- 1Yunnan Key Laboratory of Southern Medicine Utilization, College of Pharmaceutical Science, Yunnan University of Chinese Medicine, Kunming, China
- 2Chenggong Hospital of Kunming Yan’an Hospital, Kunming, China
Objectives: Polygonatum kingianum is a medicinal herb used in various traditional Chinese medicine formulations. The polysaccharide fraction of P. kingianum can reduce insulin resistance and restore the gut microbiota in a rat model of aberrant lipid metabolism by down regulating miR-122. The aim of this study was to further elucidate the effect of P. kingianum on lipid metabolism, and the roles of specific miRNAs and the gut microbiota.
Key findings: P. kingianum administration significantly altered the abundance of 29 gut microbes and 27 differentially expressed miRNAs (DEMs). Several aberrantly expressed miRNAs closely related to lipid metabolism were identified, of which some were associated with specific gut microbiota. MiR-484 in particular was identified as the core factor involved in the therapeutic effects of P. kingianum. We hypothesize that the miR-484-Bacteroides/Roseburia axis acts as an important bridge hub that connects the entire miRNA-gut microbiota network. In addition, we observed that Parabacteroides and Bacillus correlated significantly with several miRNAs, including miR-484, miR-122-5p, miR-184 and miR-378b.
Summary: P. kingianum alleviates lipid metabolism disorder by targeting the network of key miRNAs and the gut microbiota.
Introduction
Lipid metabolism homeostasis requires constant metabolic adjustment, which is partly achieved by regulating the expression of key genes. MicroRNAs (miRNAs) are small non-coding RNAs that regulate gene expression levels by silencing target mRNAs (Hu et al., 2017). and more and more studies suggest that involvement of miRNAs in lipid metabolism and related disorders (Ge et al., 2014; Blasco-Baque et al., 2017; Sliwinska et al., 2017; Sud et al., 2017; Yao et al., 2018).
Polygonatum kingianum Coll. et Hemsl (P. kingianum) is one of the constituent species in Polygonati Rhizoma, a traditional Chinese medicine formulation. It has various pharmacological activities, such as immuno-stimulatory, anti-aging, blood glucose and lipid regulatory properties (Commission of Chinese Pharmacopoeia, 2020). It has attracted considerable interest in recent years as an effective adjuvant for maintaining the steady state of glucolipid metabolism (Lu et al., 2016; Yan et al., 2017). In a previous study, we found that P. kingianum alleviated HFD-fed (HFD)-induced non-alcoholic fatty liver disease (NAFLD) by significantly promoting mitochondrial functions (Yang et al., 2018). It can also alleviate HFD-induced dyslipidemia by regulating many endogenous metabolites in the serum, urine and liver (Yang et al., 2019). There is evidence indicating that fecal miRNAs shape the gut microbiota, and are a potential therapeutic target against metabolic disorders involving dysregulation of the intestinal microbiome (Liu et al., 2016). However, whether how miRNA in liver tissue could affect gut microbiota are still lack of understanding. This reseach focus on imagining whether host miRNAs in liver tissue can also affect gut microbiota. The aim of this study was to explore the miRNAs and the gut microbiota related mechanisms that involved in the ameliorative effect of P. kingianum on lipid metabolism disorder.
Materials and Methods
Chemicals, Reagents and Materials
Chow diet (62, 26 and 12% calories obtained from carbohydrates, proteins and fats, respectively) was obtained from Suzhou Shuangshi Experimental Animal Feed Technology Co. Ltd. (Suzhou, China). Cholesterol, refined lard and eggs were supplied by Beijing Boao Extension Co. Ltd. (Beijing, China), Sichuan Green Island Co. Ltd. (Chengdu, China) and Wal-Mart Supermarket (Kunming, China) respectively. Simvastatin was purchased from Hangzhou Merck East Pharmaceutical Co. Ltd. (Hangzhou, China), and TRIzol® Reagent from Ambion (Carlsbad, CA, United States). The mircute enhanced miRNA cDNA first strand synthesis kit and the miRNA fluorescence quantitative detection kit were provided by Tiangen Biochemical Technology Co. Ltd. (Beijing, China). D4015 fecal DNA kit was provided by Feiyang Bioengineering Co. Ltd. (Guangzhou, China). Dihydrate oxalic acid was supplied by Sichuan Xiqiao Chemical Co. Ltd. (Sichuan, China) and sodium azide by Amresco (United States). High-purity deionized water was purified using a Milli-Q system (Millipore, Bedford, MA, United States). All other reagents were of analytical grade or higher P. kingianum rhizomes were purchased from Wenshan Shengnong Trueborn Medicinal Materials Cultivation Cooperation Society (Wenshan Country, Yunnan Province, China) on April 07, 2016. The samples were authenticated by Professor Jie Yu, and a specimen (No. 8426) was deposited in the Key Laboratory of Preventing Metabolic Diseases of Traditional Chinese Medicine, Yunnan University of Chinese Medicine (Kunming, China).
Processing of P. kingianum Rhizome
The fresh P. kingianum rhizomes were processed fellowed our previous studies as well (Gu et al., 2020).
Preparation of Crude Polysaccharides
The method of preparation of crude polysaccharides fellowed our previous studies as well (Gu et al., 2020).
Preparation of Total Polysaccharide and High Molecular Weight Polysaccharide Fraction
The method of preparation oftotal polysaccharide (PS) and high molecular weight polysaccharide fraction (PSF) fellowed our previous studies as well (Gu et al., 2020).
Preparation of Water Extract
The dried P. kingianum rhizomes were pulverized, weighed and extracted with 10-, 6-, 4-times volumes of distilled water for 1 h, 40 and 30 min respectively. All water extracts were combined, concentrated under reduced pressure (50 times), frozen to −80°C and then lyophilized (SIM International Group Co. Ltd., Newark, DE, United States). The lyophilized powder was ground, desiccated and stored for later use.
Quantitative Analysis of PS, PFS, PWE
The method of quantitative analysis of PS, PFS fellowed our previous studies as well (Gu et al., 2020). The tested sample of total saponins in PWE was prepared and tested according the previous method (Lu et al., 2016). PWE lyophilized powder 2.50 g was accurately transferred to 10 ml dissolved, then the tested sample of total polysaccharide in PWE was prepared and tested according the method.
Induction of Lipid Metabolism Disorder and Treatment Regimen
Feeding and modeling of HFD-rats fellowed our previous studies as well (Gu et al., 2020). In order to study the therapeutic effect of P. kingianum on HFD-rats, we refer to the dosage in the literature (Zhao et al., 2015). The middle dose of PS, PSF and PWE materials for rats was set for 240 mg/ kg, the high and low doses are converted correspondingly by 2 times and 0.5 times. Simvastatin (SIM) (1.8 mg/ kg) was administered as a positive control. The rats were randomized into the following groups (n = 10 each) and treated with the suitable drug/placebo for 14 weeks (excluding the CON group) via the intragastric route (Table 1).
