- 1Musculoskeletal Research Laboratory, Department of Orthopedics and Traumatology, The Chinese University of Hong Kong, Hong Kong, Hong Kong, SAR China
- 2Innovative Orthopaedic Biomaterial and Drug Translational Research Laboratory, Li Ka Shing Institute of Health Sciences, The Chinese University of Hong Kong, Hong Kong, Hong Kong, SAR China
Background: Osteoarthritis (OA) is one of the main causes of disability in the elderly population, accompanied by a series of underlying pathologic changes, such as cartilage degradation, synovitis, subchondral bone sclerosis, and meniscus injury. The present study aimed to identify key genes, signaling pathways, and miRNAs in knee OA associated with the entire joint components, and to explain the potential mechanisms using computational analysis.
Methods: The differentially expressed genes (DEGs) in cartilage, synovium, subchondral bone, and meniscus were identified using the Gene Expression Omnibus 2R (GEO2R) analysis based on dataset from GSE43923, GSE12021, GSE98918, and GSE51588, respectively and visualized in Volcano Plot. Venn diagram analyses were performed to identify the overlapping DEGs (overlapping DEGs) that expressed in at least two types of tissues mentioned above. Gene Ontology (GO) enrichment analysis, Kyoto Encyclopedia of Genes and Genomes (KEGG) analysis, protein-protein interaction (PPI) analysis, and module analysis were conducted. Furthermore, qRT-PCR was performed to validate above results using our clinical specimens.
Results: As a result, a total of 236 overlapping DEGs were identified, of which 160 were upregulated and 76 were downregulated. Through enrichment analysis and constructing the PPI network and miRNA-mRNA network, knee OA-related key genes, such as HEY1, AHR, VEGFA, MYC, and CXCL12 were identified. Clinical validation by qRT-PCR experiments further supported above computational results. In addition, knee OA-related key miRNAs such as miR-101, miR-181a, miR-29, miR-9, and miR-221, and pathways such as Wnt signaling, HIF-1 signaling, PI3K-Akt signaling, and axon guidance pathways were also identified. Among above identified knee OA-related key genes, pathways and miRNAs, genes such as AHR, HEY1, MYC, GAP43, and PTN, pathways like axon guidance, and miRNAs such as miR-17, miR-21, miR-155, miR-185, and miR-1 are lack of research and worthy for future investigation.
Conclusion: The present informatic study for the first time provides insight to the potential therapeutic targets of knee OA by comprehensively analyzing the overlapping genes differentially expressed in multiple joint components and their relevant signaling pathways and interactive miRNAs.
Introduction
Osteoarthritis (OA) is the most common joint disease, mainly manifesting as pain, limited joint movement, and joint deformity. The risk factors of OA include trauma, aging, obesity, and heredity (Sandell, 2012; Chen et al., 2017a; Chang et al., 2021). During OA development, the entire joint are affected and undergo articular cartilage degeneration, osteophyte formation, subchondral sclerosis, synovitis, and meniscus degeneration, respectively, indicating the complicated and interactive OA pathogenic mechanisms (Chen et al., 2017a). The efficacies of the current treatments for OA in our clinics are limited. In recent years, exploration of disease-modifying osteoarthritis drugs (DMOADs) aiming at alleviating OA symptoms and/or prevent structural progression have drawn much attention. However, the DMOADs under research and development (R&D) and/or clinical trials mainly focus on one of the OA symptoms, such as cartilage degeneration, subchondral bone remodeling, local inflammation, or joint pain, and their potential downstream targets (Latourte et al., 2020). The possibility that newly explored-drug targets have heterogeneous expression profiles in different joint components raises uncertainty of the drug effectiveness. Besides, the R&D of joint component-specific drugs are also limited so far (Latourte et al., 2020).
Based on the rapid development of high-throughput genomics technologies, such as microarray and next-generation sequencing, bioinformatic analysis has been widely used to identify key genes, signaling pathways, and microRNAs (miRNAs) in various musculoskeletal disorders (Kang et al., 2021; Li et al., 2021; Umeno et al., 2021). So far, many studies have identified and explored potential therapeutic targets of OA based on bioinformatic screening. For example, upregulated arginase 2 (ARG2) in OA cartilage was screened out by microarray and further validated to facilitate cartilage destruction via upregulating matrix metalloproteinases (MMPs) (Choi et al., 2019). Activated osteochondral turnover, neurogenesis and inflammation in OA bone marrow lesions (BML) were also identified by microarray bioinformatically (Kuttapitiya et al., 2017). Besides, miRNA candidates that have potential as biomarkers and therapeutic targets in OA were identified and validated via comprehensively paired miRNA-messenger RNA (mRNA) analysis and functional enrichment analysis (Kung et al., 2018). In addition to identification of potential therapeutic targets, bioinformatics-based bulk sample analysis further helps classify potential OA subtypes for more precise diagnosis and personalized treatments. In recently years, several studies have stepped forward substantially in classifying potential OA subtypes based on bioinformatics (Soul et al., 2018; Yuan et al., 2020). Their surprising discoveries undoubtedly deepen our understandings on knee OA and will facilitate personalized treatments in the future. However, since previous bioinformatic studies mainly focus on one type of joint components as well, investigations on the overlapping DEGs (overlapping DEGs) in different joint components during OA development are still lacking. In 2016, about 5% overlapping DEGs were observed between the DEGs of the synovium and cartilage, while no further analysis was performed on these identified overlapping DEGs (Park and Ji, 2016). Recently, another study observed about 10% overlapping DEGs in OA cartilage and subchondral bone. They identified IL11 and CHADL as two potential therapeutic targets of OA by comparing their identified cartilage-subchondral bone overlapping DEGs with previously identified OA risk genes (Styrkarsdottir et al., 2018; Tuerlings et al., 2021).
Collectively, we believe it is meaningful to comprehensively analyze the key genes that are differentially expressed during OA development in the different joint components, including articular cartilage, subchondral bone, synovium, and meniscus, and their relevant pathways and miRNAs. Such approaches may provide clues to develop adequate treatments for OA by targeting at overlapping differentially expressed genes (DEGs) in different joint components and their relevant miRNAs and signaling pathways. The present study aims at identifying key genes, signaling pathways, and miRNAs in human knee OA by comparing the preexisting gene expression profiles derived from different joint components, including articular cartilage, synovium, subchondral bone, and meniscus. Specifically, the gene expression profiles (GSE) were obtained from the public available Gene Expression Omnibus database (GEO, http://www.ncbi.nlm.nih.gov/geo/). Gene Expression Omnibus 2R (GEO2R) was performed to identify the overlapping DEGs and followed by qRT-PCR validation. Furthermore, functional enrichment analysis, protein-protein interaction (PPI) analysis, and miRNA-mRNA interaction analysis were carried out to identify relevant signaling pathways and interactive miRNAs. This study may shed light on completer and undiscovered pathogenic mechanisms of knee OA development and pave the way toward the identification of new therapeutic targets for further R&D of effective therapies and clinical translation.
Materials and Methods
Gene Expression Profiles in Human Knee OA joint Tissues
The gene expression profiling in cartilage, synovial membrane, subchondral bone, and meniscus tissues was obtained from GEO datasets GSE43923 (Klinger et al., 2013), GSE12021 (Huber et al., 2008), GSE51588 (Chou et al., 2013), and GSE98918 (Brophy et al., 2018), respectively. Three degenerated and three intact cartilage samples were retrieved from a human dataset using the Affymetrix Human Genome U133 Plus 2.0 Array platform (GSE43923). Nine normal and ten OA synovial tissue samples were retrieved from a human dataset using the Affymetrix Human Genome U133 Array platform (GSE12021). Twenty OA and five normal medial tibial subchondral bone samples were retrieved from a human study using the Agilent-026652 Whole Human Genome Microarray 4 × 44K v2 platform (GSE51588). Twelve OA and twelve normal meniscus samples were retrieved from a human dataset using the Agilent-072363 SurePrint G3 Human GE v3 8 × 60K Microarray 039494 platform (GSE98918).
Identifying DEGs
The original gene expression profiles were analyzed by GEO2R (GEO2R, RRID:SCR_016569; https://www.ncbi.nlm.nih.gov/geo/geo2r/?acc=GSE43923, https://www.ncbi.nlm.nih.gov/geo/geo2r/?acc=GSE12021, https://www.ncbi.nlm.nih.gov/geo/geo2r/?acc=GSE51588, https://www.ncbi.nlm.nih.gov/geo/geo2r/?acc=GSE98918) to identify the upregulated and downregulated DEGs in OA joint tissues, respectively. The criteria for a DEG were |log2FC|>1 and adjusted P-value<0.05. The results were visualized in volcano plots.
Identification of Overlapping DEGs in Human KOA Joint Tissues
Venn diagram (http://bioinformatics.psb.ugent.be/webtools/Venn/;VennDiagram, RRID: SCR_002414) was used to identify upregulated and downregulated overlapping DEGs in the integral joint tissues including cartilage, synovial membrane, subchondral bone, and meniscus. A specific DEG was identified as overlapping DEG when it appeared at least in two of the joint tissues. All the DEGs were identified by comparing gene expression profiles between osteoarthritic and relatively healthy joint tissues.
Gene Ontology Enrichment and Kyoto Encyclopedia of Genes and Genomes Pathway Analysis
GO enrichment analysis and KEGG pathway analysis were performed on Metascape platform (http://metascape.org/gp/index.html#/main/step1; Metascape, RRID: SCR_016620) (Zhou et al., 2019). Upregulated or/and downregulated overlapping DEGs were listed and followed by “Custom Analysis.” GO enrichment analysis and KEGG pathway analysis were performed with the thresholds of P-value<0.05 and enrichment gene count ≥2.
Construction of the Protein-Protein Interaction Network
The Search Tool for the Retrieval of Interacting Genes/Proteins (STRING) database (https://string-db.org/; STRING, RRID: SCR_005223) was used to construct the PPI network (Szklarczyk et al., 2021). The overlapping DEGs were mapped to STRING list to perform multiple proteins search and get a PPI network with interaction scores >0.4. Cytoscape V.3.7.2 (Cytoscape, RRID: SCR_003032) was used to visualize the results from the PPI network and perform module analysis. Genes with connectivity degree ≥10 were identified as hub genes (Shannon et al., 2003).
Module Analysis
Module analysis was performed using the molecular complex detection (MCODE) plugin on Cytoscape platform (MCODE, RRID: SCR_015828; Cytoscape V.3.7.2, RRID: SCR_003032). The parameters set to identify enriched functional modules were as follows: Degree Cutoff = 2, Node Score Cutoff = 0.2, K-Core = 2 and Maxium. Depth = 100. Modules with the MCODE score ≥4 were identified as significant modules and were further evaluated for GO enrichment analysis and KEGG pathway analysis with the thresholds of P-value<0.05 and enrichment gene count >2.
Construction of the miRNA-mRNA Network
Experimentally validated key gene-related miRNAs were screened out based on key genes identified above by using miRTarBase 8.0 (http://miRTarBase.cuhk.edu.cn/; miRTarBase, RRID: SCR_017355) with strong evidence (Huang et al., 2020). Those miRNAs targeting at least two key genes were identified as key miRNAs and visualized on Cytoscape V.3.7.2 by constructing the miRNA-mRNA network.
