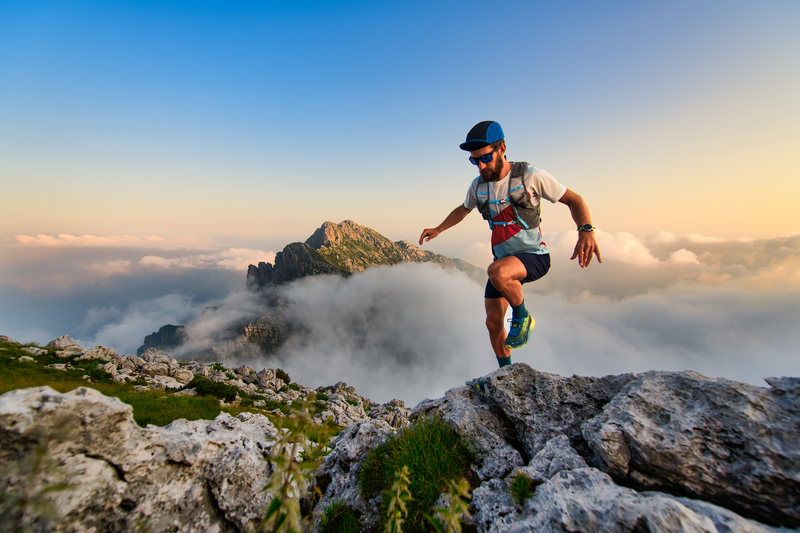
95% of researchers rate our articles as excellent or good
Learn more about the work of our research integrity team to safeguard the quality of each article we publish.
Find out more
ORIGINAL RESEARCH article
Front. Pharmacol. , 23 February 2022
Sec. Pharmacogenetics and Pharmacogenomics
Volume 12 - 2021 | https://doi.org/10.3389/fphar.2021.790832
This article is part of the Research Topic Pharmacogenomics in Asians: Differences and Similarities with other Human Populations View all 6 articles
Medication safety and efficacy-related pharmacogenomic research play a critical role in precision medicine. This study comprehensively analyzed the pharmacogenomic profiles of the central Han Chinese population in the context of medication safety and efficacy and compared them with other global populations. The ultimate goal is to improve medical treatment guidelines. We performed whole-genome sequencing in 487 Han Chinese individuals and investigated the allele frequencies of pharmacogenetic variants in 1,731 drug response-related genes. We identified 2,139 (81.18%) previously reported variants in our population with annotations in the PharmGKB database. The allele frequencies of these 2,139 clinical-related variants were similar to those in other East Asian populations but different from those in other global populations. We predicted the functional effects of nonsynonymous variants in the 1,731 pharmacogenes and identified 1,281 novel and 4,442 previously reported deleterious variants. Of the 1,281 novel deleterious variants, five are common variants with an allele frequency >5%, and the rest are rare variants with an allele frequency <5%. Of the 4,442 known deleterious variants, the allele frequencies were found to differ from those in other populations, of which 146 are common variants. In addition, we found many variants in non-coding regions, the functions of which require further investigation. This study compiled a large amount of data on pharmacogenomic variants in the central Han Chinese population. At the same time, it provides insight into the role of pharmacogenomic variants in clinical medication safety and efficacy.
Clinical medication efficacy and adverse drug reactions (ADRs) often vary widely among individuals. Pharmacogenomics aims to elucidate the effects of genetic polymorphisms and interindividual differences about the efficacy of medications (Evans and Relling, 1999; Evans and Johnson, 2001). Many studies have demonstrated that gene variants encoding drug-metabolizing enzymes, drug transporters, and drug targets affect drug responses (Choi et al., 2015; Ahmed et al., 2016). The aim of the Pharmacogenomics Knowledge base (PharmGKB; https://www.pharmgkb.org) is to collect and analyze data and then disseminate knowledge on the impact of genetic variations associated with drug responses. PharmGKB provides clinical information on genotype-phenotype relationships and variant–drug associations based on well-defined criteria and careful literature reviews.
Traditional methods to detect drug reaction-related genetic polymorphisms include PCR and microarray-based techniques (Hodel et al., 2009; Burmester et al., 2010). Although these methods are cost-effective and easy to implement, they focus on the most common pharmacogenomic variants rather than identifying novel or rare polymorphisms associated with individual differences in drug responses. Next-generation sequencing (NGS) technology addresses the shortcomings of conventional detection methods. Whole-exome sequencing (WES) and whole-genome sequencing (WGS) can be used not only for the diagnosis of Mendelian diseases but also for the comprehensive investigation of drug response-related variants in individuals (Katsila and Patrinos, 2015; Ji et al., 2018). Given the decreasing cost of NGS, many studies have applied WES and WGS to pharmacogenomic research and obtained novel insights (Altman et al., 2013; Ahn and Park, 2017; Sivadas et al., 2017; Sivadas and Scaria, 2018; Choi et al., 2019; Caspar et al., 2020).
Pharmacogenomically relevant variants, in terms of drug efficacy and adverse effects, vary widely in frequency among global populations (Yasuda et al., 2008; Ramos et al., 2014). Moreover, some drugs with safe and effective doses for ethnicities with certain genetic variants are not appropriate for others (Rieder et al., 2005; Lam et al., 2016). Therefore, it is essential to widen the scope of pharmacogenomic research to encompass populations worldwide and increase the evidence base for precision medicine.
