- 1Tianjin University of Traditional Chinese Medicine, Tianjin, China
- 2NMPA Key Laboratory for Quality Control of Traditional Chinese Medicine, Shanghai Institute for Food and Drug Control, Shanghai, China
- 3Shanghai University of Traditional Chinese Medicine, Shanghai, China
Realgar has been used as a type of mineral drug that contains arsenic for thousands of years. Previous studies have shown that Realgar-induced acute kidney injury is associated with abnormal metabolism, but the underlying mechanism is poorly understood. The aim of this study is to investigate the metabolic changes in serum and kidney tissues of mice exposed to Realgar by using a metabolomic approach and explore the molecular mechanisms of acute kidney injury induced by Realgar. Forty mice were randomly divided into four groups: Control group, 0.5-, 1.0, and 2.0 g/kg Realgar group. After 1 week, the body weight and kidney weight of the mice were measured. The serum and kidney samples were used for LC-MS spectroscopic metabolic profiling. Principal component analysis (PCA), correlation analysis, and pathway analysis were used to detect the nephrotoxic effects of Realgar. Body weight decreased significantly in the 2.0 g/kg group, and the kidney weight index also showed a dose-dependent increase in Realgar. The PCA score plot showed the serum and kidney tissue metabolic profile of mice exposed to 2.0 g/kg Realgar separated from the control group, while the lower-doses of 0.5 g/kg and 1.0 g/kg Realgar shown a similar view to the Control group. Thirty-three metabolites and seventeen metabolites were screened and identified in the serum and kidney of mice in a dose-dependent manner. respectively. Correlation analysis showed a strong correlation among these metabolites. Amino acid metabolism, lipid metabolism, glutathione metabolism, and purine metabolism pathways were found to be mainly associated with Realgar nephrotoxicity. This work illustrated the metabolic alterations in Realgar-induced nephrotoxic mice through a metabolomic approach.
Introduction
Arsenic has long been known as a toxic compound and environmental carcinogen, which exposure causes oxidative stress, apoptosis, chromosomal abnormalities, and DNA damage (Zhou and Xi, 2018; Palma-Lara et al., 2020). However, Arsenic has shown amazing therapeutic value in the treatment of hematopoietic malignancies (Zhu et al., 2019). Arsenic trioxide, as an arsenic-containing compound, has shown good remission in the treatment of acute promyelocytic leukemia (APL) (Min et al., 2020). Arsenic trioxide shows great toxic effects and causes serious adverse reactions especially in the case of long-term use (Wang et al., 2020).
Realgar, a traditional Chinese mineral medicine containing more than 90% tetraarsenic tetrasulfide (As4S4), has been widely used to treat infection, fever, and convulsion (Liu et al., 2018). Recently, Realgar has similar efficacy to arsenic trioxide in the treatment of APL and has fewer side effects (Wu et al., 2011). However, arsenic contained in Realgar is still a potentially toxic substance. Realgar and its compounds can cause adverse reactions or chronic arsenic poisoning when used chronically, in excess, and irregularly (Yi et al., 2018; Zheng et al., 2019). Some studies suggested that exposure to Realgar can lead to arsenic and various arsenical metabolites accumulation in the kidneys, which is a target organ for its metabolism and excretion (Wu et al., 2019). Thus, the metabolism of arsenic in Realgar-induced toxicity plays a leading role. Our previous work also revealed that Realgar can cause acute kidney injury and it is mainly related to the metabolic pathways (Zhang et al., 2021). Main recent studies concentrated on the determination of arsenic levels in blood, urine, and tissues (Wu et al., 2019; Yi et al., 2020). However, the metabolism changes of Realgar are still unclear.
Metabolomics is a systemic approach by applying modern advanced mass spectrometry techniques to identify and quantify mainly endogenous metabolites (around smaller than 1,000 Da) of complex systems. This consists of the holistic thinking of TCMs and metabolomics techniques are extremely suitable for evaluating the toxicity of traditional Chinese medicines and exploring the mechanisms (Wang et al., 2011). Recently, studies on the toxicological effects of Realgar or Realgar-containing medicines have been reported, and have mainly focused on hepatotoxicity (Zhang et al., 2017) and neurotoxicity (Zhang et al., 2019). However, less metabolic analysis of nephrotoxicity has been reported.
In this study, the nephrotoxic mechanism of Realgar was investigated by an untargeted metabolomic approach to analyze serum and kidney samples collected from control mice and mice treated with different doses of Realgar, which is expected to deepen the understanding of metabolic changes after exposure to Realgar.
Materials and Methods
Materials and Reagents
Realgar was provided and identified by the Shanghai Institute for Food and Drug Control (Shanghai, China) with 73.7% total arsenitc determined by Atomic Absorption Spectroscopy. Sodium carboxymethycellulose (CMC-Na) was purchased from Sinopharm (Beijiing, China). Mass spectrometry reagents including methanol, acetonitrile and formic acid were purchased from Sigma Chemical Co. (St. Louis, MO, United States).
Animals and Treatment
Male 6-week-old ICR mice weighing about 18–20 g were provided by the Experimental Animal Center of Shanghai Institute for Food and Drug Control (SCXK 2018-0006, Shanghai, China). Mice were maintained at temperature (20–25°C) and 40–60% relative humidity for a 12-h light/dark cycle. They were fed freely water and diet, after 1 week of acclimatization, the mice were randomly divided into four groups for the treatment of 0.5% CMC-Na (Control group), Realgar suspended in 0.5% CMC-Na (0.5, 1.0, and 2.0 g/kg), respectively. The doses of Realar were referred to the basis of previously published literature (Miao et al., 2011; Xia et al., 2018). The detailed experiment procedure was shown in Figure 1. The body weight was measured every 2 days. After 1 week, blood samples were collected from the retro-orbital sinus of mice, and serum was collected after centrifugation. The mice were anesthetized, and the kidneys were collected immediately on ice and stored at −80°C for metabolomic analysis. The experimental protocol was performed according to the Animal experiment guidelines and approved by the animal Ethics Committee of Shanghai Institute for Food and Drug Control (IACUC-SIFDC20111).