Clinical Specimens Sampling
Clinical specimens of preserved and degenerated cartilage were harvested from osteoarthritis patients undergoing total knee arthroplasty (TKA) surgery. The included patients had no history of chronic diseases, tumors, autoimmune diseases, and viral chronic infections (hepatitis B virus, hepatitis C virus, human immunodeficiency virus). All patients provided informed consent, and this study was approved by the Joint Chinese University of Hong Kong-New Territories East Cluster Clinical Research Ethics Committee (CREC Ref. No: 2013.248). The estimated sample size equaled to three based on a pilot study. A total of three donors (Age: 70.17 ± 3.66; gender: one male and two females; K-L: grade III) were included. The cartilage extracted from hypertrophic and the severely destructed region was classified into degenerated cartilage (DC) group, and the cartilage extracted from the relatively smooth region was classified into preserved cartilage (PC) group. About 0.5–1 g DC and PC samples were collected from each donor respectively. All specimens were stored at −80°C with 1 ml Trizol (Invitrogen, United States) after grinding and homogenizing with liquid nitrogen. TRIzol™ Plus RNA Purification Kit was used for RNA extraction. Briefly, homogenized tissues were followed by phase separation, RNA precipitation, RNA wash, and RNA redissolving according to experimental protocol. During precipitation step, a 1/10 volume of 3M Sodium acetate (Cat No. AM9740, Invitrogen, United States) was added additionally to help RNA precipitation.
Quantitative Real-Time Polymerase Chain Reaction
The cDNA was synthesized from total RNA by using PrimeScript RT Master Mix (Perfect Real Time) Kit (Takara, Japan). Quantitative real-time PCR (qRT-PCR) was performed in triplicate on a QuantStudio™ 7 Flex Real-Time PCR System (Life Technologies QuantStudio 7 Real Time PCR System, RRID: SCR_020245, United States) by using TB Green Premix Ex Taq II (Tli RNase H Plus) Kit (Takara, Japan). The primers (5′-3′) were ordered from Tech Dragon Ltd. (Hong Kong) and listed in Table 1 The relative expression of each gene was normalized to GAPDH and presented in heatmap after normalization (log10 transformation).
Statistical Analysis
All data were analyzed using SPSS Statistics 23.0 software (IBM SPSS Statistics, RRID: SCR_019096, Chicago, United States). Two groups (PC and DC) with paired data were assessed by the paired sample t-test. A P-value less than 0.05 (p < 0.05) was considered statistically significant and P-values were presented numerically.
Results
Identification of DEGs in Human Knee OA Joint Tissues
For GSE43923 dataset, a total of 542 genes were identified by GEO2R analysis, of which 466 were upregulated and 76 were downregulated. For GSE12021 dataset, a total of 807 genes were identified by GEO2R analysis, of which 122 were upregulated and 685 were downregulated. For GSE51588, a total of 2,584 genes were identified by GEO2R analysis, of which 1715 were upregulated and 869 were downregulated. For GSE98918, a total of 412 genes were identified by GEO2R analysis, of which 144 were upregulated and 268 were downregulated. The distribution of gene expression for each dataset was visualized in the corresponding volcano plot (Figures 1A–D).
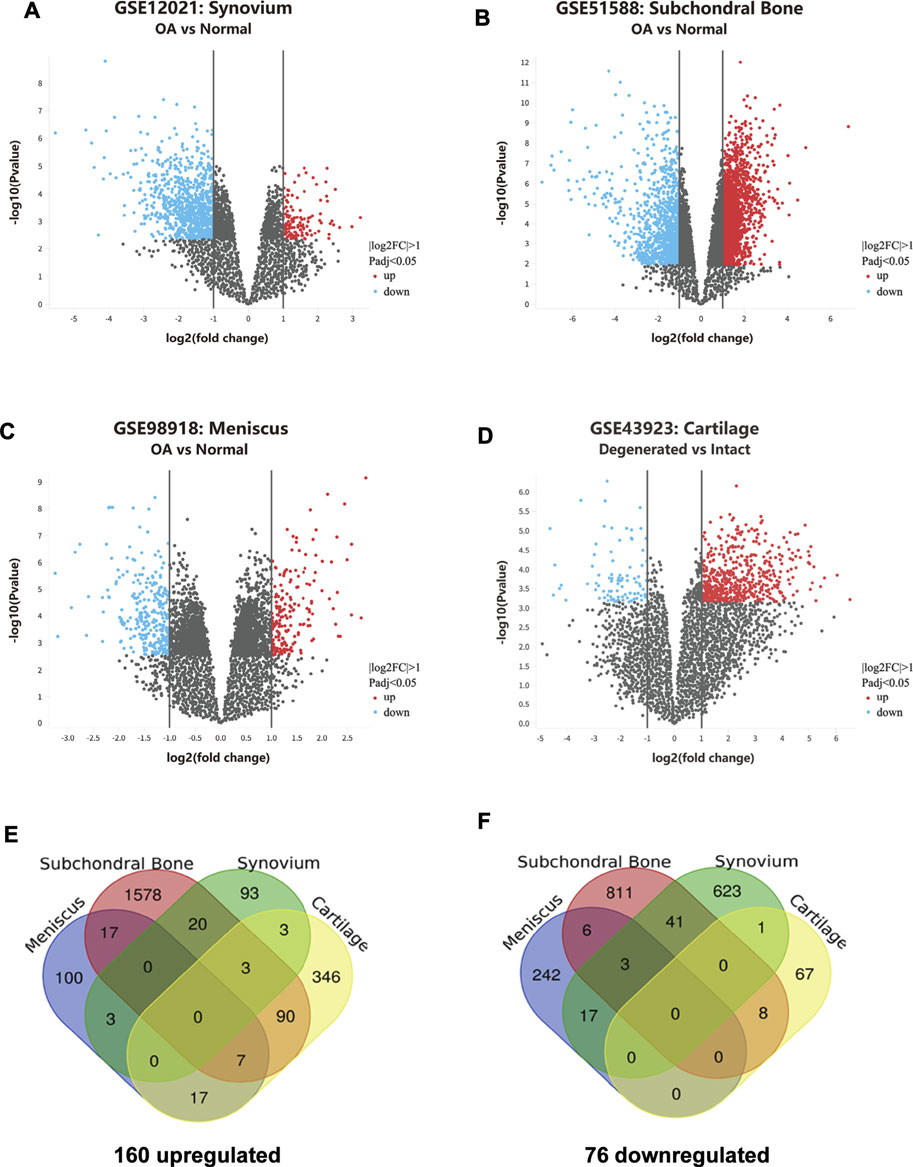
FIGURE 1. Identification of overlapping DEGs in knee OA. (A–D) Gene expression profiles of GSE12021, GSE51588, GSE98918, and GSE43923 are visualized in volcano plots respectively. DEGs are marked with red and the criteria for a DEG are |log2FC|>1 and adjusted P-value<0.05. (E) Upregulated DEGs in osteoarthritic cartilage, synovium, subchondral bone, and meniscus. (F) Downregulated DEGs in osteoarthritic cartilage, synovium, subchondral bone, and meniscus.
Identification of Overlapping DEGs in Human Knee OA Joint Tissues
As shown in the Venn Diagrams, 236 overlapping DEGs were identified, of which 160 were upregulated (Figure 1E) and 76 were downregulated (Figure 1F). No overlapping DEG was found in all the OA cartilage, synovial membrane, subchondral bone, and meniscus tissues. Those genes that appeared the most (at least three times) were identified as the most overlapping DEGs and listed in Table 2. A total of 13 most overlapping DEGs were identified. Among them, AHR, HEY1, CXCL12, MMP9, OLFML2A, SLITRK6, RHBDL2 were highly expressed in cartilage, meniscus, and subchondral bone. COL8A1, GAP43, and PTN were highly expressed in cartilage, synovial membrane, and subchondral bone. In addition, RUNX1, ARL4C, and PIM1 were lower expressed in the meniscus, synovial membrane, and subchondral bone.
GO Enrichment Analysis
The GO enrichment analysis results were presented in Figure 3. For upregulated overlapping DEGs, the most enriched GO Molecular Functions were identified as “proteoglycan binding,” “extracellular matrix structural constituent,” “lipid binding,” “collagen binding,” and “Wnt-protein binding” (Figure 2A). The most enriched GO Biological Processes mainly included “blood vessel development,” “ossification,” “cell morphogenesis involved in differentiation,” “cellular response to growth factor stimulus,” “response to mechanical stimulus” and “extracellular structure organization.” In addition, the most enriched GO Cellular Components were “extracellular matrix,” “cell-cell junction,” “dystrophin-associated glycoprotein complex,” “filopodium,” and “distal axon,” etc. For downregulated overlapping DEGs, the most enriched GO Molecular Functions mainly included “glucose transmembrane transporter activity,” “protein homodimerization activity,” “signaling adaptor activity,” “transcription factor binding,” and “cytokine activity” (Figure 2B). The GO Biological Processes were enriched in “activation of protein kinase activity,” “glucose transmembrane transport,” “cellular response to leptin stimulus,” “SMAD protein signal transduction,” “response to interleukin-6,” and “response to toxic substance.” In addition, the most enriched GO Cellular Components were identified as “secretory granule lumen,” “apical plasma membrane,” “specific granule,” “adherent junction,” and “perinuclear region of cytoplasm.”