At the end of the 14 weeks, rats were anesthetized intraperitoneally using 1% pentobarbital sodium and euthanized by cervical dislocation. Then, the liver were immediately homogenized in TRIzol (Invitrogen, Carlsbad, CA, United States), snap frozen in liquid nitrogen and stored at−80°C. All reasonable efforts were made to minimize animal suffering.
MiRNA Sequencing
Liver RNA Isolation
Three liver tissue samples from each group (upper left anterior lobe of liver) were used for total RNA isolation according to the manufacturer’s protocol. The integrity and purity of the isolated RNA were determined by 1% agarose gel electrophoresis and a NanoPhotometer® (IMPLEN, CA, United States) respectively. RNA concentration was measured using Qubit® RNA Assay Kit in the Qubit® 2.0 Flurometer (Life Technologies, CA, United States). The quality of the samples was further assessed using the RNA Nano 6000 Assay Kit of the Agilent Bioanalyzer 2,100 system (Agilent Technologies, CA, United States) to ensure suitability for high-throughput sequencing.
Library Preparation
RNA sequencing libraries were generated with 3 μg total RNA per sample using NEBNext ® Multiplex Small RNA Library Prep Set for Illumina® (NEB, USA.) according to the manufacturer’s instructions, and index codes were added to attribute sequences to each sample. The NEB 3′ SR adaptor was directly ligated to the 3′ end of miRNA, siRNA, and piRNA, and the free adapter sequences were hybridized to SR RT primer in order to prevent formation of adaptor-dimers. The 5′ SR adaptor was then ligated to the 5′ends of the transcripts using T4 RNA Ligase 1. First strand cDNA was synthesized using M-MuLV Reverse Transcriptase (RNase H), and amplified using LongAmp Taq 2X Master Mix, SR Primer for Illumina and index (X) primer. The PCR products were purified on a 8% polyacrylamide gel (100 V, 80 min), and the 140–160 bp long fragments (small noncoding RNA plus the 3′ and 5′ adaptors) were recovered in 8 μL elution buffer. Library quality was assessed on the Agilent Bioanalyzer 2,100 system using DNA High Sensitivity Chips.
Clustering and Sequencing
High-throughput sequencing was performed by Beijing Nuohe Zhiyuan Technology Co. Ltd. The index-coded samples were clustered on a cBot Cluster Generation System using TruSeq SR Cluster Kit v3-cBot-HS (Illumia) according to the manufacturer’s instructions. The library was sequenced on an Illumina Hiseq 2,500 platform and 50bp single-end reads were generated.
MiRNA Sequencing Data Analysis
Quality Control
Raw reads in the fastq format were processed through custom perl and python scripts, and the clean reads were obtained by removing low quality reads containing poly-N, 5′ adapter contaminants, lacking 3′adapter or the insert tag and containing poly A/T/G/C. The Q20, Q30, and GC-content of the raw reads were also calculated. Clean reads of a certain range of length were selected for downstream analyses. The small RNA tags were mapped to the reference sequence using Bowtie without mismatch to analyze their expression levels and distribution in the former.
Known miRNA Alignment
The mapped small RNA tags were used to screen for known miRNAs using mirdeep2, a modified version of miRBase20.0, and srna-tools-cli was used to draw the secondary structures. Custom scripts were used to obtain the miRNA counts and base bias on the first position of identified miRNAs of certain lengths, and on each position of all identified miRNAs. The tags originating from protein-coding genes, repeat sequences, rRNA, tRNA, snRNA, and snoRNA were removed by mapping to RepeatMasker, Rfam database and species specific data.
Novel miRNA Prediction
Novel miRNAs were predicted using the miREvo and mirdeep2 programs on the basis of secondary structure, Dicer cleavage site and the minimum free energy of the small RNA tags unannotated in the former steps. Custom scripts were used to analyze these identified miRNAs as described in the previous section.
MiRNA Editing, Family Analysis and Target Gene Prediction
The miRNAs with base edits in the seed region were detected by aligning all the sRNA tags to mature miRNAs with the allowance of one mismatch. The miRNA families were then identified from known miRNAs of other species using miFam.dat (http://www.Mirbase.org/ftp.shtml), and Rfam families from novel miRNA precursors using Rfam (http://rfam.sanger.ac.uk/search/). Target genes of the miRNAs were predicted by psRobot_tar in psRobot using the RNAhybrid, PITA and miRanda algorithms.
Identification of Differentially Expressed miRNAs
The miRNA expression levels were estimated in terms of TPM (transcript per million) using established criteria, and normalized as mapped read count/total reads*1000000. The significant DEMs between two conditions/groups were screened using the DESeq R package (1.8.3), with corrected (Benjamini and Hochberg method) P-value < 0.05 and fold change (log2, FC) > 1 as the thresholds.
GO and KEGG Enrichment Analysis
Gene Ontology (GO) enrichment analysis of the target gene candidates of DEMs was performed using GOseq-based Wallenius non-central hyper-geometric distribution, which can adjust for gene length bias. KEGG database was used to screen for the significantly enriched pathways among the target genes (http://www.genome.jp/kegg/), and the statistical significance was tested using KOBAS.
Quantitative RT-PCR
The DEMs identified above were validated by qRT-PCR. Total RNA was extracted from three randomly selected liver samples per group (36 in total) using Trizol reagent, and reversed transcribed into cDNA using the Tiangen miRcute miRNA cDNA First-Strand Synthesis Kit (Beijing, China). RT-PCR was performed with 2 μL cDNA template and specific primers (Supplementary Table S1) using the miR-cute miRNA Fluorescence Quantification Kit in the ABI PRISM 7300 Real-Time PCR System (Applied Biosystems, Foster City, CA, United States). The reaction parameters were as follows: initial denaturation at 50°C for 2 min, followed by 40 cycles of 95°C for 10 min and one cycle of 95°C–60°C. Two biological replicates were tested per sample, and each reaction was performed in triplicate. U6 was used as the internal standard. Relative expression levels of miRNAs were measured based on threshold cycle values (ct) as 2-ΔΔct.
Sequencing of Gut Microbiota
The gut microbiota was sequenced as described previously (Gu et al., 2020). Briefly, DNA was extracted from the frozen stool samples using the Fecal DNA Isolation Kit (United States Omega Bio-Tek Co. Ltd), and the 16s rRNA V4 region was amplified using the 515F-806R primer set. Sequencing libraries were generated using TruSeq DNA PCR-Free Sample Preparation Kit (Illumina, San Diego, CA, United States) according to the manufacturer’s instructions and the index codes were added. The library quality was assessed on a Qubit@ 2.0 Fluorimeter (Thermo Scientific Waltham, Massachusetts, United States) and Agilent Bioanalyzer 2,100 system, and sequenced on an IlluminaHiSeq 2,500 platform by Beijing Nuohe Zhiyuan Bioinformatics Co. Ltd. The 250 bp paired-end reads were clustered into OTUs (Operational Taxonomic Units) with 97% consistency by validating each sample. The OTUs abundance was converted to base log 10 to reduce the distance between samples due to the high abundance of OTUs in some.
