- Division of Clinical Pharmacology and Toxicology, Department of Anaesthesiology, Pharmacology, Intensive Care and Emergency Medicine, University Hospitals of Geneva, Geneva, Switzerland
Pharmacokinetic variability is a major source of differences in drug response and can be due to genetic variants and/or drug-drug interactions. Cytochromes P450 are among the most studied enzymes from a pharmacokinetic point of view. Their activity can be measured by phenotyping, and/or predicted by genotyping. Depending on the presence of drugs and/or diseases that can affect their in vivo activity, both approaches can be complementary. In 2014, the Geneva cocktail using dried blood spots was validated in healthy volunteers for CYP450 phenotyping. Since its clinical implementation, it has been used in approximately 500 patients in various clinical situations. Our study aims to report the concordance between CYP450 genotype and phenotype in real-life patients. The prospectively collected data from patients who were genotyped and/or phenotyped between January 2014 and December 2020 were reviewed. A total of 537 patients were genotyped and/or phenotyped for CYP450 during this period, and 241 underwent simultaneous genotyping and phenotyping allowing for genotype/phenotype concordance assessment. Genotyping correctly predicted poor metabolizer phenotypes for most CYPs isoenzymes studied, whereas agreement was more variable for intermediate, normal, and ultrarapid metabolizers. Discrepancies between the phenotype predicted on the basis of genotyping and the measured phenotype were not always explained by concurrent medication (phenotypic switch). Therefore genotyping and phenotyping tests are complementary approaches when aiming to individualize drug therapy. In the 537 patients, the majority of clinical situations were observed with analgesic/anesthetic drugs (n = 187), followed by antidepressants (n = 153), antineoplastics (n = 97), and immunosuppressants (n = 93). Inefficacy (or low drug levels) and adverse drug reaction (or high drug levels) were the main reasons for testing. Genotype and/or phenotype results explained or at least contributed to the clinical event in 44% of cases.
Introduction
Patients vary in their response to drugs. A dose that is effective in a given patient may cause an adverse drug reaction (ADR) in another patient or conversely be ineffective. Several causes of variability can be cited, genetic- or disease-related changes in drug concentrations or responsiveness, poor compliance, drug-drug interactions (DDI). Variability in drug response can affect pharmacokinetics, pharmacodynamics, or both (Roden et al., 2019). Pharmacokinetic variability is a major source of differences in drug response and can be due to genetic variants, diseases themselves and/or DDI. Cytochromes P450 (CYP450) are among the most studied enzymes from a pharmacokinetic point of view. Their activity can be predicted by genotyping and/or measured by phenotyping.
Genotyping consists of determining the patient DNA sequence and analyzing functional genetic variants coding for specific enzymes. It allows predicting the phenotype based on the identified alleles (Samer et al., 2013). The genotype offers the advantage of being immutable in a given patient. However, predicting metabolic phenotype from genotype may be challenging for CYP450 enzymes, especially given the continuously increasing number of novel alleles being discovered (Shah et al., 2016). In practice, the genotype does not necessarily correlate well with the phenotype (Waring, 2020). Another major issue is that genotyping does not account for any of the many environmental factors (diseases, drug interactions, dietary) which may impact phenotype (McGraw et al., 2018).
On the other hand, phenotyping can be considered a more useful tool for patient metabolism evaluation to anticipate possible inefficacy or ADR at conventional doses (Keller et al., 2017). However, it implies the oral administration of probe specific xenobiotics, followed by blood or urine sampling. This may represent a limitation in vulnerable populations such as children and pregnant women. Therefore, we believe that genotyping and phenotyping are complementary in clinical settings, depending on the presence of drugs and/or diseases that may affect the in vivo activity of CYP450.
At Geneva University Hospitals, we have been using, CYP450 genotyping and phenotyping methods in patients for almost two decades. In vivo phenotyping can be performed by administering a single probe drug metabolized by an individual CYP enzyme, or by a “cocktail” approach, consisting in administering several probe drugs. The cocktail approach allows for the simultaneous determination of several CYP enzymes activity (Keller et al., 2017). The first Geneva phenotyping cocktail for CYP450 phenotyping was developed in 2004. It contained 5 probe substrates used at therapeutic doses (100 mg caffeine, 50 mg flurbiprofen, 40 omeprazole, 25 mg dextromethorphan, and 7.5 mg midazolam), thus associated with a risk of therapeutic unwanted effects (Jerdi et al., 2004). Our clinical experience in patients using the “full dose” phenotyping cocktail and its subsequent variants has been published previously with psychotropic drugs (Lloret-Linares et al., 2016) and analgesic drugs (Rollason et al., 2020a). In 2014, a new mixed-probe of the Geneva cocktail, which is called the Geneva microcoktail, using dried blood spots, was validated in healthy volunteers. The Geneva micrococktail contains smaller doses of probe substrates: 50 mg caffeine, 20 mg bupropion, 10 mg flurbiprofen, 10 omeprazole, 10 mg dextromethorphan, 1 mg midazolam, and 25 mg fexofenadine (Bosilkovska et al., 2014), facilitates sample collection, requiring only 10 µL blood samples, and allows phenotyping for additional CYPs and P-glycoprotein. This new formulation of the Geneva cocktail recently showed an excellent safety profile in 265 healthy volunteers from different geographic regions (Rollason et al., 2020b). It was also used to characterize the variation in CYP450 activity in patients undergoing elective orthopedic surgery (Lenoir et al., 2020).