China comprises multiple ethnicities. For safe, reasonable, and precise personalized therapy, comprehensive pharmacogenetic analysis of the Chinese population is required. However, most studies have focused only on the frequencies of common variants in several essential genes in the Chinese population (Qian et al., 2013; Hu et al., 2017; Liu et al., 2019; Qi et al., 2020a). For example, Dai et al. and Hu et al. systematically investigated polymorphisms in the cytochrome P450 (CYP) genes CYP2C9 and CYP2C19, respectively, in the Han Chinese population (Hu et al., 2012; Dai et al., 2014). Although the sample sizes were large, both of those studies were concerned with only one gene, and variants in intronic regions were not revealed due to the methods’ limitations. Qi et al. (2020b) assessed the genetic variations in 57 CYP and cytochrome P450 oxidoreductase genes in a large-scale WGS study based on the Chinese Millionome database; however, the shallow sequencing depth may have led to rare variants being missed.
This study investigated the distribution of pharmacogenomic variants in the central Han Chinese population using high-depth WGS and compared the allele frequencies with those in other global populations. We also comprehensively analyzed the allele frequencies of variants with PharmGKB annotations. To the best of our knowledge, this is the first comprehensive pharmacogenomic study conducted in a Chinese population.
This study enrolled 487 healthy subjects (198 males and 289 females) aged 18–60 years. The subjects were not biologically related and were all Han Chinese. Based on their medical records, all of the participants were healthy. Furthermore, they all signed informed consent forms before any blood samples were collected. The ethics committee of Zhengzhou University approved the study protocol (reference number: ZZURIB 2019-002).
Peripheral venous blood samples (3–4 ml) were collected into EDTA anticoagulant tubes. Genomic DNA was extracted from white blood cells using the GenMagBio Genomic DNA Purification kit (GenMagBio, Changzhou, China). The concentration and purity of the genomic DNA were measured using the NanoDrop One instrument (Thermo Fisher Scientific, Waltham, MA, United States), and the quality of the DNA was determined by 1% agarose gel electrophoresis.
Genomic DNA was fragmented (∼400 bp) using sonication. The fragmented DNA was then end-repaired, ligated to adapters, and PCR-enriched using the VAHTS Universal DNA Library Prep Kit (Vazyme Biotech Co. Ltd., Nanjing, China) according to the manufacturer’s protocol. The resulting DNA libraries were sequenced using the HiSeq 4000 platform (Illumina Inc., San Diego, CA, United States) operating in paired-end 150 bp mode (∼30×) at the Precision Medicine Center of Zhengzhou University (Zhengzhou, China).
Sequencing adapters and low-quality reads were trimmed from raw reads using Trimmomatic (Bolger et al., 2014). Clean reads were aligned to the human reference genome hg19 using BWA-MEM (version 0.7.17-r1188) (Li, 2013). Single nucleotide variants and minor insertion/deletions were characterized using the Genome Analysis Toolkit (version 4; GATK4) HaplotypeCaller (DePristo et al., 2011). Variant annotation was performed using SnpEff and Vcfanno and several annotation databases (Cingolani et al., 2012; Liu et al., 2016; Pedersen et al., 2016). All of the bioinformatics analysis steps were performed within the framework of bcbio-nextgen (https://github.com/bcbio/bcbio-nextgen).
We downloaded the gene list from the PharmGKB database and identified 1,731 PharmGKB-annotated genes using the “Has Variant Annotation” search field (Whirl-Carrillo et al., 2012). The chromosomal locations of the pharmacogenes were obtained from the NCBI database (https://www.ncbi.nlm.nih.gov/). We extracted all variants (n = 2,459,656) in the 1,731 pharmacogenes from 487 WGS datasets. Variants with annotation information in the Single Nucleotide Polymorphism database (dbSNP; version 151) were defined as known variants, while those without dbSNP accession IDs were considered novel variants (Sherry et al., 2001). The analysis workflow is summarized in Figure 1.
FIGURE 1. The overview of analysis workflow for pharmacogenomic variant in the central Han Chinese population. PPH2, PolyPhen2. MT2, Mutation Taster 2.
We assessed Hardy–Weinberg equilibrium (HWE; p < 0.05 with false discovery rate [FDR] adjustment) using PLINK v1.9 (Chang et al., 2015) and obtained 2,398,696 (97.52%) variants for downstream analysis. We calculated the variant allele frequencies (VAF) of the 2,398,696 variants in the central Han Chinese population using VCFtools (Danecek et al., 2011).
Nonsynonymous variants (missense variant, start loss, stop loss, and stop gain) were examined for deleterious effects on the encoded proteins using SIFT (Ng and Henikoff, 2003), PolyPhen2 (Adzhubei et al., 2010), and MutationTaster2 (Schwarz et al., 2014). Variants were classified as potentially deleterious based on the predictions of at least two tools (i.e., as “damaging” by SIFT, “probably damaging” by Polyphen2, and “disease-causing” by MutationTaster2).
Pharmacogenes with a deleterious variant and allele frequency >10% in our population were mapped to drugs in the DrugBank (Wishart et al., 2018). Then, a Sankey flow diagram was constructed using Microsoft Power BI (Microsoft Corp., Redmond, WA, United States).
We downloaded the clinical annotations for pharmacogenomic variants from PharmGKB. The distributions of the 2,635 unique single nucleotide polymorphisms (SNPs) in our study population were analyzed (Whirl-Carrillo et al., 2012). The allele frequencies of variants considered to have a higher level of clinical evidence (levels 1A and 1B) (Whirl-Carrillo et al., 2012) were compared with those in other populations included in the 1000 Genomes Project phase 3 (1KG3) (ftp://ftp.ncbi.nlm.nih.gov/1000genomes/ftp/phase3/data/) (Genomes Project et al., 2015) and genome Aggregation database (gnomAD) (https://gnomad.broadinstitute.org/) (Karczewski et al., 2020) by chi-square test.
