- 1Department of Pharmacology and Toxicology, KLE College of Pharmacy Belagavi, KLE Academy of Higher Education and Research (KAHER), Belagavi, India
- 2Pharmacology Research Center, Zahedan University of Medical Sciences, Zahedan, Iran
- 3Department of Chemistry, Faculty of Science, University of Sistan and Baluchestan, Zahedan, Iran
- 4Department of Chemistry, Iran University of Science and Technology, Tehran, Iran
- 5Department of Pharmaceutical Chemistry, Laureate Institute of Pharmacy, Kangra, India
- 6Faculty of Pharmaceutical Sciences, UCSI University, Kuala Lumpur, Malaysia
- 7Faculty of Pharmacy, BBDNIIT, Lucknow, India
- 8University Centre of Excellence in Research, Baba Farid University of Health Sciences, Faridkot, India
- 9AVIPS, Shobhit University, Saharanpur, India
- 10Department of Pharmacology, Dr. B.C. Roy College of Pharmacy and Allied Health Sciences, Durgapur, India
Aim: The present study aimed to investigate huperzine A as an anti-Alzheimer agent based on the principle that a single compound can regulate multiple proteins and associated pathways, using system biology tools.
Methodology: The simplified molecular-input line-entry system of huperzine A was retrieved from the PubChem database, and its targets were predicted using SwissTargetPrediction. These targets were matched with the proteins deposited in DisGeNET for Alzheimer disease and enriched in STRING to identify the probably regulated pathways, cellular components, biological processes, and molecular function. Furthermore, huperzine A was docked against acetylcholinesterase using AutoDock Vina, and simulations were performed with the Gromacs package to take into account the dynamics of the system and its effect on the stability and function of the ligands.
Results: A total of 100 targets were predicted to be targeted by huperzine A, of which 42 were regulated at a minimum probability of 0.05. Similarly, 101 Kyoto Encyclopedia of Genes and Genomes pathways were triggered, in which neuroactive ligand–receptor interactions scored the least false discovery rate. Also, huperzine A was predicted to modulate 54 cellular components, 120 molecular functions, and 873 biological processes. Furthermore, huperzine A possessed a binding affinity of −8.7 kcal/mol with AChE and interacted within the active site of AChE via H-bonds and hydrophobic interactions.
Introduction
Alzheimer’s disease (AD) is one of the neurodegenerative pathogeneses that majorly affect geriatric subjects, characterized by increased confusion and impaired cognitive function, and difficulty in learning and organizing thoughts (Bondi et al., 2017). Compromised cholinergic function, uplifted expression of β-amyloid, and unregulated oxidative stress are considered as the major hypotheses (Bondi et al., 2017; Grewal et al., 2021) via which the AD pathogenesis propagates. Also, deregulation of multiple neurotransmitter synapses and pathways has been traced for AD progression (Francis, 2005). Also, AD pathogenesis has been categorized as polygenic (Bird, 2008) due to the involvement of multiple genes in its progression.
One of the well-accepted approaches to manage AD includes acetylcholinesterase (AChE) inhibition, via which donepezil has been developed. However, donepezil is associated with multiple side effects like nausea, vomiting, weight loss, frequent urination, and muscle cramps. Also, other anti-Alzheimer’s drugs like N-methyl-d-aspartate antagonists, nicotine receptor agonists, peroxisome proliferator-activated receptor-γ agonists, and 5-hydroxytryptamine modulators are linked with multiple side effects (Casey et al., 2010). This underlines the necessity of identifying new therapeutic agents for the pharmacotherapy of AD.
Huperzine A is a naturally occurring sesquiterpene alkaloid, obtained from the extract of firmoss Huperzia serrata. In China, H. serrata is used in treating fever, swelling, and blood disorders. Additionally, it also exhibits neuroprotective properties and is under investigation as a possible agent to deal with the AD (PubChem, 2004). Furthermore, huperzine A has been reported for its neuroprotective effect (Wang and Tang 2005) and the possibility of AChE inhibition (Pohanka 2014). However, it is yet to be examined for its potentiality in regulating the multiple proteins and pathways based on the principle “a single compound can regulate multiple proteins and trigger various pathways related to them” (Khanal et al., 2021). Thus, it becomes imperative to investigate if huperzine A can trigger multiple proteins, so as to reveal its complex pharmacological spectra linked to AD. Hence, the present study aimed to investigate huperzine A as a possible candidate against AD, via the concept of “multi-protein pathways” interaction, by utilizing a series of system biology tools.
Materials and Methods
Gene Ontology Analysis
Canonical SMILES of huperzine A and donepezil were retrieved from the PubChem (https://pubchem.ncbi.nlm.nih.gov/) database and queried in SwissTargetPrediction (Daina et al., 2019; http://www.swisstargetprediction.ch/) to predict the possible targets. The regulated targets were matched with the recorded AD targets (DisGeNET entry: C0002395; https://www.disgenet.org/home/) and visualized using venny 2.1 (Oliveros, 2007-2015; https://bioinfogp.cnb.csic.es/tools/venny/). Furthermore, these targets were enriched in STRING (Szklarczyk et al., 2019; https://string-db.org/) to identify the possibly modulated cellular components, molecular functions, and biological processes. Also, huperzine A-regulated pathways were identified using the Kyoto Encyclopedia of Genes and Genomes (KEGG; https://www.genome.jp/kegg/) database.
Molecular Docking
In target prediction, huperzine A was predicted to possess the highest probability to regulate AChE, at a probability >0.9. Hence, it was considered further for molecular docking.
Ligand Preparation
The 3D structures of huperzine A and donepezil were retrieved from PubChem database in .sdf format and converted into .pdb format using Discovery Studio 2020 (Dassault Systèmes BIOVIA (2020) Discovery Studio, 2020; Dassault Systèmes, San Diego; https://discover.3ds.com/discovery-studio-visualizer-download). The energy of both the ligands was minimized using mmff94 force field (Halgren 1996) under conjugate gradient algorithm, using Open Babel at PyRx (https://pyrx.sourceforge.io/) ver. 0.8. Then, the molecules were converted into .pdbqt format, which was later used for docking as the ligand.
Macromolecule Preparation
The 3D crystallographic structure of AChE (PDB: 4EY7; Cheung et al., 2012) was retrieved from the RCSB protein data bank (https://www.rcsb.org/), which was in a complex with water molecules and other hetero-atoms. These pre-complex hetero-atoms or molecules were removed using Discovery Studio 2020 and saved in .pdb format, which was later used as the target for docking.
Ligand–Protein Docking
Both the ligands were docked against AChE using AutoDock Vina (Trott and Olson, 2010) by setting the grid box as center x, y, z = −2.895, −40.11, 30.76 and size x = 59.75, 61.24, 72.51. After docking, 10 different confirmations of both the ligands were obtained. The ligand with the minimum binding energy was chosen to visualize the ligand–protein interactions using Discovery Studio 2020.
Molecular Dynamics Simulations
To explore the part of the ligand responsible for layout within the active site of AChE and potential inhibitory activity, the best docking pose of both the ligands was chosen. Each of the selected ligand pose was initially simulated for 150-ns molecular dynamics (MD) simulations, in explicit water. The parameters for the ligands were then extracted from the general amber force field (Wang et al., 2004) using the Amber Tools package (https://ambermd.org/AmberTools.php). The partial charge on each atom was treated with a restrained electrostatic potential charge (Vanquelef et al., 2011). The conversion of AMBER topology and coordinate file was done with AnteChamberPYthon Parser interfacE (Sousa da Silva and Vranken, 2012; ACPYPE; https://www.bio2byte.be/acpype/). All the systems were fully solvated in the TIP3P water model, and ions were added to neutralize the net charge on the system. In the present study, the Gromacs 2020.2 package was utilized for the MD simulations (Van Der Spoel et al., 2005; http://www.gromacs.org/), and the Amber99sb-ildn force field was used to treat AChE (Beauchamp et al., 2012). Furthermore, periodic boundary conditions were applied in XYZ coordinate. Additionally, the energy on each system was minimized using steeped descent algorithm. The temperature equilibration of the systems was conducted in NVT ensemble, with 0.1-ps coupling constant, in a modified Berendsen thermostat (Berendsen et al., 1984) for 500 ps to maintain the temperature at 300 K. Also, 1-ns pressure equilibration was applied in NPT with the Berendsen Barostat (Berendsen et al., 1984), with a 2.0-ps coupling constant at 1 bar. The production was run in NPT, and a time step of 2 fs was followed, till the restraint was removed. The Particle Mesh Ewald (Darden et al., 1993) method interaction was used for the long-range electrostatic study. Furthermore, a cutoff of 12 Å for long-range and LINKS algorithms for H-bond constraints were applied (Hess et al., 1997). The equilibration and production run H-bond outlines between the ligand and AChE were calculated using the g H-bond module of Gromacs, as detailed previously (Zargari et al., 2018).
