicator and beta-diversity analysis were subsequently performed. The Wilcoxon Rank-Sum test was used for statistical difference analysis between groups of diversity indicators. The Kruskal–Wallis rank-sum test was used (Games–Howell test was chosen as the post-hoc test) in conjunction with the multiple testing method of false discovery rate to screen for different bacteria. p < 0.05 after false discovery rate (FDR) correction was considered to be statistically significant. Finally, data analysis using Phylogenetic Investigation of Communities by Reconstruction of Unobserved States database (PICRUSt) was performed to predict the relevant biological pathways that may be affected by each group exhibiting different microbiota.
A 100 μl of serum sample was added to 400 μl of 80% methanol. The mixture was vortexed, shaken, and centrifuged at 15,000 g for 20 min at 4°C in an ice bath. After centrifugation, the supernatant was diluted with ultrapure water to 53% methanol and centrifuged again at 15,000 g for 20 min at 4°C. The supernatant was collected and used as the test sample. All samples were mixed in equal amounts as a quality control (QC) sample and analyzed periodically throughout the analysis process to ensure the stability and accuracy of the measurement throughout the analysis.
Chromatography was performed on a Hypesil Gold column (C18) column (2.1 mm × 100 mm, 1.9 μm) with a mobile phase consisting of (A) 0.1% formic acid and (B) methanol, using a gradient elution of 0 min, 98% A, 2% B; 1.5 min, 98% A, 2% B; 12 min, 0% A, 100% B; 14 min, 0% A, 100% B; 14.1 min, 98% A, 2% B; 17 min, and 98% A, 2% B. The column temperature was set to 40°C with a flow rate of 0.2 ml/min and an injection volume of 2 μl. MS conditions were simultaneous detected in positive- and negative-ion modes using electrospray ionization (ESI). The settings of the ESI were as follows: Spray Voltage: 3.2 kV; Sheath gas flow rate: 40 arb; Aux Gas flow rate: 10 arb; Capillary Temp: 320°C. QC samples were injected every six samples throughout the analytical run, and the data obtained were used to evaluate stability.
Molecular signature peaks in the samples were detected based on the results of high-resolution mass spectrometry. The molecular peaks were matched and identified by combining the high-quality mzCloud (https://www.mzcloud.org/), mzVault, and MassList databases constructed from the standards. The raw files (.raw) obtained by MS were imported into Compound Discoverer 3.1 (CD3.1, Thermo Fisher) software for data preprocessing. First, the data were briefly screened by the parameters of retention time and mass-to-charge ratio, and then the peaks from different samples were aligned according to the retention time deviation of 0.2 min and the mass deviation (Part per million, ppm) of 5 ppm to make identification accurate. Subsequently, the data were aligned according to the settings of 5 ppm, signal intensity deviation of 30%, signal-to-noise ratio (S/N) of 3, minimum signal intensity of 1,00,000. Adduct ions for peak extraction and peak area were also quantified. The metabolites were identified by the molecular formula prediction using molecular ion peaks and fragment ions, and were compared with the mzCloud and mzVault and MassList databases. Metabolites with a coefficient of variation of less than 30% in the QC samples were then retained as the final identification results for subsequent analysis. The peaks detected in the samples were integrated using CD3.1 software, where the peak area of each characteristic peak represented the relative quantitative values of a metabolite, and the quantitative results were normalized using the total peak area to obtain the final quantitative results for the metabolites. The data were then subjected to QC to ensure the accuracy and reliability of the results.
Next, the metabolites were subjected to a multivariate statistical analysis, including principal component analysis (PCA) and orthogonal partial least squares discriminant analysis (OPLS-DA), to identify differences in the metabolic patterns among groups. PCA and OPLS-DA were performed using SIMCA software (version 14.1, Umetrics, Sweden). Furthermore, two-tailed Student’s t-test was used to analyze the normalized peak areas for each metabolite. Differential metabolites between Control and Model groups and between Model and SHYS high-dose groups were screened based on p < 0.05 and variable importance of projection (VIP) > 1. Finally, the biological significance of metabolite correlations was explained by functional analysis including metabolic pathways. Metabolic pathway enrichment analysis was performed for differential metabolites with a fold-change (FC) > 1.20 or FC < 0.80 based on MetaboAnalyst software (https://www.metaboanalyst.ca/) and Kyoto Encyclopedia of Genes and Genomes (KEGG) database (https://www.kegg.jp/).
The experimental data were analyzed using SPSS 20.0 statistical software. Data were expressed as the mean ± standard deviation (SD). t-test was used for comparison between two groups. One-way analysis of variance and post-hoc analysis was used for comparison among multiple groups. Differences were considered statistically significant at p < 0.05.
Ferulic acid, atractylodin, tanshinone, astragaloside, hyperoside, loganin, gallic acid, morroniside, allantoin, oleanic acid, ginsenoside Rb1, ginsenoside Re, ginsenoside Rg1, paeoniflorin, vaccarin, pyrrolidinium, leonurine, and catalpol were used as the reference standards to validate the main compounds in SHYS. The detailed information of these compounds were shown in Supplementary Figure S3. The typical based peak intensity (BPI) chromatograms of SHYS and these reference standards were shown in Supplementary Figure S4. The characteristic fragment ions of these compounds were shown in Supplementary Table S1. Astragaloside in Astragalus mongholicus Bunge, ginsenoside Rb1, ginsenoside Re, and ginsenoside Rg1 in Panax quinquefolius L., allantoin in Dioscorea oppositifolia L., loganin and morroniside in Cornus officinalis Siebold and Zucc., hyperoside in Cuscuta chinensis Lam., Pyrrolidinium in Polygonatum sibiricum Redouté, Catalpol in Rehmannia glutinosa (Gaertn.) DC., gallic acid in Euryale ferox Salisb., oleanic acid in Rosa laevigata Michx., leonurine in Leonurus japonicus Houtt., tanshinone in Salvia miltiorrhiza Bunge, ferulic acid in Conioselinum anthriscoides ‘Chuanxiong’, atractylodin in Atractylodes lancea (Thunb.) DC., paeoniflorin in Paeonia lactiflora Pall., and vaccarin in Gypsophila vaccaria (L.) Sm. were identified as the preeminent compounds in SHYS.
At the end of drug administration, the body weight in the Model group decreased significantly compared with that of the Control group (p < 0.01). The body weight of the rats in the Irbesartan, SHYS low-, middle-, and high-dose groups increased significantly compared with that of the Model group (p < 0.01, p < 0.05, p < 0.01, p < 0.01, respectively, Figure 2A). FBG levels were ≥12 mmol/L in all rats 2 weeks after STZ injection. After 8 weeks of SHYS intervention, FBG levels were significantly higher in Model group compared with the Control group (p < 0.01) and were lower in the SHYS low-, middle-, and high-dose groups compared with that of the Model group (p < 0.05, p < 0.01, p < 0.01, Figure 2B). The results of renal function tests showed that the serum levels of Cr and BUN as well as 24-h urine protein levels were significantly higher in the Model group compared with that of the Control group (p < 0.01, respectively). The intervention with irbesartan and high-dose SHYS significantly reduced the serum levels of Cr (p < 0.05, p < 0.01, respectively), BUN (p < 0.05, p < 0.01, respectively), and 24-h urine protein in the DN rats (p < 0.01, respectively). The middle-dose SHYS resulted in significantly reduced 24-h urine protein (p < 0.05) and serum BUN levels in DN rats (p < 0.01, Table 1 and Figure 2C).