The clinical use of the Geneva micrococktail in real-life polymorbid and/or polymedicated patients has not been previously reported. Since its implementation, we have used it in approximately 500 patients. Our study aims to report the concordance between CYP450 genotype and phenotype using the Geneva micrococktail in real-life patients. Second, we aim to determine if genotyping and/or phenotyping help explaining unexpected clinical responses to drug administration (ADR, or inefficacy).
Methods
Patients and Setting
The protocol of this retrospective study was approved by the Ethics Commission of the Canton of Geneva, Geneva (Switzerland) (study number: 15-225). We evaluated in- and outpatients with CYP450 genotyping and/or phenotyping tests since 2014, the year of implementation of the Geneva micrococktail as used in its current formulation. As described previously (Lloret-Linares et al., 2016; Rollason et al., 2020a), we retrospectively collected results of the genetic and/or phenotypic investigations made because of non-response to drugs or excessive response to drugs performed between April 2014 and December 2020. Our previously published worked included patients who underwent phenotyping until November 2014. Since we included patients from April 2014, there is an overlap of 18 patients included in the previous as well as in the present article. In the present paper, we will focus on genotype-phenotype concordance. Therefore, patients with genotype-only testing will not be discussed in detail unlike our previously published articles. Patients with only non-CYP450 genotyping (e.g. ABCB1, COMT) were excluded from the analysis.
Genotyping
Genotyping was performed by our institutional laboratory of molecular oncology and pharmacogenomics. Genotyping techniques have considerably evolved over the last few years. From 2017, the used method was based on Next Generation Sequencing (NGS) technologies with the pharmacogenomics panel from ThermoFisher. CYP2D6 gene copy number was determined by qPCR on LC480 (Roche) using CNV Exon 9 Hs00010001_cn and CNV Intron 6 Hs04502391_cn probes for CYP2D6 (Life Technologies, with RNAse P gene used as reference gene). AlleleTyper Software (Thermo Fisher Scientific) was used to translate genetic pattern information from genotyping (Single-nucleotide polymorphisms—SNP) and copy number assay to their standardized allele name or star (*) allele nomenclature. For the NGS, the considered alleles are detailed in Table 1.
The predicted phenotypes were based on enzyme activities of the identified alleles, as listed in the Pharmacogene Variation (PharmVar) Consortium database (Gaedigk et al., 2018; Gaedigk et al., 2019) or the PharmGKB database (Whirl-Carrillo et al., 2012). Patients were classified into poor metabolizer (PM), intermediate metabolizer (IM), normal metabolizer (NM), and ultra-rapid metabolizer (UM). For the predicted phenotype of the combined CYP3A4 and CYP3A5 genotypes, we used the classification as described by Andreu et al. PMs were defined as CYP3A4*22 carriers with the CYP3A5*3/*3 genotype, IMs were CYP3A4*22 non-carriers with the CYP3A5*3/*3 genotype or CYP3A4*22 carriers with the CYP3A5*1/*1 genotype, and NMs were CYP3A4*22 non-carriers and CYP3A5*1 carriers (Andreu et al., 2017).
Phenotyping
Phenotyping was performed using the Geneva micrococktail which contained 50 mg caffeine, 20 mg bupropion, 10 mg flurbiprofen, 10 mg omeprazole, 10 mg dextromethorphan, 1 mg midazolam, and 25 mg fexofenadine. These probe substrates allow in vivo phenotyping of CYP1A2, CYP2B6, CYP2C9, CYP2C19, CYP2D6, CYP3A4/5 and P-glycoprotein, respectively (Bosilkovska et al., 2014). As previously described, the micrococktail was given orally on an empty stomach. Two hours after administration, capillary blood samples or venous blood samples were collected. In cases venous blood samples were taken, blood spots of 10 µL each were spotted on a dedicated filter card. Dried blood spots were stored at −20°C in a sealable plastic bag until analysis by a validated method using liquid chromatography tandem mass spectrometry. Phenotype determination was based on the metabolite to parent drug metabolic ratio (MR). As for genotyping, patients were classified as PM, IM, NM, and UM according to their MR (Lenoir et al., 2020).
Clinical Data
For each patient, we collected demographic data, as well as relevant medical history and current treatments, including complementary and alternative medicine therapies. When applicable, current concomitant drugs were classified as CYP inhibitors or inducers based on our table “Interactions médicamenteuses, Cytochromes P450 et P-glycoprotéine P” (Service de pharmacologie et toxicologie cliniques and HUG, 2020), and the summary of product characteristics.
We also collected the main therapeutic classes according to ATC classification and the main reason for genotyping/phenotyping, which could be one of the following: ADR/high drug levels, inefficacy/low drug levels, DDI, International Normalized Ratio (INR) variation, prescription (preemptive testing), and other (cases not concerned by any of the other categories). Finally, based on the conclusions of the interpretive report written by a senior clinical pharmacologist, we determined whether the genotype/phenotype explained the clinical event.