Principal Component and Dynamic Cross-Correlation Map Analysis
Principal component analysis (PCA) reveals the dominant modes in the motion of molecules by exploiting MD trajectories (Amadei et al., 1993; Amadei et al., 1996). This includes the elimination of the rotational and translational motion of the molecule using the least square fit to the reference structure. Furthermore, a covariance matrix is produced by a linear transformation of Cartesian coordinate space and also diagonalized to generate a set of eigenvectors to indicate the direction of the motion of the molecule. The eigenvalue corresponding to each eigenvector represents the energy contribution of that particular component to the motion. Projection of the trajectory on a particular eigenvector highlights the time-dependent motions that the components perform in a particular vibrational mode. The time average of the projection shows the contribution of components of the atomic vibrations to this mode of concerted motion (Van Aalten et al., 1995).
Dynamic cross-correlation map (DCCM) is a correlation map that identifies the correlated (positive or negative) motion between pairs of atoms by examining the magnitude of all the pairwise cross-correlation coefficients (McCammon & Harvey 1988). Herein, we have attempted to present each element of DCCM, in which Cij = 1, where the fluctuations of atoms i and j have the same period and same phase (positively correlated), whereas Cij = −1 and Cij = 0, respectively, indicate that the fluctuations of i and j are negatively correlated and not correlated.
Free Energy Calculations
One of the endpoint methods that possess an excellent ability to estimate the binding free energy of ligands in the binding site of receptors is the Molecular Mechanics Poisson–Boltzmann Surface Area (MM-PBSA) method (Cheatham et al., 1998; Kuhn & Kollman 2000), which calculates the binding free energy as
where the main form of interaction energy can be expressed as
where EMM is the molecular mechanical energy and is defined as
where Ebonded is a bond angle, is dihedral, and has improper interactions. The non-bonded interactions, i.e., Enon-bonded includes both electrostatic (Eelec) and van der Waals (Evdw) interactions, which were modeled using a Coulomb function and a Lennard–Jones potential function, respectively (Cullen et al., 1999). In the MM-PBSA, the energy of transferring the solute from vacuum into the solvent is solvation free energy, which is calculated using an implicit solvent model (Gilson & Honig 1988; Still et al., 1990) as
where Gpolar and Gnon-polar are the electrostatic and non-electrostatic contributions to the solvation free energy, respectively.
Furthermore, to obtain the binding free energy of the ligands bound to AChE, g_mmpbsa package (Ma et al., 2002; Kumari et al., 2014) was employed. To estimate the binding energy of each ligand, 150 frames from the last 120-ns MD simulations were extracted to calculate the binding energy and the contribution of each residue in the active sites of AChE.
Results
Target Prediction of Huperzine A
A total of 100 different proteins were predicted as targets of huperzine A, of which 42 were identified to be regulated at a minimum probability of 0.05 in which AChE was considered to be primarily targeted with the probability of 1. Similarly, a total of 49% of the regulated proteins were matched with the reported AD targets (DisGeNET entry: C0002395), with a total interaction of 1.4% (Figure 1). Similarly, the huperzine A-regulated targets were under seven different categories, i.e., hydrolase, kinase, protease, family A G protein-coupled receptor, ligand-gated ion channel, electrochemical transporter, and enzyme. Among these, family A G protein-coupled receptors were higher categories, i.e., 33.3% (Figure 2).
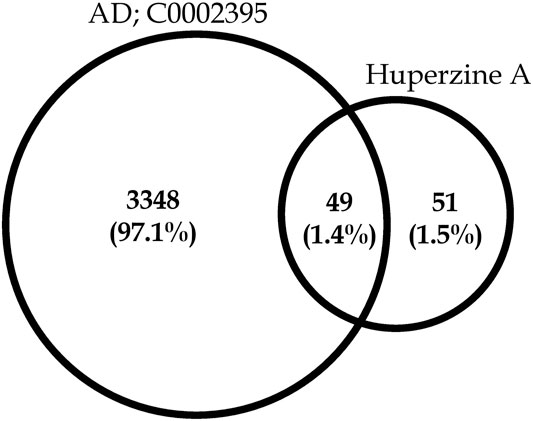
FIGURE 1. Venn diagram representing huperzine A-regulated proteins count concerning targets related to Alzheimer’s disease (C0002395).
Gene Ontology Analysis
A total of 3 Gene Ontology (GO) terms, i.e., cellular components, molecular function, and biological processes, including KEGG pathway(s), were evaluated. The protein–protein interactions of huperzine A-regulated targets are presented in Figure 3, which are based on known interactions (curated databases and experimentally determined), predicted interactions (gene neighborhood, gene fusions, and gene co-occurrence), and miscellaneous (text mining, co-expression, and protein homology).
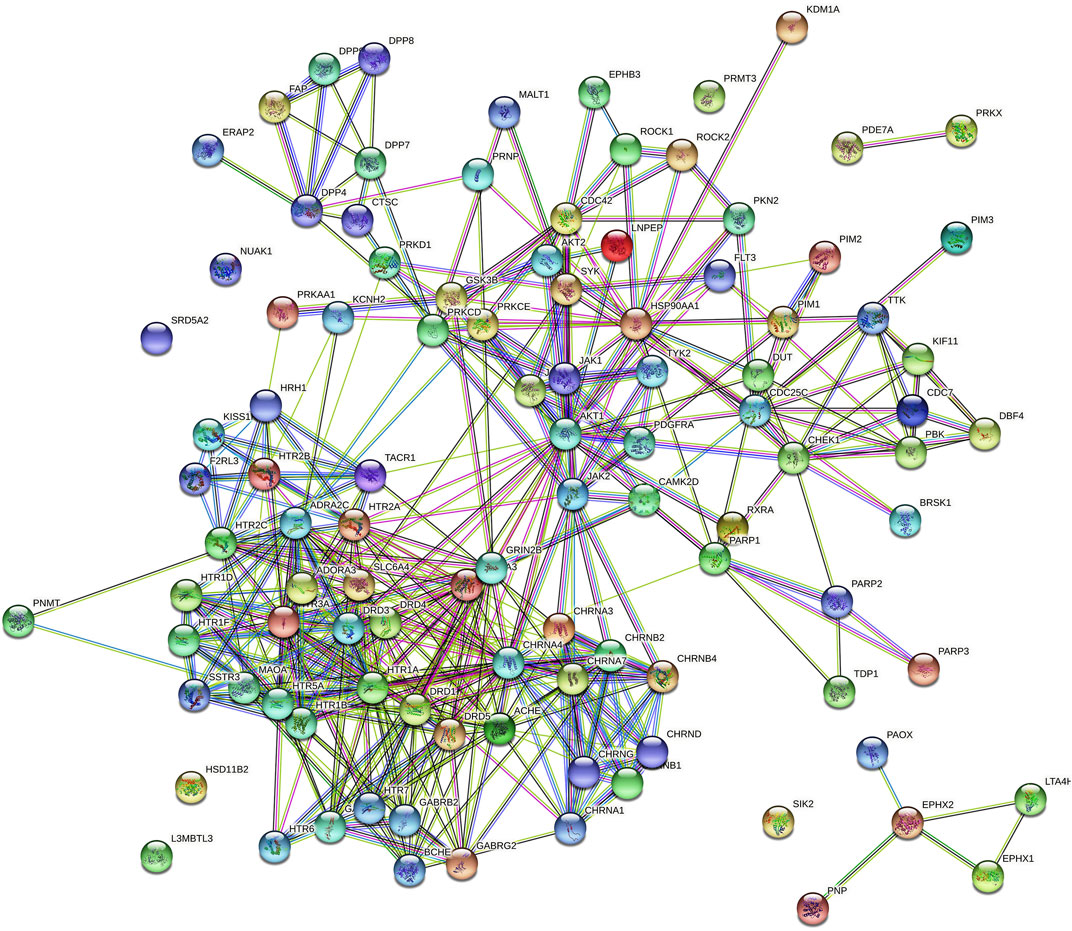
FIGURE 3. colored nodes: query proteins and first shell of interactors,
white nodes: second shell of interactors, Node content;
empty nodes: proteins of unknown 3D structure,
filled nodes: some 3D structure is known or predicted, Known Interactions;
from curated databases,
experimentally determined, Predicted Interactions;
gene neighborhood,
gene fusions,
gene co-occurrence and Others;
text mining,
co-expression,
protein homology.