FIGURE 2. SHYS treatment alleviated the body weight loss, hyperglycemia, proteinuria, pathological changes in kidney and inflammatory response in DN rats. (A) SHYS treatment alleviated the body weight loss in DN model rats. (B) SHYS treatment decreased the FBG in DN rats. (C) SHYS treatment decreased the level of 24-h urine protein. (D) H and E staining indicated that SHYS treatment significantly improved the pathological changes of kidney in DN rats (200 ×) (E–H) Masson (E,G) and Sirius red (F,H) staining indicated that SHYS treatment significantly decreased collagen depositions in kidney (200 ×). (I) SHYS treatment decreased the levels of pro-inflammatory cytokines in renal tissue homogenates. Control, Model, Irbesartan, SHYS low-dose, SHYS middle-dose and SHYS high-dose (n = 10 per group) groups. Data are presented as the mean ± SD. ##: p < 0.01 as compared to the Control group; *: p < 0.05 as compared to the Model group; **: p < 0.01 as compared to the Model group; △: p < 0.05 as compared to the Irbesartan group; △△: p < 0.01 as compared to the Irbesartan group; ^: p < 0.05 as compared to the SHYS low-dose group; ^^: p < 0.01 as compared to the SHYS low-dose group
H and E staining results of the kidneys showed that the glomeruli and tubules of the animals in the Control group had normal structure. No mesentery or mesangial stromal hyperplasia was observed and no inflammatory cell infiltration was evident. The Model group showed focal tubular degeneration and atrophy, slight thickening of the glomerular basement membrane and mesangial hyperplasia. Compared with the Model group, the lesions were reduced in each drug-treated group and the improvement was more significant in the irbesartan group as well as the SHYS middle- and high-dose groups (Figure 2D). Masson staining revealed that the collagen appeared as a blue color by light microscopy. As shown in Figures 2E,G, the glomeruli and tubules of animals in the Control group had normal structures and collagen expression was unchanged. In the Model group, however, the collagen areas in the glomerular mesangial region and basement membrane were showed significantly increased compared with the Control group (p < 0.01). The glomerular and tubulointerstitial collagen deposition was lower in Irbesartan, SHYS low-dose, SHYS middle-dose, and SHYS high-dose groups compared with those in the Model group (p < 0.01, p < 0.05, p < 0.01, p < 0.01, respectively). The results of Sirius red staining was shown in Figures 2F,H and indicated that the Model group had significant increase of renal interstitial and vascular wall collagen depositions and thickened glomerular arterioles compared with the Control group, whereas the collagen deposition was lower in Irbesartan, SHYS low-dose, SHYS middle-dose, and SHYS high-dose groups compared with those in the Model group (p < 0.01, p < 0.05, p < 0.01, p < 0.01, respectively).
We further evaluated the effects of SHYS on oxidative stress in DN rats by measuring SOD, MDA, and GSH-Px in renal tissue homogenates from each group. Compared with the Control group, the activities of SOD and GSH-Px (p < 0.01, respectively) in the renal tissue homogenates of animals in Model group were significantly decreased, whereas the level of MDA (p < 0.01) was significantly increased. Irbesartan, SHYS middle-dose, and SHYS high-dose treated animals exhibited a significant increase in SOD (p < 0.01, p < 0.05, p < 0.01, respectively) and GSH- Px (p < 0.01, p < 0.05, p < 0.01, respectively) activities and decreased MDA (p < 0.01, p < 0.05, p < 0.01, respectively) levels in renal tissue homogenates (Table 2). The effects of SHYS on the inflammatory response of DN rats was also determined by measuring the levels of pro-inflammatory factors, IL-6, IL-1β, and TNF-α, in renal tissue homogenates from each group by ELISA. The levels of proinflammatory factors, IL-6, IL-1β, and TNF-α (p < 0.01, respectively) in renal tissue homogenates were significantly increased in rats of the Model group compared with that of the Control group. The levels of IL-6 in the renal tissue homogenates of DN rats were significantly decreased following treatment with irbesartan and high-dose of SHYS (p < 0.01 and p < 0.05, respectively). The levels of IL-1β were significantly decreased in Irbesartan, SHYS middle-dose and SHYS high-dose groups compared with the Model group (p < 0.05, p < 0.05 and p < 0.01, respectively). The levels of TNF-α were lower in Irbesartan, SHYS low-dose, SHYS middle-dose and SHYS high-dose groups compared with that in the Model group (p < 0.01, p < 0.05, p < 0.05, p < 0.01, respectively, Figure 2I). These results indicated that SHYS exhibits therapeutic effects on DN rats and the efficacy is most significant at high doses. Therefore, high-dose SHYS was selected for subsequent gut microbiota and metabolomics studies.
TABLE 2. Changes of SOD, GSH-Px activities and MDA level in renal tissue homogenates after SHYS treatment.
We used 16S rRNA high-throughput sequencing to determine the changes in gut microbiota of DN rats after SHYS treatment. The abundance and diversity of the microbial communities within the samples were analyzed using alpha diversity (i.e., Shannon index and Simpson index). The results indicated that the Shannon and Simpson indexes did not reveal significant differences in the Model group compared with the Control group. Similarly, there was no significant difference in the Shannon and Simpson indexes in the SHYS high-dose group compared with the Model group (Figures 3A,B). Next, we analyzed the composition of microbial communities of different samples using beta diversity and evaluated them by principal coordinate analysis (PCoA) and cluster analysis. The PCoA analysis was performed based on unweighted distance, in which the higher the similarity of species composition structure between samples, the more clustered in the diagram. Conversely, the lower the similarity between samples, the more distant the linear distance. The PCoA results indicated that sample points in the Model group could be completely separated from those in the Control group, and sample points in the SHYS high-dose group were very close to that of the Control group (Figure 3C). The clustering analysis also showed similar results (Figure 3D). The results indicated that the distance from the Control group to the SHYS high-dose group was closer than that between the Control group and the Model group. The above results indicated that the overall structure and composition of the gut microbiota of DN rats changed significantly and high-dose SHYS effectively reversed this change.
FIGURE 3. SHYS treatment affected the diversity and abundances of gut microbiota in DN rats (A,B) No significant differences were observed among the Control, Model and SHYS high-dose groups. (C,D) PCoA and system clustering tree indicated more similar beta diversity between SHYS high-dose and Control groups than that between the Model and Control groups (C: Control group; M: Model group; S: SHYS high-dose group). (E,F) At the phylum level, SHYS treatment decreased the F to B ratio in DN rats. (G) At the genus level, SHYS treatment affected the relative abundances of Lactobacillus, Ruminococcaceae UCG-005, Allobaculum, Anaerovibrio, Bacteroides and Candidatus_Saccharimonas in DN rats (H,I) The differential metabolic pathways (written in red) of SHYS on DN were predicted using PICRUSt analysis based on the 16S rRNA sequencing data. Control, Model and SHYS high-dose (n = 6 per group) groups. Data are presented as the mean ± SD. #: p < 0.05 as compared to the Control group; ##: p < 0.01 as compared to the Control group; *: p < 0.05 as compared to the Model group; **: p < 0.01 as compared to the Model group
The composition of the gut microbiota in each group of samples at the phylum level indicated that Firmicutes and Bacteroidetes were the dominant species (Figure 3E). The Firmicutes to Bacteroidetes (F to B) ratio was significantly higher in the Model group compared with that in the Control group (p < 0.01), whereas the F to B ratio was significantly lower in the SHYS high-dose group compared with that in the Model group (p < 0.01, Figure 3F). At the genus level, Candidatus_Saccharimonas, Treponema and Desulfovibrio (p < 0.01, p < 0.01, p < 0.05, respectively) were at relatively higher abundances in Model group compared with the Control group, whereas Lactobacillus, Ruminococcaceae UCG-005, Anaerovibrio, Bacteroides and Christensenellaceae_R-7_group (p < 0.01, p < 0.05, p < 0.05, p < 0.01, p < 0.05, respectively) were relatively lower in abundance in Model group compared with the Control group. Lactobacillus, Ruminococcaceae UCG-005, Allobaculum, Anaerovibrio and Bacteroides (p < 0.01, p < 0.01, p < 0.05, p < 0.01, p < 0.05) were relatively more abundant and Candidatus_Saccharimonas (p < 0.05) was relatively less abundant in the SHYS high-dose group compared with the Model group (Figure 3G).
To determine the effect of high-dose SHYS treatment on the function of the gut microbiota in DN rats, bacterial function prediction was performed using PICRUSt analysis. The top 10 metabolic pathways with the highest proportions and with a p value less than 0.05 were listed in Figure 3H (Control group vs Model group) and Figure 3I (Model group vs SHYS high-dose group). Proportions of metabolic pathways that were increased in the Model group compared with Control group but decreased in SHYS high-dose group compared with Model group, or vice versa, were considered as differential pathways, including arachidonic acid metabolism, fructose and mannose metabolism, tricarboxylic acid (TCA) cycle, arginine and proline metabolism tyrosine metabolism, linoleic acid metabolism, and arginine biosynthesis pathways.
Liquid chromatography–mass spectrometry (LC-MS) was used to detect and analyze the sera of animals in each group in both positive- and negative-ion conditions to obtain the total ion current of the metabolites. PCA generates new characteristic variables by linearly combining metabolite variables with certain weights and categorizes the data for each group by new principal variables (principal components). The PCA model reflects the original state of the metabolome data, and the degree of aggregation and dispersion of the samples were observed from the PCA model, where the PCA plots showed that the Control group and the Model group were well-differentiated and the Model group and SHYS high-dose group were also well-differentiated (Figures 4A,B). For the identification of differential metabolites, the model of OPLS-DA was employed, and the explanatory rate (R2) and predictive power (Q2) of the model were evaluated under the established OPLS-DA model. Compared with the Control group, the Model group yielded an R2 = 0.88 and a Q2 = −0.77 (Figures 4C,D). Compared with the Model group, the SHYS high-dose group had an R2 = 0.93 and a Q2 = −0.72 (Figures 4E,F). These results indicate that the model is stable and shows good predictive ability.