Correlation and Network Analysis
To further understand the relationship between miRNA and gut microbiota in rats with lipid metabolism, the Pearson correlation analysis was performed to determine the correlation between DEMs and gut microbiota, and between DEMs and key OTUs, using p < 0.05 and R value >0.5 as the thresholds. The Cytoscape software was used to construct and visualize the biological networks between the above pairs.
Statistical Analysis
The data were analyzed by SPSS 16.0 statistical software (IBM, Armonk, NY, United States), and expressed as mean ± SD. p < 0.05 was considered statistically significant. Pearson correlation coefficient was used to determine the strength of correlation between variables as follows: 0.8–1.0–very strong correlation; 0.6–0.8–strong correlation; 0.4–0.6–moderately relevant; 0.2–0.4–weak correlation; 0.0–0.2–very weakly correlated or uncorrelated.
Results
Quantitative Analysis Results of PS, PFS, PWE
The specific results of quantitative analysis about PS and PSF as reported in our previous studies as well (Gu et al., 2020). The content of total saponins and polysaccharide in PWE were 2.19 and 1.71% respectively.
Novel miRNAs Were Identified in the Liver Tissues
The miRNAs dysregulated by HFD feeding were identified in the liver samples using high-throughput sequencing, and as shown in Supplementary Figure S2, the error rate of all but the first five bases was lower than 0.5. In addition, the Q30 score for each sample was not less than 94.02% (Supplementary Table S3), the total number of bases G and C represented the quality of sequencing data. Total G and C percentages were higher than 48.17%, indicated that the sequencing quality was satisfied. After removing low-quality reads, the resulting clean reads accounted for more than 93.58% of all sequences (Supplementary Table S4). The length of the miRNA sequences ranged from 20 to 24 nucleotides, accounting for more than 80% of the pure sequence (Supplementary Figure S3). The 22 nucleotides-long miRNAs were predominant, accounting for about 30% of all sequences, followed by those with 23 and 21 nucleotides. Bowtie analysis indicated that at least 87.07% of the sequences were aligned with the reference genome (Supplementary Table S5). Furthermore, the number of known miRNAs in the sequencing results was relatively high, the proportion of rRNA/tRNA/snRNA/snoRNA was relatively low, as were the number and proportion of positive and negative chains in the exons/introns (Supplementary Table S6). Taken together, the sequences obtained were enriched in miRNAs.
The above-mentioned reads that mapped to the reference sequence were compared with a specified range of sequences in miRBase, and 613 known mature miRNAs and 438 precursors were identified that matched the secondary structure of partially known miRNAs (Supplementary Figure S4). Furthermore, U was the most common first base of miRNAs with lengths of 18–25 nucleotides followed by A, while G and C were less frequent (Supplementary Figure S5). The base distribution of each sample is shown in Supplementary Figure S6; the most and least common first bases were U and G respectively. In addition, 137 mature miRNAs and 142 hairpins matched the secondary structure of partially predicted novel miRNAs (Supplementary Figure S7), which showed similar base preference as the known miRNAs (Supplementary Figures S8, 9).
Differential miRNA Screening
A total of 135 DEMs–including 70 upregulated (UP) and 65 downregulated (DOWN) miRNAs–were identified in the untreated HFD-fed model versus all other groups (Table 2; Supplementary Figure S10). The expression levels of the significant DEMs are summarized in Supplementary Table S7.
Hierarchical cluster analysis of the 135 DEMs classified the samples into two categories. The PSF.L, PSF.M, PSF.H, PS.L, PS.M, and PWE.H samples were clustered in one category, indicating the effect of PSF and PS on miRNA levels was largely independent of dosage. Furthermore, the presence of PWE.M and SIM in one cluster indicated that medium-dose PWE had similar effects as simvastatin (Figure 1A). Twenty-seven DEMs with more than two replicates were further screened and subjected to cluster analysis. As shown in Figure 1B, CON, MOD, PSF.M, and PSF.H groups were clustered into one category, and the remaining including PS.H and PWE.L, PWE.M and SIM into the second category, which indicated similar regulatory effects on these DEMs. To verify the accuracy of DEMs identified by high-throughput sequencing, 11 DEMs with two replicates and log2 FC > 1 were further screened by comparing the TEST group (Supplementary Table S8) with the MOD group. As shown in Figure 2, the qRT-PCR results of all DEMs were consistent with that of high-throughput sequencing, indicating reliability of the latter.
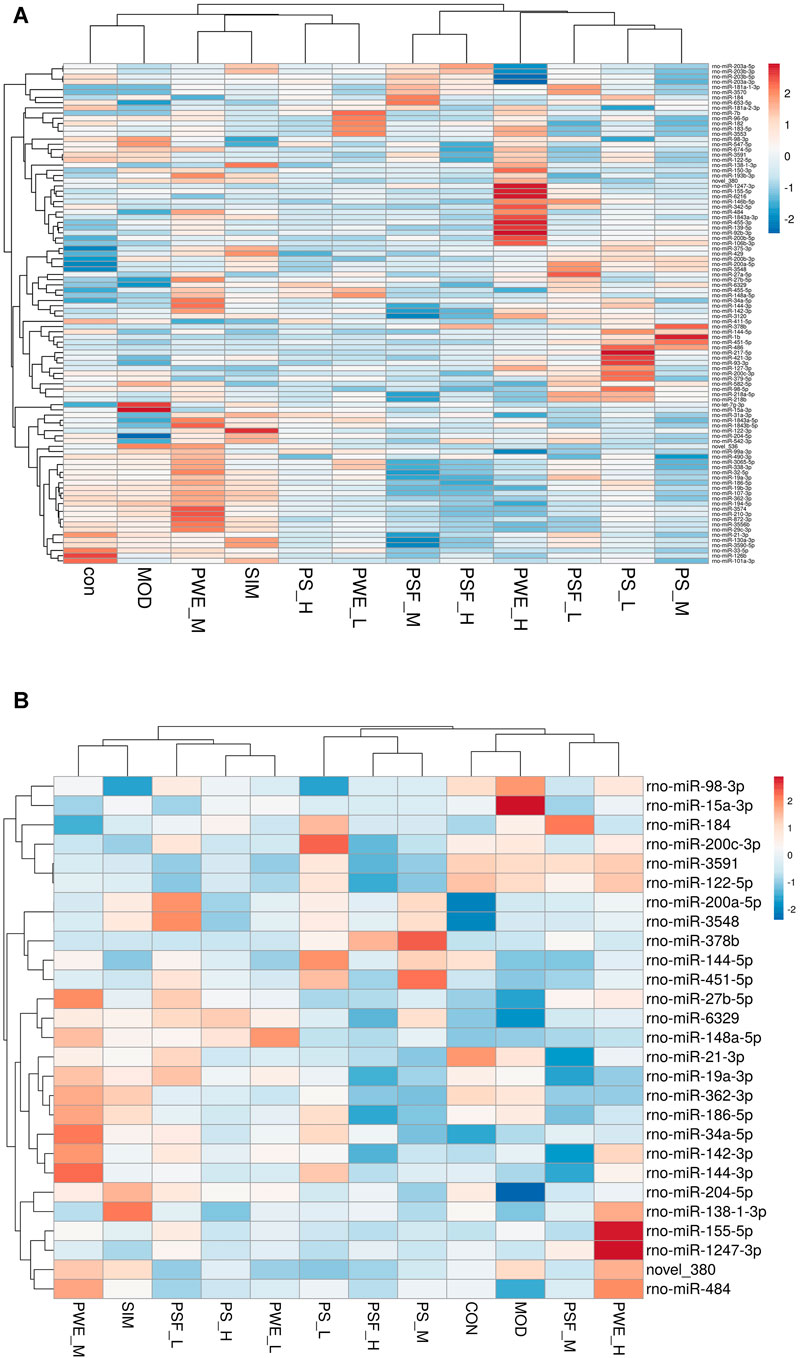
FIGURE 1. (A). Clustering maps of 135 DEMs (B). Clustering maps of 27 DEMs. The abscissa represents the sample and ordinate DEM. Red and blue blocks respectively indicate the high and low expressing miRNAs.