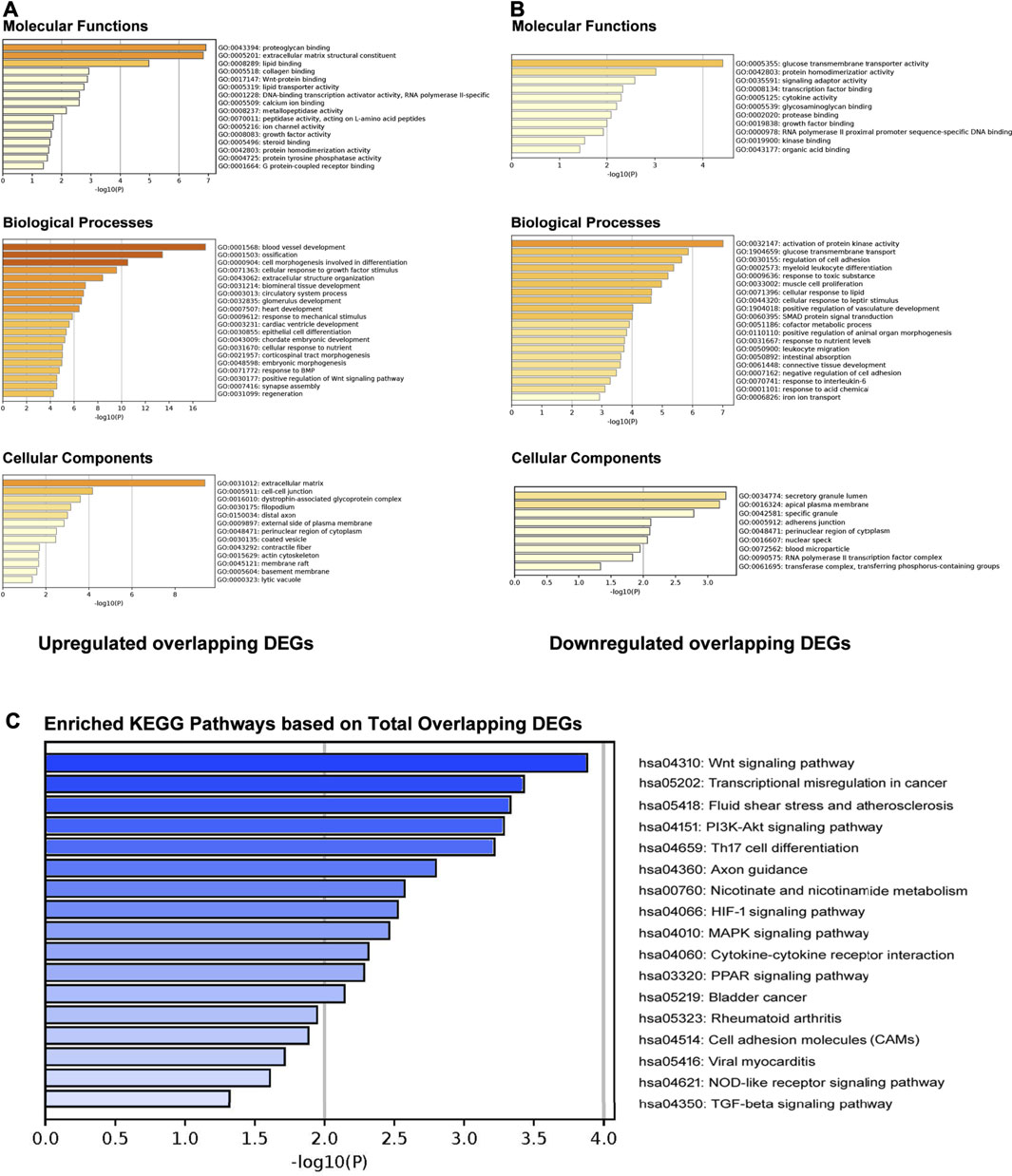
FIGURE 2. GO enrichment and KEGG pathway analyses. (A) GO molecular functions, biological processes, and cellular components enrichment analysis on upregulated overlapping DEGs. (B) GO molecular functions, biological processes, and cellular components enrichment analysis on downregulated overlapping DEGs. (C) Enriched KEGG pathways based on total overlapping DEGs.
KEGG Pathway Analysis
KEGG pathway analysis by Metascape indicated that total overlapping DEGs were enriched in 17 pathways including “Wnt signaling pathway,” “Fluid shear stress,” “Axon guidance,” “Nicotinate and nicotinamide metabolism,” “PI3K-Akt signaling pathway,” “HIF-1 signaling pathway,” “MAPK signaling pathway,” “Cytokine-cytokine receptor interaction,” “PPAR signaling pathway,” “NOD-like receptor signaling pathway,” and “TGF-beta signaling pathway,” etc. (Figure 2C). Enriched genes locating in corresponding pathways were summarized in Table 3. MYC, VEGFA, IL2RB, MAPK14, IL6R, MMP9, HLA-DQB1, and CXCL12 were most enriched in these identified KEGG pathways.
Construction of the PPI Network
A total of 168 interactions were obtained with interaction scores>0.4 by using STRING database. The PPI network was then constructed and presented at Cytoscape platform (Figure 3). In addition, 25 hub genes were obtained and presented in Table 4. The top 10 hub genes included VEGFA, MYC, MMP9, RUNX2, PTPRC, CXCL12, COL1A1, MAPK14, PECAM1, and CD34.
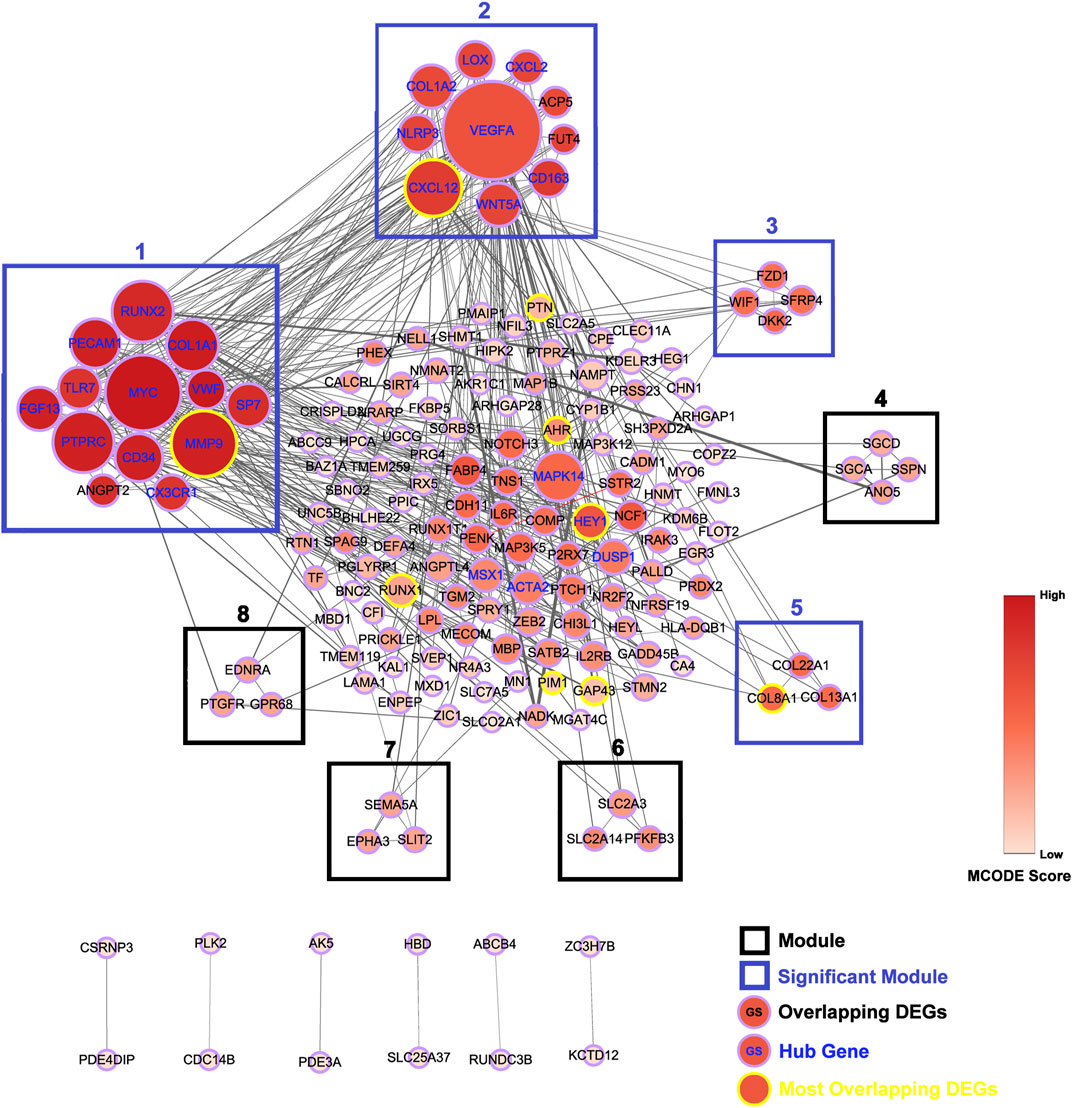
FIGURE 3. Construction of protein-protein interaction (PPI) network. PPI network for all the overlapping DEGs is constructed and followed by module analysis on Cytoscape platform. Genes with yellow border are most overlapping DEGs and genes with blue gene symbol are hub genes. Modules with blue border are significant modules. The size of circles reflects the degree of connectivity. The shades of color reflect MCODE score.
Module Analysis
A total of eight modules and four significant modules (Module 1, 2, 3, and 5) were obtained through MCODE analysis (Figure 3). Among significant modules, Module 1 included a total of 13 genes, of which 11 were upregulated and two were downregulated. GO Enrichment analysis showed that Module one was enriched in nine functions such as “stem cell proliferation,” “response to growth factor,” and “response to mechanical stimulus,” etc. For pathway analysis, Module one was significantly enriched in pathways such as “PI3K-Akt signaling pathway” and “Cell adhesion molecules (CAMs)” (Figure 4A). Module two was composited of 10 genes, of which five were upregulated and five were downregulated. Module two was enriched in six functions such as “regulation of interleukin-1 beta production,” “response to chemokine,” “anatomical structure homeostasis,” etc. In addition, “Rheumatoid arthritis” and “Cytokine-cytokine receptor interaction” pathways were enriched by genes within Module 2 (Figure 4B). Module three included four genes and all of them were upregulated. Enrichment analysis showed that Module three was enriched in “Wnt-protein binding” and “Wnt signaling pathway” (Figure 4C). Module five included three genes, all of them were upregulated. Enrichment analysis suggested that Module five was enriched in “collagen trimer” pathway (Figure 4D).
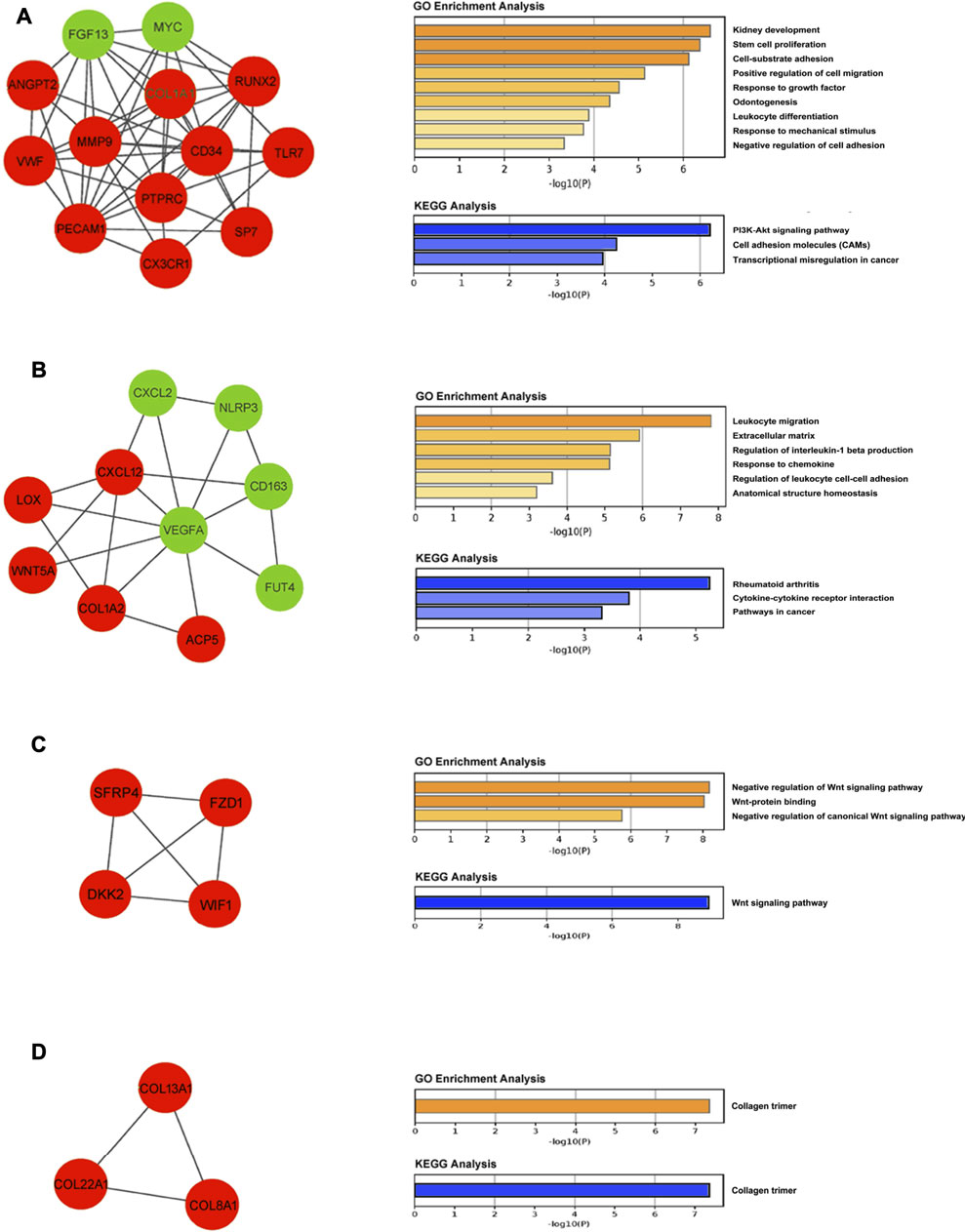
FIGURE 4. Module analysis. (A) Module one is comprised of 13 genes and enriched in PI3K-Akt signaling pathway and cell adhesion molecules (CAMs). (B) Module two is comprised of 10 genes and enriched in rheumatoid arthritis pathway and cytokine-cytokine receptor interaction pathway. (C) Module three is comprised of four genes and enriched in the Wnt signaling pathway. (D) Module five is comprised of three genes and enriched in collagen trimer pathway. Upregulated and downregulated genes are stained with red and green, respectively.