FIGURE 4. SHYS treatment modulated the serum metabolites in DN rats (A,B) Scores plots of PCA between the Control and Model groups (A) and between the Model and SHYS high-dose groups (B–D) Scores plots of OPLS-DA between the Control and Model groups and the corresponding coefficient of loading plots. (E,F) Scores plots of OPLS-DA between the Model and SHYS high-dose groups and the corresponding coefficient of loading plots (G,H) Summary of pathway analysis of serum samples between Control and Model groups (G) and between Model and SHYS high-dose groups (H), the sames pathways were written in red. a: Glycerophospholipid metabolism; b: Tryptophan metabolism; c: Alanine, aspartate and glutamate metabolism; d: Arginine biosynthesis; e: TCA cycle; f: Tyrosine metabolism; g: Arginine and proline metabolism; h: Cysteine and methionine metabolism; i: Glycerolipid metabolism; j: Phenylalanine, tyrosine and tryptophan biosynthesis; k: Phenylalanine metabolism. Control, Model and SHYS high-dose (n = 6 per group) groups.
The p < 0.05 and VIP > 1.0 were used on differential metabolites for screening, in which a total of 29 differential metabolites with 13 down-regulated and 16 up-regulated metabolites were screened in the Model group compared with the Control group. A total of 27 differential metabolites including 11 down-regulated and 16 up-regulated metabolites were screened in the SHYS high-dose group compared with the Model group (Table 3). Next, we used MetaboAnalyst software to analyze the metabolic pathways of the identified differential metabolites. Differential pathways were considered with a p-value < 0.05 and pathway impact > 0.1. Metabolic pathways that were altered between Control and Model groups included glycerophospholipid metabolism, tryptophan metabolism, alanine, aspartate and glutamate metabolism, arginine biosynthesis, TCA cycle, tyrosine metabolism, arginine and proline metabolism, cysteine and methionine metabolism, and glycerophospholipid metabolism metabolism pathways (Figure 4G). The metabolic pathways altered between SHYS high-dose and Model groups included glycerophospholipid metabolism, tryptophan metabolism, alanine, aspartate and glutamate metabolism, arginine biosynthesis, TCA cycle, tyrosine metabolism, arginine and proline metabolism, arginine and proline metabolism, phenylalanine, tyrosine and tryptophan biosynthesis, phenylalanine metabolism, and D-glutamine and D-glutamate metabolism pathways (Figure 4H). The same metabolic pathway of PICRUSt analysis that had done 16S rRNA sequencing and untargeted metabolomics pathway analysis included arginine biosynthesis, TCA cycle, tyrosine metabolism, and arginine and proline metabolism and were discussed in detail.
Spearman’s correlation analysis was conducted to analyze the relationship between physiological data, oxidative stress and inflammatory factors, differential serum metabolites and gut microbiota at genus level in the Control, Model and SHYS high-dose groups. As shown in Figure 5, Candidatus_Saccharimona, Ruminococcaceae UCG-005, and Christensenellaceae_R-7_group showed negative correlations and Treponema and Bacteroides showed positive correlations with some of the pro-inflamatory cytokines. Lactobacillus, Candidatus_Saccharimonas, Ruminococcaceae UCG-005, Allobaculum, Anaerovibrio, Bacteroides, and Christensenellaceae_R-7_group showed correlations with some of the oxidative stress factors. In addition, Lactobacillus, Candidatus_Saccharimonas, Ruminococcaceae UCG-005, Anaerovibrio, Bacteroides, and Christensenellaceae_R-7_group were correlated with most of the metabolites (Figure 6).
FIGURE 5. Correlations between between physiological data, oxidative stress and inflammatory factors and gut microbiota were analyzed using spearman’s analysis (heatmap). X-axis represents the gut microbiota with differential abundance. Y-axis represents the physiological data, oxidative stress and inflammatory factors. The colors of grids represent the correlation analysis value of spearman’s correlation analysis. Grids in red indicate positive correlations (correlation analysis value more than 0.1), while grids in blue indicate negative correlations (correlation analysis value less than −0.1). Color coding scale indicates the correlation analysis value from heatmap, the deeper red or blue indicates higher correlation values. *: p < 0.05 between physiological data, oxidative stress and inflammatory factors and gut microbiota. **: p < 0.01 between physiological data, oxidative stress and inflammatory factors and gut microbiota.
FIGURE 6. Correlation analysis of untargeted metabolomics and 16S rRNA sequencing. Correlations between between untargeted metabolomics and gut microbiota were analyzed using spearman’s analysis (heatmap). X-axis represents the differential metabolites in the serum. Y-axis represents the gut microbiota with differential abundance. The colors of grids represent the correlation analysis value of spearman’s correlation analysis. Grids in red indicate positive correlations (correlation analysis value more than 0.1), while grids in blue indicate negative correlations (correlation analysis value less than −0.1). Color coding scale indicates the correlation analysis value from heatmap, the deeper red or blue indicates higher correlation values. *: p < 0.05 between differential serum metabolites and gut microbiota. **: p < 0.01 between differential serum metabolites and gut microbiota.
In the present study, we used a HFD combined with STZ injection to establish a rat model of DN. Compared with the Control group, the rats in the Model group exhibited a significant increase in FBG levels and there were abnormal biochemical indicators related to renal function, as manifested in increased serum Cr and BUN as well as a significant increase in 24-h urine protein levels. In addition, the pathology results revealed that the renal tissues of the DN model rats showed significant tubular atrophy and glomerular hyperplasia along with a certain degree of inflammatory cell infiltration. This is consistent with the pathological manifestations of DN. The SHYS-treated groups exhibited reduced FBG, improved biochemical indicators of renal function, and alleviated renal histopathological changes to varying degrees in rats with DN. This suggests that SHYS exhibits a therapeutic effect on DN, particularly in the high-dose group. In addition, we selected irbesartan as a positive control drug. The results showed that irbesartan had no significant improvement on blood glucose in rats with DN and there was no significant difference in the improvement of renal function and pathological changes between animals in the positive control group and the high-dose SHYS-treated group. These results suggest that SHYS can be used as an alternative therapy to irbesartan for the treatment of DN.
We further examined the effect of SHYS on inflammation in rats with DN. The hyperglycemic state induces chronic inflammation in the body, which contributes to the infiltration of inflammatory cells in renal tissue and the production of large amounts of pro-inflammatory factors including IL-1β, IL6, and TNF-α (Casqueiro et al., 2012). Our results indicated that the level of pro-inflammatory factors in the renal tissues of rats in the Model group were decreased after SHYS treatment suggesting that SHYS exhibits some anti-inflammatory effects.