The variant frequencies for the central Han Chinese population were extracted based on an HWE test of the level 1A and 1B variants in PharmGKB. The frequency information in our population was compared with all populations as a whole and the East Asian populations in the gnomAD and 1KG3 database, which is illustrated by a scatterplot. The variant frequencies of high evidence levels (1A or 1B) are illustrated as a bubble diagram. The scatterplots and bubble diagrams were generated by the R package ggplot2 (R version 4.0.2) (Wickham et al., 2016). Among potentially deleterious variants, common variants (VAF> 10%) compared with other populations were visualized as a heatmap. The heatmaps were produced using the R package ComplexHeatmap (R version 4.0.2) (Gu et al., 2016).
This study analyzed a WGS dataset comprising 487 central Han Chinese individuals. Specifically, we focused on the variants in 1,731 drug response-related genes. Quality control (QC) is essential for raw NGS data. In this study, our sample’s average, minimum, and maximum Q30 values were 97.19, 95.00, and 98.31%, respectively. Sequencing reads were mapped to the human reference genome (GRCh37); the average sequencing depth data are summarized in Table 1. Coverage refers to the proportion of the genome that has been sequenced (Table 1).
After the HWE tests, a total of 2,398,696 variants in 1,731 pharmacogenes were obtained, of which 80.11% were known (i.e., had rs IDs in dbSNP v151), and 476,984 variants were novel. Variant annotation revealed 18,907 missense variants, 13,923 synonymous variants and 1,746,470 intronic variants (72.81%). The variant annotations are summarized in Supplementary Table S1.
Allele frequency analysis of the 2,398,696 variants in the central Han Chinese population showed that a large number of variants were rare (65.23%; VAF <1%), 231,447 were low frequency (9.65%, VAF = 1–5%), and 602,586 were common (25.12%; VAF >5%) (Supplementary Figure S1).
To achieve a comprehensive understanding of the 1,731 drug response-related pharmacogenetic variants identified in our central Han Chinese population, we used SIFT, PolyPhen-2, and MutationTaster2 to predict the functional impact of 19,368 nonsynonymous variants. A total of 5,723 variants were predicted to be potentially deleterious using at least two of the tools (Table 2, Supplementary Figure S2); these 5,723 variants, 1,281 of which are novel, may impair the function of 1,316 genes (Supplementary Table S2). Of the 5,723 variants, 149 were classified as common (VAF >5%) and 5,253 as rare (VAF <1%); 4,023 of the rare variants were found in only one person. The allele frequencies of 47 of the 1,281 novel variants were >1%; the others were classified as rare (Supplementary Figure S3).
Among the 5,723 potentially deleterious variants, the 85 classified as common (VAF >10%) affect the function of 67 genes. We present the allele frequencies of these 85 variants in our central Han Chinese population, along with those in the other populations included in the 1KG3 and gnomAD databases, in Figure 2 and Supplementary Table S2. Comparison of the allele frequencies revealed that 67 and 75 variants differed significantly between our dataset and the 1KG3 and gnomAD database populations, respectively (FDR-adjusted p-values < 0.05). For example, variant rs4646422, which impairs the function of CYP1A1, was highly prevalent (VAF = 0.2228) in our central Han Chinese population compared with the other populations (1KG3.ALL, VAF = 0.0242; G.ALL, VAF = 0.0077; 1KG3.EAS, VAF = 0.1151; G.EAS, VAF = 0.1535). The SH2B3 gene has a deleterious variant, rs78894077, with high frequency among East Asian populations (our cohort, VAF = 0.1140; 1KG3.EAS, VAF = 0.0635; G.EAS, VAF = 0.0546); however, it is largely absent from other populations.
FIGURE 2. Allele frequencies of 85 most common potentially deleterious variants in the central Han Chinese population compared to global populations in 1000 Genomes Project phase 3 (1KG3) and gnomAD databases (G). The table on the right of the heatmap: VARIANT: variant name, GENE: gene name, K: blue color indicates that the allele frequency in our study is different from the 1KG3.ALL frequency (q < 0.05), G: blue color indicates that the allele frequency in our study is different from the G.ALL frequency (q < 0.05), the last column represents the number of clinical annotations at various levels of evidence of 16 variants in the PharmGKB. CHC: central Han Chinese population; VAF: variant allele frequency.
In total, 16 of the 85 potentially deleterious variants have clinical annotations in the PharmGKB database (Whirl-Carrillo et al., 2012). These 16 genes include 5 “very important pharmacogenes,” CYP2A6, CYP4F2, MTHFR, SLC22A1, and SLCO1B1, which are involved in the metabolism and transport of many pharmacological agents. The 16 variants were associated with 80 clinical annotations with varying levels of evidence. The allele frequencies of 14 of these 16 variants were significantly different from those in the global populations included in the 1KG3 and gnomAD databases (Figure 2, Supplementary Table S2). The rs1801133 variant in the MTHFR gene was associated with 17 clinical annotations. MTHFR, which affects the efficacy and toxicity of antineoplastic drugs such as methotrexate, carboplatin, and cisplatin (level 2A), was more prevalent among our central Han Chinese compared with the other global and East Asian populations (our cohort, VAF = 0.6273, 1KG3.ALL, VAF = 0.2454; G.ALL, VAF = 0.2573; 1KG3.EAS, VAF = 0.2956; G.EAS, VAF = 0.2884). The MTRR variant rs1801394 is involved in the toxicity of, and ADRs to, methotrexate (level 2B); its prevalence in our population was lower than that in the global populations in the databases and similar to that in the majority of the other East Asian populations (our cohort, VAF = 0.2536; 1KG3.ALL, VAF = 0.3642; G.ALL, VAF = 0.4622; 1KG3.EAS, VAF = 0.2629; G.EAS, VAF = 0.2805). The 69 variants without clinical annotations involved 55 genes, including the “very important pharmacogenes” HLA-B and CACNA1S. Allelic variants in HLA-B have been associated with ADRs to abacavir and carbamazepine, among other drugs.