Statistical Analysis
Descriptive statistics were used. Categorical and continuous variables were described using frequency tables (n, %) and median (range), respectively. Genotype-phenotype concordance was considered adequate when the phenotype was equal to the predicted genotype based on the identified alleles. All analyses were performed using the SPSS® software package, version 25 (IBM corporation, Armonk, NY, United States).
Results
Population Characteristics
Between January 2014 and December 2020, a total of 551 patients underwent genotyping and/or phenotyping tests. Among them, 13 patients had only non-CYP450 genotyping (ABCB1, COMT, SLCO1B1, and OPRM1) and one had missing data; these patients were therefore excluded from the analysis (Figure 1). The mean age of the 537 remaining patients was 48.6 years old (range: one month-90 years) and 52.3% (n = 281) were women. Among the 537 patients, 241 (45%) underwent simultaneous genotyping and phenotyping, 235 (44%) underwent phenotyping only, and 61 (11%) underwent genotyping only (Figure 1).
Genotype and Phenotype
A total of 241 patients underwent simultaneous genotyping and phenotyping, allowing for genotype-phenotype concordance assessment. The majority of patients received the Geneva microcktail (n = 212) while the other patients (n = 29) had targeted phenotyping, i.e. focusing on one, two, or eventually three CYP enzymes, usually in children to minimize the exposure to non-authorized substances in this population, or because targeted phenotyping was justified by the clinical context. The predicted phenotypes according to the identified alleles are presented in Table 2. Figure 2 shows the distribution of metabolic ratios for each phenotype predicted by the genotype. The concordance between the predicted and measured phenotypes is presented in Table 3. As illustrated in Figure 2 and Table 3, genotyping allowed correct prediction of PM phenotypes for most of the studied CYPs isoenzymes, with a 100% concordance between the predicted and measured phenotype for CYP2C9, CYP2C19, and CYP2D6 PM. On the other hand, concordance rates between the predicted and measured phenotype for IM, NM, and UM, whatever the considered isoenzyme, varied widely with concordance rates ranging from 19 to 100%. CYP2C9 phenotypic IM and NM were correctly predicted by the genotype in approximately 60% of the cases. CYP2C19 and CYP2D6 phenotypic IM were frequently well predicted by the genotype (concordance in 91 and 73% of the cases respectively) whereas the opposite was true for CYP2C19, CYP2D6, and CYP3A4/5 NM, in which a concordance between the predicted and measured phenotype was observed in 30–38% of cases only. Finally, except for CYP2B6, individuals with a UM genotype frequently displayed a discordant phenotype (63% of cases for CYP1A2, and 80% of cases for CYP2C19 and CYP2D6). In these cases of discordance, a phenotypic switch due to the concomitant use of a CYP inhibitor explained 14–47% of cases.
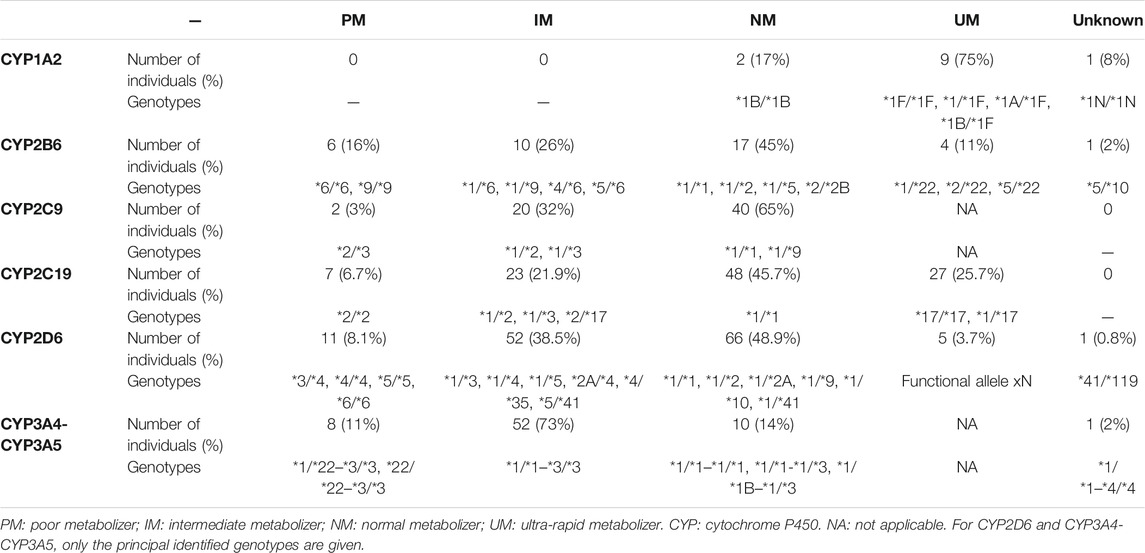
TABLE 2. In patients with simultaneous genotyping and phenotyping, CYP predicted phenotype based on the identified alleles.