Furthermore, pathway neuroactive ligand–receptor interaction (KEGG entry: hsa04080) was predicted to be primarily modulated via regulation of 33 genes (DRD4, KISS1R, F2RL3, CHRND, HTR2B, CHRNA1, CHRNB4, GABRB2, HTR2C, HTR5A, HTR6, TACR1, CHRNB1, DRD5, CHRNA3, HTR1A, HTR1F, HTR7, CHRNB2, HTR1B, CHRNA4, HTR1D, DRD3, CHRNG, DRD1, HRH1, ADRA2C, GABRA1, CHRNA7, GABRG2, HTR2A, GRIN2B, and SSTR3) under 272 background genes, at a false discovery rate of 3.18E−32 among the 101 predicted pathways. Also, a total of 573 genes were modulated in which AKT 1 and 2 appeared in 68 different pathways. The top 20 huperzine A-regulated KEGG pathways are presented in Figure 4.
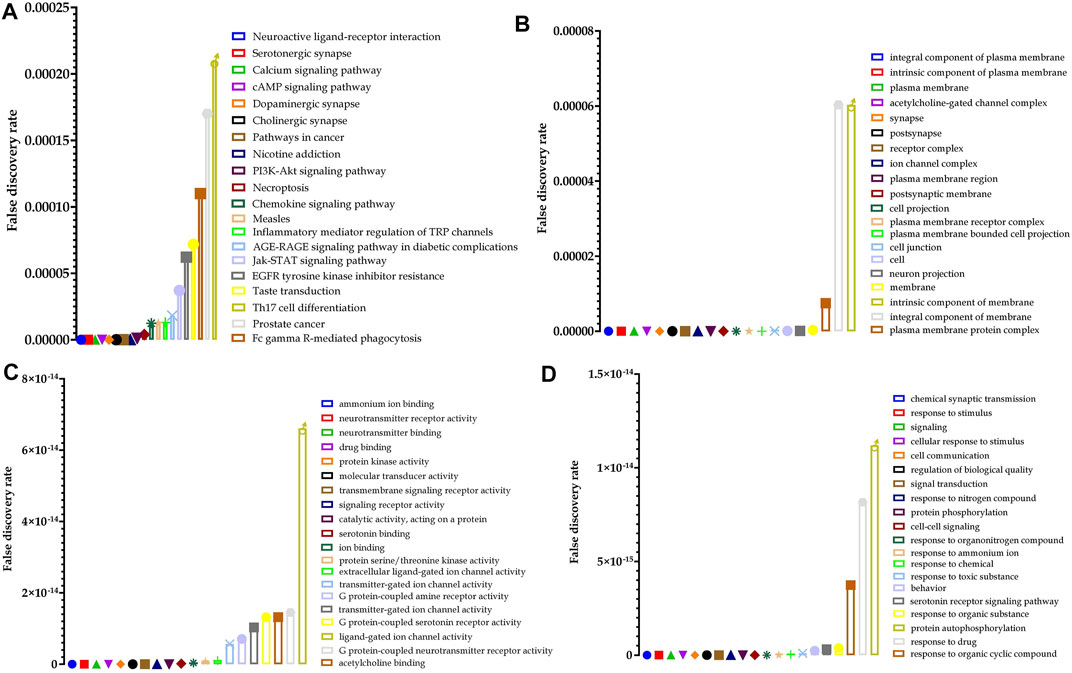
FIGURE 4. Top 20 huperzine A-regulated (A) Kyoto Encyclopedia of Genes and Genomes (KEGG) pathways, (B) cellular components, (C) molecular function, and (D) biological processes along with their false discovery rate.
Similarly, 54 cellular components were predicted to be modulated via the regulation of 41 genes (DRD4, LNPEP, KISS1R, FLT3, F2RL3, PDGFRA, CHRND, HTR2B, CHRNA1, SLC6A4, CHRNB4, KCNH2, SLC6A3, GABRB2, HTR2C, HTR5A, HTR6, TACR1, CHRNB1, DRD5, CHRNA3, HTR1A, HTR1F, EPHB3, HTR7, CHRNB2, ADORA3, HTR1B, CHRNA4, HTR1D, DRD3, CHRNG, DRD1, HRH1, ADRA2C, GABRA1, CHRNA7, GABRG2, HTR2A, GRIN2B, and SSTR3) under 1,564 background genes at a false discovery rate of 3.10E−16. Furthermore, 120 molecular functions were identified in which ammonium ion binding was majorly modulated via the regulation of 26 (DRD4, CHRND, HTR2B, CHRNA1, SLC6A4, CHRNB4, BCHE, SLC6A3, HTR2C, HTR5A, ACHE, CHRNB1, DRD5, CHRNA3, HTR1A, HTR1F, HTR7, HTR3A, CHRNB2, HTR1B, CHRNA4, HTR1D, DRD3, DRD1, CHRNA7, and HTR2A) genes under 66 background proteins, at a false discovery rate of 4.70E−36. Additionally, a total of 873 biological processes were predicted to be regulated by huperzine A, in which the chemical synaptic transmission was primarily modulated via the regulation of 32 genes (DRD4, CHRND, CHRNA1, SLC6A4, CHRNB4, SLC6A3, GABRB2, HTR2C, HTR6, ACHE, TACR1, CHRNB1, DRD5, BRSK1, CHRNA3, HTR1F, GSK3B, HTR7, CHRNB2, HTR1B, CHRNA4, HTR1D, DRD3, CHRNG, DRD1, GABRA1, CHRNA7, GABRG2, HTR2A, AKT1, GRIN2B, and SSTR3) against 402 background genes, at a false discovery rate of 1.67E−24. The 25 hits of GO terms under each category are presented in Figure 4.
Molecular Docking
Molecular docking revealed that huperzine A possessed binding affinity with AChE (binding energy of −8.7 kcal/mol) via 2 H-bond interactions with Tyr382 (22.69) and Ala528 (26.88). Similarly, donepezil showed a binding affinity of −9.4 kcal/mol and had no H-bond interaction but possessed Pi-Pi stacked with Tyr341 (4.35) and Pi-alkyl with Trp286 (5.40) of the benzene and heterocyclic rings, respectively. The interactions of donepezil and huperzine A with AChE are presented in Figure 5.
Molecular Dynamics Simulations
Structural Analysis
In the present study, the root-mean-square deviation (RMSD) of Cα atoms of protein backbones was monitored throughout 150-ns MD simulations (Figure 6). The RMSD analysis showed that both ligands, i.e., donepezil and huperzine A, which were bound with AChE, began to relax at ∼20 ns. The RMSD values of AChE-bound ligands fluctuated within 0.5 Å as compared with the docking pose. The root-mean-square fluctuation (RMSF) is defined as the change in the position of every single atom from its average position. The RMSF of MD systems (130-ns simulations) as a function of protein is presented in Figure 7.
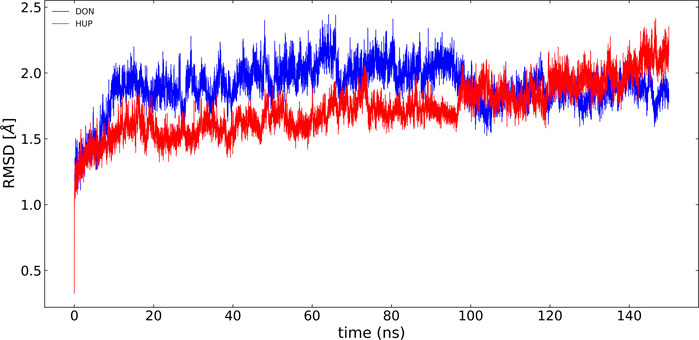
FIGURE 6. RMSD of acetylcholinesterase (AChE) with donepezil and huperzine A via least-square fit to the backbone of starting structure.