To analyze the effect of disrupted pharmacogenes in drug pathways, we mapped the 67 genes with deleterious variants and a VAF >10% to drugs in the DrugBank database. In total, 416 drugs were associated with 32 genes; the drug pathways are presented as a Sankey flow diagram (Figure 3, Supplementary Table S3). These 32 genes harbored the 40 most common deleterious variants and were associated with two carriers, 10 transporter, seven enzyme, and 20 target genes. The drug pathway map includes a wide range of drug classes (e.g., cardiovascular, antineoplastic, and immunomodulating agents). As an example, bezafibrate, a hypolipidemic agent, may be affected by transport and metabolic functions because of its transporter gene (SLCO1B1) and primary metabolizing enzyme (CYP1A1) both contained deleterious variants in more than 10% of our central Han Chinese population. Nifedipine is a dihydropyridine L-type calcium channel blocker used to treat hypertension; its target gene (CACNA1S) and primary enzyme genes (CYP1A1 and CYP2A6) all had deleterious variants in our population. These findings shed light on the interplay between drug-related genes in drug pathways and drug responses in central Han Chinese populations.
FIGURE 3. Drug pathway map describing functionally-impaired pharmacogenes in the central Han Chinese population. The four columns in the map represent the major drug category affected by putatively deleterious variants, transporter/carrier genes, enzyme genes, and target genes. NA: None Affected; Nil: No known genes.
To investigate the overall distribution of variants with PharmGKB clinical annotations among our central Han Chinese population, we attempted to match the 2,635 SNP variants from PharmGKB to the list of 2,398,696 variants identified in our cohort; 2,139 (81.18%) clinically relevant variants were matched (Supplementary Table S4). Among these 2,139 variants, the allele frequencies of 85.83% (N = 1,836) were >5%, while 7.01% (n = 150) were rare in our population (Supplementary Figure S4). Compared with all populations in the 1KG3 and gnomAD databases, the frequencies of 1,790 and 1,920 variants, respectively, were significantly different (FDR <0.05). The frequencies of 393 and 333 variants were also significantly different from those in the East Asian populations in the 1KG3 and gnomAD databases, respectively (FDR <0.05) (Figure 4). Of the 2,139 variants, 24 (30 clinical annotations) had high evidence levels (1A or 1B) and were related to 15 genes and 34 therapeutic agents (Table 3, Supplementary Table S4).
FIGURE 4. Compared with the mutation frequencies of all populations in the 1KG3 and gnomAD databases, 1,790 and 1,920 genetic mutations are statistically different (FDR <0.05). Compared with the mutation frequencies of the East Asian populations in the 1KG3 and gnomAD databases, respectively. There were statistical differences between 393 and 333 genetic variants (FDR <0.05). VAF_Han_Chinese: variant allele frequency in the central Han Chinese population; VAF_1KG3_ALL: Variant Allele Frequency for all populations in 1KG3; VAF_1KG3_EAS: Variant Allele Frequency for East Asian population in 1KG3; VAF_gnomAD_ALL: Variant Allele Frequency for all populations in gnomAD; VAF_gnomAD_EAS: Variant Allele Frequency for East Asian population in gnomAD.
TABLE 3. Clinical annotations of 30 variants with a higher level of evidence (Level 1A and 1B) in PharmGKB.
We then compared the allele frequencies of 24 clinically significant variants in our study population with those in the global populations included in the 1KG3 and gnomAD databases (Figure 5). The frequencies of 17 alleles were significantly different from those of the average global population (FDR-adjusted p-value < 0.05). However, only five variants in our population showed significant differences compared with the other East Asian populations. The VKORC1 variant rs7294, associated with warfarin dosage, showed a lower frequency among our central Han Chinese population (VAF = 0.0698) compared with the global populations (1KG3.ALL, VAF = 0.4197; G.ALL, VAF = 0.3948) and other East Asian populations (1KG3.EAS, VAF = 0.1121, G.EAS, VAF = 0.1013). In addition, the other variants in VKORC1, rs9923231 and rs9934438, showed significantly higher prevalences (VAF = 0.9271 and 0.9271, respectively) in our population compared with the global populations (1KG3.ALL, VAF = 0.3556 and 0.3558, respectively; G.ALL, VAF = 0.3260 and 0.3261, respectively). The NUDT15 variant rs116855232, associated with azathioprine and mercaptopurine dosage, toxicity, and ADRs, was more widely observed among the central Han Chinese population (VAF = 0.1346) compared with the global populations (1KG3.ALL, VAF = 0.0395; G.ALL, VAF = 0.0110) and other East Asian populations (1KG3.EAS, VAF = 0.0952; G.EAS, VAF = 0.0972).