MiRNA Target Gene Prediction
A total of 16,038 targets were predicted for the 135 DEMs using RNAhybrid, PITA, and miRanda (Supplementary Table S9). As shown in Supplementary Table S10, the biological functions of the target genes were diverse, and most of them participate in metabolic pathways of glucose, lipids and nucleotides (p < 0.05). KEGG analysis further showed a significant enrichment in type 2 diabetes, insulin secretion and glycerolipid metabolism pathways (Supplementary Figures S11A-C).
Regulatory Effect of P. kingianum on Gut Microbiota in Rats With Lipid Metabolism
P. kingianum administration significantly altered the abundance of 29 gut microbes (Supplementary Table S11), which differed in their relative composition across the groups. Similar results were obtained in a previous study as well (Gu et al., 2020). The most significant changes were seen in Roseburia, Bacteroides and Lactobacillus. P. kingianum extracts increased the relative abundance of Roseburia compared to that in the untreated HFD-fed rats. Furthermore, except for PSF.L, the other extracts/dosages increased the abundance of Bacteroides, and all extracts significantly reduced the relative abundance of Lactobacillus. The three genera are closely related to lipid metabolism (Chen et al., 2015; Dalziel et al., 2017; Huang et al., 2019). The 50 most significantly altered OTUs were screened to further verify the relationship between miRNAs and gut microbiota after P. kingianum administration.
Correlation Between DEMs and Gut Microbiota
We further analyzed the correlation between the 27 DEMs and the 29 most significantly altered gut microbes, and found that some DEMs were correlated with specific genera in the P. kingianum-treated animals (Figure 4). Network analysis further showed that miR-484, miR-204-5p and miR-19a-3p were most significantly correlated with the gut microbiota (Figure 3). As shown in Figure 3; Figure 4 miR-484 was positively correlated with Bacteroides, Roseburia, Blautia, Prevotella and Coprococcus, and negatively with Ruminococcus and Christensenellaceae. In fact, miR-484 and Bacteroides were the hub that connected the entire network. Furthermore, miR-204-5p showed positive correlation with Helicobacter, and negative correlation with Lactobacillus and Psychrobacter. MiR-19a-3p was negatively correlated with Bacteroides, Bacillus and Thermobacillus. Finally, miR-6329, miR-148a-5p and miR-27b-5p were positively correlated with Oscillibacter and negatively correlated with Lactobacillus. Taken together, P. kingianum affected the interactions between DEMs and the gut microbiota in rats with lipid metabolism disorder.
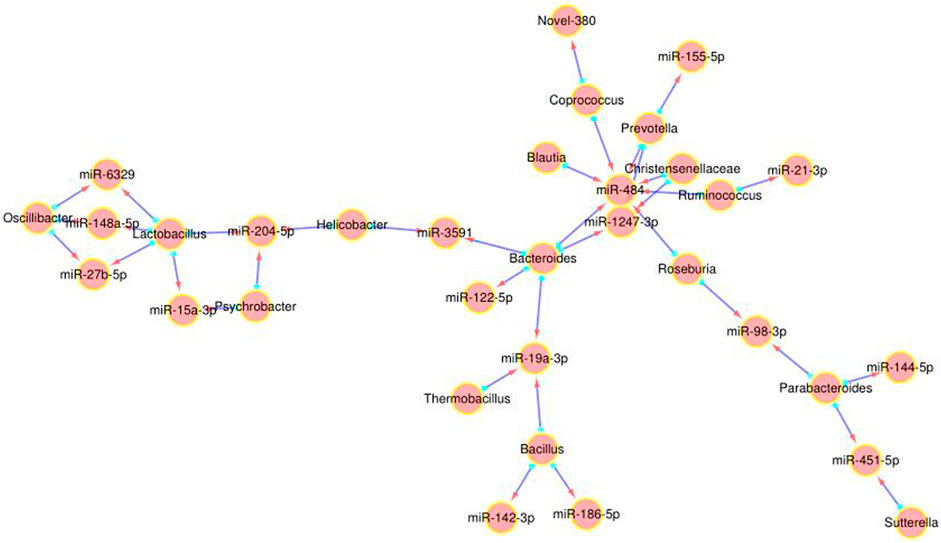
FIGURE 3. Network analysis of DE miRNA and gut microbiota interactions. Red arrow indicates the miRNA, and the blue origin the intestinal microbes.