We investigated the effect of high-dose SHYS on the gut microbiota of rats in DN model using 16S RNA sequencing technology. There were no significant differences in the Shannon and Simpson indexes between the groups, suggesting that the alpha diversity of gut microbiota in the rat model of DN was unchanged. SHYS did not affect the alpha diversity of gut microbiota in rats with DN. However, in the beta diversity analysis, we found significant differences between animals in the Model group and the Control group in the PCoA plot. The beta diversity of gut microbiota in rats from the high-dose SHYS-treated group was significantly different from that of the Model group. Interestingly, the PCoA plot showed that the beta diversity of gut microbiota in rats from the SHYS-treated groups was more similar to that of the Control group compared with the Model group. These results suggest that SHYS restores the beta diversity of gut microbiota in this DN rat model to a level similar to the Control group. Further analysis of the abundance of microbiota revealed that at the phylum level, the primary species of gut microbiota in the rats from each group were Firmicutes and Bacteroides. The rats in the DN model showed an increase in the ratio of F to B, whereas SHYS significantly reduced the F to B ratio. The elevated F to B ratio is closely associated with the inflammatory response, metabolic disorders, and other pathological states in DN (Lee et al., 2019). Further analysis at the genus level revealed that SHYS could increase the abundance of Lactobacillus, Ruminococcaceae UCG-005, Allobaculum, Anaerovibrio and Bacteroides in DN model rats. Lactobacillus is a major probiotic with an important role in the regulation of metabolism and immunity (Zhang et al., 2020). A reduced abundance of Lactobacillus was found in models of diabetes, fatty liver, and obesity (Mishra et al., 2016; Nova et al., 2016). The metabolic and inflammatory responses of the organism can be improved by the transplantation of Lactobacillus. Ruminococcaceae UCG-005, Allobaculum, and Bacteroides produce short-chain fatty acids (SCFAs) by degrading cellulose in food (Zhang et al., 2015; Wu et al., 2020; Xue et al., 2020). SCFAs are important metabolites of the gut microbiota and studies have shown that increasing the SCFA-producing microbiota can significantly improve a number of metabolic diseases, including diabetes and obesity (Yang et al., 2019; Zhang et al., 2019; Birkeland et al., 2020; Tian et al., 2021). In addition, recent studies have shown that SCFAs inhibit the inflammatory responses of renal tissue in DN through G protein-coupled receptors (GPR43 and GPR109A) (Li et al., 2020a). Anaerovibrio is capable of catabolizing lipids. Studies have shown that polyphenols in green tea can alleviate chronic inflammation caused by translocation of gut microbiota in an obesity model, while increasing the amount of Anaerovibrio in the gut. However, the specific relationship of Anaerovibrio to DN remains unknown (Li et al., 2020b). SHYS treatment also caused a decrease of Candidatus_Saccharimonas in gut. Candidatus_Saccharimonas is a conditional pathogenic bacterium that is significantly elevated in the gut of gout patients (Shao et al., 2017). In addition, green tea leaf powder ameliorates the abnormal lipid metabolism induced by a HFD, while reducing the abundance of Candidatus_Saccharimonas in the gut (Li et al., 2020b). Likewise, our correlation analysis also showed negative correlations of Lactobacillus, Candidatus_Saccharimonas, Ruminococcaceae UCG-005, Allobaculum, Anaerovibrio, Bacteroides, and Christensenellaceae_R-7_grou with some of the physiological indicies (FBG, Cr, BUN, body weight and Urine Protein), oxidative stress factor (MDA) and pro-inflammatory cytokines (IL-1β, IL-6, TNF-α). Correlation analysis also showed positive correlations of Treponema and Desulfovibrio with physiological indicies (BUN, Urine Protein) and pro-inflammatory cytokine (IL-6). Further studies should also be carried out using fecal transplantation or gut microbiota depletion models to verify whether SHYS can ameliorate hyperglycemia, hyperlipidemia, IR, oxidative stress and inflammatory responses to treat DN through regulating these gut microbiota.
PCA and OPLS-DA of serum untargeted metabolomics revealed that the metabolites of DN in the rat model were significantly different from those of normal rats. In addition, the serum metabolite levels of rats after SHYS treatment were also significantly different from those of DN rats. Metabolic pathway analysis for differential metabolites using MetaboAnalyst showed that SHYS had an impact on several metabolic pathways including arginine and proline metabolism, alanine, aspartate and glutamate metabolism, arginine biosynthesis, TCA cycle, tyrosine metabolism, and glycerophospholipid metabolism. After correlating the differential metabolic pathways obtained from metabolomics with those deduced from 16s rRNA sequencing, the pathways of arginine biosynthesis, TCA cycle, tyrosine metabolism, and arginine and proline metabolism were the most common, suggesting that SHYS may play a role in the treatment of DN by regulating gut microbiota, which in turn affects arginine biosynthesis, the TCA cycle, tyrosine metabolism, and arginine and proline metabolism.
Amino acid metabolism is closely related to metabolic disorders during DN. In the present study, we found that the level of guanidineacetic acid and L-glutamic acid were elevated in DN model rats and significantly decreased after treatment with SHYS. The level of creatine, D-proline, and L-glutamine were decreased and significantly increased after treated with SHYS. Arginine is the largest nitrogen-donating amino acid and is a precursor to proline and creatine (Ge et al., 2020). Proline metabolizes and generates electrons as well as reactive oxygen species, leading to a variety of downstream effects including blocking the cell cycle, autophagy, and apoptosis (Selen et al., 2015). Creatine is a naturally produced nitrogen-containing molecule that promotes the ATP cycle and provides energy to muscles and cells (Arlin et al., 2014). Creatine may reduce damage by inhibiting inflammation, oxidative stress, and aging (Deminice et al., 2016; Aljobaily et al., 2020). Studies have found that supplementation of creatine to diabetic patients results in hypoglycemic effects (Gualano et al., 2011). Guanidineacetic acid is an immediate precursor of creatine biosynthesis, however, this reaction causes elevated levels of homocysteine in the blood. This can result in vasculopathy, which is one of the causes of diabetic macrovascular and microvascular complications in diabetic patients (De Luis et al., 2005; Zhao et al., 2018; Ma et al., 2020). L-glutamic acid and L-glutamine are interconvertible. L-glutamine is an abundant free amino acid inside and outside of human cells that plays an essential role in protein and energy metabolism. It is a precursor substance for the synthesis of amino acids, proteins, and nucleic acids (Tanaka et al., 2015; Kou et al., 2016). Paul et al. found that L-glutamine is effective at resisting obesity and insulin tolerance (Petrus et al., 2020). L-glutamine provides energy to immune cells, such as lymphocytes and macrophages, ensuring immune cell proliferation, and enhancing immunity (Csibi et al., 2014). Also, L-glutamine has anti-inflammatory and antioxidant effects. L-glutamine is a precursor substance for glutathione synthesis and reduces the inflammatory response by increasing glutathione synthesis, inhibiting the NF-κB pathway, and decreasing the levels of IL-8 and TNF-α (Pusapati et al., 2016). Interestingly, the ratio of glutamine to glutamic acid is strongly associated with diabetes and insulin resistance. An elevated ratio is significantly associated with a low risk of developing diabetes (Cheng et al., 2012; Rhee et al., 2018), which primarily occurs through various cell related metabolic events, such as protein synthesis, muscle growth, uropoiesis in the liver, insulin secretion, hepatic and renal gluconeogenesis, neurotransmitter synthesis and glutathione production (Newsholme et al., 2003). A Spearman analysis revealed that guanidineacetic acid was positively correlated with Allobaculum and negatively correlated with Desulfovibrio; D-Proline was positively correlated with Lactobacillus, Ruminococcaceae UCG-005, Anaerovibrio, Bacteroides and Christensenellaceae_R-7_group and negatively correlated with Candidatus_Saccharimona; creatine was positively correlated with Lactobacillus, Bacteroides and Christensenellaceae_R-7_group; and L-glutamic acid was positively correlated with Anaerovibrio. Therefore, we hypothesize that the effect of SHYS on arginine biosynthesis and arginine and proline metabolism may be related to the regulation of the abundance of Allobaculum, Desulfovibrio, Lactobacillus, Ruminococcaceae UCG- 005, Anaerovibrio, Bacteroides, Candidatus_Saccharimona, and Christensenellaceae_R-7_group abundance.
The TCA cycle is the final metabolic pathway for carbohydrates, lipids, and amino acids (Chen et al., 2014). It also affects the immune system and is closely associated with various metabolic diseases, including diabetes (Choi et al., 2021). In the present study, we found that fumaric acid was significantly elevated in the DN rat model and decreased after SHYS administration, whereas alpha-ketoglutaric acid was increased after SHYS administration. Fumaric acid is a dicarboxylic acid that is produced by succinic dehydrogenase from precursor adenosine in the TCA cycle and is converted to malic acid by fumarate hydratase (You et al., 2015). The metabolic disorder of fumaric acid may be associated with renal impairment in diabetes, in which its accumulation leads to oxidative stress and prolonged oxidative stress leads to kidney injury in DN (Zheng et al., 2015). Studies have found that the accumulation of fumaric acid is positively associated with progression toward DN in patients (Liu, et al., 2018). Elevated levels of fumaric acid in DN causes endoplasmic reticulum stress and HIF-1α expression, steering metabolism to the glycolytic pathways and leading to pathological renal injury (Isaacs et al., 2005; Tong et al., 2011; Linehan and Rouault, 2013). Alpha-ketoglutaric acid is a key intermediate in the TCA cycle located between succinyl-coenzyme A and isocitric acid. As a key member of the anaplerotic reactions, alpha-ketoglutaric acid regulates ATP production and reduces NAD+/NADH production in the TCA cycle, thereby affecting ROS levels and immune system homeostasis (B Zdzisińska et al., 2017). In addition, alpha-ketoglutaric acid is an important source of glutamine and glutamate, which are required for the synthesis of amino acids and collagen (Xiao et al., 2016). It was found that alpha-ketoglutaric acid supplementation protects mice from myocardial ischemia reperfusion injury (Olenchock et al., 2016). A Spearman analysis showed that fumaric acid was positively correlated with Candidatus_ Saccharimonas and Desulfovibrio, and negatively correlated with Lactobacillus and Anaerovibrio. No bacterial was found significantly corrected with alpha-ketoglutaric acid. Therefore, we hypothesized that the effect of SHYS on the TCA cycle may be associated with the regulating the abundance of Candidatus_Saccharimonas, Desulfovibri, Lactobacillus, and Anaerovibrio.