FIGURE 5. Comparison of the Han Chinese allele frequencies of clinically significant PharmGKB variants with populations included in 1000 Genomes Project phase 3 (1KG3) and gnomAD databases (G). The variants are arranged according to the category of drugs they affect. The size of the solid circle represents the allele frequencies ranging from 0.00 to 1.00. The black outer ellipse represents that the variant allele frequency of Han Chinese has a statistical difference compared to global population averages (1KG3.ALL and G.ALL).
The allele frequencies of pharmacogenomic markers of drug efficacy and toxicity vary among ethnicities (Ramos et al., 2014). Genetic variants can impact medication doses and therapeutic decision-making, for which there is a need to avoid ADRs (Limdi et al., 2008; Li et al., 2018). However, many studies only focused on a few variants in several commonly investigated genes; thus, rare variants may have been missed, resulting in inappropriate drug prescriptions in some cases. Therefore, it is necessary to expand the scope of pharmacogenomic research to encompass multiple ethnic populations. WES and WGS provide an opportunity for a more comprehensive analysis of pharmacogenomic profiles (Petersen et al., 2017). In the present study of 487 central Han Chinese individuals, we used high-depth WGS data to assess the allele frequencies of variants with PharmGKB clinical annotations, with deleterious variants potentially affecting the function of pharmacogenes.
The screening of variants with PharmGKB clinical annotations is of high clinical utility; 2,139 (81.18%) clinically relevant variants were found in our population. Among the 119 variants in PharmGKB with a higher level of evidence (1A or 1B), only 24 SNPs were found in our central Han Chinese population, whereas a large proportion of the variants (79.83%) were not detected. The differences among the populations demonstrated the genetic heterogeneity among ethnic groups. The 24 variants with clinical annotations involved 14 genes, such as the CYP gene family, VKORC1, etc. According to research by Biswas (2021), the phenotype of the CYP2C19 gene is divided into extensive metabolizers (EM), poor metabolizers (PM), intermediate metabolizers (IM), and ultrarapid metabolizers (UM). Among them, UM (CYP2C19*1/*17; CYP2C19* 17/*17) and PM (CYP2C19*2/*2; CYP2C19*3/*3, CYP2C19*2/*3) were considered high risk phenotypes. UM were prevalent high in Africa (33.7%) and low in the Central Han Chinese Population (1.2%). The prevalence of PM in South Asia and the Central Han Chinese Population is similar, about 11%. Further research is needed to fully understand the polymorphisms in the Han Chinese population.”
Functional predictions of the variants in 1,731 pharmacogenes revealed that the functions of 1,316 genes may be affected by 5,723 potentially deleterious variants, 5253 (91.77%) of which were classified as rare. This shows the importance of NGS for discovering rare variants that may account for a large proportion of the unexplained interindividual differences in metabolic phenotypes observed for some drugs (Ingelman-Sundberg et al., 2018). Among the 5,723 deleterious variants in this study, 1,281 novel variants were identified; their effects on the functions of pharmacogenes need to be elucidated in further studies. Finally, we highlighted the differences in the allele frequencies of 85 common (VAF >10%) deleterious variants between our cohort and other global populations. This information could facilitate optimal drug selection and dosing regimens.
In conclusion, this is the first study to analyze pharmacogenomic variants in the central Han Chinese population comprehensively. In total, 2,139 clinically relevant variants were identified, of which 24 had high levels of evidence (1A or 1B). We also found that 5,723 of 2,398,696 variants are potentially deleterious, of which 1,281 are novel. We compared the allele frequencies of 85 common (VAF >10%) deleterious variants with those in other populations. The differences in allele frequencies among the populations demonstrated the genetic heterogeneity among ethnic groups. WGS shows great potential based on the results of our study but also faces challenges such as difficulty in interpreting variants of unknown significance in drug-related genes. A comprehensive understanding of genetic polymorphisms at the population level is essential for safe, rational, and effective utilization of drugs and for precision medicine. However, the effects of certain novel and rare pharmacogenetic variants need to be verified by functional experiments and clinical studies.
The raw sequencing data supporting this article cannot be placed in public repository due to national legislation/guidelines, specifically the Regulation of the People's Republic of China on the Administration of Human Genetic Resources (http://www.gov.cn/zhengce/content/2019-06/10/content_5398829.htm, http://english.www.gov.cn/policies/latest_releases/2019/06/10/content_281476708945462.htm). As required by the funding bodies, the raw sequencing data were deposited in the National Supercomputing Center in Zhengzhou. Please email bnNjY0B6enUuZWR1LmNu for detailed application guidance. The accession code can be obtained by emailing the corresponding authors upon reasonable request.
The studies involving human participants were reviewed and approved by The Ethics Committee of Zhengzhou University. Written informed consent to participate in this study was provided by the participants or their legal guardians.
Participated in research design: JG, HX, JT and JZ. Conducted experiments: JT, JZ, SR, JS, and XH. Performed data analysis: JT, JZ, ZY, SF, SL, XX and BY. Wrote or contributed to the writing of the manuscript: JZ, JT, HX, and JG.
The study was funded by the Collaborative Innovation Project of Zhengzhou (Zhengzhou University) (grant number: 20XTZX05014); the Joint Project of Medical Science and Technology Research in Henan Province of China (grant number: SBGJ2018041); the Key Scientific and Technological Research Projects in Henan Province of China (grant number: 192102310216).
The authors declare that the research was conducted in the absence of any commercial or financial relationships that could be construed as a potential conflict of interest.