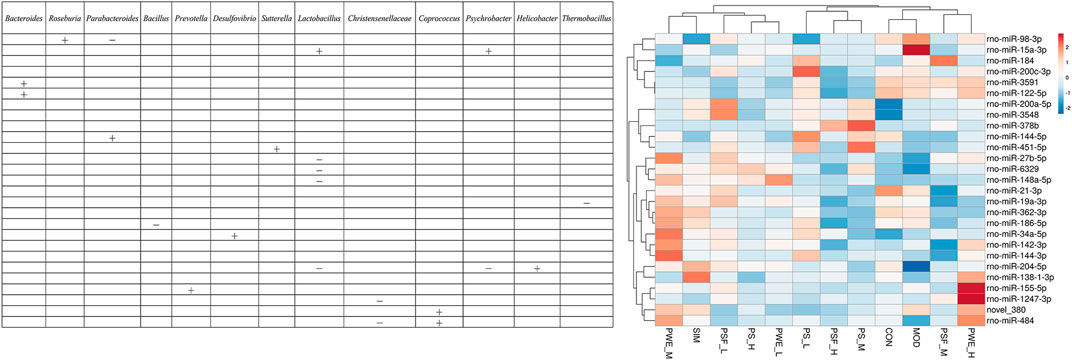
FIGURE 4. Correlation between significantly altered miRNAs and significantly altered gut microbiota “ + ” Stands for positive correlation, “ − ” stands for negative correlation.
As shown in Figure 5, 22 DEMs were significantly correlated with 44 key OTUs, of which miR-484, miR-122-5p, miR-184 and miR-378 were closely related to lipid metabolism. MiR-484 was positively correlated with OTU 243 (Parabacteroides), and negatively with OTU 6 (Bacteroidetes), OTU 137 (Bacteroidetes), OTU 238 (Bacteroidetes), OTU 261 (Bacteroidetes), OTU 1324 (Bacteroidetes), OTU 21 (Firmicutes), OTU 35 (Firmicutes), OTU 248 (Firmicutes) and OTU 2400 (Firmicutes). MiR-122-5p were negatively correlated with OTU 39 (Bacteroidetes) and OTU 88 (Firmicutes), and positively with OTU 172 (Bacteroides), OTU 68 (Bacteroidetes), OTU 119 (Firmicutes), OTU 229 (Firmicutes) and OTU 729 (Firmicutes). In addition, miR-184 were positively correlated with OTU 77 (Parabacteroides), OTU 137 (Bacteroidetes), OTU 261 (Bacteroidetes) and OTU 5 (Lactobacillus). Finally, miR-378b were negatively correlated with OTU 83 (Alloprevotella) and OTU 670 (Rikenella), and positively with OTU 475 (Parapedobacter).
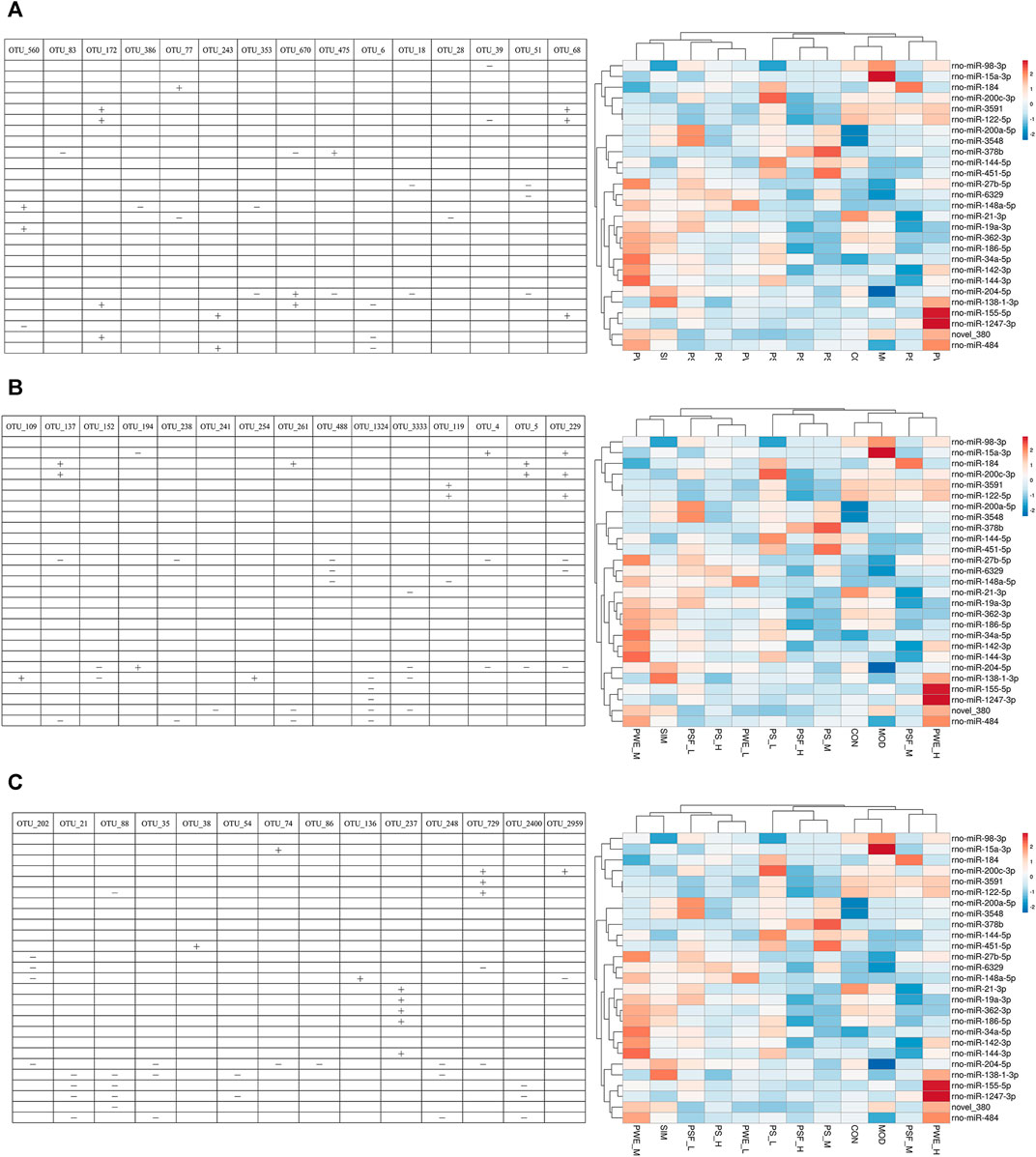
FIGURE 5. Correlation between significantly altered miRNAs and key OTUs “ + ” Stands for positive correlation, “ − ” stands for negative correlation. (A) (OTU_560—OTU_68); (B) (OTU_109—OTU_229); (C) (OTU_202—OTU_2959).
Discussion
Effect of P. kingianum on miRNAs and its Target Genes After HFD-Fed
High-throughput sequencing can identify a large number of genes across the entire genome in a relatively short time, and is therefore an effective tool for discovering and identifying novel miRNAs (Kebschull et al., 2017; Li et al., 2017) It obviates the over-reliance of bioinformatics analysis on species-specific genome-wide information, as well as the interference of the overexpressed cDNA clones in miRNA discovery, and allows identification of novel and differentially expressed miRNAs at different developmental stages and physiological conditions (Wold and Myers, 2008) It is especially suitable for detecting miRNAs alternations following drug administration (Wei et al., 2015; Wang et al., 2019; Zheng et al., 2019). The different extracts of P. kingianum significantly altered the liver miRNA profiles of the HFD-fed rats. While the PSF.M significantly increased the relative expression of miR-1247-3p and reduced that of miR-21-3p, PSF.L markedly decreased the expression of miR-122-5p. In addition, PS.M upregulated miR-378b, and PWE.M respectively increased and decreased the levels of miR-484 and miR-184. Some of these miRNAs had been reported to play key regulatory roles in lipid metabolism.