Tyrosine metabolism is associated with a variety of diseases including fatty liver, insulin resistance, and obesity (Gaggini et al., 2018; Gitto et al., 2018). In the present study, we found that L-tyrosine was significantly elevated in rats of the model group and decreased after the administration of SHYS. Therefore, high levels of tyrosine may promote fatty acid synthesis, which further promotes fat deposition in the liver (Jin et al., 2016). Meanwhile, the characteristic nitrification of tyrosine residues in proteins using peroxynitrite (ONOO-) produces nitrotyrosine (NT). NT is involved in the development of diabetes and its complications and elevated levels of NT can disrupt renal pathology and cause renal dysfunction in diabetic rats (Chew et al., 2010). Increased levels of NT have also been observed in patients with DN (Julius et al., 2008; Castro et al., 2011). NT induces glomerular mesangial cells to express NF-κB, MCP-1, and TGF-β1, causing inflammatory injury and further aggravating nephropathy (Jing et al., 2018). In addition, NT is also a marker of oxidative stress in diabetic patients and NT activates the ERK pathway to increase iNOS and produce excess ROS to mediate oxidative stress damage in the body (Inoguchi and Takayanagi, 2008). A Spearman analysis showed that L-tyrosine was positively correlated with Candidatus_Saccharimonas and Treponema, and negatively correlated with Lactobacillus, Ruminococcaceae UCG-005, Allobaculum, and Bacteroides. Therefore, we speculated that the effect of SHYS on the tyrosine metabolism may be with the regulation of the abundance of Candidatus_Saccharimonas, Treponema, Lactobacillus, Ruminococcaceae UCG-005, Allobaculum, and Bacteroides.
In conclusion, our study revealed multiple ameliorative effects of SHYS on DN including the alleviation of hyperglycemia and the improvement of renal function, pathological changes in kidney, oxidative stress, and the inflammatory response. The mechanism of SHYS on DN may be related to the improvement of gut microbiota which regulates arginine biosynthesis, TCA cycle, tyrosine metabolism, and arginine and proline metabolism.
The datasets presented in this study can be found in online repositories. The names of the repository/repositories and accession number(s) can be found below: NCBI (accession: PRJNA778921).
The animal study was reviewed and approved by the study was approved by the Ethics Committee of Cangzhou Hospital of Integrated Traditional Chinese and Western Medicine.
XS carried out the experiments and manuscript writing. WY, AL, and CW provided experimental help, and performed data analysis and result interpretation. XLi, JG, and XLiu finished molecular bioassays. WJ, YY, and SL provided ideas and technical guidance for the whole work. All authors contributed to the article and approved the submitted version.
This work was supported by the Scientific Program Project of Administration of Traditional Chinese Medicine in Hebei Province (No. 2021311).
The authors declare that the research was conducted in the absence of any commercial or financial relationships that could be construed as a potential conflict of interest.
All claims expressed in this article are solely those of the authors and do not necessarily represent those of their affiliated organizations, or those of the publisher, the editors and the reviewers. Any product that may be evaluated in this article, or claim that may be made by its manufacturer, is not guaranteed or endorsed by the publisher.
The Supplementary Material for this article can be found online at: https://www.frontiersin.org/articles/10.3389/fphar.2021.808867/full#supplementary-material
Aljobaily, N., Viereckl, M. J., Hydock, D. S., Aljobaily, H., Wu, T.-Y., Busekrus, R., et al. (2020). Creatine Alleviates Doxorubicin-Induced Liver Damage by Inhibiting Liver Fibrosis, Inflammation, Oxidative Stress, and Cellular Senescence. Nutrients 13, 41. doi:10.3390/nu13010041
Arlin, J.-B., Bhardwaj, R. M., Johnston, A., Miller, G. J., Bardin, J., Macdougall, F., et al. (2014). Structure and Stability of Two Polymorphs of Creatine and its Monohydrate. Crystengcomm 16, 8197–8204. doi:10.1039/C4CE00508B
Bian, S. S., Zhuang, K. S., Zhang, F. G., Wang, F., Li, L. Z., Jiang, X., et al. (2011). Effect of Sanhuang Yishen Capsule on Microinflammatory State in Patients with Maintenance Hemodialysis. Chin. Arch. Tradit. Chin. Med. 12, 57–58. doi:10.13457/j.cnki.jncm.2011.12.025
Birkeland, E., Gharagozlian, S., Birkeland, K. I., Valeur, J., Måge, I., Rud, I., et al. (2020). Correction to: Prebiotic Effect of Inulin-type Fructans on Faecal Microbiota and Short-chain Fatty Acids in Type 2 Diabetes: a Randomised Controlled Trial. Eur. J. Nutr. 59, 3339–3340. doi:10.1007/s00394-020-02282-5
Cai, T. T., Ye, X. L., Li, R. R., Chen, H., Wang, Y. Y., Yong, H. J., et al. (2020). Resveratrol Modulates the Gut Microbiota and Inflammation to Protect against Diabetic Nephropathy in Mice. Front. Pharmacol. 11, 1249. doi:10.3389/fphar.2020.01249
Casqueiro, J., Casqueiro, J., and Alves, C. (2012). Infections in Patients with Diabetes Mellitus: A Review of Pathogenesis. Indian J. Endocrinol. Metab. 16 (Suppl. 1), S27–S36. doi:10.4103/2230-8210.94253
Castro, L., Demicheli, V., Tórtora, V., and Radi, R. (2011). Mitochondrial Protein Tyrosine Nitration. Free Radic. Res. 45, 37–52. doi:10.3109/10715762.2010.516254
Chen, L., Liu, T., Zhou, J., Wang, Y., Wang, X., Di, W., et al. (2014). Citrate Synthase Expression Affects Tumor Phenotype and Drug Resistance in Human Ovarian Carcinoma. PLoS One 9, e115708. doi:10.1371/journal.pone.0115708
Cheng, S., Rhee, E. P., Larson, M. G., Lewis, G. D., Mccabe, E. L., Shen, D., et al. (2012). Metabolite Profiling Identifies Pathways Associated with Metabolic Risk in Humans. Circulation 125, 2222–2231. doi:10.1161/CIRCULATIONAHA.111.067827
Chew, P., Yuen, D. Y., Stefanovic, N., Pete, J., Coughlan, M. T., Jandeleit-Dahm, K. A., et al. (2010). Antiatherosclerotic and Renoprotective Effects of Ebselen in the Diabetic Apolipoprotein E/GPx1-double Knockout Mouse. Diabetes 59, 3198–3207. doi:10.2337/db10-0195
Chi, X. E., Lv, S. Q., Wang, Y. S., Wang, X., Su, X., Jia, C., et al. (2013). Protective Effect on Kidney and Mechanism of Sanhuang Yishen Capsule in Rats with Diabetes. J. Liaoning Univ. Tradit Chin. Med. 15, 41–43.