Construction of the miRNA-mRNA Network
The above identified most overlapping DEGs and hub genes were regarded as knee OA-related key genes. By constructing the PPI network of these key genes, we then screened out the experimentally validated key gene-related miRNAs targeting at above OA-related key genes by using miRTarBase (Table 5). As a result, a total of 57 key miRNAs were obtained and visualized in Figure 5. The top 10 key miRNAs included miR-29, miR-101, miR-17, miR-181a, miR-124, miR-1, miR-9, miR-21, miR-155, and miR-185. Key miRNAs were further compared with OA-related miRNAs that were obtained from the Human microRNA Disease Database (HMDD v3.2, http://www.cuilab.cn/hmdd) to check the reliability of our analyses and screen out miRNAs that lack researches so far (Huang et al., 2019).
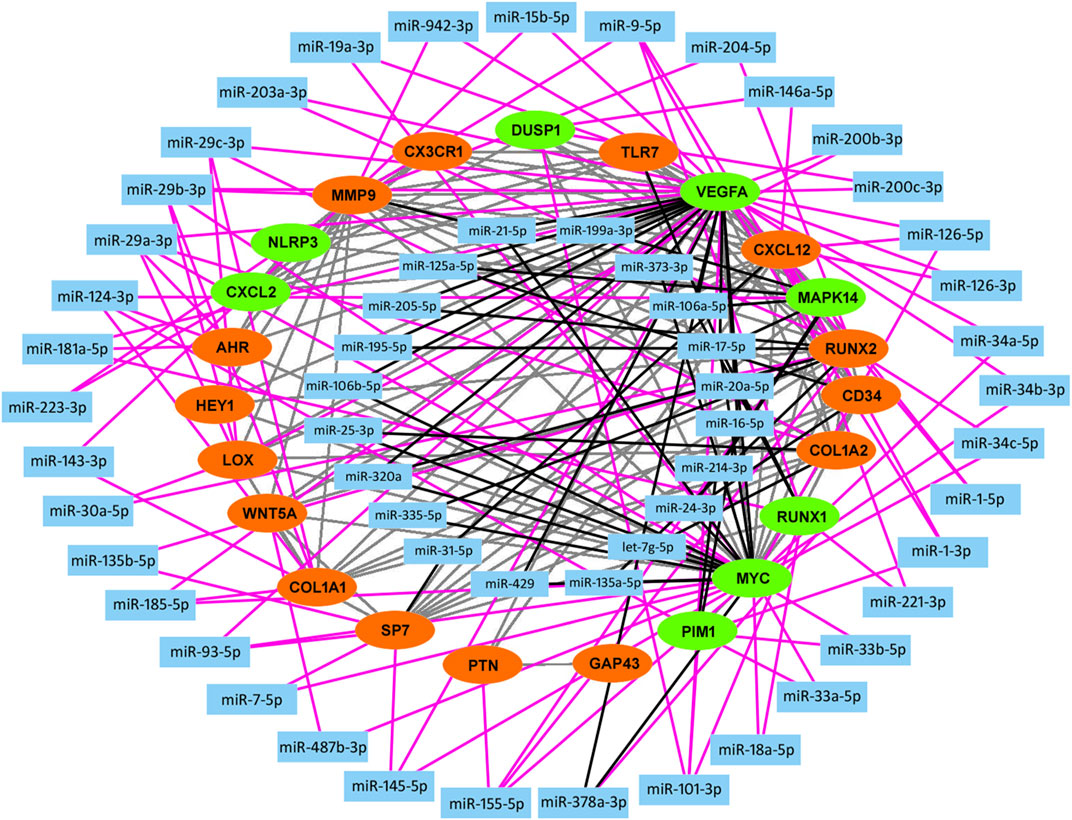
FIGURE 5. Construction of miRNA-mRNA network. A total of 23 knee OA-related key genes (red, upregulated; green, downregulated) are used in construction of miRNA-mRNA network backbone. Key miRNAs are then screened out by miRTarBase database and visualized in Cytoscape. Grey line, protein-protein interaction; Black line, interaction between key gene and key miRNA which belongs to inner circle. Pink line, interaction between key gene and key miRNA which belongs to outside circle.
Clinical Validation
Quantitatively Real-time PCR assay (qRT-PCR) was performed to evaluate the relative expression of putative knee OA-related key genes in osteoarthritic cartilage specimens. As a result, significantly upregulated AHR, CYP1A1, and HEY1 were observed in degenerated cartilage compared to the intact cartilage. Besides, MYC and CXCL12 were also upregulated, while no significant difference was observed (Figures 6A–F).
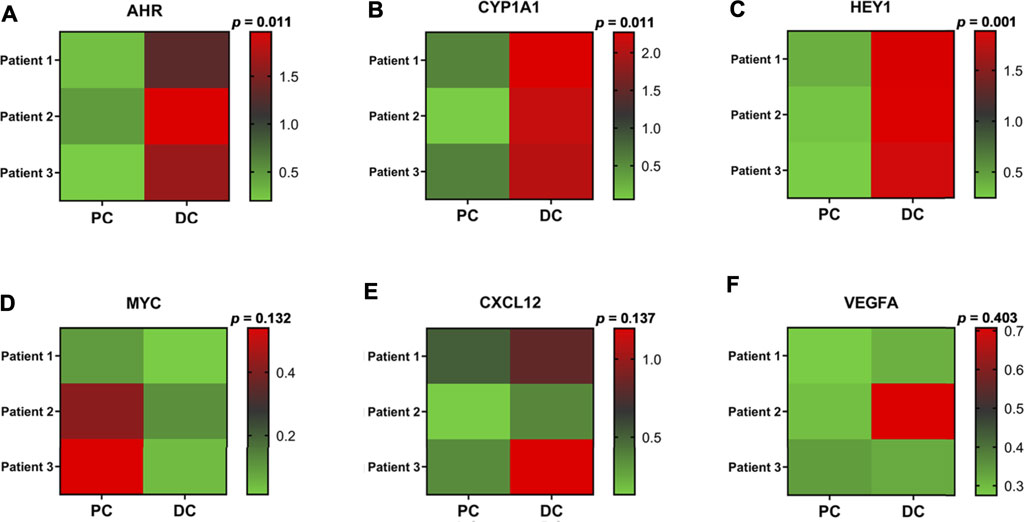
FIGURE 6. Clinical validation. (A–F) Heat maps of the relative expression of several identified knee OA-related key genes in osteoarthritic cartilage derived from knee OA patients. p, P-value; PC, preserved cartilage; DC, degenerated cartilage.
Discussion
The present study aims to screen out key DEGs, their relevant signaling pathways, and interactive miRNAs in human knee OA based on bioinformatic analysis. The gene expression profiles derived from different joint tissues are covered and followed by identifying overlapping DEGs. To the best of our knowledge, this is the first time that overlapping DEGs in knee OA are identified from four different OA joint tissues, including articular cartilage, synovial membrane, subchondral bone, and meniscus. Gene expression profiles are all obtained from GEO database.
After identification of DEGs in different joint tissues, overlapping DEGs are then identified via Venn Diagram. As a result, 236 overlapping DEGs are identified, of which 160 are upregulated and 76 are downregulated. Those DEGs that are differentially expressed in at least three joint tissues are identified as the most overlapping DEGs. As a result, a total of 13 most overlapping DEGs are identified in our study, include AHR, HEY1, CXCL12, MMP9, COL8A1, GAP43, PTN, RUNX1, PIM1, OLFML2A, SLITRK6, RHBDL2, and ARL4C, while no overlapping genes are differentially expressed in all four components. Among above identified most overlapping DEGs, OLFML2A, SLITRK6, RHBDL2, and ARL4C are excluded from our constructed PPI network, suggesting their relatively limited values and will not be discussed in the following context.
MMP9 is an enzyme implicated in the cartilage destruction and has been identified as a hallmark of OA previously, the same as other MMPs like MMP1, MMP3 and MMP13. Previous studies have reported the upregulation of MMP9 in OA cartilage, synovial membrane, subchondral bone, and synovial fluid (Yang et al., 2010; Kim et al., 2014). COL8A1, which maintains cartilage stability through participating in collagen synthesis, is also reported to be highly expressed in OA cartilage (Fang et al., 2019). CXCL12, also known as SDF-1, is a chemokine that plays important role in angiogenesis, bone metabolism, cartilage homeostasis, and pro-inflammatory responses (De Klerck et al., 2005; Garcia-Cuesta et al., 2019). Upregulation of CXCL12 in OA cartilage, subchondral bone, synoviocytes, and synovial fluid has been reported by previous studies (Chen et al., 2017b; Bragg et al., 2019). Furthermore, inhibition of CXCL12/CXCR4 signaling was shown to prevent subchondral bone loss and significantly attenuate cartilage degradation (Dong et al., 2016; Chen et al., 2017b). GAP43 is a nervous tissue-specific cytoplasmic protein related to nerve regeneration. Previous study showed that the expression of GAP43 in pain-related sensory innervation of dorsal-root ganglia (DRG) was upregulated during OA progression (Kawarai et al., 2018). Thus, the upregulation of GAP43 in joint tissues may reflect joint sensory innervation, which is closed related to nociceptive sense and osteophyte formation (Wu et al., 2002; Orita et al., 2011). Pleiotrophin (PTN) is an 18-kDa heparin-binding neurite outgrowth-promoting growth factor. Previous studies have reported the upregulation of PTN in OA cartilage, synovial membrane, and synovial fluid. Notably, PTN is initially abundant in fetal or juvenile cartilage and then becomes absent in mature cartilage. During early stages of OA, PTN becomes re-expressed again (Pufe et al., 2003; Mentlein 2007). Besides, PTN is also proven to facilitate chondrocyte proliferation (Pufe et al., 2007). Interestingly, GAP43 and PTN are two nerve growth-related genes, suggesting the important role of nerve innervation and axon guidance during OA development in the entire joint. Their specific role in OA development needs to be further investigated. PIM1 is an enzyme that play important roles in cell cycle progression, apoptosis, and transcriptional activation (Bachmann and Moroy, 2005). However, there is no study reporting their relationships so far.