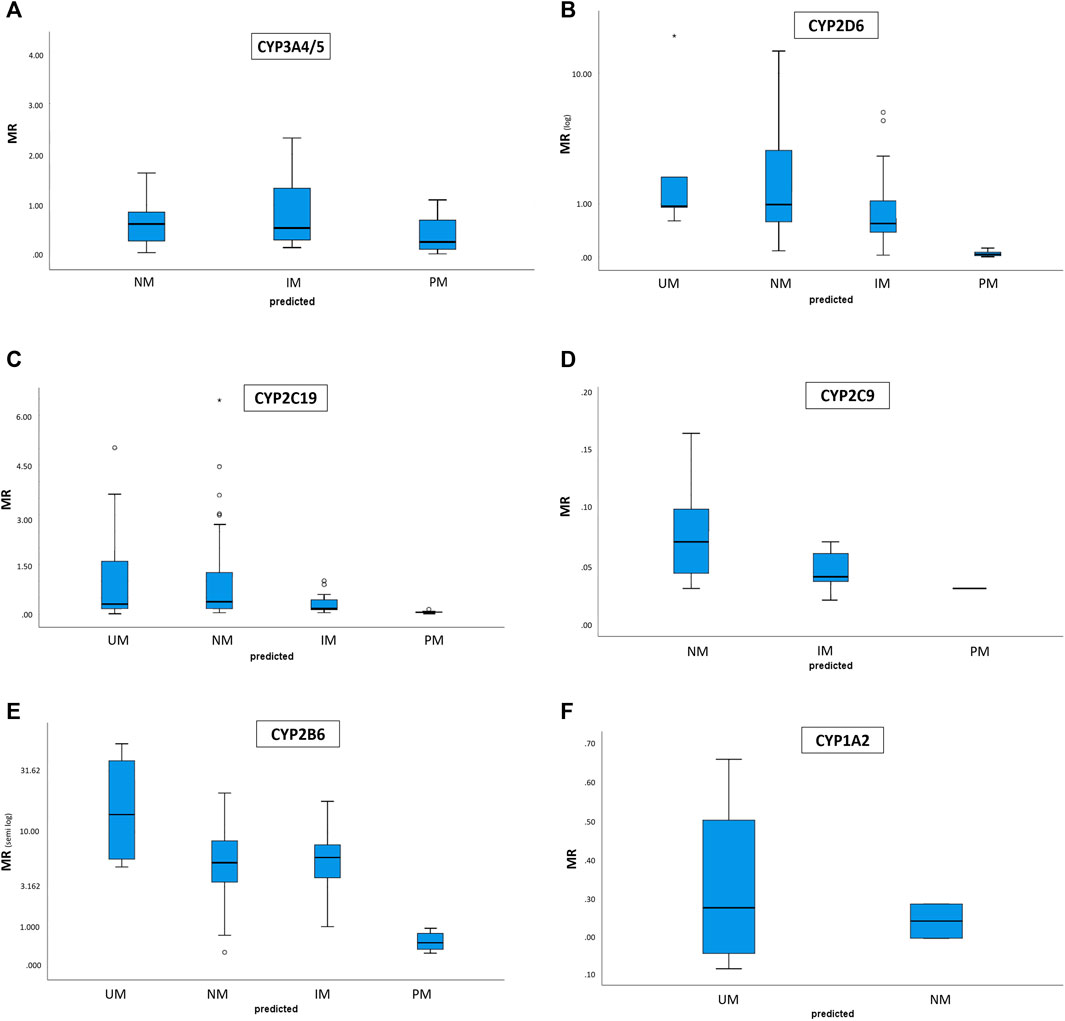
FIGURE 2. Distribution of metabolic ratios (y axis) for each phenotype predicted by the genotype (x axis). Boxes indicate the interquartile ranges. (A) CYP1A2. (B) CYP2B6. (C) CYP2C9. (D) CYP2C19. (E) CYP2D6. (F) CYP3A4 and CYP3A5.
As illustrated in Figure 2, for CYP1A2, carriers and non-carriers of the CYP1A2*1F allele, which has been associated with increased inducibility (Thorn et al., 2012), had great overlap in their MR. For CYP2B6, patients predicted as NM et IM according to their genotype also displayed great overlap in their MR. This wide distribution of MR associated with overlap between phenotype subgroups was also observed for CYP2C19 genotypic UM and NM, CYP2D6 genotypic UM and NM, and CYP3A genotypic NM and IM.
Phenotype
A total of 235 patients underwent phenotyping only. The majority of patients received the Geneva microcktail (n = 218) while the other patients (n = 17) had targeted phenotyping for the reasons explained previously. The distribution of the phenotypes, according to CYP isoenzymes is presented in Table 4. As expected, the majority of patients were categorized as NM, regardless of the isoenzyme examined. The proportion of patients displaying slow CYP metabolism (PM + IM) was low for CYP2B6 (approximately 2%), intermediate for CYP1A2 (10%), CYP2C9 (18%), and CYP3A (17%), and high for CYP2C19 and CYP2D6 (more than 30%). Finally, the proportion of patients categorized as UM was quite high for CYP1A2 (24%) and CYP2B6 (15%), while it was measured around 7–11% for the other isoenzymes (CYP2C9, CYP2C19, CYP2D6, and CYP3A).
Genotype
Sixty-one patients underwent genotyping only, with a total of 115 genes evaluated. The most frequently investigated enzyme was CYP2D6 (n = 33), followed by CYP3A4 and CYP3A5 (n = 23 for both). Other genes were infrequently (CYP2C19 n = 16; CYP2C9 n = 10; CYP2B6 n = 8) or very rarely (CYP1A2 n = 2) investigated.