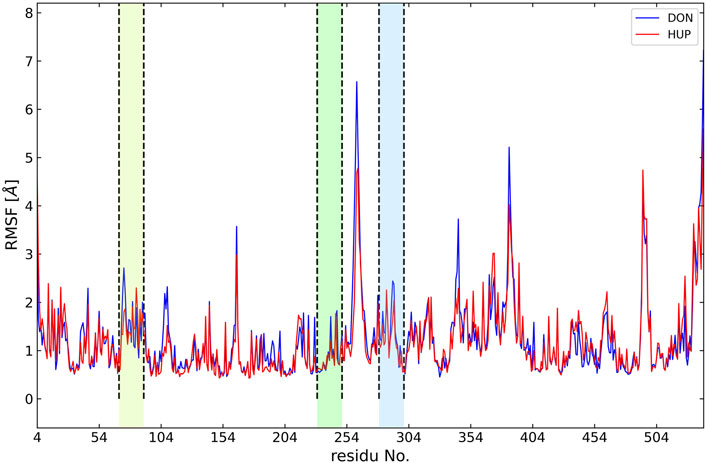
FIGURE 7. Root-mean-square fluctuation (RMSF) of all molecular dynamics systems throughout 130 ns of simulation as a function of protein residue number. The active site of the protein is depicted as vertical regions.
One of the descriptive plots obtained from molecular motion is DCCM. This can address the structural motions of an enzyme in its bound and unbound states. However, the PCA is used to construct the correlation matrix for all Cα atoms. It can also be used to assess required eigenvectors to describe the most essential motion of AChE. In our model for DCCM, the first 2 principal components (PCs) were used to generate correlation motion for each structure. Figure 8 illustrates the DCCMs for the unbound state of AChE as well as the bound state with donepezil and huperzine A. In Figure 8, different forms of motions can be observed for the naked state of the enzyme as compared with its bound states. Both donepezil and huperzine A changed the fluctuations of the first 300 residues, including the active site of the enzyme, but donepezil changed all correlated motions into anticorrelated ones when it binds. Huperzine A, on the other hand, changes the anticorrelated motions observed in the apostate into correlated ones, especially in residues 100–300 (Figure 8).
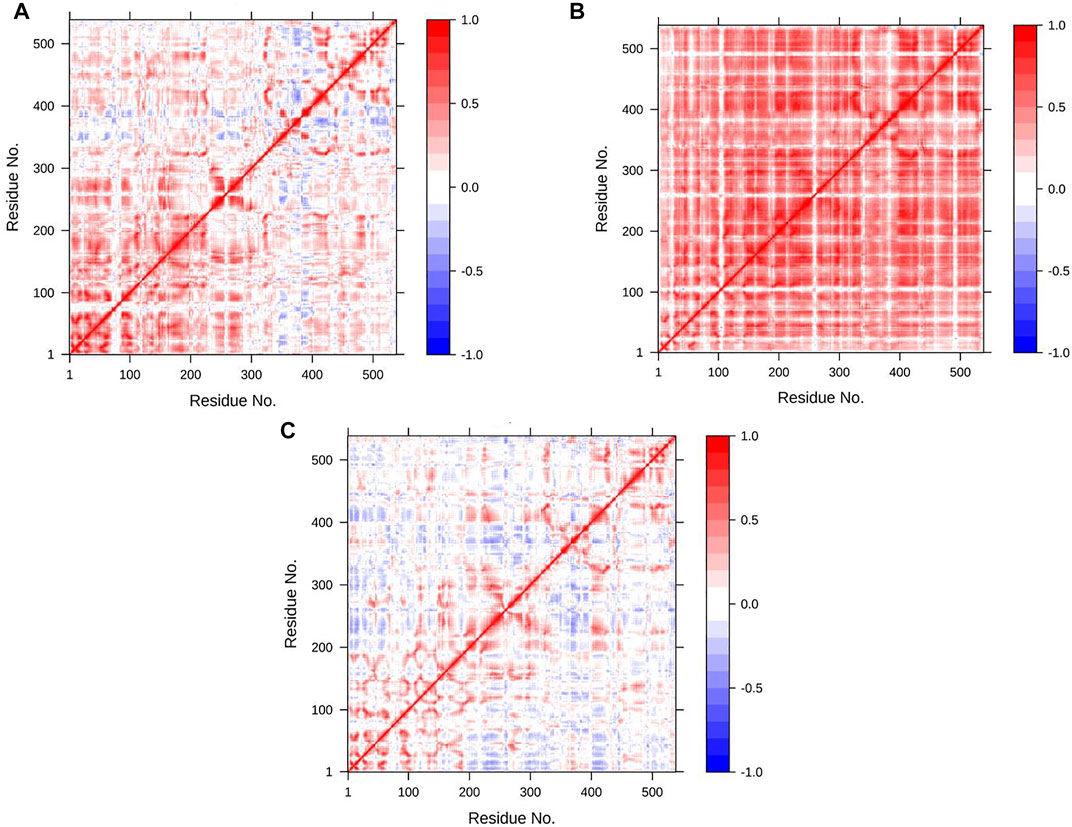
FIGURE 8. Dynamic cross-correlation map (DCCM) for acetylcholinesterase (AChE) in (A) apo state, (B) AChE/donepezil, and (C) AChE/huperzine A complexes. The positive regions, which are colored in red, are indications of strongly correlated motions of Cα atoms (Cij =1), while the negative regions, which are colored blue, are associated with the anticorrelated movements (Cij = −1).
Besides the correlation map analysis, which depicts the movements of every atom of the protein, one needs to inspect the overall collective motions that are responsible for the dominant protein’s conformational changes. This can be achieved via the projection of conformations onto the orthogonal collective motions (eigenvectors) output of PCs. In Figure 9, the first main PC (PC1) for protein bound to the inhibitors and its apo form is presented. The vector field representation in PyMOL was used to present the direction and magnitude of each Cα atom in PC1. In the enzyme apo form, the large-magnitude motions were observed within the amino acid residues of the active site of the protein (depicted as rectangles in Figure 9A), primarily belonging to the loops. However, these collective motions vanished in the presence of donepezil and huperzine A, indicating the role of loops in the overall movement of the protein and its function. In Figures 9 (D–F), the contribution of each residue to the PC1 (red) and PC2 (blue) for protein in bound and unbound states is depicted. As depicted in Figure 9D, protein adopts a large magnitude of motion within the active site to the protein wherein donepezil and huperzine A are predicted to bind (residues 70–90 and 360–400). However, these movements were drastically diminished when donepezil (Figure 9E) and huperzine A (Figure 9F) bound to the enzyme. In addition, dominant interactions to stabilize donepezil were within the active site of AChE H-bonds, through the phenolic and piperidine moieties with Tyr449, Tyr337, and Gly342 (Figure 10A). Also, huperzine A seemed to act within the active site of AChE to have an H-bond with Asp74 and 2 hydrophobic interactions with Thr337 and Tyr124 (Figure 10B).