MiR-122 was the first lipid metabolism-related miRNA to be discovered, and is specifically expressed in the liver, accounting for approximately 70% of all liver miRNAs (Filipowicz and Grosshans, 2011). It plays an important role in maintaining normal lipid metabolism (Jopling, 2012), and regulates cholesterol biosynthesis (Krutzfeldt et al., 2005)., fatty acid synthesis and β-oxidation (Elhanati et al., 2016), resulting in lower plasma and liver cholesterol levels and a decrease in fatty acid synthesis (Esau et al., 2006). Consistent with this, PSF significantly downregulated miRNA-122 in the liver, leading to decreased expression of genes involved in downstream lipid synthesis.
MiR-484 is downregulated in pancreatic beta cells in response to hyperglycemic conditions, suggesting that elevated glucose levels in insulin resistance (IR) may affect miR-484 levels in the peripheral blood as well (Tang et al., 2009). Furthermore, miR-484 targets the mitochondrial fission gene Fis1 (Wang et al., 2012), and since mitochondrial fission is increased during diabetes and contributes to circulating insulin levels, downregulation of miR-84 drives the pathogenesis of IR (Wang et al., 2012). PWE.H significantly upregulated miR-484, which is the likely mechanistic basis of its ameliorative effects on lipid metabolism.
Effect of P. kingianum on the miRNAs and Gut Microbiota Network
Studies show the extensive involvement of miRNAs maintaining intestinal homeostasis and the gut microbiota, a complex micro-ecological system that controls host energy metabolism, immune system and inflammatory responses. A previous study identified 16 differentially expressed miRNAs in the cecal tissues of sterile and SPF mice (Singh et al., 2012). Transplanting fecal bacteria from the SPF mice into sterile mice altered the expression levels of 9 miRNAs in the ileum and colon, which in turn affected the expression of at least 700 genes involved in intestinal barrier function and immune regulation (Dalmasso et al., 2011). Consistent with this, the lipid metabolism-related DEMs showed a significant correlation with the altered gut microbiota in the HFD-fed animals, and miR-484, miR-204-5p, and miR-19a-3p were most closely related to changes in the gut microbiota. Correlation analysis of DEMs and key OTUs, along with previous reports on lipid metabolism-related miRNAs, further identified miR-484, miR-122-5p, miR-184, and miR-378b, of which miR-484 was significantly correlated with several gut microbiota such as Bacteroides, Roseburia, Ruminococcus, Blautia, Prevotella, Christensenellaceae and Coprococcus. miR-484 was significantly decreased in the HFD-fed rats and restored by P. kingianum and simvastatin in this research.
Bacteroides is a core intestinal genus (Luis et al., 2018), and shows a lower abundance in the gut of obese individuals (Guo et al., 2008) that is restored following dietary restriction and weight loss. Consistent with this, the abundance of Bacteroides was significantly reduced in the HFD-fed animals, and increased after administering different P. kingianum extracts. In fact, previous studies have shown a significant regulatory effect of P. kingianum polysaccharides on the gut microbiota (Yang et al., 2018; Gu et al., 2020). We found that PS and PSF improved both diabetic symptoms and lipid metabolism. PS and PSF also modulated the gut microbiota composition, abundance and diversity of HFD rats, increased the relative abundance of short chain fatty acid (SCFA) producing bacteria and increased SCFA production, reduced intestinal permeability, relieved gastrointestinal inflammation, and improved lipid metabolism. The abundance of Roseburia was also significantly decreased after HFD feeding, and restored by P. kingianum and simvastatin. Likewise, Neyrinck et al. (2011) also detected a lower abundance of Roseburia in the gut microbiota of HFD-induced obese mice, which increased significantly after treatment.
Since miR-484 interacted with various gut microbiota, we surmised that it formed the “core” of the regulatory network targeted by P. kingianum during lipid metabolism disorder. We hypothesize that the miR-484-Bacteroides/Roseburia axis acts an important bridge hub that connects the entire miRNA-gut microbiota network. Further studies should focus on this network, along with miR-148a-5p, miR-27b-5p, miR-6329, miR-204-5p, and miR-19a-3p that interact with multiple intestinal microorganisms. In addition, we observed that Parabacteroides and Bacillus correlated significantly with several miRNAs, including miR-484, miR-122-5p, miR-184, and miR-378b. The “Parabacteroides-miRNAs” and “Bacillus-miRNAs” networks therefore also warrant further study.
Summary
In conclusion, P. kingianum extracts can alleviate lipid metabolism disorders by targeting the miRNA-gut microbiota network. The causality between miRNAs and gut microbiota, and their interaction play significant role in the preventive and therapeutic effects of P. kingianum on lipid metabolism disorders. MiR-484 in particular was identified as the core factor involved in the therapeutic effects of P. kingianum. We hypothesize that the miR-484-Bacteroides/Roseburia axis acts as the core factor involved in the therapeutic effects of P. kingianum.
Data Availability Statement
The data presented in the study are deposited in the National Center for Biotechnology Information repository, accession number PRJNA772872
Ethics Statement
The animal study was reviewed and approved by Approval from the Institutional Ethical Committee on Animal Care and Experimentations of Yunnan University of Chinese Medicine (R-0620160026) was obtained for this study.
Author Contributions
JD and JY wrote the manuscript. JD, LZ, XW, YW, FL, XY, and MY conducted the experiments. JY, WG, and XY provided technical support and helpful discussions. JY and WG designed the study. All authors have read and approved the manuscript.
Funding
This research was supported by the grants from the National Natural Science Foundation of China (Grants 81760733 (JY), 81960710 (JY), 81660684 (WG), 81660596 (XY)), the Application and Basis Research Project of Yunnan China) Grants 2018FF001-005 (JY), 202001AV070007 (JY), 2016FD050 (XY), 2019IB009 (JY), 2017FF117-013 (XY), 2017FF116-003 (XX), 2017FF117-015 (Hongli Y)), Academician Workstation in Yunnan Province (JY), the University Scientific and Technological Innovation Team of Prevention and Treatment of Metabolic Diseases by Chinese Medicine of Yunnan (JY), Kunming Key Laboratory for Metabolic Diseases Prevention and Treatment by Chinese Medicine (JY). Among them, 81960710 and 2018FF001-005 contributed to the overall research designand major financial support. Other fundings provide some biological samples, reagents, gut microbiota sequencing, and some experimental apparatus.