Choi, I., Son, H., and Baek, J.-H. (2021). Tricarboxylic Acid (TCA) Cycle Intermediates: Regulators of Immune Responses. Life 11, 69. doi:10.3390/life11010069
Csibi, A., Lee, G., Yoon, S. O., Tong, H., Ilter, D., Elia, I., et al. (2014). The mTORC1/S6K1 Pathway Regulates Glutamine Metabolism through the eIF4B-dependent Control of C-Myc Translation. Curr. Biol. 24, 2274–2280. doi:10.1016/j.cub.2014.08.007
De Luis, D. A., Fernandez, N., Arranz, M. L., Aller, R., Izaola, O., and Romero, E. (2005). Total Homocysteine Levels Relation with Chronic Complications of Diabetes, Body Composition, and Other Cardiovascular Risk Factors in a Population of Patients with Diabetes Mellitus Type 2. J. Diabetes Complications 19, 42–46. doi:10.1016/j.jdiacomp.2003.12.003
Deminice, R., Cella, P. S., Padilha, C. S., Borges, F. H., da Silva, L. E., Campos-Ferraz, P. L., et al. (2016). Creatine Supplementation Prevents Hyperhomocysteinemia, Oxidative Stress and Cancer-Induced Cachexia Progression in Walker-256 Tumor-Bearing Rats. Amino Acids 48, 2015–2024. doi:10.1007/s00726-016-2172-9
Edgar, R. C. (2004). MUSCLE: Multiple Sequence Alignment with High Accuracy and High Throughput. Nucleic Acids Res. 32, 1792–1797. doi:10.1093/nar/gkh340
Edgar, R. C. (2013). UPARSE: Highly Accurate OTU Sequences from Microbial Amplicon Reads. Nat. Methods 10, 996–998. doi:10.1038/nmeth.2604
Gaggini, M., Carli, F., Rosso, C., Buzzigoli, E., Marietti, M., Della Latta, V., et al. (2018). Altered Amino Acid Concentrations in NAFLD: Impact of Obesity and Insulin Resistance. Hepatology 67, 145–158. doi:10.1002/hep.29465
Ge, S., Zhang, Q., Tian, Y., Hao, L., Duan, J., and Zhang, B. (2020). Cell Metabolic Profiling of Colorectal Cancer via 1H NMR. Clin. Chim. Acta 510, 291–297. doi:10.1016/j.cca.2020.07.039
Gitto, S., Schepis, F., Andreone, P., and Villa, E. (2018). Study of the Serum Metabolomic Profile in Nonalcoholic Fatty Liver Disease: Research and Clinical Perspectives. Metabolites 8, 17. doi:10.3390/metabo8010017
Gong, X., Xiong, L., Bi, C., and Zhang, B. (2021). Diosmetin Ameliorate Type 2 Diabetic Mellitus by Up-Regulating Corynebacterium Glutamicum to Regulate IRS/PI3K/AKT-mediated Glucose Metabolism Disorder in KK-Ay Mice. Phytomedicine 87, 153582. doi:10.1016/j.phymed.2021.153582
Grigor’eva, I. N. (2020). Gallstone Disease, Obesity and the Firmicutes/Bacteroidetes Ratio as a Possible Biomarker of Gut Dysbiosis. Jpm 11, 13. doi:10.3390/jpm11010013
Gualano, B., De Salles Painneli, V., Roschel, H., Artioli, G. G., Neves, M., De Sá Pinto, A. L., et al. (2011). Creatine in Type 2 Diabetes: a Randomized, Double-Blind, Placebo-Controlled Trial. Med. Sci. Sports Exerc. 43, 770–778. doi:10.1249/mss.0b013e3181fcee7d
Guarner, F. (2006). Enteric flora in Health and Disease. Digestion 73 (Suppl. 1), 5–12. doi:10.1159/000089775
Guo, Z. A., Yu, C. J., Liu, G., Meng, F. C., Li, Y., and Peng, S. L. (2014). Treatment of Stage 3b Diabetic Kidney Disease Patients with Macroalbuminuria by Qizhi Jiangtang Capsule: a Multicenter Randomized Control Clinical Study. Zhongguo Zhong Xi Yi Jie He Za Zhi 34, 1047–1052. doi:10.1016/s0254-6272(15)30043-1
Inoguchi, T., and Takayanagi, R. (2008). Role of Oxidative Stress in Diabetic Vascular Complications. Fukuoka Igaku Zasshi 99, 47–55. doi:10.2337/diacare.19.3.257
Isaacs, J. S., Jung, Y. J., Mole, D. R., Lee, S., Torres-Cabala, C., Chung, Y. L., et al. (2005). HIF Overexpression Correlates with Biallelic Loss of Fumarate Hydratase in Renal Cancer: Novel Role of Fumarate in Regulation of HIF Stability. Cancer Cell 8, 143–153. doi:10.1016/j.ccr.2005.06.017
Jin, H., Piao, S. G., Jin, J. Z., Jin, Y. S., Cui, Z. H., Jin, H. F., et al. (2014). Synergistic Effects of Leflunomide and Benazepril in Streptozotocin-Induced Diabetic Nephropathy. Nephron Exp. Nephrol. 126, 148–156. doi:10.1159/000362556
Jin, R., Banton, S., Tran, V. T., Konomi, J. V., Li, S., Jones, D. P., et al. (2016). Amino Acid Metabolism Is Altered in Adolescents with Nonalcoholic Fatty Liver Disease-An Untargeted, High Resolution Metabolomics Study. J. Pediatr. 172, 14–19. doi:10.1016/j.jpeds.2016.01.026
Jing, W., Jabbari, B., and Vaziri, N. D. (2018). Uremia Induces Upregulation of Cerebral Tissue Oxidative/inflammatory cascade, Down-Regulation of Nrf2 Pathway and Disruption of Blood Brain Barrier. Am. J. Transl Res. 10, 2137–2147. Available at https://escholarship.org/uc/item/9qv048rm.
Julius, U., Drel, V., Gräßler, J., Obrosova, I., Schmidt, W. E., and Meier, J. J. (2008). Nitrosylated Proteins in Monocytes as a New Marker of Oxidative-Nitrosative Stress in Diabetic Subjects with Macroangiopathy. Exp. Clin. Endocrinol. Diabetes 117, 72–77. doi:10.1055/s-2008-1078710
Kou, Y., Zheng, W. T., and Zhang, Y. R. (2016). Inhibition of miR-23 Protects Myocardial Function from Ischemia-Reperfusion Injury through Restoration of Glutamine Metabolism. Eur. Rev. Med. Pharmacol. Sci. 20, 4286–4293. Available at https://www.europeanreview.org/article/11599.
Lee, H. C., Yu, S. C., Lo, Y. C., Lin, I. H., Tung, T. H., and Huang, S. Y. (2019). A High Linoleic Acid Diet Exacerbates Metabolic Responses and Gut Microbiota Dysbiosis in Obese Rats with Diabetes Mellitus. Food Funct. 10, 786–798. doi:10.1039/C8fo02423e
Li, S., He, Y., Zhang, H., Zheng, R., Xu, R., Liu, Q., et al. (2020). Formulation of Traditional Chinese Medicine and its Application on Intestinal flora of Constipated Rats. Microb. Cel Fact 19, 212. doi:10.1186/s12934-020-01473-3
Li, Y., Chen, X., Kwan, T., Loh, Y., Singer, J., Liu, Y., et al. (2020a). Sat-160 Dietary Fibre and Bacterial Scfa Modulate Renal Inflammation in Diabetic Nephropathy through Activation of G-Protein Coupled Receptors Gpr43 and Gpr109a. Kidney Int. Rep. 5, S68–S69. doi:10.1016/j.ekir.2020.02.170
Li, Y., Rahman, S. U., Huang, Y., Zhang, Y., Ming, P., Zhu, L., et al. (2020b). Green tea Polyphenols Decrease Weight Gain, Ameliorate Alteration of Gut Microbiota, and Mitigate Intestinal Inflammation in Canines with High-Fat-Diet-Induced Obesity. J. Nutr. Biochem. 78, 108324. doi:10.1016/j.jnutbio.2019.108324