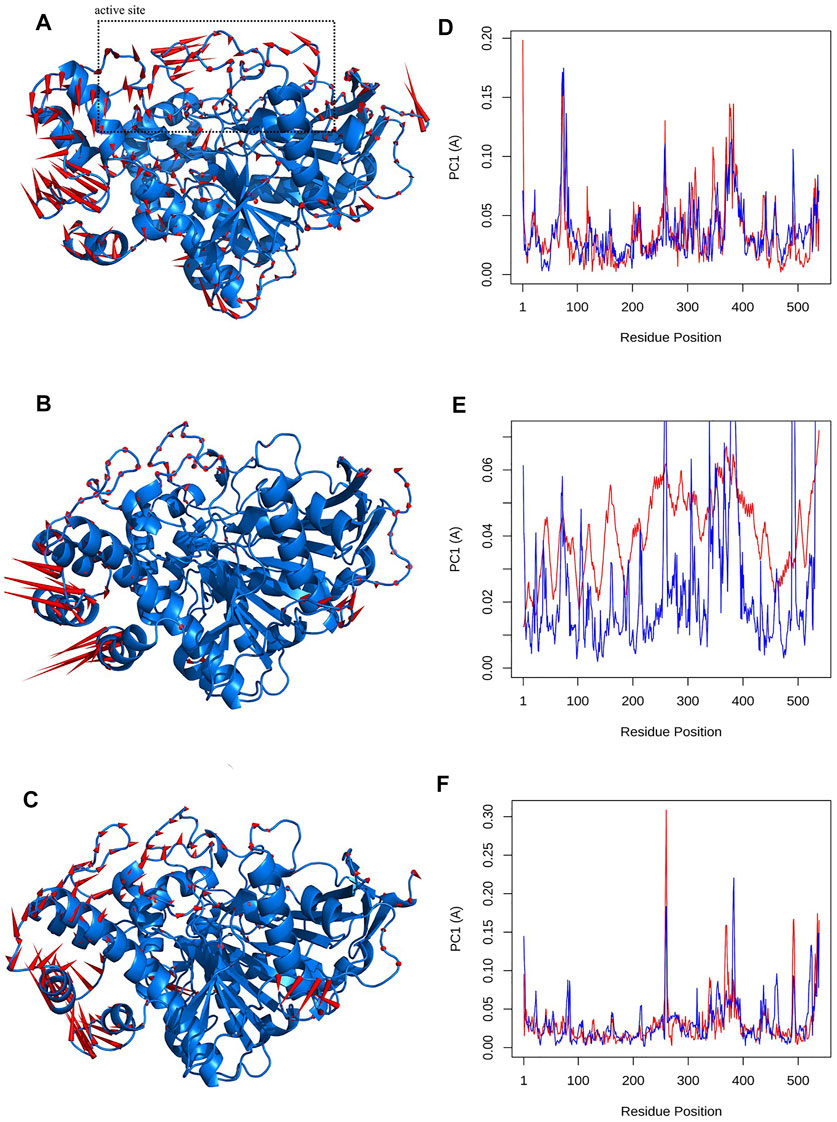
FIGURE 9. Visualizations of first principal component (PC1) for (A) apo state, (B) donepezil and, (C) huperzine A bound to acetylcholinesterase (AChE). The Q20 residue-wise plots for PC1 (red) and PC2 (blue) for (D) AChE in Q20 apo state. (E) AChE–donepezil and (F) AChE–huperzine A are also represented.
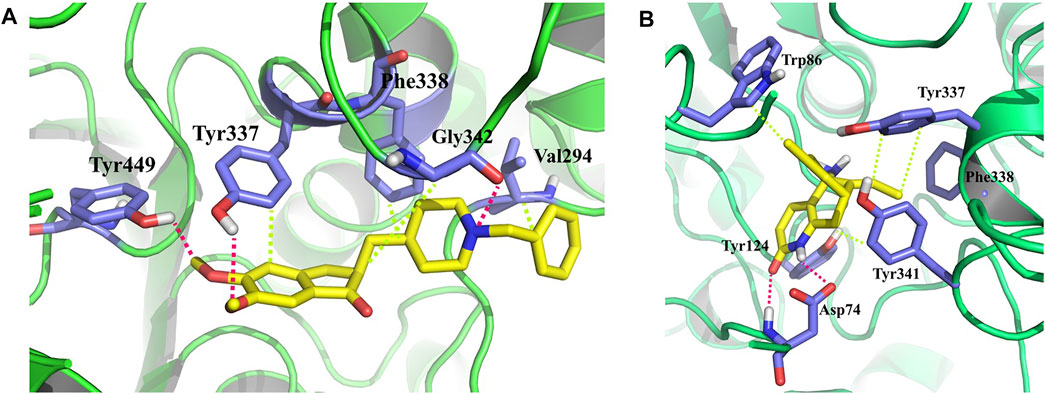
FIGURE 10. 3D representation of representative structures of (A) donepezil and (B) huperzine A in the active site of acetylcholinesterase (AChE). Hydrophobic interaction is depicted as lime dash lines, whereas the H-bond is illustrated as a red dash line.
H-Bond Analysis
To evaluate quantitatively the firmness of H-bonds between donepezil and huperzine A with AChE, MD analysis of ligand–AChE stability was monitored during the 150 ns of the trajectory period. The threshold for H-bond was 3.5 Å with 30 ∠ (different from the PLIP server mentioned above), as summarized in Table 1. Furthermore, huperzine A possessed strong H-bonds with the amino acid residues within the active site of AChE. The higher occupancies regarding O1 and N2 atoms in huperzine A involved H-bonds with Asp74 and Ser125. The percent of occupancy showed the number of frames in which the particular H-bond had been monitored, out of all the processed frames. Also, donepezil interacted with residues in the active site of AChE occupying Tyr124, Tyr337, and Ser198.
Binding Free Energy and Energy Decomposition Analysis
The binding affinity of both the ligands was evaluated based on the binding free energy using the MM-PBSA method. Table 2 summarizes the details of the contribution in terms of the binding free energies for the inhibitors as defined by Eqs 1–4. The binding affinity of donepezil with AChE was observed to be higher as compared with huperzine A.
Furthermore, it was observed that Van der Waals’s energy strongly favored the stability of donepezil within the active site of AChE. The electrostatic energy favored just the huperzine A ligand in AChE protein, while the SASA energy asserted almost the same effect on both ligands.
Another practical statistic is that the g_mmpbsa tool helps to discover the reciprocal contribution of each residue to the binding free energy of AChE–ligand complexes, based on the dynamic of systems, as depicted in Figure 11. In the case of donepezil, decomposition analysis revealed that Tyr341, Phe338, Trp286, Glu202, and Asp74 had a considerable contribution to the binding energy of this ligand within the active site of AChE. Also, Glu202, Tyr341, Glu450, Glu452, Glu334, and Phe338 favored the binding energy of huperzine A.
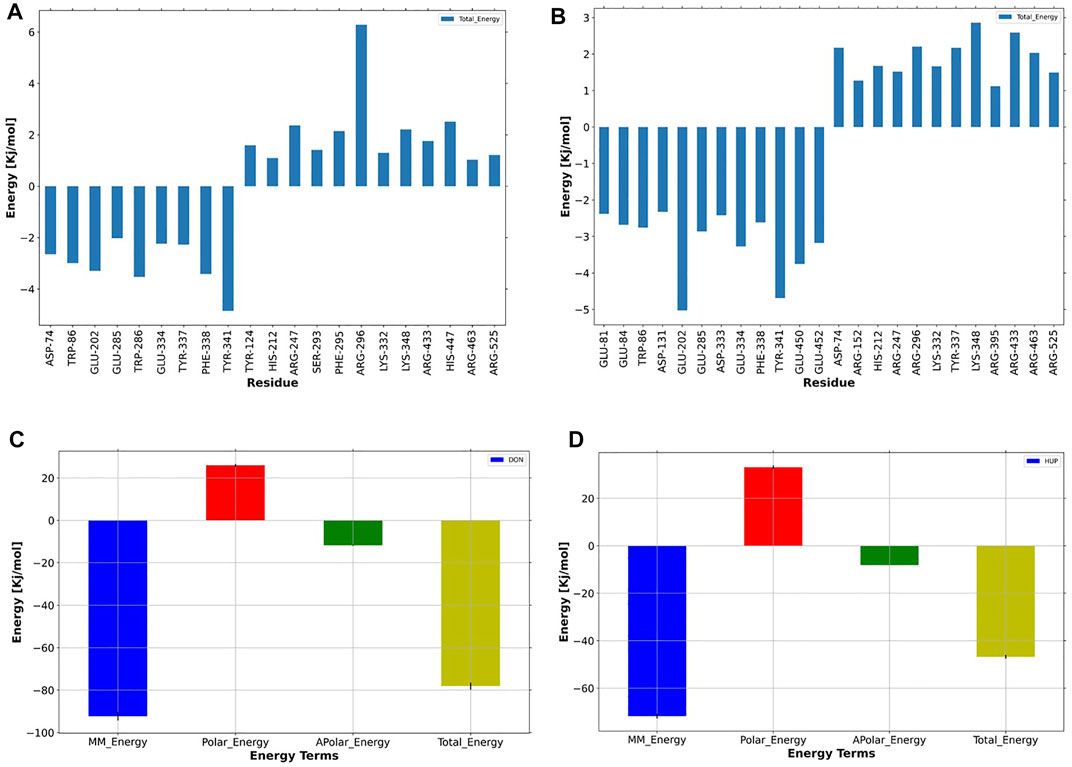
FIGURE 11. Per-residue binding energy decomposition of predicted (A) donepezil/acetylcholinesterase (AChE) and (B) huperzine A/AChE. The energy contribution larger than ±1 kcal/mol is displayed. The blue bars show the residues with an absolute binding free energy. Energy decomposition of each ligand versus energy terms depicted for (C) donepezil and (D) huperzine A, where MM, polar, nonpolar, and total energies of ligand contribution to the binding free energies are colored as dark-blue, red, green, and yellow, respectively.