All claims expressed in this article are solely those of the authors and do not necessarily represent those of their affiliated organizations, or those of the publisher, the editors, and the reviewers. Any product that may be evaluated in this article, or claim that may be made by its manufacturer, is not guaranteed or endorsed by the publisher.
We sincerely thank all the individuals who volunteered to participate in this study and thank the help and support of the members of the Precision Medicine Center of Zhengzhou University. We also thank the Supercomputing Center of Zhengzhou University for providing computational and storage resources.
The Supplementary Material for this article can be found online at: https://www.frontiersin.org/articles/10.3389/fphar.2021.790832/full#supplementary-material
ADR, adverse drug reactions; gnomAD, genome Aggregation Database; NGS, next-generation sequencing; SNVs, single nucleotide variants; VAF, variant allele frequency in the central han chinese population; VIPs, very important pharmacogenes; WES, whole-exome sequencing; WGS, whole-genome sequencing; 1KG3, 1000 genomes project phase 3.
Adzhubei, I. A., Schmidt, S., Peshkin, L., Ramensky, V. E., Gerasimova, A., Bork, P., et al. (2010). A Method and Server for Predicting Damaging Missense Mutations. Nat. Methods 7 (4), 248–249. doi:10.1038/nmeth0410-248
Ahmed, S., Zhou, Z., Zhou, J., and Chen, S. Q. (2016). Pharmacogenomics of Drug Metabolizing Enzymes and Transporters: Relevance to Precision Medicine. Genomics Proteomics Bioinformatics 14 (5), 298–313. doi:10.1016/j.gpb.2016.03.008
Ahn, E., and Park, T. (2017). Analysis of Population-specific Pharmacogenomic Variants Using Next-Generation Sequencing Data. Sci. Rep. 7 (1), 8416. doi:10.1038/s41598-017-08468-y
Altman, R. B., Whirl-Carrillo, M., and Klein, T. E. (2013). Challenges in the Pharmacogenomic Annotation of Whole Genomes. Clin. Pharmacol. Ther. 94 (2), 211–213. doi:10.1038/clpt.2013.111
Biswas, M. (2021). Global Distribution of CYP2C19 Risk Phenotypes Affecting Safety and Effectiveness of Medications. Pharmacogenomics J. 21 (2), 190–199. doi:10.1038/s41397-020-00196-3
Bolger, A. M., Lohse, M., and Usadel, B. (2014). Trimmomatic: a Flexible Trimmer for Illumina Sequence Data. Bioinformatics 30 (15), 2114–2120. doi:10.1093/bioinformatics/btu170
Burmester, J. K., Sedova, M., Shapero, M. H., and Mansfield, E. (2010). DMET Microarray Technology for Pharmacogenomics-Based Personalized Medicine. Methods Mol. Biol. 632, 99–124. doi:10.1007/978-1-60761-663-4_7
Caspar, S. M., Schneider, T., Meienberg, J., and Matyas, G. (2020). Added Value of Clinical Sequencing: WGS-Based Profiling of Pharmacogenes. Int. J. Mol. Sci. 21 (7), 2308. doi:10.3390/ijms21072308
Chang, C. C., Chow, C. C., Tellier, L. C., Vattikuti, S., Purcell, S. M., and Lee, J. J. (2015). Second-generation PLINK: Rising to the challenge of Larger and Richer Datasets. Gigascience 4, 7. doi:10.1186/s13742-015-0047-8
Choi, J., Tantisira, K. G., and Duan, Q. L. (2019). Whole Genome Sequencing Identifies High-Impact Variants in Well-Known Pharmacogenomic Genes. Pharmacogenomics J. 19 (2), 127–135. doi:10.1038/s41397-018-0048-y
Choi, J. R., Kim, J. O., Kang, D. R., Shin, J. Y., Zhang, X. H., Oh, J. E., et al. (2015). Genetic Variations of Drug Transporters Can Influence on Drug Response in Patients Treated with Docetaxel Chemotherapy. Cancer Res. Treat. 47 (3), 509–517. doi:10.4143/crt.2014.012
Cingolani, P., Platts, A., Wang, le. L., Coon, M., Nguyen, T., Wang, L., et al. (2012). A Program for Annotating and Predicting the Effects of Single Nucleotide Polymorphisms, SnpEff: SNPs in the Genome of Drosophila melanogaster Strain W1118; Iso-2; Iso-3. Fly (Austin) 6 (2), 80–92. doi:10.4161/fly.19695
Dai, D. P., Xu, R. A., Hu, L. M., Wang, S. H., Geng, P. W., Yang, J. F., et al. (2014). CYP2C9 Polymorphism Analysis in Han Chinese Populations: Building the Largest Allele Frequency Database. Pharmacogenomics J. 14 (1), 85–92. doi:10.1038/tpj.2013.2
Danecek, P., Auton, A., Abecasis, G., Albers, C. A., Banks, E., DePristo, M. A., et al. (2011). The Variant Call Format and VCFtools. Bioinformatics 27 (15), 2156–2158. doi:10.1093/bioinformatics/btr330
DePristo, M. A., Banks, E., Poplin, R., Garimella, K. V., Maguire, J. R., Hartl, C., et al. (2011). A Framework for Variation Discovery and Genotyping Using Next-Generation DNA Sequencing Data. Nat. Genet. 43 (5), 491–498. doi:10.1038/ng.806