Association Between Clinical Response and Genotype And/Or Phenotype
The majority of clinical situations were observed with analgesic/anesthetic drugs (n = 187), followed by antidepressants (n = 153), antineoplastics (n = 97), and immunosuppressants (n = 93) (Figure 3).
Genotypic and/or phenotypic explorations were mainly performed because of inefficacy/low drug levels (38%) followed by ADR/high drug levels (33%), and preemptively before prescribing (18%). Based on the clinical report, the genotype and/or phenotype results interestingly explained or at least contributed to almost half of the clinical event (44% of the cases). In 26% of the cases, the association between the genotype/phenotype and a clinical event could not be evaluated (preemptive testing, evaluation of a DDI, INR variation) (Table 5). In the 237 cases where the genotype/phenotype could contribute to the clinical event, the differential contribution of the different tests were as followed. In 47% of the patients who underwent genotyping only, the results explained or contributed to the clinical event. This proportion was 34% in patients who underwent phenotyping only, and 53% in patients who underwent simultaneous genotyping and phenotyping. In most of the patients in whom the genotype provided an explanation, the clinical event was ADR/high drug levels and the most frequently involved therapeutic classes were psychotropic and analgesic/anesthetic drugs. In most of the patients in whom the phenotype provided an explanation, the clinical event was of inefficacy/low drug levels, with also psychotropic and analgesic/anesthetic drugs frequently involved. Finally, combined genotype and phenotype mostly explained ADR/high drug levels with various therapeutic classes involved (psychotropic, analgesic, antineoplastic, immunosuppressant, and antithrombotic drugs).
Discussion
Our retrospective study describes for the first time the clinical use of the Geneva CYP450 phenotyping micrococktail in patients suffering from various comorbidities and taking numerous comedications. The new version of the Geneva phenotyping cocktail using low doses of probe substrates was validated in 2014 in healthy volunteers (Bosilkovska et al., 2014) using CYP inhibitors and inducers to generate MR thresholds allowing to characterize the different phenotypes (e.g. PM, NM, UM). We report here concordance between the genotypes and the measured phenotypes as well as associated MR. We showed that the concordance between the predicted and measured phenotypes was excellent for PM of all CYPs. Genotypic prediction of CYP2C9, CYP2C19, and CYP2D6 IM was satisfying to good, and prediction performance of CYPs NM was moderate. On the other hand, discrepancies were frequent for patients predicted as UM. Discrepancies between the predicted phenotype as based on genotyping and the measured phenotyping were sometimes explained by concurrent medication (phenotypic switch). A previous article from our research group showed that the overall concordance rate between the predicted and measured phenotype was around 50% for CYP2D6 and CYP2C9 using a previous version of the Geneva cocktail (Rollason et al., 2020b). The poor correlation between genotype and phenotype for UM had already been shown previously for CYP2D6 using the AmpliChip CYP450 test for genotyping and dextromethorphan/dextrorphan urine metabolic ratio for phenotyping (Rebsamen et al., 2009). More recently, Dorado et al. also showed that CYP2D6 genotype was not a good predictor of UM phenotype as measured with debrisoquin probe drug. Only 25% of phenotypic UM were explained by their genotype (carrying more than two active CYP2D6 genes) (Dorado et al., 2017). De Andrés et al. evaluated the correlation between CYPs genotype and phenotype using a cocktail approach in several populations (De Andrés et al., 2016; de Andrés et al., 2017; de Andrés et al., 2020). Their different studies showed that the drug-metabolizing capacity predicted from the genotype was frequently not concordant with the actual capacity as measured by phenotyping. For example, in their study in Mexican Amerindian, CYP2C19 genotypic UM displayed a wide range of MR, and several CYP2C19 genotypic NM had higher metabolic activity than UM. Similar to our results, they observed no association between CYP1A2*1F and enhanced CYP1A2 metabolic capacity (de Andrés et al., 2017).
Our results and those from others underscore the complementary roles of genotyping and phenotyping tests when aiming to personalize patient treatment. To minimize the risk of ADRs or therapeutic failure, the individual’s drug metabolic capacities should be assessed at the time of initiation of treatment, for drugs metabolized by CYPs. Since such preemptive testing is time-consuming and might be difficult to implement in clinical practice, the simpler approach of genotyping has been suggested. Indeed, since the genome is constant throughout a lifetime, this might in theory be a useful surrogate marker for drug toxicity or inefficacy (Waring, 2020). However, prediction from the genotype can be limited by the lack of accurate results for unknown genotypes/variants (particularly with NGS technologies) and misclassification of phenotype due to errors in genetic predictions. Moreover, measured phenotype can be influenced by environmental factors such as concomitant drugs, dietary habits, or concomitant diseases (McGraw et al., 2018).