Discussion
The present study utilized a series of system biology tools to evaluate the efficacy of huperzine A against AD. Initially, huperzine A-modulated targets were identified, which were later enriched in STRING. Furthermore, huperzine A was also docked against AChE, as it was predicted to be majorly modulated with the highest probability, followed by MD simulations.
AChE has been identified as the major target against AD, on which multiple selective or non-selective AChE inhibitors have been established (Russ and Morling, 2012), which check the hydrolysis of acetylcholine. Also, AChE deregulation has been demonstrated in multiple neurodegenerative pathogeneses (Mushtaq et al., 2014). Thus, AChE inhibition may help in managing the pathogenesis of AD. Therefore, the present study investigated the probability and potential of huperzine A to act over AChE.
Though the concept of “lock and key” is an established approach to develop new therapeutic agents against various diseases, it is to be understood that a “single compound may regulate multiple proteins” with the concept of “master key can unlock multiple locks” (Patwardhan 2014; Duyu et al., 2021; Khanal & Patil 2021), in which the master key can be conceptualized for its broad-spectrum pharmacological activity. Based on this theory, huperzine A-regulated targets were enriched, which identified a total of 101 different pathways. Among them, neuroactive ligand–receptor interaction was predicted to be majorly modulated, with the least false discovery rate. Overall, two main proteins, i.e., Akt 1 and 2, were identified to be common in a total of 68 multiple pathways.
KEGG pathway classifies neuroactive ligand–receptor interaction (KEGG entry: hsa04080; https://www.genome.jp/dbget-bin/www_bget?hsa04080) as an environmental information processor and also contributes to the interaction of signaling molecules, based on which multiple centrally acting drugs over serotonin, dopamine, adrenergic, and cell surface glycoproteins are established. Furthermore, its ortholog presents the association of this pathway (KEGG entry: ko04080; https://www.genome.jp/dbget-bin/www_bget?ko04080) with muscarinic, adrenergic, dopamine, histamine, serotonin, gamma-aminobutyric acid (GABA), nicotinic, and glutamine receptors, which have a direct or indirect contribution in various neurodegenerative pathogeneses. Thus, it may be assumed that the efficacy of huperzine A to modulate these surface receptors can be utilized against AD, as the approach has been approved to target the above-mentioned proteins in dealing AD. Additionally, serotonergic synapse (KEGG entry: hsa04726; https://www.genome.jp/dbget-bin/www_bget?pathway+hsa04726), cholinergic synapse (KEGG entry: hsa04725; https://www.genome.jp/entry/pathway+hsa04725), and nicotine addiction (KEGG entry: hsa05033; https://www.genome.jp/dbget-bin/www_bget?hsa05033) are also identified, which are directly involved in the progression of AD pathogenesis.
Furthermore, GO analysis outlined the multiple terms concerning the various neurodegenerative diseases, including AD. In the cellular components, it was identified that the acetylcholine-gated channel complex (GO:0005892) and the GABA-A receptor complex (GO:1902711) may get regulated via huperzine A-regulated protein–protein interaction. In AD, there is a malfunction of the surface receptor(s) and an increase in β-amyloid deposition (Murphy and LeVine, 2010). In the present study, huperzine A-regulated proteins showed efficacy to trigger the neurotransmitter receptor activity (GO:0030594), neurotransmitter binding (GO:0042165), G protein-coupled serotonin receptor activity (GO:0004993), acetylcholine binding (GO:0042166), G protein-coupled neurotransmitter receptor activity (GO:0099528), and β-amyloid binding (GO:0001540) and could thus ameliorate AD.
Owing to the complex pathogenesis of AD, attributed to the deregulation of multiple signaling pathways (Calabrò et al., 2020), it has been categorized as a polygenic pathogenesis (Bird 2008). Previously, decreased memory (Jahn 2013), altered β-amyloid response (Rauch et al., 2011), and deregulated serotonin (Ouchi et al., 2009), dopamine (Pan et al., 2019), and choline pathways (Whiley et al., 2014) had been linked with AD. In the present study, we observed the regulation of serotonin receptor signaling pathway (GO:0007210), cholinergic synaptic transmission (GO:0007271), memory (GO:0007613), dopamine metabolic process (GO:0042417), acetylcholine receptor signaling pathway (GO:0095500), and response to β-amyloid (GO:1904645) as the multiple biological spectra against AD.
AChE has been reported to be the major target to manage AD, based on which multiple cholinesterase inhibitors, including donepezil, were established (Mushtaq et al., 2014). Inhibitors of these enzymes monitor the AChE function (Nordberg et al., 2013; Mushtaq et al., 2014) and regulate the hydrolysis of acetylcholine. In target prediction, huperzine A was identified to act primarily over AChE. Hence, the independent action of huperzine A over AChE was evaluated via molecular docking, which showed that the binding affinity of huperzine A was almost nearer to a clinically practiced gold standard cholinesterase inhibitor (donepezil). Thus, it was further corroborated with MD simulation evaluation.
Structural analyses, such as RMSD and RMSF, are efficient tools to examine the molecular interaction effectiveness of molecules (Khanal et al., 2021). In the MD simulations, the dynamics of AChE in apo forms were altered, as compared with the bound states, due to the binding site occupation by the ligands whose illustrations reflected the swinging of the docking pose within 0.5–0.7 Å for the complex indicating the docking poses to be reliable. Furthermore, clustering analysis is chiefly used to discover protein conformations by diminishing the size of the problem of conformational analysis by dividing the conformations gathered into separate groups (Knaggs et al., 2007). The mid-point structure is commonly used to establish the conformations, within a specific cluster to secure the cluster representative, which is a physically sensible structure (Knaggs et al., 2007). Conformational clustering was performed on the trajectories obtained from the MD simulations of the ligand–AChE complex to select the representative conformations for further experimentation. Clustering was performed using Cα backbone atoms, least-squares alignment, and the Gromos algorithm (Daura et al., 1999), at a cutoff of 0.15 nm, by the g_cluster module as implemented in Gromacs 2020.2. However, the center structures were the most populated. Hence, the more stable clusters of each complex were considered to be representatives of the binding mode conformations.
It was observed that two factors, i.e., H-bond and hydrophobic forces, were important for the stabilization of ligands within the active sites of AChE, as showed by the donepezil–AChE stabilization via the hydrogen and hydrophobic interactions. Similarly, the huperzine A–AChE complex was also observed within the same active site, stabilized via hydrogen and hydrophobic interactions.
Further, it was observed that the binding energy in molecular docking and MD simulations of both the ligands showed that donepezil had a higher binding affinity as compared with huperzine A. However, not limiting to AChE inhibition, huperzine A was predicted to be involved with regulating multiple pathways, and biological processes and functions that are related to AD.
Conclusion
The study revealed that huperzine A regulated the multiple proteins and pathways concerning AD. Also, it revealed the probability to act over various neurotransmitter synapse and metabolic pathways, other than the direct action over the AChE. Since the present outcomes are derived from multiple computational approaches, the findings need to be validated via the in vitro and in vivo experimental approaches. Thus, the study not only establishes the potential of huperzine A in AD through in silico studies but also provides a lead to the modification of the structure of huperzine A to enhance its binding affinity with AChE.
Data Availability Statement
The raw data supporting the conclusions of this article will be made available by the authors, without undue reservation.