Evans, W. E., and Johnson, J. A. (2001). Pharmacogenomics: the Inherited Basis for Interindividual Differences in Drug Response. Annu. Rev. Genomics Hum. Genet. 2, 9–39. doi:10.1146/annurev.genom.2.1.9
Evans, W. E., and Relling, M. V. (1999). Pharmacogenomics: Translating Functional Genomics into Rational Therapeutics. Science 286 (5439), 487–491. doi:10.1126/science.286.5439.487
Genomes Project, C., Auton, A., Brooks, L. D., Durbin, R. M., Garrison, E. P., Kang, H. M., et al. (2015). A Global Reference for Human Genetic Variation. Nature 526 (7571), 68–74. doi:10.1038/nature15393
Gu, Z., Eils, R., and Schlesner, M. (2016). Complex Heatmaps Reveal Patterns and Correlations in Multidimensional Genomic Data. Bioinformatics 32 (18), 2847–2849. doi:10.1093/bioinformatics/btw313
Hodel, E. M., Ley, S. D., Qi, W., Ariey, F., Genton, B., and Beck, H. P. (2009). A Microarray-Based System for the Simultaneous Analysis of Single Nucleotide Polymorphisms in Human Genes Involved in the Metabolism of Anti-malarial Drugs. Malar. J. 8, 285. doi:10.1186/1475-2875-8-285
Hu, G. X., Dai, D. P., Wang, H., Huang, X. X., Zhou, X. Y., Cai, J., et al. (2017). Systematic Screening for CYP3A4 Genetic Polymorphisms in a Han Chinese Population. Pharmacogenomics 18 (4), 369–379. doi:10.2217/pgs-2016-0179
Hu, L. M., Dai, D. P., Hu, G. X., Yang, J. F., Xu, R. A., Yang, L. P., et al. (2012). Genetic Polymorphisms and Novel Allelic Variants of CYP2C19 in the Chinese Han Population. Pharmacogenomics 13 (14), 1571–1581. doi:10.2217/pgs.12.141
Ingelman-Sundberg, M., Mkrtchian, S., Zhou, Y., and Lauschke, V. M. (2018). Integrating Rare Genetic Variants into Pharmacogenetic Drug Response Predictions. Hum. Genomics 12 (1), 26. doi:10.1186/s40246-018-0157-3
Ji, Y., Si, Y., McMillin, G. A., and Lyon, E. (2018). Clinical Pharmacogenomics Testing in the Era of Next Generation Sequencing: Challenges and Opportunities for Precision Medicine. Expert Rev. Mol. Diagn. 18 (5), 411–421. doi:10.1080/14737159.2018.1461561
Karczewski, K. J., Francioli, L. C., Tiao, G., Cummings, B. B., Alföldi, J., Wang, Q., et al. (2020). The Mutational Constraint Spectrum Quantified from Variation in 141,456 Humans. Nature 581 (7809), 434–443. doi:10.1038/s41586-020-2308-7
Katsila, T., and Patrinos, G. P. (2015). Whole Genome Sequencing in Pharmacogenomics. Front. Pharmacol. 6, 61. doi:10.3389/fphar.2015.00061
Lam, S. W., Guchelaar, H. J., and Boven, E. (2016). The Role of Pharmacogenetics in Capecitabine Efficacy and Toxicity. Cancer Treat. Rev. 50, 9–22. doi:10.1016/j.ctrv.2016.08.001
Li, H. (2013). Aligning Sequence Reads, Clone Sequences and Assembly Contigs with BWA-MEM. arXiv:1303.3997. arXiv preprint. Available at http://arxiv.org/abs/1303.3997v2.
Li, J., Yang, W., Xie, Z., Yu, K., Chen, Y., and Cui, K. (2018). Impact of VKORC1, CYP4F2 and NQO1 Gene Variants on Warfarin Dose Requirement in Han Chinese Patients with Catheter Ablation for Atrial Fibrillation. BMC Cardiovasc. Disord. 18 (1), 96. doi:10.1186/s12872-018-0837-x
Limdi, N. A., Beasley, T. M., Crowley, M. R., Goldstein, J. A., Rieder, M. J., Flockhart, D. A., et al. (2008). VKORC1 Polymorphisms, Haplotypes and Haplotype Groups on Warfarin Dose Among African-Americans and European-Americans. Pharmacogenomics 9 (10), 1445–1458. doi:10.2217/14622416.9.10.1445
Liu, S., Wang, C., Chen, Y., Peng, S., Chen, X., and Tan, Z. (2019). Association of SLC15A1 Polymorphisms with Susceptibility to Dyslipidaemia in a Chinese Han Population. J. Clin. Pharm. Ther. 44 (6), 868–874. doi:10.1111/jcpt.13016
Liu, X., Wu, C., Li, C., and Boerwinkle, E. (2016). dbNSFP v3.0: A One-Stop Database of Functional Predictions and Annotations for Human Nonsynonymous and Splice-Site SNVs. Hum. Mutat. 37 (3), 235–241. doi:10.1002/humu.22932
Ng, P. C., and Henikoff, S. (2003). SIFT: Predicting Amino Acid Changes that Affect Protein Function. Nucleic Acids Res. 31 (13), 3812–3814. doi:10.1093/nar/gkg509
Pedersen, B. S., Layer, R. M., and Quinlan, A. R. (2016). Vcfanno: Fast, Flexible Annotation of Genetic Variants. Genome Biol. 17 (1), 118. doi:10.1186/s13059-016-0973-5
Petersen, B. S., Fredrich, B., Hoeppner, M. P., Ellinghaus, D., and Franke, A. (2017). Opportunities and Challenges of Whole-Genome and -exome Sequencing. BMC Genet. 18 (1), 14. doi:10.1186/s12863-017-0479-5