Sample Preparation
Serum samples were collected after following oral feeding Realgar for 30 min, then 50 μL of serum was mixed with 200 μL pre-cooled methanol and vortex mixed for 10 min. The mixture was then centrifuged at 14,000 rpm for 15 min at 4°C, and the supernatant is dried with nitrogen and reconstituted with 20% methanol. The supernatant was centrifuged at 14,000 rpm for 15 min at 4°C and used to analyze by LC-MS. A mixture of equal quantities was extracted from all samples and used as quality control (QC) sample for the LC-MS analysis.
The kidney tissues were dried by lyophilization and ground into powder. The powder (10 mg) was homogenized with pre-cooled 80% methanol (300 µL), mixed by vortexing, and subjected to sonication for 10 min on iced water (×3). The homogenate was then incubated at −20°C for 30 min and centrifuged at 14,000 rpm for 20 min at 4°C. The supernatant is dried with nitrogen and reconstituted with 80% methanol and centrifuged at 14,000 rpm at 4°C for 15 min. The supernatant was added to the sample bottle for detection. A mixture of equal quantities was extracted from all samples and used as QC sample for the LC-MS analysis.
LC-MS Data Analysis
Kidney metabolic profiling adopted an UltiMate 3,000 ultra-high-performance LC system (Thermo Fisher Scientific) ACQUITY UPLC® BEH C18 column (2.1 × 100 mm, 1.7 µm; Waters Corp.), coupled with Orbitrap Fusion Lumos high-resolution mass spectrometer (Thermo Fisher Scientific, USA). The mobile phase consisted of solvent A (0.1% formic acid in water) and solvent B (acetonitrile) with running a gradient elution: 5% phase B for 4 min; 5–70% phase B for 7 min, 70–95% phase B for 15 min, 95% for 17 min, 95–5% phase B for 20 min at the flow rate of 0.2 ml/min. The injection volume was 5 μL. The MS signal acquisition was performed in positive and negative ion scanning modes respectively. One primary mass spectrometry scan resolution is 15,000 and secondary mass spectrometry scans resolution is 6,000, and both the first and secondary scanning ranges were 105 m/z—1,050 m/z. A QC sample was acquired for every six analytical samples to evaluate the stability of the analytical system and assess the reliability of the results.
Data Analysis
All data were presented as the mean ± SEM. The comparisons between two groups were performed with an unpaired two-tailed Student’s t-test using SPSS 23.0. The comparisons between several groups were performed with a one-way analysis of variance (ANOVA) using SPSS 23.0. Value of p < 0.05 was considered significant differences. The mass spectrometry-generated metabolomics data is in RAW file format. The quantitative analysis of the compound was conducted using Compound Discoverer Software 3.2 (Thermo Fisher Scientific, USA). The data was subject to principle components analysis (PCA) and orthogonal partial least squares discriminant analysis (OPLS-DA) using SIMCA-P version 14.1 software. The metabolites with VIP >1 and p < 0.05 were considered as potential biomarkers, and the online databases HMDB (http://www.hmdb.ca) were used to identify the compounds by matching the mass spectrum. Pathway enrichment was acquired according to the MetaboAnalyst 5.0 (http://www.metaboanalyst.ca/) and the Kyoto Encyclopedia of Genes and Genomes (KEGG, https://www.kegg.jp/) database.
Result
Weight Change and Kidney Weight Index
The body mass and kidney index of mice are shown in Table 1. Realgar at the dose of 2.0 g/kg had an effect on the decreased body weight (p < 0.05) and increased kidney weight index (p > 0.05).
Realgar Altered Serum and Kidney Metabolic Profile
The PCA score plots shown good system stability of the analyses since the QC samples were tightly clustered in the serum and kidney samples (Figure 2), and all of the samples were within the confidence interval and the PCA showed no abnormal samples. Two principal components were obtained in positive and negative modes respectively. A slight separation for both serum and kidney samples was presented between the Control group and the Realgar groups (especially 2.0 g/kg Realgar group) in the PCA score plot, which may be due to large differences between samples.
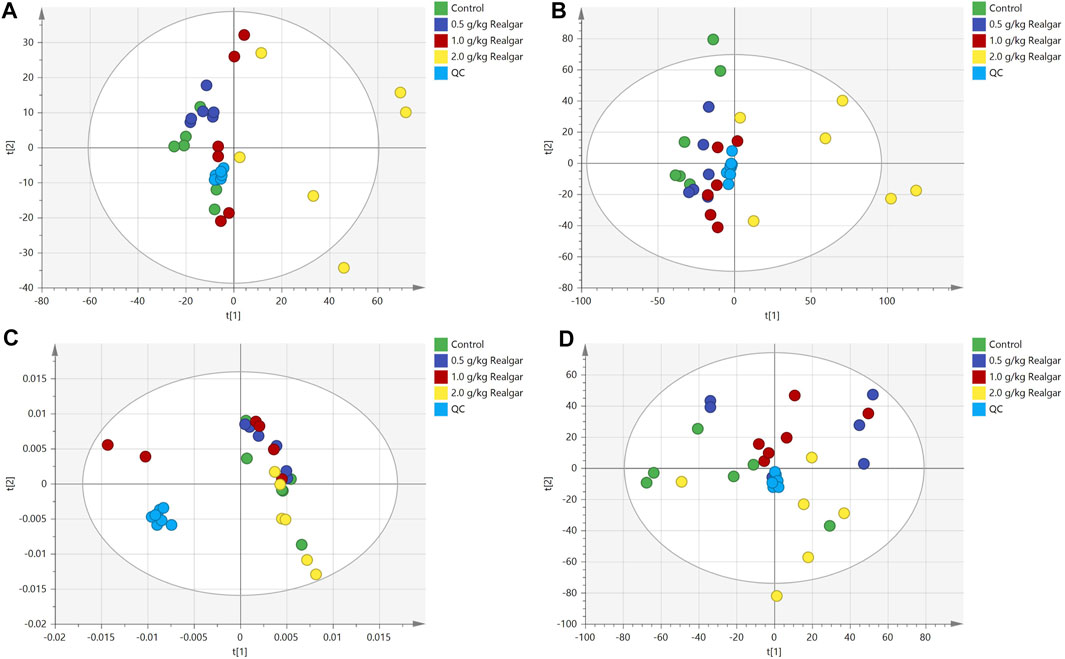
FIGURE 2. Scoring plots of PCA analysis [(A and B) of the positive and negative ion modes of serum samples, respectively; (C and D) of the positive and negative ion modes of the kidney samples, respectively] models with the statistical parameters as follows: (A) R2X = 0.495, Q2 = 0.138; (B) R2X = 0.388, R2Y = 0.841, Q2 = 0.530; (C) R2X = 0.525, Q2 = 0.207; (D) R2X = 0.391, R2Y = 0.867, Q2 = 0.552.