Li, Y. J., Chen, X., Kwan, T. K., Loh, Y. W., Singer, J., Liu, Y., et al. (2020). Dietary Fiber Protects Against Diabetic Nephropathy Through Short-Chain Fatty Acid-Mediated Activation of G Protein-Coupled Receptors GPR43 and GPR109A. J. Am. Soc. Nephrol. 31, 1267–1281. doi:10.1681/ASN.2019101029
Linehan, W. M., and Rouault, T. A. (2013). Molecular Pathways: Fumarate Hydratase-Deficient Kidney Cancer-Ttargeting the Warburg Effect in Cancer. Clin. Cancer Res. 19, 3345–3352. doi:10.1158/1078-0432.CCR-13-0304
Liu, I. M., Tzeng, T. F., Liou, S. S., and Chang, C. J. (2009). The Amelioration of Streptozotocin Diabetes-Induced Renal Damage by Wu-Ling-San (Hoelen Five Herb Formula), a Traditional Chinese Prescription. J. Ethnopharmacol. 124, 211–218. doi:10.1016/j.jep.2009.04.021
Liu, J. J., Liu, S., Gurung, R. L., Ching, J., Kovalik, J. P., Tan, T. Y., et al. (2018). Urine Tricarboxylic Acid Cycle Metabolites Predict Progressive Chronic Kidney Disease in Type 2 Diabetes. J. Clin. Endocrinol. Metab. 103, 4357–4364. doi:10.1210/jc.2018-00947
Ma, Y., Zhou, H., Li, C., Zou, X., Luo, X., Wu, L., et al. (2020). Differential Metabolites in Chinese Autistic Children: A Multi-Center Study Based on Urinary 1H-NMR Metabolomics Analysis. Front. Psychiatry 12, 624767. doi:10.3389/fpsyt.2021.624767
Macho-González, A., Garcimartín, A., Redondo, N., Cofrades, S., Bastida, S., Nova, E., et al. (2021). Carob Fruit Extract-Enriched Meat, as Preventive and Curative Treatments, Improves Gut Microbiota and Colonic Barrier Integrity in a Late-Stage T2DM Model. Food Res. Int. 141, 110124. doi:10.1016/j.foodres.2021.110124
Magne, F., Gotteland, M., Gauthier, L., Zazueta, A., Pesoa, S., Navarrete, P., et al. (2020). The Firmicutes/Bacteroidetes Ratio: A Relevant Marker of Gut Dysbiosis in Obese Patients? Nutrients 12, E1474. doi:10.3390/nu12051474
Mann, J. F., Green, D., Jamerson, K., Ruilope, L. M., Kuranoff, S. J., Littke, T., et al. (2010). Avosentan for Overt Diabetic Nephropathy. J. Am. Soc. Nephrol. 21, 527–535. doi:10.1681/ASN.2009060593
Mishra, A. K., Dubey, V., and Ghosh, A. R. (2016). Obesity: An Overview of Possible Role(s) of Gut Hormones, Lipid Sensing and Gut Microbiota. Metabolism 65, 48–65. doi:10.1016/j.metabol.2015.10.008
Newsholme, P., Procopio, J., Lima, M. M., Pithon-Curi, T. C., and Curi, R. (2003). Glutamine and Glutamate-Ttheir central Role in Cell Metabolism and Function. Cell Biochem. Funct. 21, 1–9. doi:10.1002/cbf.1003
Nova, E., Pérez de Heredia, F., Gómez-Martínez, S., and Marcos, A. (2016). The Role of Probiotics on the Microbiota: Effect on Obesity. Nutr. Clin. Pract. 31, 387–400. doi:10.1177/0884533615620350
Olenchock, B. A., Moslehi, J., Baik, A. H., Davidson, S. M., Williams, J., Gibson, W. J., et al. (2016). EGLN1 Inhibition and Rerouting of α-Ketoglutarate Suffice for Remote Ischemic Protection. Cell 165, 497–895. doi:10.1016/j.cell.2016.02.006
Pazdro, R., and Burgess, J. R. (2010). The Role of Vitamin E and Oxidative Stress in Diabetes Complications. Mech. Ageing Dev. 131, 276–286. doi:10.1016/j.mad.2010.03.005
Petrus, P., Lecoutre, S., Dollet, L., Wiel, C., Sulen, A., Gao, H., et al. (2020). Glutamine Links Obesity to Inflammation in Human White Adipose Tissue. Cell Metab 31, 375–e11. doi:10.1016/j.cmet.2019.11.019
Pusapati, R. V., Daemen, A., Wilson, C., Sandoval, W., Gao, M., Haley, B., et al. (2016). mTORC1-Dependent Metabolic Reprogramming Underlies Escape from Glycolysis Addiction in Cancer Cells. Cancer Cell 29, 548–562. doi:10.1016/j.ccell.2016.02.018
Quast, C., Pruesse, E., Yilmaz, P., Gerken, J., Schweer, T., Yarza, P., et al. (2012). The SILVA Ribosomal RNA Gene Database Project: Improved Data Processing and Web-Based Tools. Nucleic Acids Res. 41, D590–D596. doi:10.1093/nar/gks1219
Rhee, S. Y., Jung, E. S., Park, H. M., Jeong, S. J., Kim, K., Chon, S., et al. (2018). Plasma Glutamine and Glutamic Acid Are Potential Biomarkers for Predicting Diabetic Retinopathy. Metabolomics 14, 89. doi:10.1007/s11306-018-1383-3
Selen, E. S., Bolandnazar, Z., Tonelli, M., Bütz, D. E., Haviland, J. A., Porter, W. P., et al. (2015). NMR Metabolomics Show Evidence for Mitochondrial Oxidative Stress in a Mouse Model of Polycystic Ovary Syndrome. J. Proteome Res. 14, 3284–3291. doi:10.1021/acs.jproteome.5b00307
Shao, T., Shao, L., Li, H., Xie, Z., He, Z., and Wen, C. (2017). Combined Signature of the Fecal Microbiome and Metabolome in Patients with Gout. Front. Microbiol. 8, 268. doi:10.3389/fmicb.2017.00268
Shen, Y. L., Jiang, Y. P., Li, X. Q., Wang, S. J., Ma, M. H., Zhang, C. Y., et al. (2020). ErHuang Formula Improves Renal Fibrosis in Diabetic Nephropathy Rats by Inhibiting CXCL6/JAK/STAT3 Signaling Pathway. Front. Pharmacol. 10, 1596. doi:10.3389/fphar.2019.01596
Song, X. Y., Chen, Q., and Qi, X. Y. (2004). Effect of Liuwei Dihuang Pill on Erythrocyte Aldose Reductase Activity in Early Diabetic Nephropathy Patients. Zhongguo Zhong Xi Yi Jie He Za Zhi 24, 1087–1090. Available at https://pubmed.ncbi.nlm.nih.gov/15658651/.
Su, X. H., Lv, S. Q., Wang, X. Y., and Tian, F. S. (2015a). Clinical Observation of Sanhuang Yishen Capsule to Early Diabetic Nephropathy. J. Liaoning Univer TCM 13, 18–19. Available at http://en.cnki.com.cn/Article_en/CJFDTOTAL-LZXB201101008.htm.
Su, X. H., Lv, S. Q., Zhang, S. F., Li, S. L., Yu, W. X., Guo, R. Q., et al. (2015b). Sanhuang Yishen Capsule Combined with Epalrestat in the Treatment of Early Diabetes Clinical Observation of Nephropathy. J. Basic Chin. Med. 21, 1169–1170. doi:10.3969/j.issn.1674-7860.2013.19.003
Tanaka, K., Sasayama, T., Irino, Y., Takata, K., Nagashima, H., Satoh, N., et al. (2015). Compensatory Glutamine Metabolism Promotes Glioblastoma Resistance to mTOR Inhibitor Treatment. J. Clin. Invest. 125, 1591–1602. doi:10.1172/jci78239
Tang, D. Y., Guo, S. S., and Sun, R. Y. (2005). Effect of Xianzhen Tablet on Content of Advanced Glycosylation End Products (AGEs) and mRNA Expression of AGE-specific Cellular Receptor in Renal Cortex of Diabetic Rats. Zhongguo Zhong Xi Yi Jie He Za Zhi 25, 60–63. Available at https://pubmed.ncbi.nlm.nih.gov/15719755/.