The rest of most overlapping DEGs, including HEY1, AHR, RUNX1, and HEY1, are all transcription factors. HEY1 is a basic helix-loop-helix protein (bHLH) transcription factor that belongs to HES/HEY family. Besides, HEY1 is also a direct target of canonical Notch signaling. Previous studies indicated that inhibition of Notch1 exacerbated experimental OA, while increased levels or activity of Notch2 contributed to the progression of OA (Hosaka et al., 2013; Lin et al., 2016). In addition, HEY1 is not only transcriptionally induced by Notch ligands, but also induced by BMP/TGF-β axis to exert as a transcription repressor. Its functions on repressing osteogenic differentiation, neuronal differentiation, and pro-inflammatory production have been reported before (Weber et al., 2014). Hes1, another transcription factor that belongs to HES/HEY family like HEY1, has been reported to be upregulated in OA cartilage and accelerate cartilage destruction via promoting MMP3, a disintegrin and metalloproteinase with thrombospondin motifs 5 (ADAMTS5), and interleukin-6 (IL-6) transcription (Sugita et al., 2015). Nonetheless, the specific role of HEY1 in OA progression remain to be investigated. AHR is a bHLH transcription factor that plays important role in development, immune system, and toxic response. Previous studies have demonstrated that AHR signaling activation significantly alleviated progression of rheumatoid arthritis (RA) through repressing C-reactive protein (CRP), NLR family pyrin domain containing 3 (NLRP3), tumor necrosis factor-alpha (TNF-α), and IL-6 expression (Jin et al., 2011; Huai et al., 2014; Liang et al., 2019; Piper et al., 2019), and enhancing nuclear factor erythroid 2-related factor 2 (NRF2) and IL-10 expression in B cells, macrophages, or hepatocytes (Tsuji et al., 2012; Piper et al., 2019; Rosser et al., 2020). However, several reports also indicated that AHR signaling activation exacerbated RA inflammation through activating cytokine-mediated inflammatory signaling in primary human fibroblast-like synoviocytes (Adachi et al., 2013; Lahoti et al., 2013). Ogando J et al. reported that the AHR signaling pathway was significantly more active in OA synovial tissues than in RA synovial tissues (Ogando et al., 2016). Furthermore, compared to resting chondrocytes, significantly upregulated AHR was also observed in hypertrophic chondrocytes (Cedervall et al., 2015). Nevertheless, the specific effects of AHR on OA are still in debate and remain to be investigated. RUNX1 was reported to be significantly downregulated in OA cartilage compared with normal control. Furthermore, its anabolic effect on chondrocytes contributes to the maintenance of cartilage homeostasis during OA development and that has also been recognized as a disease-modifying target of OA (Yano et al., 2013; Aini et al., 2016; Yano et al., 2019). In addition, the anti-angiogenic effect of RUNX1 through repressing vascular endothelial growth factor (VEGF) expression has also been reported (Ter Elst et al., 2011).
According to KEGG pathway analysis based on overlapping DEGs, we observe that overlapping DEGs are enriched in “PI3K-Akt signaling pathway,” “Wnt signaling pathway,” “Fluid shear stress,” “Nicotinate and nicotinamide metabolism,” “HIF-1 signaling pathway,” “MAPK signaling pathway,” “Cytokine-cytokine receptor interaction,” “PPAR signaling pathway,” “NOD-like receptor signaling pathway,” “Axon guidance,” and “TGF-β signaling pathway.” The above pathways are well consistent with existing research findings. For example, PI3K-Akt signaling and MAPK signaling are closely involved in inflammatory response to induce the production of catabolic markers such as MMPs, Adamts, IL-1β, and TNF-α in OA (Herrero-Beaumont et al., 2019; Chow and Chin, 2020). Nicotinate and nicotinamide metabolism play important roles in redox reaction. So far, several studies have reported the important role of nicotinate and nicotinamide metabolism in OA development (Yang et al., 2015; Junker et al., 2017). In 2015, Yang et al. (2015) demonstrated that nicotinamide phosphoribosyltransferase (NAMPT), a rate-limiting enzyme in the Nicotinamide adenine dinucleotide (NAD+) salvage pathway, acted as a crucial catabolic regulator of osteoarthritic cartilage destruction. In addition, aberrantly activated Wnt signaling or TGF-β signaling contributes to cartilage degradation, osteophyte formation, and formation of subchondral bone marrow osteoid islets (Zhen et al., 2013; van der Kraan 2017). Therapies targeting Wnt signaling have been undergoing clinical trials (ClinicalTrials.gov Identifier: NCT03928184). HIF-1α and HIF-2α were also reported to exert anabolic and catabolic effects on chondrocytes, respectively (Zhang et al., 2015). PPAR signaling, in particular PPARγ, which is significantly downregulated in OA cartilage, has been demonstrated to maintain articular cartilage homeostasis via regulating the mTOR pathway (Vasheghani et al., 2015; Zhu et al., 2019). NOD-like receptor signaling, which mediates innate immunity and participates in regulating inflammatory and apoptotic responses, mainly includes two subfamilies, NODs and NLRPs (Platnich and Muruve, 2019). Both NOD-dependent pathway and NLRP-dependent inflammasome pathway were validated to mediate OA development under external stimulus (Jin et al., 2011; Xu et al., 2015). Fluid shear stress (FSS) was known as one of the pathogenic mechanisms of OA and has also been used in the construction of an osteoarthritic cell model for a long time (Yang et al., 2020). In addition to above well-validated OA related-pathways, axon guidance pathway is lack of research up to now. Only several in vitro studies reported the effects of an axon guidance molecule, Semaphorin 3A (Sema3A), on osteoarthritic chondrocytes (Okubo et al., 2011; Sumi et al., 2018).
Through constructing the PPI network and miRNA-mRNA network in Cytoscape, a total of 25 hub genes, four significant modules, and 57 key miRNAs are identified. Among hub genes, MAPK14, IL2RB, and IL6R are all involved in cytokine-mediated inflammatory response during OA (Boileau et al., 2005, Liang et al., 2018, Shkhyan et al., 2018). A genome-wide association (GWAS) study has identified a single nucleotide polymorphism (SNP) of HLA-DQB1, rs7775228, associating with knee OA in Asian population (Valdes and Spector, 2011). Furthermore, a small molecule gp130 modulator (RCGD 423) was proven to improve chondrocyte proliferation and inhibit cartilage degradation via upregulating transcription factor MYC and suppressing IL-6-mediated inflammatory response (Shkhyan et al., 2018). For growth factor VEGFA, it is closely related to angiogenesis and inflammatory response (Gao et al., 2013). To validate above computational results, we performed qRT-PCR to validate the relative expression of identified key genes in OA cartilage clinically. Our results show that AHR and its downstream target CYP1A1, HEY1, MYC, and CXCL12 are indeed upregulated in degenerated cartilage compared to preserved cartilage (Figure 6).
According to module analysis, majority of hub genes are located in module one and module 2. In addition, MMP9, CXCL12, and HEY1 are not only the most overlapping DEGs, but also hub genes. Module analysis shows that Modules 1, 2, 3, and 5 are significant modules with the MCODE score ≥4. For Module 1, all of the genes within Module 1, except ANGPT2, are hub genes. MMP9 is located in Module 1. Enrichment analysis suggests that Module one plays important role in PI3K-Akt signaling pathway and cell adhesion molecules (CAMs) pathway. Regarding genes within Module 2, all of them are hub genes except ACP5 and FUT4. Genes in Module two play important roles in rheumatoid arthritis and cytokine-cytokine receptor interaction pathways. Furthermore, CXCL12 is located in Module 2. Module three is a group of genes associated with Wnt signaling pathway. No most overlapping DEGs or hub genes locate in Module 3. Module five includes COL8A1, COL13A1, and COL22A1, all of which are associated with collagen trimer pathway and have been demonstrated to be aberrantly expressed in degraded cartilage (Karlsson et al., 2010; Feng et al., 2019). A recent study reported that Lgr5+/Col22a1+ stem cells play important roles in differentiation toward articular chondrocytes and Col22a1-expressing cartilage superficial layer contributed to repair of cartilage defect (Feng et al., 2019).
Collectively, based on above identified overlapping DEGs, hub genes, pathways, and functional modules, the present study depicted the potential OA mechanisms covering the entire knee joint. We believe that our identified knee OA-related key genes, such as AHR, HEY1, MYC, GAP43, and PTN, and their relevant signaling pathways, including AHR signaling, Notch signaling and TGF-β signaling, C-MYC signaling, and axon guidance pathway may play important roles in knee OA development. Our predicted genes are worthy of being explored as novel targets of DMOADs in the future.
By comparing key miRNAs with OA-related miRNAs included in HMDD v3.2 database, 41 out of 57 miRNAs (about 71.9%) have been reported to be associated with OA, suggesting the considerable reliability of our miRNA-mRNA interactome predictions. For example, miR-29 family, including miR-29a, miR-29b, and miR-29c, was differentially expressed in OA cartilage and negatively regulated Smad, NF-κB, and canonical Wnt signaling (Le et al., 2016). In addition, upregulation of miR-101 significantly facilitated cartilage degradation and chondrocyte apoptosis (Dai et al., 2015; Lü et al., 2020). For miR-181a, Akihiro Nakamura et al. demonstrated that intra-articular injections of locked nucleic acid (LNA) miR-181a antisense oligonucleotides (ASO) significantly attenuated cartilage destruction in facet and knee joints in vivo (Nakamura et al., 2019). Another study reported that miR-9 promoted IL-6 expression and exacerbated cartilage degradation by targeting MCPIP1 expression (Makki et al., 2015). In 2019, a hydrogel-based drug delivery system equipped with locked nucleic acid (LNA) miR-221 inhibitor was constructed and enhanced cartilage regeneration significantly (Lolli et al., 2019). miR-199a* was also shown to inhibit IL-1β-induced Cyclooxygenase 2 (COX-2) expression as a direct regulator (Akhtar and Haqqi, 2012). Notably, a RNA sequencing-based miRNA-mRNA interactome study which confirmed a OA-specific miRNAs array showed considerable overlap with our identified key miRNAs as well, including miR-143, miR-155, let-7g, miR-7, miR-15, miR-101, miR-21, miR-19a, miR-16, miR-30a, miR-29, miR-1, miR-133a-3p, miR-20a, miR-320a, miR-31, miR-93, miR-221, and miR-335 (Coutinho de Almeida et al., 2019). Among our identified top 10 key miRNAs, in addition to those that have been reported before, miR-17, miR-21, miR-155, miR-185, and miR-1 are still lack of research and remain to be investigated in the future.