Qi, G., Han, C., Sun, Y., and Zhou, Y. (2020). Genetic Insight into Cytochrome P450 in Chinese from the Chinese Millionome Database. Basic Clin. Pharmacol. Toxicol. 126 (4), 341–352. doi:10.1111/bcpt.13356
Qi, G., Yin, S., Zhang, G., and Wang, X. (2020). Genetic and Epigenetic Polymorphisms of eNOS and CYP2D6 in mainland Chinese Tibetan, Mongolian, Uygur, and Han Populations. Pharmacogenomics J. 20 (1), 114–125. doi:10.1038/s41397-019-0104-2
Qian, J. C., Xu, X. M., Hu, G. X., Dai, D. P., Xu, R. A., Hu, L. M., et al. (2013). Genetic Variations of Human CYP2D6 in the Chinese Han Population. Pharmacogenomics 14 (14), 1731–1743. doi:10.2217/pgs.13.160
Ramos, E., Doumatey, A., Elkahloun, A. G., Shriner, D., Huang, H., Chen, G., et al. (2014). Pharmacogenomics, Ancestry and Clinical Decision Making for Global Populations. Pharmacogenomics J. 14 (3), 217–222. doi:10.1038/tpj.2013.24
Rieder, M. J., Reiner, A. P., Gage, B. F., Nickerson, D. A., Eby, C. S., McLeod, H. L., et al. (2005). Effect of VKORC1 Haplotypes on Transcriptional Regulation and Warfarin Dose. N. Engl. J. Med. 352 (22), 2285–2293. doi:10.1056/NEJMoa044503
Schwarz, J. M., Cooper, D. N., Schuelke, M., and Seelow, D. (2014). MutationTaster2: Mutation Prediction for the Deep-Sequencing Age. Nat. Methods 11 (4), 361–362. doi:10.1038/nmeth.2890
Sherry, S. T., Ward, M. H., Kholodov, M., Baker, J., Phan, L., Smigielski, E. M., et al. (2001). dbSNP: the NCBI Database of Genetic Variation. Nucleic Acids Res. 29 (1), 308–311. doi:10.1093/nar/29.1.308
Sivadas, A., Salleh, M. Z., Teh, L. K., and Scaria, V. (2017). Genetic Epidemiology of Pharmacogenetic Variants in South East Asian Malays Using Whole-Genome Sequences. Pharmacogenomics J. 17 (5), 461–470. doi:10.1038/tpj.2016.39
Sivadas, A., and Scaria, V. (2018). Pharmacogenomic Survey of Qatari Populations Using Whole-Genome and Exome Sequences. Pharmacogenomics J. 18 (4), 590–600. doi:10.1038/s41397-018-0022-8
Whirl-Carrillo, M., McDonagh, E. M., Hebert, J. M., Gong, L., Sangkuhl, K., Thorn, C. F., et al. (2012). Pharmacogenomics Knowledge for Personalized Medicine. Clin. Pharmacol. Ther. 92 (4), 414–417. doi:10.1038/clpt.2012.96
Wickham, H., Chang, W., and ggplot2, Henry. L. (2016). Elegant Graphics for Data Analysis. New York: Springer-Verlag.
Wishart, D. S., Feunang, Y. D., Guo, A. C., Lo, E. J., Marcu, A., Grant, J. R., et al. (2018). DrugBank 5.0: a Major Update to the DrugBank Database for 2018. Nucleic Acids Res. 46 (D1), D1074–d82. doi:10.1093/nar/gkx1037
Keywords: pharmacogenomics, genetic polymorphisms, allele frequency, whole-genome sequencing, the central Han Chinese population
Citation: Tian J, Zhang J, Yang Z, Feng S, Li S, Ren S, Shi J, Hou X, Xue X, Yang B, Xu H and Guo J (2022) Genetic Epidemiology of Medication Safety and Efficacy Related Variants in the Central Han Chinese Population With Whole Genome Sequencing. Front. Pharmacol. 12:790832. doi: 10.3389/fphar.2021.790832
Received: 07 October 2021; Accepted: 14 December 2021;
Published: 23 February 2022.
Edited by:
Chonlaphat Sukasem, Mahidol University, ThailandReviewed by:
Mohitosh Biswas, Rajshahi University, BangladeshCopyright © 2022 Tian, Zhang, Yang, Feng, Li, Ren, Shi, Hou, Xue, Yang, Xu and Guo. This is an open-access article distributed under the terms of the Creative Commons Attribution License (CC BY). The use, distribution or reproduction in other forums is permitted, provided the original author(s) and the copyright owner(s) are credited and that the original publication in this journal is cited, in accordance with accepted academic practice. No use, distribution or reproduction is permitted which does not comply with these terms.
*Correspondence: Hongen Xu, aG9uZ2VuX3h1QHp6dS5lZHUuY24=; Jiancheng Guo, Z2pjQHp6dS5lZHUuY24=
†These authors have contributed equally to this work
Disclaimer: All claims expressed in this article are solely those of the authors and do not necessarily represent those of their affiliated organizations, or those of the publisher, the editors and the reviewers. Any product that may be evaluated in this article or claim that may be made by its manufacturer is not guaranteed or endorsed by the publisher.
Research integrity at Frontiers
Learn more about the work of our research integrity team to safeguard the quality of each article we publish.