Conflict of Interest
The authors declare that the research was conducted in the absence of any commercial or financial relationships that could be construed as a potential conflict of interest.
Publisher’s Note
All claims expressed in this article are solely those of the authors and do not necessarily represent those of their affiliated organizations, or those of the publisher, the editors and the reviewers. Any product that may be evaluated in this article, or claim that may be made by its manufacturer, is not guaranteed or endorsed by the publisher.
Supplementary Material
The Supplementary Material for this article can be found online at: https://www.frontiersin.org/articles/10.3389/fphar.2021.740528/full#supplementary-material
References
Blasco-Baque, V., Coupé, B., Fabre, A., Handgraaf, S., Gourdy, P., Arnal, J. F., et al. (2017). Associations Between Hepatic miRNA Expression, Liver Triacylglycerols and Gut Microbiota During Metabolic Adaptation to High-Fat Diet in Mice. Diabetologia. 60 (4), 690–700. doi:10.1007/s00125-017-4209-3
Chen, D., Yang, Z., Chen, X., Huang, Y., Yin, B., Guo, F., et al. (2015). Effect of Lactobacillus Rhamnosus Hsryfm 1301 on the Gut Microbiota and Lipid Metabolism in Rats Fed a High-Fat Diet. J. Microbiol. Biotechnol. 25 (5), 687–695. doi:10.4014/jmb.1409.09085
Commission of Chinese Pharmacopoeia (2020). Pharmacopoeia of the People’s Republic of China. (Beijing: Chinese Medicine Science and Technology Publishing House) 1, 319.
Dalmasso, G., Nguyen, H. T., Yan, Y., Laroui, H., Charania, M. A., Ayyadurai, S., et al. (2011). Microbiota Modulate Host Gene Expression via microRNAs. PLoS One. 6 (4), e19293. doi:10.1371/journal.pone.0019293
Dalziel, J. E., Fraser, K., Young, W., Mckenzie, C. M., Bassett, S. A., and Roy, N. C. (2017). Gastroparesis and Lipid Metabolism-Associated Dysbiosis in Wistar-Kyoto Rats. Am. J. Physiol. Gastrointest. Liver Physiol. 313 (1), G62–G72. doi:10.1152/ajpgi.00008.2017
Elhanati, S., Ben-Hamo, R., Kanfi, Y., Varvak, A., Glazz, R., Lerrer, B., et al. (2016). Reciprocal Regulation Between SIRT6 and miR-122 Controls Liver Metabolism and Predicts Hepatocarcinoma Prognosis. Cell Rep. 14 (2), 234–242. doi:10.1016/j.celrep.2015.12.023
Esau, C., Davis, S., Murray, S. F., Yu, X. X., Pandey, S. K., Pear, M., et al. (2006). miR-122 Regulation of Lipid Metabolism Revealed by In Vivo Antisense Targeting. Cell Metab. 3 (2), 87–98. doi:10.1016/j.cmet.2006.01.005
Filipowicz, W., and Grosshans, H. (2011). The Liver-Specific microRNA miR-122: Biology and Therapeutic Potential. Prog. Drug Res. 67, 221–238. doi:10.1007/978-3-7643-8989-5_11
Ge, Q., Brichard, S., Yi, X., and Li, Q. (2014). microRNAs as a New Mechanism Regulating Adipose Tissue inflammation in Obesity and as a Novel Therapeutic Strategy in the Metabolic Syndrome. J. Immunol. Res. 2014, 987285. doi:10.1155/2014/987285
Gu, W., Wang, Y., Zeng, L., Dong, J., Bi, Q., Yang, X., et al. (2020). Polysaccharides From Polygonatum Kingianum improve Glucose and Lipid Metabolism in Rats Fed a High Fat Diet. Biomed. Pharmacother. 125, 109910. doi:10.1016/j.biopha.2020.109910
Guo, X., Xia, X., Tang, R., Zhou, J., Zhao, H., and Wang, K. (2008). Development of a Real-Time PCR Method for Firmicutes and Bacteroidetes in Faeces and its Application to Quantify intestinal Population of Obese and Lean Pigs. Lett. Appl. Microbiol. 47 (5), 367–373. doi:10.1111/j.1472-765X.2008.02408.x
Hu, Y., Ehli, E. A., and Boomsma, D. I. (2017). MicroRNAs as Biomarkers for Psychiatric Disorders With a Focus on Autism Spectrum Disorder: Current Progress in Genetic Association Studies, Expression Profiling, and Translational Research. Autism Res. 10 (7), 1184–1203. doi:10.1002/aur.1789
Huang, S., Pang, D., Li, X., You, L., Zhao, Z., Cheung, P. C., et al. (2019). A Sulfated Polysaccharide From Gracilaria Lemaneiformis Regulates Cholesterol and Bile Acid Metabolism in High-Fat Diet Mice. Food Funct. 10 (6), 3224–3236. doi:10.1039/c9fo00263d
Jopling, C. (2012). Liver-Specific microRNA-122: Biogenesis and Function. RNA Biol. 9 (2), 137–142. doi:10.4161/rna.18827
Kebschull, M., Fittler, M. J., Demmer, R. T., and Papapanou, P. N. (2017). Differential Expression and Functional Analysis of High-Throughput -Omics Data Using Open Source Tools. Methods Mol. Biol. 1537, 327–345. doi:10.1007/978-1-4939-6685-1_19
Krützfeldt, J., Rajewsky, N., Braich, R., Rajeev, K. G., Tuschl, T., Manoharan, M., et al. (2005). Silencing of microRNAs In Vivo With 'Antagomirs'. Nature. 438 (7068), 685–689. doi:10.1038/nature04303
Li, S., Shao, Z., Fu, X., Xiao, W., Li, L., Chen, M., et al. (2017). Identification and Characterization of Prunus Persica miRNAs in Response to UVB Radiation in Greenhouse Through High-Throughput Sequencing. BMC Genomics. 18 (1), 938. doi:10.1186/s12864-017-4347-5
Liu, S., da Cunha, A. P., Rezende, R. M., Cialic, R., Wei, Z., Bry, L., et al. (2016). The Host Shapes the Gut Microbiota via Fecal MicroRNA. Cell Host Microbe. 19 (1), 32–43. doi:10.1016/j.chom.2015.12.005
Lu, J. M., Wang, Y. F., Yan, H. L., Lin, P., Gu, W., and Yu, J. (2016). Antidiabetic Effect of Total Saponins From Polygonatum Kingianum in Streptozotocin-Induced Daibetic Rats. J. Ethnopharmacol. 179, 291–300. doi:10.1016/j.jep.2015.12.057
