- 1Key Laboratory of Chinese Materia Medica Ministry of Education, Heilongjiang University of Chinese Medicine, Harbin, China
- 2School of Traditional Chinese Medicine, Guangdong Pharmaceutical University, Guangzhou, China
With an increase in aging populations worldwide, age-related diseases such as Alzheimer’s disease (AD) have become a global concern. At present, a cure for neurodegenerative disease is lacking. There is an urgent need for a biomarker that can facilitate the diagnosis, classification, prognosis, and treatment response of AD. The recent emergence of highly sensitive mass-spectrometry platforms and high-throughput technology can be employed to discover and catalog vast datasets of small metabolites, which respond to changed status in the body. Metabolomics analysis provides hope for a better understanding of AD as well as the subsequent identification and analysis of metabolites. Here, we review the state-of-the-art emerging candidate biomarkers for AD.
Introduction
Alzheimer’s disease (AD) is a progressive neurodegenerative disorder. It is the most common form of dementia (comprises about 60–80% of cases) (Figure 1). AD is characterized by difficulties in memory recall, language, thinking, and other problem-solving abilities that severely affect a person’s ability to perform daily activities (Alzheimer’s Association, 2020). This is a major public health problem that causes devastating physical and economic consequences for patients, their families, and society.
The total estimated cost of dementia care worldwide was ∼$1 trillion in 2018, and this cost is expected to double by 2030 (Peña-Bautista et al., 2020). The World Health Organization estimates ∼50 million people worldwide have dementia and that ∼150 million people will have dementia by 2050 (Peña-Bautista et al., 2020). According to the Alzheimer’s Association, although deaths from other major diseases (e.g., heart disease, cancer, stroke, and infection by the human immunodeficiency virus) have declined significantly or remained approximately identical in the past decade, the number of deaths caused by AD increased by 146% between 2000 and 2018 (Figure 2). This increased prevalence of mortality from AD is due, in large part, to AD becoming a common cause of death among the aging population as well as greater accuracy in diagnosing clinical dementia and recording the cause of demise (Stamate et al., 2019).
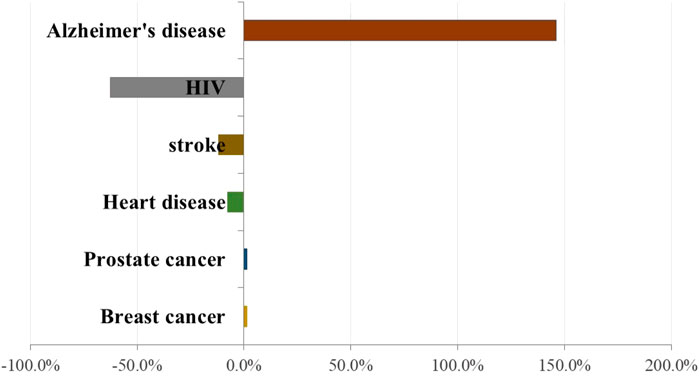
FIGURE 2. Percentage change in reported deaths related to specific diseases between 2000 and 2018. Based on Alzheimer’s disease reporter association in 2020 (Alzheimer’s Association, 2020).
AD is thought to begin ≥20 years before symptom onset and become worse with time (Villemagne et al., 2013; Gordon et al., 2018). The prodromal phase of AD would provide a critical “window of opportunity” for therapeutic intervention to delay AD onset and slow the progress of neurodegeneration (Sperling et al., 2011; Sevigny et al., 2016). Therefore, it is essential to explore more effective preclinical-stage diagnostic methods, which can be used to slow down or prevent dementia onset.
Stages of Alzheimer’s Disease
With respect to AD progression, three broad stages have been outlined in the new guidelines for the diagnosis (Albert et al., 2011; Jack et al., 2011; McKhann et al., 2011) (Figure 3). Phase 1 is preclinical AD (no symptoms and brain change is unnoticeable to the person affected but he/she carries a high risk of developing AD). Phase 2 is mild cognitive impairment (MCI) due to AD (changes in memory and thinking abilities are very mild and do not interfere with daily activities). Phase 3 is dementia due to AD (changes in memory, thinking, and behavioral abilities are clear in various degrees and interfere with daily activities). In addition, the dementia phase can be broken down further into “mild,” “moderate,” and “severe” stages, which reflect the degree to which symptoms interfere with the ability to undertake everyday activities.
With regard to the preclinical phase of AD, a recent study involving rare genetic mutations found that amyloid beta (Aβ) levels were increased significantly, starting 22 years before symptoms were expected to develop (Gordon et al., 2018). Moreover, individuals with these genetic mutations usually develop symptoms at the same (or nearly the same) age as their parent who suffered AD. Meanwhile, glucose metabolism decreases and brain atrophy begins 18 and 13 years before expected symptom onset, respectively (Gordon et al., 2018). Data have shown that the rate of conversion from MCI to AD occurs at 10–15% per year, with 80% conversion by the sixth year of follow-up, approximately (DeCarli, 2003). Therefore, the early diagnosis and identification of AD patients requires careful screening.