Discovery and Identification of Potential Biomarkers
The 2.0 g/kg dose group revealed a significant change of metabolites in comparison to the control group, so the OPLS-DA was used to monitor the changes of metabolites in the 2.0 g/kg dose group and the control group (Figure 3). R2X (cum) and Q2 (cum) parameters showed the model good fitting and prediction ability, and the separation between the control group and the 2.0 g/kg dose group was significant in the OPLS-DA score plot, indicating that the metabolite content of the 2.0 g/kg dose group of Realgar was different from that of the control group. Permutation tests with 200 iterations showed that the model was not over-fitted (Figure 3). As a visualization method, the S-plot was also used to select potential biomarkers (Figure 3). The importance of variables to classification was measured by the variable importance in the projection (VIP) value, and the variables were further screened according to VIP.
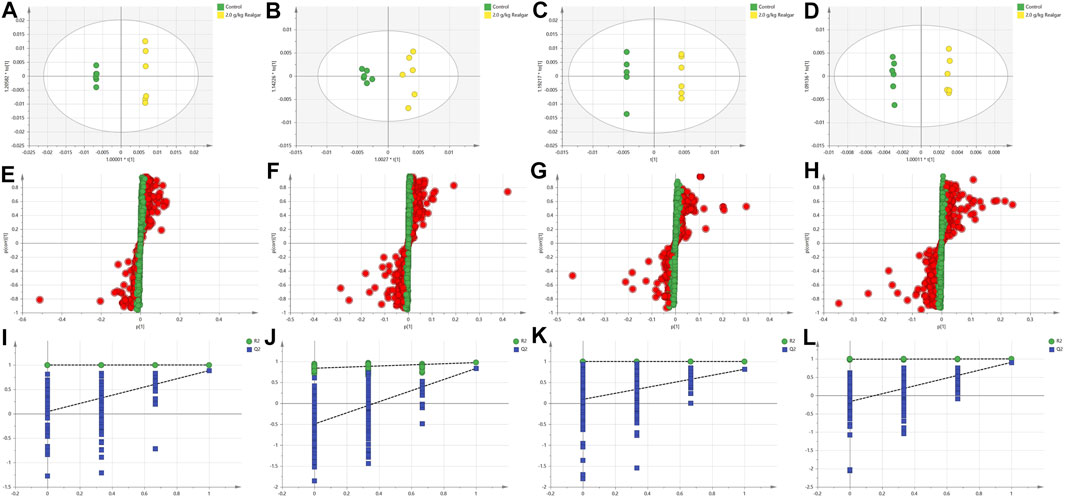
FIGURE 3. OPLS-DA analysis [(A and B) of the positive and negative ion modes of serum samples, respectively; (C and D) of the positive and negative ion modes of the kidney samples, respectively], Permutation test results [(E and F) of the positive and negative ion modes of serum samples, respectively; (G and H) of the positive and negative ion modes of the kidney samples, respectively], and S-plot (I and J of the positive and negative ion modes of serum samples, respectively; (K and L) of the positive and negative ion modes of the kidney samples, respectively). The models with the statistical parameters as follows: (A) R2X = 0.768, R2Y = 1, Q2 = 0.884; (B) R2X = 0.434, R2Y = 0.973, Q2 = 0.834; (C) R2X = 0.897, R2Y = 1, Q2 = 0.814; (D) R2X = 0.654, R2Y = 0.999, Q2 = 0.904; (I) R2 inter = 0.999, Q2 inter = 0.0432; (J) R2 inter = 0.828, Q2 inter = −0.494; (K) R2 inter = 0.1, Q2 inter = 0.0944; (L) R2 inter = 0.99, Q2 inter = −0.167.
The potential biomarkers then were screened based on the strict criteria: 1) the variables with VIP value more than the one is an important screening parameter; 2) the p value less than 0.05 is considered as a significant difference in the candidate metabolite between the Control group and Realgar groups; 3) online databases HMDB (http://www.hmdb.ca) was searched to characterize differential metabolites by matching the mass spectrum; and 4) an error of less than 5 ppm. Following the above criteria, then thirty-three metabolites from serum samples and twenty-three from kidney samples were identified considered as potential biomarkers (Supplementary Tables 1, 2). In order to find the metabolites most related to Realgar-acute kidney injury, Spearman’s rank correlation analysis was performed using SPSS 23.0 software between the relative intensity (total area normalization) of metabolites and Realgar dose. Thirty-three metabolites from serum samples and seventeen from kidney samples were screened (Tables 2, 3 and Supplementary Tables 3, 4). To further understand metabolic differences among the Control group and treatment groups at different doses of Realgar, the metabolites were visualized in a clustering heatmap (Figure 4). The trend of metabolites in the Control group and 2.0 g/kg Realgar group could be easily observed from the heatmap in the mice serum, and similar results were observed in kidney tissues.