Author Contributions
PK performed the experiments (GO analysis and molecular docking) and drafted, reviewed, and edited the manuscript. FZ and BF contributed to the molecular dynamics simulation study. YD generated the concept of the study and supervised the work. MR and YM contributed to the literature survey. DK and SS contributed to drafting and revising the manuscript. MS, NJ, PB, and RS helped in the preparation of the manuscript. All authors of this manuscript have read and approved the manuscript for submission.
Conflict of Interest
The authors declare that the research was conducted in the absence of any commercial or financial relationships that could be construed as a potential conflict of interest.
Publisher’s Note
All claims expressed in this article are solely those of the authors and do not necessarily represent those of their affiliated organizations, or those of the publisher, the editors, and the reviewers. Any product that may be evaluated in this article, or claim that may be made by its manufacturer, is not guaranteed or endorsed by the publisher.
References
Amadei, A., Linssen, A. B., and Berendsen, H. J. (1993). Essential Dynamics of Proteins. Proteins 17 (4), 412–425. doi:10.1002/prot.340170408
Amadei, A., Linssen, A. B., de Groot, B. L., van Aalten, D. M., and Berendsen, H. J. (1996). An Efficient Method for Sampling the Essential Subspace of Proteins. J. Biomol. Struct. Dyn. 13 (4), 615–625. doi:10.1080/07391102.1996.10508874
Beauchamp, K. A., Lin, Y. S., Das, R., and Pande, V. S. (2012). Are Protein Force Fields Getting Better? A Systematic Benchmark on 524 Diverse NMR Measurements. J. Chem. Theor. Comput 8 (4), 1409–1414. doi:10.1021/ct2007814
Berendsen, H. J. C., Postma, J. P. M., van Gunsteren, W. F., DiNola, A., and Haak, J. R. (1984). Molecular Dynamics with Coupling to an External bath. J. Chem. Phys. 81 (8), 3684–3690. doi:10.1063/1.448118
Bird, T. D. (2008). Genetic Aspects of Alzheimer Disease. Genet. Med. 10 (4), 231–239. doi:10.1097/GIM.0b013e31816b64dc
Bondi, M. W., Edmonds, E. C., and Salmon, D. P. (2017). Alzheimer's Disease: Past, Present, and Future. J. Int. Neuropsychol. Soc. 23 (9-10), 818–831. doi:10.1017/S135561771700100X
Calabrò, M., Rinaldi, C., Santoro, G., and Crisafulli, C. (2021). The Biological Pathways of Alzheimer Disease: a Review. AIMS Neurosci. 8 (1), 86–132. doi:10.3934/Neuroscience.2021005
Casey, D. A., Antimisiaris, D., and O'Brien, J. (2010). Drugs for Alzheimer's Disease: Are They Effective? P T 35 (4), 208–211.
Cheatham, T. E., Srinivasan, J., Case, D. A., and Kollman, P. A. (1998). Molecular Dynamics and Continuum Solvent Studies of the Stability of polyG-polyC and polyA-polyT DNA Duplexes in Solution. J. Biomol. Struct. Dyn. 16 (2), 265–280. doi:10.1080/07391102.1998.10508245
Cheung, J., Rudolph, M. J., Burshteyn, F., Cassidy, M. S., Gary, E. N., Love, J., et al. (2012). Structures of Human Acetylcholinesterase in Complex with Pharmacologically Important Ligands. J. Med. Chem. 55 (22), 10282–10286. doi:10.1021/jm300871x
Cullen, M. H., Billingham, L. J., Woodroffe, C. M., Chetiyawardana, A. D., Gower, N. H., Joshi, R., et al. (1999). Mitomycin, Ifosfamide, and Cisplatin in Unresectable Non-small-cell Lung Cancer: Effects on Survival and Quality of Life. J. Clin. Oncol. 17 (10), 3188–3194. doi:10.1200/JCO.1999.17.10.3188
Daina, A., Michielin, O., and Zoete, V. (2019). SwissTargetPrediction: Updated Data and New Features for Efficient Prediction of Protein Targets of Small Molecules. Nucleic Acids Res. 47 (W1), W357–W364. doi:10.1093/nar/gkz382
Darden, T., York, D., and Pedersen, L. (1993). Particle Mesh Ewald: AnN⋅Log(N) Method for Ewald Sums in Large Systems. J. Chem. Phys. 98 (12), 10089–10092. doi:10.1063/1.464397
Dassault Systèmes BIOVIA (2020). Discovery Studio, 2019. San Diego: DassaultSystèmes. Available at https://discover.3ds.com/discovery-studio-visualizer-download.
Daura, X., Gademann, K., Jaun, B., Seebach, D., Van Gunsteren, W. F., and Mark, A. E. (1999). Peptide Folding: when Simulation Meets experiment. Angew. Chem. Int. Ed. 38 (1‐2), 236–240. doi:10.1002/(sici)1521-3773(19990115)38:1/2<236:aid-anie236>3.0.co;2-m
Duyu, T., Khanal, P., Dey, Y. N., and Jha, S. K. (2021). Network Pharmacology of Withania Somnifera against Stress Associated Neurodegenerative Diseases. Adv. Tradit Med. (Adtm) 21, 565–578. doi:10.1007/s13596-020-00530-x
Francis, P. T. (2005). The Interplay of Neurotransmitters in Alzheimer's Disease. CNS Spectr. 10 (11 Suppl. 18), 6–9. doi:10.1017/s1092852900014164
Gilson, M. K., and Honig, B. (1988). Calculation of the Total Electrostatic Energy of a Macromolecular System: Solvation Energies, Binding Energies, and Conformational Analysis. Proteins 4 (1), 7–18. doi:10.1002/prot.340040104
Grewal, A. K., Singh, T. G., Sharma, D., Sharma, V., Singh, M., Rahman, M. H., et al. (2021). Mechanistic Insights and Perspectives Involved in Neuroprotective Action of Quercetin. Biomed. Pharmacother. 140, 111729. doi:10.1016/j.biopha.2021.111729
Halgren, T. A. (1996). Merck Molecular Force Field. I. Basis, Form, Scope, Parameterization, and Performance of MMFF94. J. Comput. Chem. 17 (5‐6), 490–519. doi:10.1002/(sici)1096-987x(199604)17:5/6<490:aid-jcc1>3.0.co;2-p
Hess, B., Bekker, H., Berendsen, H. J. C., and Fraaije, J. G. E. M. (1997). LINCS: a Linear Constraint Solver for Molecular Simulations. J. Comput. Chem. 18 (12), 1463–1472. doi:10.1002/(sici)1096-987x(199709)18:12<1463:aid-jcc4>3.0.co;2-h
Jahn, H. (2013). Memory Loss in Alzheimer's Disease. Dialogues Clin. Neurosci. 15 (4), 445–454. doi:10.31887/DCNS.2013.15.4/hjahn
Khanal, P., and Patil, B. M. (2021). Consolidation of Network and Experimental Pharmacology to Divulge the Antidiabetic Action of Ficus Benghalensis L. Bark. 3 Biotech. 11 (5), 238. doi:10.1007/s13205-021-02788-7
Khanal, P., Dey, Y. N., Patil, R., Chikhale, R., Wanjari, M. M., Gurav, S. S., et al. (2021). Combination of System Biology to Probe the Anti-viral Activity of Andrographolide and its Derivative against COVID-19. RSC Adv. 11 (9), 5065–5079. doi:10.1039/D0RA10529E
Knaggs, M. H., Salsbury, F. R., Edgell, M. H., and Fetrow, J. S. (2007). Insights into Correlated Motions and Long-Range Interactions in CheY Derived from Molecular Dynamics Simulations. Biophys. J. 92 (6), 2062–2079. doi:10.1529/biophysj.106.081950
Kuhn, B., and Kollman, P. A. (2000). Binding of a Diverse Set of Ligands to Avidin and Streptavidin: an Accurate Quantitative Prediction of Their Relative Affinities by a Combination of Molecular Mechanics and Continuum Solvent Models. J. Med. Chem. 43 (20), 3786–3791. doi:10.1021/jm000241h
Kumari, R., Kumar, R., and Lynn, A. (2014). g_mmpbsa-A GROMACS Tool for High-Throughput MM-PBSA Calculationsg_mmpbsa-Aa GROMACS Tool for High-Throughput MM-PBSA Calculations. J. Chem. Inf. Model. 54 (7), 1951–1962. doi:10.1021/ci500020m
Ma, C., Baker, N. A., Joseph, S., and McCammon, J. A. (2002). Binding of Aminoglycoside Antibiotics to the Small Ribosomal Subunit: a Continuum Electrostatics Investigation. J. Am. Chem. Soc. 124 (7), 1438–1442. doi:10.1021/ja016830+
McCammon, J. A., and Harvey, S. C. (1988). Dynamics of Proteins and Nucleic Acids. Cambridge: Cambridge University Press.