In this review, we update the state of diagnostic methods (initial assessment, screening tools, and brain imaging) and biomarker development (based on brain tissue and blood) for preclinical AD. In addition, we make the case for consistency in collection of clinical data for all types of AD biomarkers to allow more thorough and rapid comparisons between studies.
Metabolomics Analysis and Alzheimer’s Disease
The US Food and Drug Administration defines a “biological marker” as an indicator of normal biologic or pathogenic processes or pharmacological responses to a therapeutic intervention. In general, biomarkers in the body are essential not only for the early diagnosis of a disease but also in the assessment of prognosis, classification of disease progression, and disease-modifying treatment (Cummings, 2011; Zhang et al., 2012). Several biochemical processes, such as the metabolism of amyloid precursor protein (APP), phosphorylation of tau protein (p-tau), oxidative stress, impaired energetics, mitochondrial dysfunction, inflammation, dysregulation of membrane lipids, and disruption of neurotransmitter pathways, are affected in AD (de la Monte and Tong, 2014). These changes can be reflected by biomarkers. Therefore, the metabolomics analysis (MA) provides a new method to reveal the nature of a multifactorial disease.
MA is an emerging high-throughput “omics” technology. It is used to comprehensively and simultaneously identify metabolites within a biological system that show altered expression from the genomic, transcriptomic, and proteomic system in a high-throughput pattern (Fiehn, 2002; Beckonert et al., 2007). Recently, MA has been shown to aid discovery of novel biomarkers and explore the potential mechanism of disease by analyzing the entire biological system rather than single metabolites (Snyder et al., 2014). In addition, MA provides detailed biochemical information about drug candidates, therapeutic effects, and side effects during the discovery and development of drugs (Kaddurah-Daouk and Krishnan, 2009), and offers insights into the full complexity of the disease phenotype (Eckhart et al., 2012).
Several MA studies have reported that AD involves disordered metabolism of branched-chain amino acids (Tynkkynen et al., 2018), phosphatidylcholine (Mapstone et al., 2014), glycerophospholipids, and sphingolipids (Orešič et al., 2011; Varma et al., 2018). MA is a new “frontier” in the diagnosis of multifactorial chronic disease because hundreds of metabolites can be detected rapidly and simultaneously (Hassan-Smith et al., 2012).
Assessment of Alzheimer’s Disease in Clinical Practice
Cognition Assessment in Alzheimer’s Disease
The initial diagnosis of AD commonly involves family, close friends, or the general practitioner of a person who is concerned about memory and behavioral changes in daily life. Several diseases featuring delirium, depression, and pain syndromes can present with the similar impairment in memory, perception, and cognitive skills observed in AD. In the early phase of AD, changes in memory (particularly short-term memory) and in behavior and mood (confusion regarding the time of day/familiar places, aphasia, forgotten items, and storing items in inappropriate places) can occur. Then, with aging, these symptoms worsen.
AD can be excluded rapidly in older patients by urine tests, blood tests, and physical examination. In addition, a brief cognitive test may also be conducted, for example, the Mini-Mental State Examination (MMSE) (Andrade-Moraes et al., 2013), to screen for the presence and level of cognitive impairment. Once other likely causes have been excluded, patients are referred to a geriatrician or neurologist for further testing. These specialists will undertake a lengthy clinical test and may require a neuropsychological assessment, which concentrates on the specific domain of cognition. Behavioral and functional assessments may also be implemented (though these are used more commonly in more severe stages of dementia). Behavioral assessment involves investigation of the noncognitive part of dementia (including personality, emotion, and psychotic symptoms) and unusual behaviors as well as obstacles to sleeping, eating, and sexual activity (Fuller et al., 2019). Assessment of noncognitive characteristics has improved the diagnostic accuracy of AD as well as aiding assessment of care requirements and distinguishing between different causes of dementia. The usual assessment approaches and applications for AD in clinical practice are shown in Table 1.
Also, imaging of the brain can be carried out by computed tomography (CT), electroencephalography (EEG), magnetic resonance imaging (MRI), and single-photon emission computed tomography (SPECT). However, these imaging modalities are expensive, time-consuming, and may be available only in major cities as part of research programs.
Diagnostic Biomarkers in Alzheimer’s Disease
Several diagnostic methods for AD are available based on the measurement of the Aβ level in cerebrospinal fluid (CSF) (Hulstaert et al., 1999) and “neurofibrillary tangles” (Caselli et al., 2017), which begin to form years before some symptoms of dementia appear (Perrin et al., 2009). MRI can be used to quantify metabolic abnormalities for measurement of brain atrophy (Reiman and Jagust, 2012). SPECT (Román and Pascual, 2012) and positron emission tomography can be employed to measure the rate of glucose metabolism and Aβ burden (with the radiotracers Pittsburgh compound B and 18F-Florbetapir) (Bruno et al., 2010). Unfortunately, those diagnostic methods are limited because they are invasive, time-consuming, and expensive.