Luis, A. S., Briggs, J., Zhang, X., Farnell, B., Ndeh, D., Labourel, A., et al. (2018). Dietary Pectic Glycans Are Degraded by Coordinated Enzyme Pathways in Human Colonic Bacteroides. Nat. Microbiol. 3 (2), 210–219. doi:10.1038/s41564-017-0079-1
Neyrinck, A. M., Possemiers, S., Druart, C., Van de Wiele, T., De Backer, F., Cani, P. D., et al. (2011). Prebiotic Effects of Wheat Arabinoxylan Related to the Increase in Bifidobacteria, Roseburia and Bacteroides/Prevotella in Diet-Induced Obese Mice. PLoS One. 6 (6), e20944. doi:10.1371/journal.pone.0020944
Singh, N., Shirdel, E. A., Waldron, L., Zhang, R. H., Jurisica, I., and Comelli, E. M. (2012). The Murine Caecal microRNA Signature Depends on the Presence of the Endogenous Microbiota. Int. J. Biol. Sci. 8 (2), 171–186. doi:10.7150/ijbs.8.171
Sliwinska, A., Kasinska, M. A., and Drzewoski, J. (2017). MicroRNAs and Metabolic Disorders - Where Are We Heading? Arch. Med. Sci. 13 (4), 885–896. doi:10.5114/aoms.2017.65229
Sud, N., Zhang, H., Pan, K., Cheng, X., Cui, J., and Su, Q. (2017). Aberrant Expression of microRNA Induced by High-Fructose Diet: Implications in the Pathogenesis of Hyperlipidemia and Hepatic Insulin Resistance. J. Nutr. Biochem. 43, 125–131. doi:10.1016/j.jnutbio.2017.02.003
Tang, X., Muniappan, L., Tang, G., and Ozcan, S. (2009). Identification of Glucose-Regulated miRNAs from Pancreatic {beta} Cells Reveals a Role for miR-30d in Insulin Transcription. RNA. 15 (2), 287–293. doi:10.1261/rna.1211209
Wang, K., Long, B., Jiao, J. Q., Wang, J. X., Liu, J. P., Li, Q., et al. (2012). miR-484 Regulates Mitochondrial Network Through Targeting Fis1. Nat. Commun. 3, 781. doi:10.1038/ncomms1770
Wang, Y., Peng, M., Chen, Y., Wang, W., He, Z., Yang, Z., et al. (2019). Analysis of Panax Ginseng miRNAs and Their Target Prediction Based on High-Throughput Sequencing. Planta Med. 85 (14-15), 1168–1176. doi:10.1055/a-0989-7302
Wei, R., Qiu, D., Wilson, I. W., Zhao, H., Lu, S., Miao, J., et al. (2015). Identification of Novel and Conserved microRNAs in Panax Notoginseng Roots by High-Throughput Sequencing. BMC Genomics. 16, 835. doi:10.1186/s12864-015-2010-6
Wold, B., and Myers, R. M. (2008). Sequence Census Methods for Functional Genomics. Nat. Methods. 5 (1), 19–21. doi:10.1038/nmeth1157
Yan, H., Lu, J., Wang, Y., Gu, W., Yang, X., and Yu, J. (2017). Intake of Total Saponins and Polysaccharides From Polygonatum Kingianum Affects the Gut Microbiota in Diabetic Rats. Phytomedicine. 26, 45–54. doi:10.1016/j.phymed.2017.01.007
Yang, X. X., Wang, X., Shi, T. T., Dong, J. C., Li, F. J., Zeng, L. X., et al. (2019). Mitochondrial Dysfunction in High-Fat Diet-Induced Nonalcoholic Fatty Liver Disease: The Alleviating Effect and its Mechanism of Polygonatum Kingianum. Biomed. Pharmacother. 117, 109083. doi:10.1016/j.biopha.2019.109083
Yang, X. X., Wei, J. D., Mu, J. K., Liu, X., Dong, J. C., Zeng, L. X., et al. (2018). Integrated Metabolomic Profiling for Analysis of Antilipidemic Effects of Polygonatum Kingianum Extract on Dyslipidemia in Rats. World J. Gastroenterol. 24 (48), 5505–5524. doi:10.3748/wjg.v24.i48.5505
Yao, Z. Y., Chen, W. B., Shao, S. S., Ma, S. Z., Yang, C. B., Li, M. Z., et al. (2018). Role of Exosome-Associated microRNA in Diagnostic and Therapeutic Applications to Metabolic Disorders. J. Zhejiang Univ. Sci. B. 19 (3), 183–198. doi:10.1631/jzus.B1600490
Zhao, H. l., Xu, Y., Zhao, H. Y., Liu, Y., and Zhao, W. M. (2015). Effect of Polygonatum Polysaccharide on SREBP-1c and SCD-1 Protein Expression in Type 2 Diabetic Rats. Pharmacol. Clin. Chin. Materia Med. (01), 106–109. doi:10.13412/j.cnki.zyyl.2015.01.034
Zheng, Y., Chen, C., Liang, Y., Sun, R., Gao, L., Liu, T., et al. (2019). Genome-Wide Association Analysis of the Lipid and Fatty Acid Metabolism Regulatory Network in the Mesocarp of Oil palm (Elaeis Guineensis Jacq.) Based on Small Noncoding RNA Sequencing. Tree Physiol. 39 (3), 356–371. doi:10.1093/treephys/tpy091
Keywords: microRNA, Polygonatum kingianum Coll. et Hemsl, gut microbiota, lipid metabolism, high-throughput sequencing, correlation analysis
Citation: Dong J, Gu W, Yang X, Zeng L, Wang X, Mu J, Wang Y, Li F, Yang M and Yu J (2021) Crosstalk Between Polygonatum kingianum, the miRNA, and Gut Microbiota in the Regulation of Lipid Metabolism. Front. Pharmacol. 12:740528. doi: 10.3389/fphar.2021.740528
Received: 04 August 2021; Accepted: 27 September 2021;
Published: 27 October 2021.
Edited by:
Vincent Kam Wai Wong, Macau University of Science and Technology, Macao, SAR ChinaReviewed by:
Huaiyou Wang, Henan University, ChinaMin Wu, China Academy of Chinese Medical Sciences, China
Guoxin Huang, Macau University of Science and Technology, Macao, SAR China
Copyright © 2021 Dong, Gu, Yang, Zeng, Wang, Mu, Wang, Li, Yang and Yu. This is an open-access article distributed under the terms of the Creative Commons Attribution License (CC BY). The use, distribution or reproduction in other forums is permitted, provided the original author(s) and the copyright owner(s) are credited and that the original publication in this journal is cited, in accordance with accepted academic practice. No use, distribution or reproduction is permitted which does not comply with these terms.
*Correspondence: Jie Yu, eXVqaWVAeW51dGNtLmVkdS5jbg==