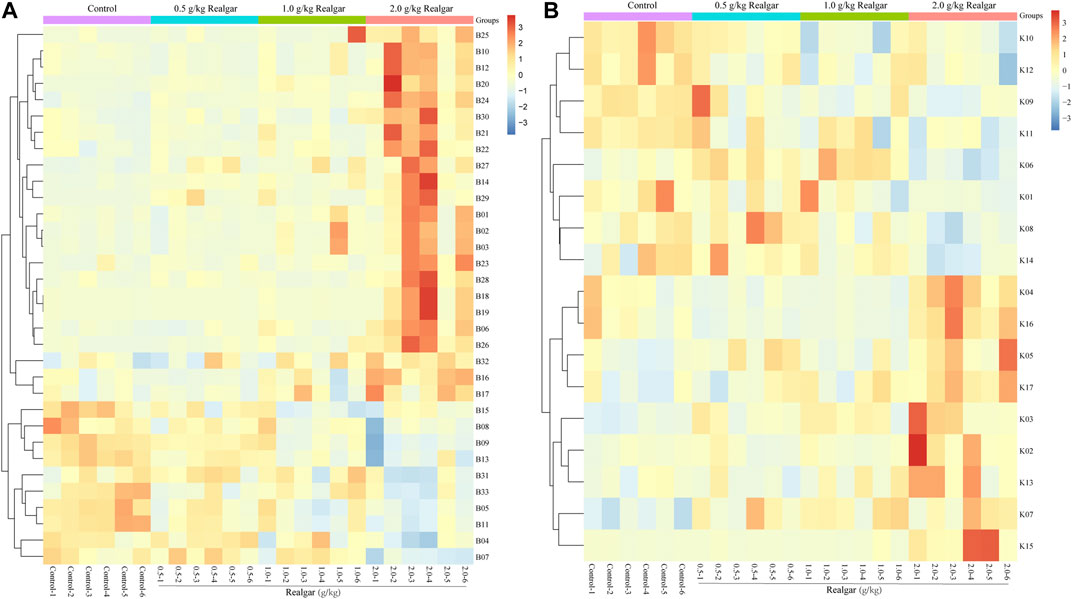
FIGURE 4. Heatmap of potential biomarkers of (A) serum and (B) kidney (n = 6). Rows represent the relative intensities of metabolites, where red represents high relative abundance metabolites and blue represents low relative abundance metabolites. Columns represent individual serum or kidney samples.
Correlation Analysis
To further understand the relationships between metabolites, Pearson’s correlation analysis was performed and shown in Figure 5. Most of the metabolites in the mice had a strong correlation, and they may have similar metabolic pathways and related biological effects. For example, metabolites between amino acid and bile acid components showed a high positive correlation in the serum samples, and there was a high positive correlation between the lipid components in the kidney samples.
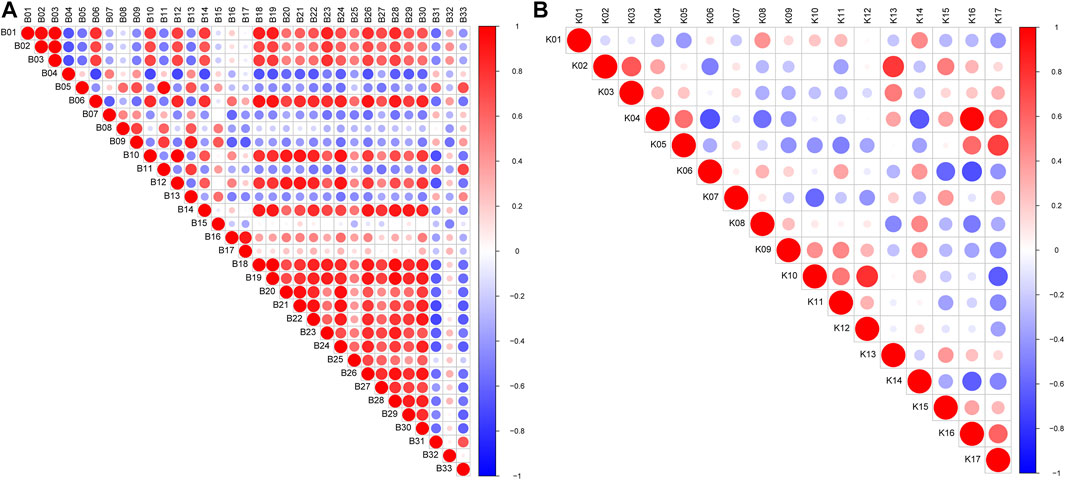
FIGURE 5. Pearson’s correlations of marker metabolites detected by LC/MS in mice (A) serum and (B) kidney. Red color indicates positive correlation between compounds and blue color indicates negative correlation between compounds; and the darker the color, the stronger the correlation.
Metabolic Pathway Analysis
To further clarify the possible metabolic pathways that were affected by Realgar, the metabolites in serum and kidney tissues were integrated and imported into Metaboanalyst 5.0 for pathway analysis, and the pathway was filtered by the parameter Impact >0. As shown in Figure 6 and Supplementary Tables 5, 6, ten metabolic pathways were highlighted from serum samples focusing on including Amino acid metabolism, Glutathione metabolism, and Purine metabolism, and seven metabolic pathways were highlighted from kidney samples focusing on including Amino acid metabolism, Lipid metabolism, Glutathione metabolism, and Purine metabolism.