The present study also has several limitations. For example, although we originally intended to include as many data sets as possible, only four expression profiles met the criteria and were included into the data analysis. In addition, due to difficulties in obtaining appropriate specimens, only OA articular cartilage specimens were used to carry out clinical validation experiments. The relative expression of our identified knee OA-related key genes in other joint components need to be further validated.
In conclusion, the present study provides a comprehensive bioinformatics analysis of key genes, signaling pathways, and miRNAs in different joint tissues of knee OA patients. A total of 35 knee OA-related key genes and 57 key miRNAs were identified. Among them, key genes such as AHR, HEY1, MYC, GAP43, and PTN, and key miRNAs such as miR-17, miR-21, miR-155, miR-185, and miR-1 are lack of research so far. For key genes identified in the present study, their downstream mechanisms and specific effects on the different joint components also need to be explored, respectively. Through enrichment analysis, a number of OA-related pathways were identified, including PI3K-Akt signaling, Wnt signaling, fluid shear stress, nicotinate and nicotinamide metabolism, HIF-1 signaling, MAPK signaling, cytokine-cytokine receptor interaction, PPAR signaling, NOD-like receptor signaling, TGF-β signaling, and axon guidance pathways. Among them, many pathways have been well investigated and even under clinical trials, except for axon guidance pathway which is implicated in nerve innervation and axon guidance while still lack of research so far. Our study provides insight for the first time in identification of potential therapeutic targets of knee OA by comprehensively analyzing the overlapping genes differentially expressed in multiple joint components based on bioinformatics.
Data Availability Statement
The original contributions presented in the study are included in the article/supplementary material, further inquiries can be directed to the corresponding authors.
Ethics Statement
For clinical specimens sampling, all donors have provided informed consent, and this study was approved by the Joint Chinese University of Hong Kong-New Territories East Cluster Clinical Research Ethics Committee (CREC Ref. No: 2013.248).
Author Contributions
LQ, JX, and LC designed the study; LC carried out the experiments; HY, ZY, WT, and BD helped performed the experiments, KH, and MO helped collected clinical specimens; LC performed computational analysis and analyzed the data; LC wrote the manuscript; JX and LQ supervised the project and helped amend the manuscript. All authors read and approved the final manuscript.
Funding
This work was fully supported by Theme-based Research Scheme from RGC-Hong Kong (No. T13-402/17N) and partially supported by Health and Medical Research Fund (18190481).
Conflict of Interest
The authors declare that the research was conducted in the absence of any commercial or financial relationships that could be construed as a potential conflict of interest.
Publisher’s Note
All claims expressed in this article are solely those of the authors and do not necessarily represent those of their affiliated organizations, or those of the publisher, the editors and the reviewers. Any product that may be evaluated in this article, or claim that may be made by its manufacturer, is not guaranteed or endorsed by the publisher.
Acknowledgments
The authors would like to thank the support of Tech Dragon Ltd. (Hong Kong, China) for primers preparation. Furthermore, we would like to appreciate all reviewers for their insightful comments and constructive suggestions to polish this paper in high quality.
References
Adachi, M., Okamoto, S., Chujyo, S., Arakawa, T., Yokoyama, M., Yamada, K., et al. (2013). Cigarette Smoke Condensate Extracts Induce IL-1-Beta Production From Rheumatoid Arthritis Patient-Derived Synoviocytes, but Not Osteoarthritis Patient-Derived Synoviocytes, Through Aryl Hydrocarbon Receptor-Dependent NF-Kappa-B Activation and Novel NF-Kappa-B Sites. J. Interferon Cytokine Res. 33 (6), 297–307. doi:10.1089/jir.2012.0107
Aini, H., Itaka, K., Fujisawa, A., Uchida, H., Uchida, S., Fukushima, S., et al. (2016). Messenger RNA Delivery of a Cartilage-Anabolic Transcription Factor as a Disease-Modifying Strategy for Osteoarthritis Treatment. Sci. Rep. 6, 18743. doi:10.1038/srep18743
Akhtar, N., and Haqqi, T. M. (2012). MicroRNA-199a* Regulates the Expression of Cyclooxygenase-2 in Human Chondrocytes. Ann. Rheum. Dis. 71 (6), 1073–1080. doi:10.1136/annrheumdis-2011-200519
Bachmann, M., and Möröy, T. (2005). The Serine/threonine Kinase Pim-1. Int. J. Biochem. Cel Biol. 37 (4), 726–730. doi:10.1016/j.biocel.2004.11.005
Boileau, C., Pelletier, J. P., Tardif, G., Fahmi, H., Laufer, S., Lavigne, M., et al. (2005). The Regulation of Human MMP-13 by Licofelone, an Inhibitor of Cyclo-Oxygenases and 5-lipoxygenase, in Human Osteoarthritic Chondrocytes Is Mediated by the Inhibition of the P38 MAP Kinase Signalling Pathway. Ann. Rheum. Dis. 64 (6), 891–898. doi:10.1136/ard.2004.026906
Bragg, R., Gilbert, W., Elmansi, A. M., Isales, C. M., Hamrick, M. W., Hill, W. D., et al. (2019). Stromal Cell-Derived Factor-1 as a Potential Therapeutic Target for Osteoarthritis and Rheumatoid Arthritis. Ther. Adv. Chronic Dis. 10, 2040622319882531. doi:10.1177/2040622319882531
Brophy, R. H., Zhang, B., Cai, L., Wright, R. W., Sandell, L. J., and Rai, M. F. (2018). Transcriptome Comparison of Meniscus from Patients With and Without Osteoarthritis. Osteoarthritis Cartilage. 26 (3), 422–432. doi:10.1016/j.joca.2017.12.004
Cedervall, T., Lind, P. M., and Sävendahl, L. (2015). Expression of the Aryl Hydrocarbon Receptor in Growth Plate Cartilage and the Impact of its Local Modulation on Longitudinal Bone Growth. Int. J. Mol. Sci. 16 (4), 8059–8069. doi:10.3390/ijms16048059
Chang, L., Liu, A., Xu, J., Xu, X., Dai, J., Wu, R., et al. (2021). TDP-43 Maintains Chondrocyte Homeostasis and Alleviates Cartilage Degradation in Osteoarthritis. Osteoarthritis Cartilage. 29 (7), 1036–1047. doi:10.1016/j.joca.2021.03.015
Chen, D., Shen, J., Zhao, W., Wang, T., Han, L., Hamilton, J. L., et al. (2017a). Osteoarthritis: Toward a Comprehensive Understanding of Pathological Mechanism. Bone Res. 5, 16044. doi:10.1038/boneres.2016.44
Chen, Y., Lin, S., Sun, Y., Guo, J., Lu, Y., Suen, C. W., et al. (2017b). Attenuation of Subchondral Bone Abnormal Changes in Osteoarthritis by Inhibition of SDF-1 Signaling. Osteoarthritis Cartilage. 25 (6), 986–994. doi:10.1016/j.joca.2017.01.008
Choi, W. S., Yang, J. I., Kim, W., Kim, H. E., Kim, S. K., Won, Y., et al. (2019). Critical Role for Arginase II in Osteoarthritis Pathogenesis. Ann. Rheum. Dis. 78 (3), 421–428. doi:10.1136/annrheumdis-2018-214282
Chou, C. H., Wu, C. C., Song, I. W., Chuang, H. P., Lu, L. S., Chang, J. H., et al. (2013). Genome-Wide Expression Profiles of Subchondral Bone in Osteoarthritis. Arthritis Res. Ther. 15 (R190), R190. doi:10.1186/ar4380
Chow, Y. Y., and Chin, K. Y. (2020). The Role of Inflammation in the Pathogenesis of Osteoarthritis. Mediators Inflamm. 2020, 8293921. doi:10.1155/2020/8293921
Coutinho de Almeida, R., Ramos, Y. F. M., Mahfouz, A., den Hollander, W., Lakenberg, N., Houtman, E., et al. (2019). RNA Sequencing Data Integration Reveals an miRNA Interactome of Osteoarthritis Cartilage. Ann. Rheum. Dis. 78 (2), 270–277. doi:10.1136/annrheumdis-2018-213882
Dai, L., Zhang, X., Hu, X., Liu, Q., Man, Z., Huang, H., et al. (2015). Silencing of miR-101 Prevents Cartilage Degradation by Regulating Extracellular Matrix-Related Genes in a Rat Model of Osteoarthritis. Mol. Ther. 23 (8), 1331–1340. doi:10.1038/mt.2015.61
De Klerck, B., Geboes, L., Hatse, S., Kelchtermans, H., Meyvis, Y., Vermeire, K., et al. (2005). Pro-Inflammatory Properties of Stromal Cell-Derived Factor-1 (CXCL12) in Collagen-Induced Arthritis. Arthritis Res. Ther. 7 (6), R1208–R1220. doi:10.1186/ar1806
Dong, Y., Liu, H., Zhang, X., Xu, F., Qin, L., Cheng, P., et al. (2016). Inhibition of SDF-1α/CXCR4 Signalling in Subchondral Bone Attenuates Post-Traumatic Osteoarthritis. Int. J. Mol. Sci. 17 (6), 943. doi:10.3390/ijms17060943
Fang, Y., Wang, P., Xia, L., Bai, S., Shen, Y., Li, Q., et al. (2019). Aberrantly Hydroxymethylated Differentially Expressed Genes and the Associated Protein Pathways in Osteoarthritis. PeerJ. 7, e6425. doi:10.7717/peerj.6425
Feng, C., Chan, W. C. W., Lam, Y., Wang, X., Chen, P., Niu, B., et al. (2019). Lgr5 and Col22a1 Mark Progenitor Cells in the Lineage Toward Juvenile Articular Chondrocytes. Stem Cel Rep. 13 (4), 713–729. doi:10.1016/j.stemcr.2019.08.006