The complex interplay between genetics and drug-drug interaction has been recently reviewed by Storelli et al. Through a systematic review of case reports, they identified several mechanisms of complex gene-drug interaction such as enhancement of the magnitude of interaction due to a genetic variant directly impacting the CYP isoform of interest, increased vulnerability to phenoconversion caused by a genetic variant directly affecting the inhibited/induced metabolic pathway, increased exposure of the perpetrator drug due to genetic polymorphisms, and modification of the relative contribution of a minor pathway by a genetic variant affecting the major pathway. For example, a PM of a specific CYP isoform will not be greatly affected by an inhibitor of this isoform. In healthy CYP2D6 PM Caucasian volunteers, no increase in the AUC of metoprolol was observed when coadministered with dronedarone, a moderate CYP2D6 inhibitor (Storelli et al., 2018). In other words, CYP2D6 PMs are not sensitive to phenoconversion or phenotypic switch by CYP2D6 inhibitors. Phenoconversion corresponds to the modification of the activity of a drug-metabolizing enzyme by an inhibitor/inducer that mimics a genetic defect. For example, a CYP2D6 genotypic EM patient undergoing phenoconversion with a CYP2D6 inhibitor would behave pharmacologically as a PM. Other causes of phenoconversion include liver transplant or liver disease (Shah and Smith, 2015) as well as possibly other diseases (inflammation, surgery, etc.).
From a clinical point of view, the genotypes and/or phenotypes contributed to the observed clinical event (i.e. inefficacy, ADR) in 44% of the cases globally. When looking in more detail into the differential contribution of genotyping versus phenotyping results, we observed that simultaneous genotyping and phenotyping allowed explaining the clinical event in a greater proportion of patients then when doing genotyping or phenotyping only. This 44% proportion is quite similar to previously published results from our group focusing on analgesic (Rollason et al., 2020a) and psychotropic drugs (Lloret-Linares et al., 2017), with data collected until the end of 2014. As in the previous analysis, the main therapeutic classes in our study were analgesic/anesthetic drugs and antidepressants. A possible bias in this observation is the other clinical specialty of clinical pharmacologists in our division (experts in pain and psychopharmacology). On the other hand, many drugs from these two therapeutic classes are also subject to metabolism by CYP450, therefore potentially influenced by CYP genetic polymorphisms. This is reflected by numerous genotype-based dosing recommendations found on the PharmaGKB website (https://www.pharmgkb.org/).
When classifying patients in metabolic subgroups according to their genotypes, there were around 8% of CYP2D6 PM, 39% of IM, 49% of NM, and 4% of UM. Geneva is considered to have a population from various ethnicities. Our frequencies of extreme metabolizer (PM and UM) were similar to those reported by del Tredici et al. in their study conducted in more than 100’000 patients in the US from multiple ethnic groups (6% PM and 2% UM), while IM were more prevalent in our study (39 versus 11%) (Del Tredici et al., 2018). As reported by Gaedick et al., the distribution frequency of CYP2D6 shows considerable differences across different world populations (Gaedigk et al., 2017). Regarding CYP2C19, we found 7% of PM, 20% of IM, 45% of NM, and 25% of rapid/ultrarapid metabolizers. Our frequency of PM was higher than what is usually observed in Caucasians from different parts of the world as reported by Fricke-Galindo et al. (2–3% approximately), but the frequency of UM was comparable (Fricke-Galindo et al., 2016). The frequency of CYP2C19 PM was also much higher in our study than reported by Fricke-Galindo et al. for Europe (20.3 versus 2.2% (Fricke-Galindo et al., 2016).
Our study has some limitations. Bias related to retrospective analysis might have led to missing information, such as follow-up data if the genotyping and/or phenotyping results led to dosage/therapeutic changes. For some isoenzymes such as CYP1A2 and CYP2B6, the limited number of patients undergoing simultaneous genotyping and phenotyping limited definitive conclusions on genotype/phenotype concordance.
Conclusion
Our study reported for the first time the clinical use of the Geneva micrococktail in patients as well as genotype/phenotype concordance. We showed that genotyping and/or phenotyping tests were useful in explaining or solving clinical events in almost half of the cases. We also observed that genotype/phenotype concordance was excellent for poor metabolizers but more variable for normal, and ultrarapid metabolizers. Our results highlight the complementary aspects of genotyping and phenotyping tests in helping to individualize drug therapy, and these tests should therefore be offered concomitantly more routinely in the clinic.
Data Availability Statement
The raw data supporting the conclusion of this article will be made available by the authors, without undue reservation.
Ethics Statement
The studies involving human participants were reviewed and approved by the Ethics Commission of the Canton of Geneva. Written informed consent to participate in this study was provided by the participants’ legal guardian/next of kin.
Author Contributions
KI, VR, MB, JD, and CS conceived the study concept and design. YD performed the phenotyping analyses. KI and SB acquired and analyzed the data. KI wrote the first draft of the manuscript. All authors participated in refining, and reviewing the manuscript, and approved the final version.
Conflict of Interest
The authors declare that the research was conducted in the absence of any commercial or financial relationships that could be construed as a potential conflict of interest.
Publisher’s Note
All claims expressed in this article are solely those of the authors and do not necessarily represent those of their affiliated organizations, or those of the publisher, the editors and the reviewers. Any product that may be evaluated in this article, or claim that may be made by its manufacturer, is not guaranteed or endorsed by the publisher.