Murphy, M. P., and LeVine, H. (2010). Alzheimer's Disease and the Amyloid-Beta Peptide. J. Alzheimers Dis. 19 (1), 311–323. doi:10.3233/JAD-2010-1221
Mushtaq, G., Greig, N. H., Khan, J. A., and Kamal, M. A. (2014). Status of Acetylcholinesterase and Butyrylcholinesterase in Alzheimer's Disease and Type 2 Diabetes Mellitus. CNS Neurol. Disord. Drug Targets 13 (8), 1432–1439. doi:10.2174/1871527313666141023141545
Nordberg, A., Ballard, C., Bullock, R., Darreh-Shori, T., and Somogyi, M. (2013). A Review of Butyrylcholinesterase as a Therapeutic Target in the Treatment of Alzheimer's Disease. Prim. Care Companion CNS Disord. 15 (2), 12r01412. doi:10.4088/PCC.12r01412
Oliveros, J. C. (2007-2015). Venny. An Interactive Tool for Comparing Lists with Venn’s Diagrams. Available at https://bioinfogp.cnb.csic.es/tools/venny/index.html.
Ouchi, Y., Yoshikawa, E., Futatsubashi, M., Yagi, S., Ueki, T., and Nakamura, K. (2009). Altered Brain Serotonin Transporter and Associated Glucose Metabolism in Alzheimer Disease. J. Nucl. Med. 50 (8), 1260–1266. doi:10.2967/jnumed.109.063008
Pan, X., Kaminga, A. C., Wen, S. W., Wu, X., Acheampong, K., and Liu, A. (2019). Dopamine and Dopamine Receptors in Alzheimer's Disease: A Systematic Review and Network Meta-Analysis. Front. Aging Neurosci. 11, 175. doi:10.3389/fnagi.2019.00175
Patwardhan, B. (2014). Editorial: The New Pharmacognosy. Cchts 17 (2), 97. doi:10.2174/138620731702140119160627
Pohanka, M. (2014). Inhibitors of Acetylcholinesterase and Butyrylcholinesterase Meet Immunity. Int. J. Mol. Sci. 15 (6), 9809–9825. doi:10.3390/ijms15069809
[Internet] PubChem (2004). Bethesda (MD): National Library of Medicine (US), National Center for Biotechnology Information. PubChem Compound Summary for CID 854026, Huperzine A. Available at: https://pubchem.ncbi.nlm.nih.gov/compound/854026 (Accessed July 5, 2021).
Rauch, S. M., Huen, K., Miller, M. C., Chaudry, H., Lau, M., Sanes, J. R., et al. (2011). Changes in Brain β-amyloid Deposition and Aquaporin 4 Levels in Response to Altered Agrin Expression in Mice. J. Neuropathol. Exp. Neurol. 70 (12), 1124–1137. doi:10.1097/NEN.0b013e31823b0b12
Russ, T. C., and Morling, J. R. (2012). Cholinesterase Inhibitors for Mild Cognitive Impairment. Cochrane Database Syst. Rev. 2012 (9), CD009132. doi:10.1002/14651858.CD009132.pub2
Sousa da Silva, A. W., and Vranken, W. F. (2012). ACPYPE - AnteChamber PYthon Parser interfacE. BMC Res. Notes 5, 367. doi:10.1186/1756-0500-5-367
Still, W. C., Tempczyk, A., Hawley, R. C., and Hendrickson, T. (1990). Semianalytical Treatment of Solvation for Molecular Mechanics and Dynamics. J. Am. Chem. Soc. 112 (16), 6127–6129. doi:10.1021/ja00172a038
Szklarczyk, D., Gable, A. L., Lyon, D., Junge, A., Wyder, S., Huerta-Cepas, J., et al. (2019). STRING V11: Protein-Protein Association Networks with Increased Coverage, Supporting Functional Discovery in Genome-wide Experimental Datasets. Nucleic Acids Res. 47 (D1), D607–D613. doi:10.1093/nar/gky1131
Trott, O., and Olson, A. J. (2010). AutoDock Vina: Improving the Speed and Accuracy of Docking with a New Scoring Function, Efficient Optimization, and Multithreading. J. Comput. Chem. 31 (2), 455–461. doi:10.1002/jcc.21334
Van Aalten, D. M., Findlay, J. B., Amadei, A., and Berendsen, H. J. (1995). Essential Dynamics of the Cellular Retinol-Binding Protein-Eevidence for Ligand-Induced Conformational Changes. Protein Eng. 8 (11), 1129–1135. doi:10.1093/protein/8.11.1129
Van Der Spoel, D., Lindahl, E., Hess, B., Groenhof, G., Mark, A. E., and Berendsen, H. J. (2005). GROMACS: Fast, Flexible, and Free. J. Comput. Chem. 26 (16), 1701–1718. doi:10.1002/jcc.20291
Vanquelef, E., Simon, S., Marquant, G., Garcia, E., Klimerak, G., Delepine, J. C., et al. (2011). R.E.D. Server: a Web Service for Deriving RESP and ESP Charges and Building Force Field Libraries for New Molecules and Molecular Fragments. Nucleic Acids Res. 39 (Web Server issue), W511–W517. doi:10.1093/nar/gkr288
Wang, J., Wolf, R. M., Caldwell, J. W., Kollman, P. A., and Case, D. A. (2004). Development and Testing of a General Amber Force Field. J. Comput. Chem. 25 (9), 1157–1174. doi:10.1002/jcc.20035
Wang, R., and Tang, X. C. (2005). Neuroprotective Effects of Huperzine A. A Natural Cholinesterase Inhibitor for the Treatment of Alzheimer's Disease. Neurosignals 14 (1-2), 71–82. doi:10.1159/000085387
Whiley, L., Sen, A., Heaton, J., Proitsi, P., García-Gómez, D., Leung, R., et al. (2014). Evidence of Altered Phosphatidylcholine Metabolism in Alzheimer's Disease. Neurobiol. Aging 35 (2), 271–278. doi:10.1016/j.neurobiolaging.2013.08.001
Keywords: Alzheimer’s disease, huperzine A, system biology, donepezil, ligand–receptor interactions
Citation: Khanal P, Zargari F, Far BF, Kumar D, R M, Mahdi YK, Jubair NK, Saraf SK, Bansal P, Singh R, Selvaraja M and Dey YN (2021) Integration of System Biology Tools to Investigate Huperzine A as an Anti-Alzheimer Agent. Front. Pharmacol. 12:785964. doi: 10.3389/fphar.2021.785964
Received: 29 September 2021; Accepted: 05 November 2021;
Published: 13 December 2021.
Edited by:
Vijay Kumar, Leiden University Medical Center, NetherlandsReviewed by:
Budheswar Dehury, Regional Medical Research Center (ICMR), IndiaStylianos Chatzichronis, National and Kapodistrian University of Athens, Greece
Copyright © 2021 Khanal, Zargari, Far, Kumar, R, Mahdi, Jubair, Saraf, Bansal, Singh, Selvaraja and Dey. This is an open-access article distributed under the terms of the Creative Commons Attribution License (CC BY). The use, distribution or reproduction in other forums is permitted, provided the original author(s) and the copyright owner(s) are credited and that the original publication in this journal is cited, in accordance with accepted academic practice. No use, distribution or reproduction is permitted which does not comply with these terms.
*Correspondence: Malarvili Selvaraja, malarvili@ucsiuniversity.edu.my; Yadu Nandan Dey, yadunandan132@gmail.com
†These authors have contributed equally to this work and share first authorship
‡ORCID: Pukar Khanal, https://orcid.org/0000-0002-8187-2120; Farshid Zargari, https://orcid.org/0000-0002-0287-0013; Yadu Nandan Dey, https://orcid.org/0000-0003-4016-4379