Gao, W., Sweeney, C., Walsh, C., Rooney, P., McCormick, J., Veale, D. J., et al. (2013). Notch Signalling Pathways Mediate Synovial Angiogenesis in Response to Vascular Endothelial Growth Factor and Angiopoietin 2. Ann. Rheum. Dis. 72 (6), 1080–1088. doi:10.1136/annrheumdis-2012-201978
García-Cuesta, E. M., Santiago, C. A., Vallejo-Díaz, J., Juarranz, Y., Rodríguez-Frade, J. M., and Mellado, M. (2019). The Role of the CXCL12/CXCR4/ACKR3 Axis in Autoimmune Diseases. Front. Endocrinol. (Lausanne). 10, 585. doi:10.3389/fendo.2019.00585
Herrero-Beaumont, G., Pérez-Baos, S., Sánchez-Pernaute, O., Roman-Blas, J. A., Lamuedra, A., and Largo, R. (2019). Targeting Chronic Innate Inflammatory Pathways, the Main Road to Prevention of Osteoarthritis Progression. Biochem. Pharmacol. 165, 24–32. doi:10.1016/j.bcp.2019.02.030
Hosaka, Y., Saito, T., Sugita, S., Hikata, T., Kobayashi, H., Fukai, A., et al. (2013). Notch Signaling in Chondrocytes Modulates Endochondral Ossification and Osteoarthritis Development. Proc. Natl. Acad. Sci. U S A. 110 (5), 1875–1880. doi:10.1073/pnas.1207458110
Huai, W., Zhao, R., Song, H., Zhao, J., Zhang, L., Zhang, L., et al. (2014). Aryl Hydrocarbon Receptor Negatively Regulates NLRP3 Inflammasome Activity by Inhibiting NLRP3 Transcription. Nat. Commun. 5, 4738. doi:10.1038/ncomms5738
Huang, H. Y., Lin, Y. C., Li, J., Huang, K. Y., Shrestha, S., Hong, H. C., et al. (2020). miRTarBase 2020: Updates to the Experimentally Validated microRNA-Target Interaction Database. Nucleic Acids Res. 48 (D1), D148–D154. doi:10.1093/nar/gkz896
Huang, Z., Shi, J., Gao, Y., Cui, C., Zhang, S., Li, J., et al. (2019). HMDD v3.0: a Database for Experimentally Supported Human microRNA-Disease Associations. Nucleic Acids Res. 47 (D1), D1013–D1017. doi:10.1093/nar/gky1010
Huber, R., Hummert, C., Gausmann, U., Pohlers, D., Koczan, D., Guthke, R., et al. (2008). Identification of Intra-Group, Inter-Individual, and Gene-Specific Variances in mRNA Expression Profiles in the Rheumatoid Arthritis Synovial Membrane. Arthritis Res. Ther. 10 (4), R98. doi:10.1186/ar2485
Jin, C., Frayssinet, P., Pelker, R., Cwirka, D., Hu, B., Vignery, A., et al. (2011). NLRP3 Inflammasome Plays a Critical Role in the Pathogenesis of Hydroxyapatite-Associated Arthropathy. Proc. Natl. Acad. Sci. U S A. 108 (36), 14867–14872. doi:10.1073/pnas.1111101108
Junker, S., Frommer, K. W., Krumbholz, G., Tsiklauri, L., Gerstberger, R., Rehart, S., et al. (2017). Expression of Adipokines in Osteoarthritis Osteophytes and Their Effect on Osteoblasts. Matrix Biol. 62, 75–91. doi:10.1016/j.matbio.2016.11.005
Kang, Y. J., Yoo, J. I., and Baek, K. W. (2021). Differential Gene Expression Profile by RNA Sequencing Study of Elderly Osteoporotic Hip Fracture Patients with Sarcopenia. J. Orthop. Translat. 29, 10–18. doi:10.1016/j.jot.2021.04.009
Karlsson, C., Dehne, T., Lindahl, A., Brittberg, M., Pruss, A., Sittinger, M., et al. (2010). Genome-Wide Expression Profiling Reveals New Candidate Genes Associated with Osteoarthritis. Osteoarthritis Cartilage. 18 (4), 581–592. doi:10.1016/j.joca.2009.12.002
Kawarai, Y., Orita, S., Nakamura, J., Miyamoto, S., Suzuki, M., Inage, K., et al. (2018). Changes in Proinflammatory Cytokines, Neuropeptides, and Microglia in an Animal Model of Monosodium Iodoacetate-Induced Hip Osteoarthritis. J. Orthop. Res. 36 (11), 2978–2986. doi:10.1002/jor.24065
Kim, J. H., Jeon, J., Shin, M., Won, Y., Lee, M., Kwak, J. S., et al. (2014). Regulation of the Catabolic Cascade in Osteoarthritis by the Zinc-ZIP8-MTF1 Axis. Cell. 156 (4), 730–743. doi:10.1016/j.cell.2014.01.007
Klinger, P., Beyer, C., Ekici, A. B., Carl, H. D., Schett, G., Swoboda, B., et al. (2013). The Transient Chondrocyte Phenotype in Human Osteophytic Cartilage: A Role of Pigment Epithelium-Derived Factor? Cartilage. 4 (3), 249–255. doi:10.1177/1947603513480809
Kung, L. H. W., Ravi, V., Rowley, L., Angelucci, C., Fosang, A. J., Bell, K. M., et al. (2018). Cartilage MicroRNA Dysregulation during the Onset and Progression of Mouse Osteoarthritis Is Independent of Aggrecanolysis and Overlaps with Candidates From End-Stage Human Disease. Arthritis Rheumatol. 70 (3), 383–395. doi:10.1002/art.40378
Kuttapitiya, A., Assi, L., Laing, K., Hing, C., Mitchell, P., Whitley, G., et al. (2017). Microarray Analysis of Bone Marrow Lesions in Osteoarthritis Demonstrates Upregulation of Genes Implicated in Osteochondral Turnover, Neurogenesis and Inflammation. Ann. Rheum. Dis. 76 (10), 1764–1773. doi:10.1136/annrheumdis-2017-211396
Lahoti, T. S., John, K., Hughes, J. M., Kusnadi, A., Murray, I. A., Krishnegowda, G., et al. (2013). Aryl Hydrocarbon Receptor Antagonism Mitigates Cytokine-Mediated Inflammatory Signalling in Primary Human Fibroblast-Like Synoviocytes. Ann. Rheum. Dis. 72 (10), 1708–1716. doi:10.1136/annrheumdis-2012-202639
Latourte, A., Kloppenburg, M., and Richette, P. (2020). Emerging Pharmaceutical Therapies for Osteoarthritis. Nat. Rev. Rheumatol. 16 (12), 673–688. doi:10.1038/s41584-020-00518-6
Le, L. T., Swingler, T. E., Crowe, N., Vincent, T. L., Barter, M. J., Donell, S. T., et al. (2016). The MicroRNA-29 Family in Cartilage Homeostasis and Osteoarthritis. J. Mol. Med. (Berl). 94 (5), 583–596. doi:10.1007/s00109-015-1374-z
Li, Y., Pan, D., Liu, S., Xing, X., Zhou, H., Zhang, B., et al. (2021). Identification of Circ-Fam169a Sponges miR-583 Involved in the Regulation of Intervertebral Disc Degeneration. J. Orthop. Translat. 26, 121–131. doi:10.1016/j.jot.2020.07.007
Liang, C., Li, J., Lu, C., Xie, D., Liu, J., Zhong, C., et al. (2019). HIF1α Inhibition Facilitates Leflunomide-AHR-CRP Signaling to Attenuate Bone Erosion in CRP-Aberrant Rheumatoid Arthritis. Nat. Commun. 10 (1), 4579. doi:10.1038/s41467-019-12163-z
Liang, Y., Chen, S., Yang, Y., Lan, C., Zhang, G., Ji, Z., et al. (2018). Vasoactive Intestinal Peptide Alleviates Osteoarthritis Effectively via Inhibiting NF-Κb Signaling Pathway. J. Biomed. Sci. 25 (1), 25. doi:10.1186/s12929-018-0410-z
Lin, N. Y., Distler, A., Beyer, C., Philipi-Schöbinger, A., Breda, S., Dees, C., et al. (2016). Inhibition of Notch1 Promotes Hedgehog Signalling in a HES1-Dependent Manner in Chondrocytes and Exacerbates Experimental Osteoarthritis. Ann. Rheum. Dis. 75 (11), 2037–2044. doi:10.1136/annrheumdis-2015-208420
Lolli, A., Sivasubramaniyan, K., Vainieri, M. L., Oieni, J., Kops, N., Yayon, A., et al. (2019). Hydrogel-Based Delivery of antimiR-221 Enhances Cartilage Regeneration by Endogenous Cells. J. Control. Release. 309, 220–230. doi:10.1016/j.jconrel.2019.07.040
Lü, G., Li, L., Wang, B., and Kuang, L. (2020). LINC00623/miR-101/HRAS Axis Modulates IL-1β-Mediated ECM Degradation, Apoptosis and Senescence of Osteoarthritis Chondrocytes. Aging. 12 (4), 3218–3237. doi:10.18632/aging.102801
Makki, M. S., Haseeb, A., and Haqqi, T. M. (2015). MicroRNA-9 Promotion of Interleukin-6 Expression by Inhibiting Monocyte Chemoattractant Protein-Induced Protein 1 Expression in Interleukin-1β-Stimulated Human Chondrocytes. Arthritis Rheumatol. 67 (8), 2117–2128. doi:10.1002/art.39173
Mentlein, R. (2007). Targeting Pleiotropin to Treat Osteoarthritis. Expert Opin. Ther. Targets. 11 (7), 861–867. doi:10.1517/14728222.11.7.861
Nakamura, A., Rampersaud, Y. R., Nakamura, S., Sharma, A., Zeng, F., Rossomacha, E., et al. (2019). MicroRNA-181a-5p Antisense Oligonucleotides Attenuate Osteoarthritis in Facet and Knee Joints. Ann. Rheum. Dis. 78 (1), 111–121. doi:10.1136/annrheumdis-2018-213629
Ogando, J., Tardáguila, M., Díaz-Alderete, A., Usategui, A., Miranda-Ramos, V., Martínez-Herrera, D. J., et al. (2016). Notch-Regulated miR-223 Targets the Aryl Hydrocarbon Receptor Pathway and Increases Cytokine Production in Macrophages From Rheumatoid Arthritis Patients. Sci. Rep. 6, 20223. doi:10.1038/srep20223
Okubo, M., Kimura, T., Fujita, Y., Mochizuki, S., Niki, Y., Enomoto, H., et al. (2011). Semaphorin 3A Is Expressed in Human Osteoarthritic Cartilage and Antagonizes Vascular Endothelial Growth Factor 165-promoted Chondrocyte Migration: an Implication for Chondrocyte Cloning. Arthritis Rheum. 63 (10), 3000–3009. doi:10.1002/art.30482
Orita, S., Ishikawa, T., Miyagi, M., Ochiai, N., Inoue, G., Eguchi, Y., et al. (2011). Pain-Related Sensory Innervation in Monoiodoacetate-Induced Osteoarthritis in Rat Knees That Gradually Develops Neuronal Injury in Addition to Inflammatory Pain. BMC Musculoskelet. Disord. 12, 134. doi:10.1186/1471-2474-12-134
Park, R., and Ji, J. D. (2016). Unique Gene Expression Profile in Osteoarthritis Synovium Compared With Cartilage: Analysis of Publicly Accessible Microarray Datasets. Rheumatol. Int. 36 (6), 819–827. doi:10.1007/s00296-016-3451-1
Piper, C. J. M., Rosser, E. C., Oleinika, K., Nistala, K., Krausgruber, T., Rendeiro, A. F., et al. (2019). Aryl Hydrocarbon Receptor Contributes to the Transcriptional Program of IL-10-Producing Regulatory B Cells. Cell Rep. 29 (7), 1878–e7. doi:10.1016/j.celrep.2019.10.018