References
Andreu, F., Colom, H., Elens, L., van Gelder, T., van Schaik, R. H., Hesselink, D. A., et al. (2017). A New CYP3A5*3 and CYP3A4*22 Cluster Influencing Tacrolimus Target Concentrations: A Population Approach. Clin. Pharmacokinet. 56, 963. doi:10.1007/s40262-016-0491-3
Bosilkovska, M., Samer, C. F., Déglon, J., Rebsamen, M., Staub, C., Dayer, P., et al. (2014). Geneva Cocktail for Cytochrome P450 and P-Glycoprotein Activity Assessment Using Dried Blood Spots. Clin. Pharmacol. Ther. 96, 349–359. doi:10.1038/clpt.2014.83
de Andrés, F., Altamirano-Tinoco, C., Ramírez-Roa, R., Montes-Mondragón, C. F., Dorado, P., Peñas-Lledó, E. M., et al. (2020). Relationships between CYP1A2, CYP2C9, CYP2C19, CYP2D6 and CYP3A4 Metabolic Phenotypes and Genotypes in a Nicaraguan Mestizo Population. Pharmacogenomics J. 21, 140. doi:10.1038/s41397-020-00190-9
de Andrés, F., Sosa-Macías, M., Ramos, B. P. L., Naranjo, M. G., and LLerena, A. (2017). CYP450 Genotype/Phenotype Concordance in Mexican Amerindian Indigenous Populations-Where to from Here for Global Precision Medicine? OMICS 21, 509–519. doi:10.1089/omi.2017.0101
De Andrés, F., Terán, S., Hernández, F., Terán, E., and LLerena, A. (2016). To Genotype or Phenotype for Personalized Medicine? CYP450 Drug Metabolizing Enzyme Genotype-Phenotype Concordance and Discordance in the Ecuadorian Population. OMICS 20, 699–710. doi:10.1089/omi.2016.0148
Del Tredici, A. L., Malhotra, A., Dedek, M., Espin, F., Roach, D., Zhu, G. D., et al. (2018). Frequency of CYP2D6 Alleles Including Structural Variants in the United States. Front. Pharmacol. 9, 305. doi:10.3389/fphar.2018.00305
Dorado, P., Gonzalez, I., Naranjo, M. E., de Andres, F., Penas-Lledo, E. M., Calzadilla, L. R., et al. (2017). Lessons from Cuba for Global Precision Medicine: CYP2D6 Genotype Is Not a Robust Predictor of CYP2D6 Ultrarapid Metabolism. OMICS 21, 17–26. doi:10.1089/omi.2016.0166
Fricke-Galindo, I., Cespedes-Garro, C., Rodrigues-Soares, F., Naranjo, M. E., Delgado, A., de Andres, F., et al. (2016). Interethnic Variation of CYP2C19 Alleles, 'predicted' Phenotypes and 'measured' Metabolic Phenotypes across World Populations. Pharmacogenomics J. 16, 113–123. doi:10.1038/tpj.2015.70
Gaedigk, A., Ingelman-Sundberg, M., Miller, N. A., Leeder, J. S., Whirl-Carrillo, M., and Klein, T. E. (2018). The Pharmacogene Variation (PharmVar) Consortium: Incorporation of the Human Cytochrome P450 (CYP) Allele Nomenclature Database. Clin. Pharmacol. Ther. 103, 399–401. doi:10.1002/cpt.910
Gaedigk, A., Sangkuhl, K., Whirl-Carrillo, M., Klein, T., and Leeder, J. S. (2017). Prediction of CYP2D6 Phenotype from Genotype across World Populations. Genet. Med. 19, 69–76. doi:10.1038/gim.2016.80
Gaedigk, A., Sangkuhl, K., Whirl-Carrillo, M., Twist, G. P., Klein, T. E., and Miller, N. A. (2019). The Evolution of PharmVar. Clin. Pharmacol. Ther. 105, 29–32. doi:10.1002/cpt.1275
Jerdi, M. C., Daali, Y., Oestreicher, M. K., Cherkaoui, S., and Dayer, P. (2004). A Simplified Analytical Method for a Phenotyping Cocktail of Major CYP450 Biotransformation Routes. J. Pharm. Biomed. Anal. 35, 1203–1212. doi:10.1016/j.jpba.2004.03.021
Keller, G. A., Gago, M. L. F., Diez, R. A., and Di Girolamo, G. (2017). In Vivo Phenotyping Methods: Cytochrome P450 Probes with Emphasis on the Cocktail Approach. Curr. Pharm. Des. 23, 2035–2049. doi:10.2174/1381612823666170207100724
Lenoir, C., Daali, Y., Rollason, V., Curtin, F., Gloor, Y., Bosilkovska, M., et al. (2020). Impact of Acute Inflammation on Cytochromes P450 Activity Assessed by the Geneva Cocktail. Clin. Pharmacol. Ther. 109, 1668. doi:10.1002/cpt.2146
Lloret-Linares, C., Rollason, V., Lorenzini, K. I., Samer, C., Daali, Y., Gex-Fabry, M., et al. (2016). Screening for Genotypic and Phenotypic Variations in CYP450 Activity in Patients with Therapeutic Problems in a Psychiatric Setting, a Retrospective Study. Pharmacol. Res. 118, 104–110. doi:10.1016/j.phrs.2016.07.002
Lloret-Linares, C., Rollason, V., Lorenzini, K. I., Samer, C., Daali, Y., Gex-Fabry, M., et al. (2017). Screening for Genotypic and Phenotypic Variations in CYP450 Activity in Patients with Therapeutic Problems in a Psychiatric Setting, a Retrospective Study. Pharmacol. Res. 118, 104–110. doi:10.1016/j.phrs.2016.07.002