Tian, B., Zhao, J., Zhang, M., Chen, Z., Ma, Q., Liu, H., et al. (2021). Lycium Ruthenicum Anthocyanins Attenuate High-Fat Diet-Induced Colonic Barrier Dysfunction and Inflammation in Mice by Modulating the Gut Microbiota. Mol. Nutr. Food Res. 65, e2000745. doi:10.1002/mnfr.202000745
Tone, A., Shikata, K., Sasaki, M., Ohga, S., Yozai, K., Nishishita, S., et al. (2005). Erythromycin Ameliorates Renal Injury via Anti-inflammatory Effects in Experimental Diabetic Rats. Diabetologia 48, 2402–2411. doi:10.1007/s00125-005-1945-6
Tong, W. H., Sourbier, C., Kovtunovych, G., Jeong, S. Y., Vira, M., Ghosh, M., et al. (2011). The Glycolytic Shift in Fumarate-Hydratase-Deficient Kidney Cancer Lowers AMPK Levels, Increases Anabolic Propensities and Lowers Cellular Iron Levels. Cancer Cell 20, 315–327. doi:10.1016/j.ccr.2011.07.018
Tuttle, K. R., Brosius, F. C., Adler, S. G., Kretzler, M., Mehta, R. L., Tumlin, J. A., et al. (2018). JAK1/JAK2 Inhibition by Baricitinib in Diabetic Kidney Disease: Results from a Phase 2 Randomized Controlled Clinical Trial. Nephrol. Dial. Transpl. 33, 1950–1959. doi:10.1093/ndt/gfx377
Wang, D., Guan, M. P., Zheng, Z. J., Li, W. Q., Lyv, F. P., Pang, R. Y., et al. (2015). Transcription Factor Egr1 Is Involved in High Glucose-Induced Proliferation and Fibrosis in Rat Glomerular Mesangial Cells. Cell. Physiol. Biochem. 36, 2093–2107. doi:10.1159/000430177
Wang, F. L., Wang, Y. H., Han, L., An, H. Y., Zhang, J. H., Zhang, X. Y., et al. (2018). Renoprotective Effect of Yiqi Yangyin Huayu Tongluo Formula against Diabetic Nephropathy in Diabetic Rats. Evid. Based Complement. Alternat Med. 2018, 4276052. doi:10.1155/2018/4276052
Wang, H., Zhang, H., Chen, X., Zhao, T., Kong, Q., Yan, M., et al. (2016). The Decreased Expression of Electron Transfer Flavoprotein β Is Associated with Tubular Cell Apoptosis in Diabetic Nephropathy. Int. J. Mol. Med. 37, 1290–1298. doi:10.3892/ijmm.2016.2533
Wu, G., Shi, Y., Han, L., Feng, C., Ge, Y., Yu, Y., et al. (2020). Dietary Methionine Restriction Ameliorated Fat Accumulation, Systemic Inflammation, and Increased Energy Metabolism by Altering Gut Microbiota in Middle-Aged Mice Administered Different Fat Diets. J. Agric. Food Chem. 68, 7745–7756. doi:10.1021/acs.jafc.0c02965
Wu, Y. L., Wei, C., and Wang, H. T. (2007). Effect of Tongshenluo Capsule on the Components of Extracellular Matrix and Their Metabolism in Kidney of Rats with Diabetic Nephropathy. Zhongguo Zhong Xi Yi Jie He Za Zhi 27, 326–330. Available at https://pubmed.ncbi.nlm.nih.gov/17526172/.
Xiao, D., Zeng, L., Yao, K., Kong, X., Wu, G., and Yin, Y. (2016). The Glutamine-Alpha-Ketoglutarate (AKG) Metabolism and its Nutritional Implications. Amino Acids 48, 2067–2080. doi:10.1007/s00726-016-2254-8
Xue, H., Zhang, M., Ma, J., Chen, T., Wang, F., and Tang, X. (2020). Lactose-Induced Chronic Diarrhea Results from Abnormal Luminal Microbial Fermentation and Disorder of Ion Transport in the Colon. Front. Physiol. 11, 877. doi:10.3389/fphys.2020.00877
Yang, Y., Zhang, Y., Xu, Y., Luo, T., Ge, Y., Jiang, Y., et al. (2019). Dietary Methionine Restriction Improves the Gut Microbiota and Reduces Intestinal Permeability and Inflammation in High-Fat-Fed Mice. Food Funct. 10, 5952–5968. doi:10.1039/c9fo00766k
You, Y. H., Quach, T., Saito, R., Pham, J., and Sharma, K. (2015). Metabolomics Reveals a Key Role for Fumarate in Mediating the Effects of NADPH Oxidase 4 in Diabetic Kidney Disease. J. Am. Soc. Nephrol. 27, 466–481. doi:10.1681/ASN.2015030302
Zdzisińska, B., Żurek, A., and Kandefer-Szerszeń, M. (2017). Alpha-Ketoglutarate as a Molecule with Pleiotropic Activity: Well-Known and Novel Possibilities of Therapeutic Use. Arch. Immunol. Ther. Exp. (Warsz) 65, 21–36. doi:10.1007/s00005-016-0406-x
Zhang, B., Li, G., Shahid, M. S., Gan, L., Fan, H., Lv, Z., et al. (2020). Dietary L-Arginine Supplementation Ameliorates Inflammatory Response and Alters Gut Microbiota Composition in Broiler Chickens Infected with Salmonella enterica Serovar Typhimurium. Poult. Sci. 99, 1862–1874. doi:10.1016/j.psj.2019.10.049
Zhang, L., Shi, M., Ji, J., Hu, X., and Chen, F. (2019). Gut Microbiota Determines the Prevention Effects of Luffa Cylindrica (L.) Roem Supplementation against Obesity and Associated Metabolic Disorders Induced by High-Fat Diet. FASEB J. 33, 10339–10352. doi:10.1096/fj.201900488R
Zhang, M. H., Feng, L., Zhu, M. M., Gu, J. F., Jiang, J., Cheng, X. D., et al. (2014). The Anti-inflammation Effect of Moutan Cortex on Advanced Glycation End Products-Induced Rat Mesangial Cells Dysfunction and High-Glucose-Fat Diet and Streptozotocin-Induced Diabetic Nephropathy Rats. J. Ethnopharmacol. 151, 591–600. doi:10.1016/j.jep.2013.11.015
Zhang, X., Zhao, Y., Xu, J., Xue, Z., Zhang, M., Pang, X., et al. (2015). Modulation of Gut Microbiota by Berberine and Metformin during the Treatment of High-Fat Diet-Induced Obesity in Rats. Sci. Rep. 5, 14405. doi:10.1038/srep14405
Zhang, Y., Peng, Y., Zhao, L., Zhou, G., and Li, X. (2021). Regulating the Gut Microbiota and SCFAs in the Faeces of T2DM Rats Should Be One of Antidiabetic Mechanisms of Mogrosides in the Fruits of Siraitia Grosvenorii. J. Ethnopharmacol. 274, 114033. doi:10.1016/j.jep.2021.114033
Zhao, M., Yuan, M. M., Yuan, L., Huang, L. L., Liao, J. H., Yu, X. L., et al. (2018). Chronic Folate Deficiency Induces Glucose and Lipid Metabolism Disorders and Subsequent Cognitive Dysfunction in Mice. PLoS One 13, e0202910. doi:10.1371/journal.pone.0202910
Zheng, L., Cardaci, S., Jerby, L., MacKenzie, E. D., Sciacovelli, M., Johnson, T. I., et al. (2015). Fumarate Induces Redox-dependent Senescence by Modifying Glutathione Metabolism. Nat. Commun. 6, 6001. doi:10.1038/ncomms7001
Zhong, Y., Menon, M. C., Deng, Y., Chen, Y., and He, J. C. (2015). Recent Advances in Traditional Chinese Medicine for Kidney Disease. Am. J. Kidney Dis. 66, 513–522. doi:10.1053/j.ajkd.2015.04.013
Zhong, Y. F., Zhou, Y. H., Liu, S. X., Guo, J. L., and Zuo, D. Y. (2016). Clinical Observation on Curative Effect of IgA Nephropathy Treated with Sanhuang Yishen Capsule Combining with Corticosteroids and Impact on Serum VEGF. Chin. J. Tradit Med. Sci. Tech. 5, 3. Available at http://en.cnki.com.cn/Article_en/CJFDTOTAL-TJYY201605008.htm.
Keywords: San-Huang-Yi-Shen capsule, diabetic nephropathy, gut microbiota, arginine biosynthesis, tricarboxylic acid cycle, tyrosine metabolism, arginine and proline metabolism
Citation: Su X, Yu W, Liu A, Wang C, Li X, Gao J, Liu X, Jiang W, Yang Y and Lv S (2022) San-Huang-Yi-Shen Capsule Ameliorates Diabetic Nephropathy in Rats Through Modulating the Gut Microbiota and Overall Metabolism. Front. Pharmacol. 12:808867. doi: 10.3389/fphar.2021.808867
Received: 04 November 2021; Accepted: 10 December 2021;
Published: 04 January 2022.
Edited by:
Shuai Ji, Xuzhou Medical University, ChinaReviewed by:
Meng Xianglong, Shanxi University of Traditional Chinese Medicine, ChinaCopyright © 2022 Su, Yu, Liu, Wang, Li, Gao, Liu, Jiang, Yang and Lv. This is an open-access article distributed under the terms of the Creative Commons Attribution License (CC BY). The use, distribution or reproduction in other forums is permitted, provided the original author(s) and the copyright owner(s) are credited and that the original publication in this journal is cited, in accordance with accepted academic practice. No use, distribution or reproduction is permitted which does not comply with these terms.
*Correspondence: Shuquan Lv, Y3psdnNodXF1YW5AMTYzLmNvbQ==
Disclaimer: All claims expressed in this article are solely those of the authors and do not necessarily represent those of their affiliated organizations, or those of the publisher, the editors and the reviewers. Any product that may be evaluated in this article or claim that may be made by its manufacturer is not guaranteed or endorsed by the publisher.
Research integrity at Frontiers
Learn more about the work of our research integrity team to safeguard the quality of each article we publish.