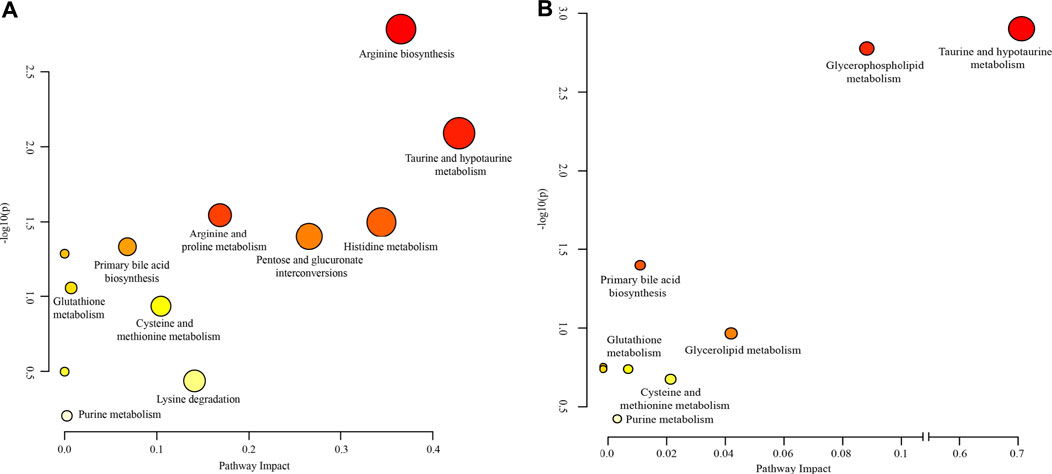
FIGURE 6. Weighted analysis of the metabolic pathways based on the potential biomarkers in (A) serum and (B) kidney samples.
Discussion
The traditional toxicity evaluations showed that Realgar treatments elicited nephrotoxicity, as characterized by elevated blood urea nitrogen, creatinine levels in serum, and kidney histopathologic alterations. These have been confirmed in our previous studies, and Realgar at a high dose induced more severe acute kidney injury than exposure to a low dose (Zhang et al., 2021). In this study, a significant reduction in body weight were observed in the 2.0 g/kg Realgar group, which may be due to persistent non-remission of Realgar-acute kidney injury, increased toxin levels, and inadequate intake of ingested nutrients. Moreover, our previous study also found that metabolic disruption was observed in the kidney of mice exposed to Realgar for 1 week (Zhang et al., 2021). Therefore, a metabolomic approach was used to further investigate the mechanism of acute nephrotoxicity of Realgar-induced acute nephrotoxicity in mice.
High-resolution mass spectrometry combined with metabolomics was used to analyze metabolite alterations in serum and kidney of Realgar mice. Thirty-three endogenous metabolites in serum and seventeen endogenous metabolites in the kidney were selected as toxic biomarkers, which are mainly involved in amino acid metabolism, Lipid metabolism, Glutathione metabolism, and Purine metabolism. Amino acid metabolism, Glautathione metabolism, and Purine metabolism were the same metabolic pathways between serum and kidney, and Lipid metabolism might be partly attributed to oxidative stress caused by Realgar. Therefore, these four pathways were of great attention to us. The mechanism of toxicity was proposed by analyzing their formation and transformation in the pathway (Figure 7).
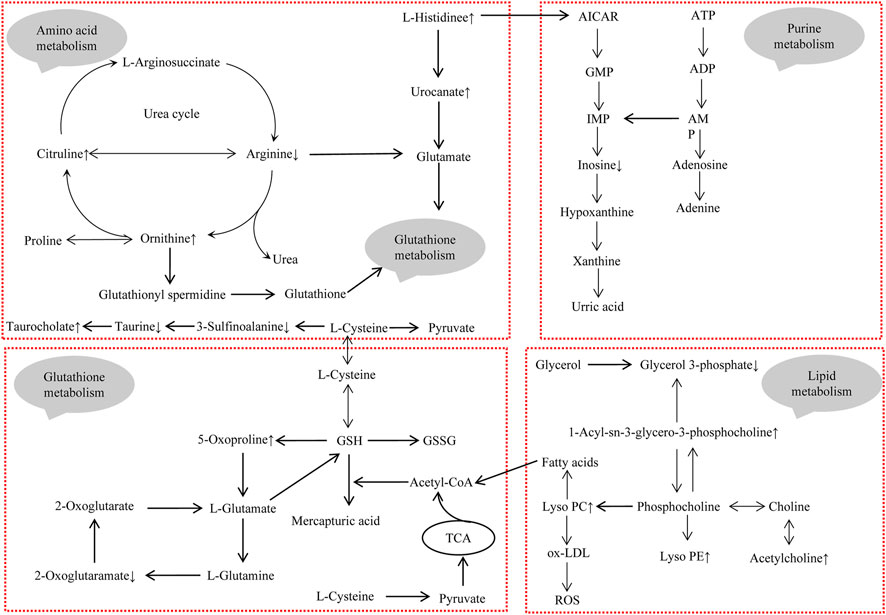
FIGURE 7. The integrated metabolic pathway related to Realgar-induced acute kidney injury. Upward arrows indicate elevated levels after exposure to Realgar, and conversely, downward arrows indicate decreased levels after exposure to Realgar.
Amino Acid Metabolism
Taurine has many different biological functions, bile acid binding, antioxidant, etc., which are important for organism health and disease. Taurine has been reported to have a protective effect against drug-induced nephrotoxicity (Aslanturk and Uzunhisarcikli, 2020). In Realgar-induced hepatotoxicity, the level of taurine was decreased (Huo et al., 2016). In this study, taurine levels were decreased in both serum and kidney of mice. Therefore, taurine may be an important potential marker associated with Realgar-induced nephrotoxicity.