McGraw, J., Gerhardt, A., and Morris, T. C. (2018). Opportunities and Obstacles in Genotypic Prediction of Cytochrome P450 Phenotypes. Expert Opin. Drug Metab. Toxicol. 14, 659–661. doi:10.1080/17425255.2018.1484451
Rebsamen, M. C., Desmeules, J., Daali, Y., Chiappe, A., Diemand, A., Rey, C., et al. (2009). The AmpliChip CYP450 Test: Cytochrome P450 2D6 Genotype Assessment and Phenotype Prediction. Pharmacogenomics J. 9, 34–41. doi:10.1038/tpj.2008.7
Roden, D. M., McLeod, H. L., Relling, M. V., Williams, M. S., Mensah, G. A., Peterson, J. F., et al. (2019). Pharmacogenomics. Lancet 394, 521–532. doi:10.1016/S0140-6736(19)31276-0
Rollason, V., Lloret-Linares, C., Lorenzini, K. I., Daali, Y., Gex-Fabry, M., Piguet, V., et al. (2020a). Evaluation of Phenotypic and Genotypic Variations of Drug Metabolising Enzymes and Transporters in Chronic Pain Patients Facing Adverse Drug Reactions or Non-response to Analgesics: A Retrospective Study. J. Pers Med. 10, 198. doi:10.3390/jpm10040198
Rollason, V., Mouterde, M., Daali, Y., Cizkova, M., Priehodova, E., Kulichova, I., et al. (2020b). Safety of the Geneva Cocktail, a Cytochrome P450 and P-Glycoprotein Phenotyping Cocktail, in Healthy Volunteers from Three Different Geographic Origins. Drug Saf. 43, 1181–1189. doi:10.1007/s40264-020-00983-8
Samer, C. F., Lorenzini, K. I., Rollason, V., Daali, Y., and Desmeules, J. A. (2013). Applications of CYP450 Testing in the Clinical Setting. Mol. Diagn. Ther. 17, 165–184. doi:10.1007/s40291-013-0028-5
Service de pharmacologie et toxicologie cliniquesHUG (2020). Interactions médicamenteuses et cytochromes P450. 03.2020 Genève. Available at https://www.hug.ch/sites/interhug/files/structures/pharmacologie_et_toxicologie_cliniques/images/carte_des_cytochromes_2020.pdf.
Shah, R. R., Gaedigk, A., LLerena, A. L., Eichelbaum, M., Stingl, J., and Smith, R. L. (2016). CYP450 Genotype and Pharmacogenetic Association Studies: a Critical Appraisal. Pharmacogenomics 17, 259–275. doi:10.2217/pgs.15.172
Shah, R. R., and Smith, R. L. (2015). Addressing Phenoconversion: the Achilles' Heel of Personalized Medicine. Br. J. Clin. Pharmacol. 79, 222–240. doi:10.1111/bcp.12441
Storelli, F., Samer, C., Reny, J. L., Desmeules, J., and Daali, Y. (2018). Complex Drug-Drug-Gene-Disease Interactions Involving Cytochromes P450: Systematic Review of Published Case Reports and Clinical Perspectives. Clin. Pharmacokinet. 57, 1267. doi:10.1007/s40262-018-0650-9
Thorn, C. F., Aklillu, E., Klein, T. E., and Altman, R. B. (2012). PharmGKB Summary: Very Important Pharmacogene Information for CYP1A2. Pharmacogenet Genomics 22, 73–77. doi:10.1097/FPC.0b013e32834c6efd
Waring, R. H. (2020). Cytochrome P450: Genotype to Phenotype. Xenobiotica 50, 9–18. doi:10.1080/00498254.2019.1648911
Keywords: cytochromes P450, phenotype, genotype, phenoconversion, drug-drug interaction, inefficacy, adverse drug reaction
Citation: Ing Lorenzini K, Desmeules J, Rollason V, Bertin S, Besson M, Daali Y and Samer CF (2021) CYP450 Genotype—Phenotype Concordance Using the Geneva Micrococktail in a Clinical Setting. Front. Pharmacol. 12:730637. doi: 10.3389/fphar.2021.730637
Received: 25 June 2021; Accepted: 13 August 2021;
Published: 26 August 2021.
Edited by:
Amit V. Pandey, University of Bern, SwitzerlandReviewed by:
Volker Martin Lauschke, Karolinska Institutet (KI), SwedenJulio Benitez, University of Extremadura, Spain
Copyright © 2021 Ing Lorenzini, Desmeules, Rollason, Bertin, Besson, Daali and Samer. This is an open-access article distributed under the terms of the Creative Commons Attribution License (CC BY). The use, distribution or reproduction in other forums is permitted, provided the original author(s) and the copyright owner(s) are credited and that the original publication in this journal is cited, in accordance with accepted academic practice. No use, distribution or reproduction is permitted which does not comply with these terms.
*Correspondence: Kuntheavy Ing Lorenzini, S3VudGhlYXZ5LXJvc2VsaW5lLmluZ0BoY3VnZS5jaA==