Platnich, J. M., and Muruve, D. A. (2019). NOD-Like Receptors and Inflammasomes: A Review of Their Canonical and Non-Canonical Signaling Pathways. Arch. Biochem. Biophys. 670, 4–14. doi:10.1016/j.abb.2019.02.008
Pufe, T., Bartscher, M., Petersen, W., Tillmann, B., and Mentlein, R. (2003). Pleiotrophin, an Embryonic Differentiation and Growth Factor, Is Expressed in Osteoarthritis. Osteoarthritis Cartilage. 11 (4), 260–264. doi:10.1016/s1063-4584(02)00385-0
Pufe, T., Groth, G., Goldring, M. B., Tillmann, B., and Mentlein, R. (2007). Effects of Pleiotrophin, a Heparin-Binding Growth Factor, on Human Primary and Immortalized Chondrocytes. Osteoarthritis Cartilage. 15 (2), 155–162. doi:10.1016/j.joca.2006.07.005
Rosser, E. C., Piper, C. J. M., Matei, D. E., Blair, P. A., Rendeiro, A. F., Orford, M., et al. (2020). Microbiota-Derived Metabolites Suppress Arthritis by Amplifying Aryl-Hydrocarbon Receptor Activation in Regulatory B Cells. Cell Metab. 31 (4), 837–e10. doi:10.1016/j.cmet.2020.03.003
Sandell, L. J. (2012). Etiology of Osteoarthritis: Genetics and Synovial Joint Development. Nat. Rev. Rheumatol. 8 (2), 77–89. doi:10.1038/nrrheum.2011.199
Shannon, P., Markiel, A., Ozier, O., Baliga, N. S., Wang, J. T., Ramage, D., et al. (2003). Cytoscape: a Software Environment for Integrated Models of Biomolecular Interaction Networks. Genome Res. 13 (11), 2498–2504. doi:10.1101/gr.1239303
Shkhyan, R., Van Handel, B., Bogdanov, J., Lee, S., Yu, Y., Scheinberg, M., et al. (2018). Drug-Induced Modulation of Gp130 Signalling Prevents Articular Cartilage Degeneration and Promotes Repair. Ann. Rheum. Dis. 77 (5), 760–769. doi:10.1136/annrheumdis-2017-212037
Soul, J., Dunn, S. L., Anand, S., Serracino-Inglott, F., Schwartz, J. M., Boot-Handford, R. P., et al. (2018). Stratification of Knee Osteoarthritis: Two Major Patient Subgroups Identified by Genome-Wide Expression Analysis of Articular Cartilage. Ann. Rheum. Dis. 77 (3), 423. doi:10.1136/annrheumdis-2017-212603
Styrkarsdottir, U., Lund, S. H., Thorleifsson, G., Zink, F., Stefansson, O. A., Sigurdsson, J. K., et al. (2018). Meta-analysis of Icelandic and UK Data Sets Identifies Missense Variants in SMO, IL11, COL11A1 and 13 More New Loci Associated With Osteoarthritis. Nat. Genet. 50 (12), 1681–1687. doi:10.1038/s41588-018-0247-0
Sugita, S., Hosaka, Y., Okada, K., Mori, D., Yano, F., Kobayashi, H., et al. (2015). Transcription Factor Hes1 Modulates Osteoarthritis Development in Cooperation With Calcium/Calmodulin-Dependent Protein Kinase 2. Proc. Natl. Acad. Sci. U S A. 112 (10), 3080–3085. doi:10.1073/pnas.1419699112
Sumi, C., Hirose, N., Yanoshita, M., Takano, M., Nishiyama, S., Okamoto, Y., et al. (2018). Semaphorin 3A Inhibits Inflammation in Chondrocytes under Excessive Mechanical Stress. Mediators Inflamm. 2018, 5703651. doi:10.1155/2018/5703651
Szklarczyk, D., Gable, A. L., Nastou, K. C., Lyon, D., Kirsch, R., Pyysalo, S., et al. (2021). The STRING Database in 2021: Customizable Protein-Protein Networks, and Functional Characterization of User-Uploaded Gene/measurement Sets. Nucleic Acids Res. 49 (D1), D605–D612. doi:10.1093/nar/gkaa1074
Ter Elst, A., Ma, B., Scherpen, F. J., de Jonge, H. J., Douwes, J., Wierenga, A. T., et al. (2011). Repression of Vascular Endothelial Growth Factor Expression by the Runt-Related Transcription Factor 1 in Acute Myeloid Leukemia. Cancer Res. 71 (7), 2761–2771. doi:10.1158/0008-5472.CAN-10-0402
Tsuji, G., Takahara, M., Uchi, H., Matsuda, T., Chiba, T., Takeuchi, S., et al. (2012). Identification of Ketoconazole as an AhR-Nrf2 Activator in Cultured Human Keratinocytes: the Basis of its Anti-inflammatory Effect. J. Invest. Dermatol. 132 (1), 59–68. doi:10.1038/jid.2011.194
Tuerlings, M., van Hoolwerff, M., Houtman, E., Suchiman, E. H. E. D., Lakenberg, N., Mei, H., et al. (2021). RNA Sequencing Reveals Interacting Key Determinants of Osteoarthritis Acting in Subchondral Bone and Articular Cartilage: Identification of IL11 and CHADL as Attractive Treatment Targets. Arthritis Rheumatol. 73 (5), 789–799. doi:10.1002/art.41600
Umeno, J., Matsumoto, T., Fuyuno, Y., Esaki, M., and Torisu, T. (2021). SLCO2A1 Gene Is the Causal Gene for Both Primary Hypertrophic Osteoarthropathy and Hereditary Chronic Enteropathy. J. Orthop. Translat. 28, 10–11. doi:10.1016/j.jot.2020.12.005
Valdes, A. M., and Spector, T. D. (2011). Genetic Epidemiology of Hip and Knee Osteoarthritis. Nat. Rev. Rheumatol. 7 (1), 23–32. doi:10.1038/nrrheum.2010.191
van der Kraan, P. M. (2017). The Changing Role of TGFβ in Healthy, Ageing and Osteoarthritic Joints. Nat. Rev. Rheumatol. 13 (3), 155–163. doi:10.1038/nrrheum.2016.219
Vasheghani, F., Zhang, Y., Li, Y. H., Blati, M., Fahmi, H., Lussier, B., et al. (2015). PPARγ Deficiency Results in Severe, Accelerated Osteoarthritis Associated With Aberrant mTOR Signalling in the Articular Cartilage. Ann. Rheum. Dis. 74 (3), 569–578. doi:10.1136/annrheumdis-2014-205743
Weber, D., Wiese, C., and Gessler, M. (2014). Hey BHLH Transcription Factors. Curr. Top. Dev. Biol. 110, 285–315. doi:10.1016/B978-0-12-405943-6.00008-7
Wu, Z., Nagata, K., and Iijima, T. (2002). Involvement of Sensory Nerves and Immune Cells in Osteophyte Formation in the Ankle Joint of Adjuvant Arthritic Rats. Histochem. Cel Biol. 118 (3), 213–220. doi:10.1007/s00418-002-0443-x
Xu, J., Jiang, C., Zhu, W., Wang, B., Yan, J., Min, Z., et al. (2015). NOD2 Pathway via RIPK2 and TBK1 Is Involved in the Aberrant Catabolism Induced by T-2 Toxin in Chondrocytes. Osteoarthritis Cartilage. 23 (9), 1575–1585. doi:10.1016/j.joca.2015.04.016
Yang, H., Wen, Y., Zhang, M., Liu, Q., Zhang, H., Zhang, J., et al. (2020). MTORC1 Coordinates the Autophagy and Apoptosis Signaling in Articular Chondrocytes in Osteoarthritic Temporomandibular Joint. Autophagy. 16 (2), 271–288. doi:10.1080/15548627.2019.1606647
Yang, S., Kim, J., Ryu, J. H., Oh, H., Chun, C. H., Kim, B. J., et al. (2010). Hypoxia-Inducible Factor-2Alpha Is a Catabolic Regulator of Osteoarthritic Cartilage Destruction. Nat. Med. 16 (6), 687–693. doi:10.1038/nm.2153
Yang, S., Ryu, J. H., Oh, H., Jeon, J., Kwak, J. S., Kim, J. H., et al. (2015). NAMPT (Visfatin), a Direct Target of Hypoxia-Inducible Factor-2α, Is an Essential Catabolic Regulator of Osteoarthritis. Ann. Rheum. Dis. 74 (3), 595–602. doi:10.1136/annrheumdis-2013-204355
Yano, F., Hojo, H., Ohba, S., Fukai, A., Hosaka, Y., Ikeda, T., et al. (2013). A Novel Disease-Modifying Osteoarthritis Drug Candidate Targeting Runx1. Ann. Rheum. Dis. 72 (5), 748–753. doi:10.1136/annrheumdis-2012-201745
Yano, F., Ohba, S., Murahashi, Y., Tanaka, S., Saito, T., and Chung, U. I. (2019). Runx1 Contributes to Articular Cartilage Maintenance by Enhancement of Cartilage Matrix Production and Suppression of Hypertrophic Differentiation. Sci. Rep. 9 (1), 7666. doi:10.1038/s41598-019-43948-3
Yuan, C., Pan, Z., Zhao, K., Li, J., Sheng, Z., Yao, X., et al. (2020). Classification of Four Distinct Osteoarthritis Subtypes With a Knee Joint Tissue Transcriptome Atlas. Bone Res. 8 (1), 38. doi:10.1038/s41413-020-00109-x
Zhang, F. J., Luo, W., and Lei, G. H. (2015). Role of HIF-1α and HIF-2α in Osteoarthritis. Jt. Bone Spine. 82 (3), 144–147. doi:10.1016/j.jbspin.2014.10.003
Zhen, G., Wen, C., Jia, X., Li, Y., Crane, J. L., Mears, S. C., et al. (2013). Inhibition of TGF-β Signaling in Mesenchymal Stem Cells of Subchondral Bone Attenuates Osteoarthritis. Nat. Med. 19 (6), 704–712. doi:10.1038/nm.3143
Zhou, Y., Zhou, B., Pache, L., Chang, M., Khodabakhshi, A. H., Tanaseichuk, O., et al. (2019). Metascape Provides a Biologist-Oriented Resource for the Analysis of Systems-Level Datasets. Nat. Commun. 10 (1), 1523. doi:10.1038/s41467-019-09234-6
Keywords: osteoarthiritis, overlapping genes, signaling pathways, miRNAs, bioinformatics
Citation: Chang L, Yao H, Yao Z, Ho KK-W, Ong MT-Y, Dai B, Tong W, Xu J and Qin L (2021) Comprehensive Analysis of Key Genes, Signaling Pathways and miRNAs in Human Knee Osteoarthritis: Based on Bioinformatics. Front. Pharmacol. 12:730587. doi: 10.3389/fphar.2021.730587
Received: 25 June 2021; Accepted: 12 August 2021;
Published: 23 August 2021.
Edited by:
Liangliang Xu, Guangzhou University of Chinese Medicine, ChinaCopyright © 2021 Chang, Yao, Yao, Ho, Ong, Dai, Tong, Xu and Qin. This is an open-access article distributed under the terms of the Creative Commons Attribution License (CC BY). The use, distribution or reproduction in other forums is permitted, provided the original author(s) and the copyright owner(s) are credited and that the original publication in this journal is cited, in accordance with accepted academic practice. No use, distribution or reproduction is permitted which does not comply with these terms.
*Correspondence: Jiankun Xu, amlhbmt1bnh1QGN1aGsuZWR1Lmhr; Ling Qin, bGluZ3FpbkBjdWhrLmVkdS5oaw==