Histidine, Citrulline, Ornithine were only detected in serum samples, and the disruption of amino acid metabolism was observed mainly in serum samples. Histidine is one of the 22 proteinogenic amino acids and can be catabolized to glutamate, which has antioxidant properties (Brosnan and Brosnan, 2020). In this study, Histidine and the catabolic product urocanic acid were increased, indicating that the oxidative response has been activated in mice. Notably, increased level of Histidine in the blood is also a major manifestation of congenital disorders of Histidine metabolism, including histidinemia, maple syrup urine disease, propionic acidemia, and tyrosinemia I (Moro et al., 2020). Ammonia accumulation occurs when amino acid metabolism is disturbed and the urea cycle converts ammonia to urea, which is excreted in the urine. Citrulline, Ornithine, and Arginine play a key role in the urea cycle (Morris, 2002). The increased levels of Citrulline and Ornithine in serum after exposure to Realgar implied an acceleration of the urea cycle. The decreased level of arginine was observed and may reduce the production of glutamate. In addition, increased serum levels of bile acids may be associated with deranged amino acid metabolism.
Lipid Metabolism
Some reports have shown that Realgar can cause the disorders of lipids metabolism in rats and mice (Zhang et al., 2019; Zhang et al., 2017), Pearson’s correlation analysis from kidney samples also indicated that the perturbation of lipids metabolism may play an important role in the nephrotoxicity induced by Realgar. In this study, the increased levels of lysophosphatidylcholine (LPC) and lysophosphatidyl ethanolamine (LPE) were detected in kidney tissue aqueous extracts after Realgar treatment. PC, PE, LPC, and LPE are the main phospholipid components to constitute the cell membrane structure and can mutually transform (Fujita et al., 2014; Canning et al., 2016). LPC is an important fragment of ox-LDL, notably, ox-LDL is an indicator of lipid oxidation that induces ROS formation and causes oxidative damage to cells (Bao et al., 2018). Furthermore, LPC can directly increase Ca2+ concentration and induce ROS production to initially induce oxidative stress (Jung et al., 2017). Arsenic has also been shown to induce cellular autophagy, and PE is involved in the synthetic conversion of the key protein LC3 to LC3-II (Wei et al., 2019; You et al., 2019). However, the levels of LPE in serum were unstable, which may be due to serum metabolites come from multiple organs.
Glutathione Metabolism
Glutathione (GSH), an important intracellular regulatory metabolite, is involved in several reaction systems, especially in defense against oxidative stress. In our study, pathway analysis showed that metabolites in serum and metabolites in the kidney simultaneously regulate glutathione metabolism. As shown in Figure 7, several metabolites (such as Ornithine, Arginine, 2-Oxoglutaramate, and 5-Oxoproline) are closely associated with glutathione metabolism, suggesting that Realgar induced glutathione metabolic processes in mice kidney tissue, which is consistent with the literature (Zhou et al., 2017). There may be an exchange of substances between serum metabolites and renal metabolites, with amino acids in serum providing the basic substances involved in glutathione metabolism, such as glutamate, which are metabolized by the kidneys and returned to serum. Furthermore, arsenic has been shown to cause toxicity mainly by binding to sulfhydryl-containing proteins (Mandal, 2017). The GSH molecule is characterized by an active sulfhydryl group, and also activates sulfhydryl (SH) enzyme-coenzyme activity, which can participate in heavy metal toxicity reactions (Chen et al., 2021).
Purine Metabolism
Histidine metabolic disruption has been observed in this study, and its metabolite AICAR activates the purine metabolic process (Daignan-Fornier and Pinson, 2012). Most of the purine compounds are oxidized to uric acid, which is excreted in the urine, and the level of blood uric acid is closely related to kidney damage. However, in this study, changes in uric acid levels were not detected in either serum or kidney.
This study provided more comprehensive information about the toxicity of Realgar through testing both serum and renal metabolic profiling. However, limitations of this study need to be further addressed. Firstly, serum metabolites come from multiple organs, such as kidney, liver, intestine and so on. Therefore, this result is not specific for reflecting the nephrotoxicity of Realgar. This is one of the tasks that our research group is currently preparing to carry out. Secondly, some metabolites may be not associated with Realgar, but due to acute kidney injury. Acute kidney injury animal model will be established to screen out specific metabolites by comparing Realgar-induced acute kidney injury with other causes-induced acute kidney injury. In addition, we are also trying to find clinical samples of acute kidney injury patients.
Conclusion
This work aimed to study Realgar-induced acute kidney injury through using a high-resolution mass spectrometry technology combined with a metabolomics approach. LC-MS analysis of mice serum and kidney highlighted some disturbances in the endogenous metabolite profile, which may be related to disturbances in the biochemical pathways of acute kidney injury induced by Realgar. In the study, amino acid metabolism is most affected by Realgar. Lipid metabolism, Glutathione metabolism, and Purine metabolism were also affected by Realgar. The high-resolution mass spectrometry combined with metabolomics can capture and explore the metabolic alterations induced by traditional Chinese medicines in toxicological effects and can be used to further investigate the toxicity mechanisms of traditional Chinese medicines.
Data Availability Statement
The original contributions presented in the study are included in the article/Supplementary Material, further inquiries can be directed to the corresponding author.
Ethics Statement
The animal study was reviewed and approved by animal Ethics Committee of Shanghai Institute for Food and Drug Control (IACUC-SIFDC20111).
Author Contributions
SZ wrote the paper. SZ, CL, and TF performed the experiments. SC and HZ prepared the figures. LL, QH, and, XM analyzed and commented the results. SJ designed this project supervised the project. All authors contributed to the article and approved the submitted version.
Funding
This study was supported by the National Key R&D Program (Grant number: 2017YFC1700800), Science and Technology Commission Technical Standards Project of Shanghai (Grant number: 19DZ2202800), and Science and Technology Commission R&D Platform Project of Shanghai (Grant number: 18DZ2292200).
Conflict of Interest
The authors declare that the research was conducted in the absence of any commercial or financial relationships that could be construed as a potential conflict of interest.
Publisher’s Note
All claims expressed in this article are solely those of the authors and do not necessarily represent those of their affiliated organizations, or those of the publisher, the editors and the reviewers. Any product that may be evaluated in this article, or claim that may be made by its manufacturer, is not guaranteed or endorsed by the publisher.
Supplementary Material
The Supplementary Material for this article can be found online at: https://www.frontiersin.org/articles/10.3389/fphar.2021.706249/full#supplementary-material
References
Aslanturk, A., and Uzunhisarcikli, M. (2020). Protective Potential of Curcumin or Taurine on Nephrotoxicity Caused by Bisphenol A. Environ. Sci. Pollut. Res. Int. 27 (19), 23994–24003. doi:10.1007/s11356-020-08716-1
Bao, L., Qi, J., Wang, Y. W., Xi, Q., Tserennadmid, T., Zhao, P. F., et al. (2018). The Atherogenic Actions of LPC on Vascular Smooth Muscle Cells and its LPA Receptor Mediated Mechanism. Biochem. Biophys. Res. Commun. 503 (3), 1911–1918. doi:10.1016/j.bbrc.2018.07.135
Brosnan, M. E., and Brosnan, J. T. (2020). Histidine Metabolism and Function. J. Nutr. 150 (Suppl. 1), 2570S–2575S. doi:10.1093/jn/nxaa079
Canning, P., Kenny, B. A., Prise, V., Glenn, J., Sarker, M. H., Hudson, N., et al. (2016). Lipoprotein-Associated Phospholipase A2 (Lp-PLA2) as a Therapeutic Target to Prevent Retinal Vasopermeability During Diabetes. Proc. Natl. Acad. Sci. U. S. A. 113 (26), 7213–7218. doi:10.1073/pnas.1514213113
Chen, Q. Y., Liu, L., Yang, L., Dong, B., Wen, Y. Z., Zhang, Z., et al. (2021). Response of Sulfhydryl Compounds in Subcells of Cladophora Rupestris under Pb Stress. Environ. Sci. Pollut. Res. Int. 28 (11), 13112–13123. doi:10.1007/s11356-020-11577-3
Daignan-Fornier, B., and Pinson, B. (2012). 5-Aminoimidazole-4-Carboxamide-1-beta-D-Ribofuranosyl 5'-Monophosphate (AICAR), a Highly Conserved Purine Intermediate with Multiple Effects. Metabolites 2 (2), 292–302. doi:10.3390/metabo2020292
Fujita, K., Sugiyama, A., Otoshi, K., Taogoshi, T., Kimura, Y., Kishikawa, N., et al. (2014). Partial Characterization of Proapoptotic Action of Biliary Deteriorated Lipids on Biliary Epithelial Cells in Pancreaticobiliary Diseases. J. Hepatobiliary Pancreat. Sci. 21 (3), 212–218. doi:10.1002/jhbp.20
Huo, T., Fang, Y., Zhao, L., Xiong, Z., Zhang, Y., Wang, Y., et al. (2016). 1HNMR-Based Metabonomic Study of Sub-chronic Hepatotoxicity Induced by Realgar. J. Ethnopharmacol 192, 1–9. doi:10.1016/j.jep.2016.07.003
Jung, H. J., Im, S. S., Song, D. K., and Bae, J. H. (2017). Effects of Chlorogenic Acid on Intracellular Calcium Regulation in Lysophosphatidylcholine-Treated Endothelial Cells. BMB Rep. 50 (6), 323–328. doi:10.5483/bmbrep.2017.50.6.182
Liu, J., Wei, L. X., Wang, Q., Lu, Y. F., Zhang, F., Shi, J. Z., et al. (2018). A Review of Cinnabar (HgS) And/or Realgar (As4S4)-Containing Traditional Medicines. J. Ethnopharmacol 210, 340–350. doi:10.1016/j.jep.2017.08.037
Mandal, P. (2017). Molecular Insight of Arsenic-Induced Carcinogenesis and its Prevention. Naunyn Schmiedebergs Arch. Pharmacol. 390 (5), 443–455. doi:10.1007/s00210-017-1351-x
Miao, J. W., Liang, S. X., Wu, Q., Liu, J., and Sun, A. S. (2011). Toxicology Evaluation of Realgar-Containing Niu-Huang-Jie-Du Pian as Compared to Arsenicals in Cell Cultures and in Mice. ISRN Toxicol. 2011, 250387. doi:10.5402/2011/250387
Min, G. J., Cho, B. S., Park, S. S., Park, S., Jeon, Y. W., Yahng, S. A., et al. (2020). Safety and Efficacy of Arsenic Trioxide and All-Trans Retinoic Acid Therapy in Acute Promyelocytic Leukemia Patients with a High Risk for Early Death. Ann. Hematol. 99 (5), 973–982. doi:10.1007/s00277-020-04010-9
Moro, J., Tomé, D., Schmidely, P., Demersay, T. C., and Azzout-Marniche, D. (2020). Histidine: A Systematic Review on Metabolism and Physiological Effects in Human and Different Animal Species. Nutrients 12 (5), 1414. doi:10.3390/nu12051414
Morris, S. M. (2002). Regulation of Enzymes of the Urea Cycle and Arginine Metabolism. Annu. Rev. Nutr. 22, 87–105. doi:10.1146/annurev.nutr.22.110801.140547
Palma-Lara, I., Martínez-Castillo, M., Quintana-Pérez, J. C., Arellano-Mendoza, M. G., Tamay-Cach, F., Valenzuela-Limón, O. L., et al. (2020). Arsenic Exposure: A Public Health Problem Leading to Several Cancers. Regul. Toxicol. Pharmacol. 110, 104539. doi:10.1016/j.yrtph.2019.104539
Wang, Q. Q., Hua, H. Y., Naranmandura, H., and Zhu, H. H. (2020). Balance between the Toxicity and Anticancer Activity of Arsenic Trioxide in Treatment of Acute Promyelocytic Leukemia. Toxicol. Appl. Pharmacol. 409, 115299. doi:10.1016/j.taap.2020.115299
Wang, X., Sun, H., Zhang, A., Sun, W., Wang, P., and Wang, Z. (2011). Potential Role of Metabolomics Apporoaches in the Area of Traditional Chinese Medicine: as Pillars of the Bridge between Chinese and Western Medicine. J. Pharm. Biomed. Anal. 55 (5), 859–868. doi:10.1016/j.jpba.2011.01.042
Wei, S., Qiu, T., Yao, X., Wang, N., Jiang, L., Jia, X., et al. (2019). Arsenic Induces Pancreatic Dysfunction and Ferroptosis via Mitochondrial ROS-Autophagy-Lysosomal Pathway. J. Hazard. Mater. 384, 121390. doi:10.1016/j.jhazmat.2019.121390
Wu, J., Shao, Y., Liu, J., Chen, G., and Ho, P. C. (2011). The Medicinal Use of Realgar (As₄S₄) and its Recent Development as an Anticancer Agent. J. Ethnopharmacol 135 (3), 595–602. doi:10.1016/j.jep.2011.03.071
Wu, X., Guan, R., Liu, Y., Wu, S., Song, M., and Hang, T. (2019). Comparative Health Risk Assessment of Realgar and NiuHuangJieDu Tablets Based on Tissue Arsenic Levels after Multiple Oral Administration to Rats. J. Ethnopharmacol 249, 112370. doi:10.1016/j.jep.2019.112370
Xia, F., Li, A., Chai, Y., Xiao, X., Wan, J., Li, P., et al. (2018). UPLC/Q-TOFMS-Based Metabolomics Approach to Reveal the Protective Role of Other Herbs in An-Gong-Niu-Huang Wan against the Hepatorenal Toxicity of Cinnabar and Realgar. Front. Pharmacol. 9, 618. doi:10.3389/fphar.2018.00618
Yi, Y., Gao, S., Xia, J., Li, C., Zhao, Y., Zhang, Y., et al. (2018). Data on the Sub-chronic Toxicity in Rats after 30 Days of Oral Realgar Administration and the Accumulation and Distribution of Arsenic Species. Data Brief 23, 103572. doi:10.1016/j.dib.2018.12.011
Yi, Y., Gao, S., Xia, J., Li, C., Zhao, Y., Zhang, Y., et al. (2020). Study of the Accumulation and Distribution of Arsenic Species and Association with Arsenic Toxicity in Rats after 30 Days of Oral Realgar Administration. J. Ethnopharmacol 247, 111576. doi:10.1016/j.jep.2018.10.037
You, Z., Xu, Y., Wan, W., Zhou, L., Li, J., Zhou, T., et al. (2019). TP53INP2 Contributes to Autophagosome Formation by Promoting LC3-ATG7 Interaction. Autophagy 15 (8), 1309–1321. doi:10.1080/15548627.2019.1580510
Zhang, M. H., Chen, J. Q., Guo, H. M., Li, R. T., Gao, Y. Q., Tian, Y., et al. (2017). Combination of LC/MS and GC/MS Based Metabolomics to Study the Hepatotoxic Effect of Realgar Nanoparticles in Rats. Chin. J. Nat. Med. 15 (9), 684–694. doi:10.1016/S1875-5364(17)30098-5
Zhang, S., Li, C., Feng, T., Cao, S., Zhou, H., Li, L., et al. (2021). Proteomics Analysis in the Kidney of Mice Following Oral Feeding Realgar. J. Ethnopharmacol 275, 114118. doi:10.1016/j.jep.2021.114118
Zhang, W., Huo, T., Li, A., Wu, X., Feng, C., Liu, J., et al. (2019). Identification of Neurotoxicity Markers Induced by Realgar Exposure in the Mouse Cerebral Cortex Using Lipidomics. J. Hazard. Mater. 389, 121567. doi:10.1016/j.jhazmat.2019.121567
Zheng, J., Zhang, K., Liu, Y., and Wang, Y. (2019). Fatal Acute Arsenic Poisoning by External Use of Realgar: Case Report and 30 Years Literature Retrospective Study in China. Forensic Sci. Int. 300, e24–e30. doi:10.1016/j.forsciint.2019.03.012
Zhou, Q., and Xi, S. (2018). A Review on Arsenic Carcinogenesis: Epidemiology, Metabolism, Genotoxicity and Epigenetic Changes. Regul. Toxicol. Pharmacol. 99, 78–88. doi:10.1016/j.yrtph.2018.09.010
Zhou, Y., Wang, Y., Su, J., Wu, Z., Wang, C., Zhong, W., et al. (2017). Integration of microRNAome, Proteomics and Metabolomics to Analyze Arsenic-Induced Malignant Cell Transformation. Oncotarget 8 (53), 90879–90896. doi:10.18632/oncotarget.18741
Keywords: realgar, acute kidney injury, metabolomics, serum, kidney, LC-MS
Citation: Zhang S, Li C, Feng T, Cao S, Zhou H, Li L, Hu Q, Mao X and Ji S (2021) A Metabolic Profiling Study of Realgar-Induced Acute Kidney Injury in Mice. Front. Pharmacol. 12:706249. doi: 10.3389/fphar.2021.706249
Received: 07 May 2021; Accepted: 11 August 2021;
Published: 23 August 2021.
Edited by:
Alex Boye, University of Cape Coast, GhanaReviewed by:
Iman Karimzadeh, Shiraz University of Medical Sciences, IranXinhui Liu, Shenzhen Traditional Chinese Medicine Hospital, China
Copyright © 2021 Zhang, Li, Feng, Cao, Zhou, Li, Hu, Mao and Ji. This is an open-access article distributed under the terms of the Creative Commons Attribution License (CC BY). The use, distribution or reproduction in other forums is permitted, provided the original author(s) and the copyright owner(s) are credited and that the original publication in this journal is cited, in accordance with accepted academic practice. No use, distribution or reproduction is permitted which does not comply with these terms.
*Correspondence: Shen Ji, jishen2021@126.com