Based on the “amyloid hypothesis,” the Aβ-42 isoform of Aβ has been focused upon as a biomarker for measurement (Hardy and Selkoe, 2002). In CSF, a low Aβ-42 concentration as well as increased total tau (t-tau) and p-tau levels could indicate conversion from MCI to AD (Blennow et al., 2001; Shaw et al., 2009). Recent studies have reported that an increased t-tau level in CSF is observed in neuronal degeneration, but Aβ deposition is detected in normal ageing (Aizenstein et al., 2008). However, Aβ-42 in CSF is not specific to AD because it can be observed in neuronal degeneration and normal ageing. The accuracy of AD diagnosis can be increased ≤90% (in tandem with increasing costs to patients) if analyses of specific protein levels are combined with brain imaging (Cedazo-Minguez and Winblad, 2010). AD can be diagnosed clearly only after death through examination of brain tissue and pathology in an autopsy. Hence, even though use of blood-based biomarkers is attractive, they cannot be used to detect preclinical AD with the requisite sensitivity and specificity (Thambisetty and Lovestone, 2010).
Neuropathologic Evaluation of Alzheimer’s Disease
Accumulation of protein fragments of Aβ (“Aβ plaques”) outside neurons and accumulation of an abnormal form of tau protein (“tau tangles”) within neurons are two of several brain changes associated with AD. Aβ plaques are considered a hallmark feature in the brain of AD patients. They consist mainly of aggregated Aβ peptides of size 4 kDa (ranging from 39 to 43 amino acids). Aβ peptides are the normal breakdown products of APP. The latter is a widely expressed transmembrane protein and cleaved by α-, β-, and γ-secretase. APP is cleaved within the Aβ domain by α-secretase to release the neurotrophic ectodomain of APP into the CSF and prevent Aβ generation (Sisodia, 1992). In addition, β- and γ-secretase act with APP to generate the N and C termini of the Aβ peptide (Haass and Selkoe, 1993). The specific site of cleavage by γ-secretase is variable and generates mostly Aβ-40 peptide (Mann et al., 1996). Approximately 10% of secreted Aβ is Aβ-42, which aggregates readily and acts as a nidus for plaque formation by recruiting Aβ-40 (Jarrett and Lansbury, 1993; Iwatsubo et al., 1994). However, the longer forms of Aβ1–42 are produced in excess and aggregate into oligomers and then form amyloid fibrils in AD (Blennow and Hampel, 2003). Plaque deposition usually begins in the isocortex (frontal, temporal, and occipital lobes of the gray matter of the cortex) and then, in the entorhinal cortex, hippocampal formation, amygdala, insular, and cingulated cortices can be detected (Serrano-Pozo et al., 2011).
Tau tangles (also called neurofibrillary tangles) are swirls or strands of fibers within neurons. They consist mainly of aggregates of microtubule-associated tau protein. The severity of AD symptoms is closely related to the extent of deposition of tau tangles (Arriagada et al., 1992; Serrano-Pozo et al., 2011). In AD, tau protein appears to be abnormally hyperphosphorylated, with phosphate groups attached at specific sites on the protein. Aβ plaques and tau tangles induce damage and death of neurons by interfering with neuron-to-neuron communication and blocking the transport of nutrients and other essential molecules within neurons, respectively (Hyman and Trojanowski, 1997).
It has been proposed that Aβ may begin to accumulate before accumulation of abnormal tau protein and that increasing Aβ accumulation is related to subsequent increases in tau protein (Sato et al., 2018; Hanseeuw et al., 2019). In addition, microglial infiltration, widespread loss of synapses and neurons, and brain shrinkage occur. The toxicity of Aβ and tau proteins can activate microglia (which are the immune system cells in the brain). The main function of microglia is to clear toxic proteins and debris from dead and dying cells. Chronic inflammation may occur if microglia cannot maintain this function. However, shrinkage or atrophy of the brain may develop if synapses and neurons are lost, and normal brain function may be damaged further by the reduced metabolism of glucose (the main fuel in the brain).
Biomarker-Based Metabolomics Analysis
Brain and Blood Samples in Alzheimer’s Disease Studies
Metabolites are intermediates and products of metabolism required for cell growth and are the basis of many other biological components (Mamas et al., 2011). Due to their close relationship to the host’s phenotype, the profile of metabolites shows the current physiological state of a cell and the final result of upstream biological information, which flows from the genome to the transcriptome, proteome, and metabolome (Brink-Jensen et al., 2013). Therefore, metabolites are a relatively more suitable target for phenotype-based research than transcription factors and proteins, both of which are information messengers and executors of biochemical reactions (Enche Ady et al., 2017). An imbalance of metabolic homeostasis is a precursor for disease, so assessment of changes in metabolomes through MA may become a major application for disease description and development of novel therapeutic strategies (Kiehntopf et al., 2013).
A preclinical biomarker is essential for the early diagnosis, stratification, and prevention of AD. The biomarkers for preclinical studies are levels of tau protein and Aβ, as well as structural and functional MRI (Franceschi et al., 2000). The predictive value of these biochemical and imaging markers is very limited because they reflect the final stage of AD (Winblad and Lönneborg, 2014). Hampel and colleagues showed that the concentration of Aβ1–42, t-tau and p-tau in CSF could be used to differentiate people with MCI or AD from healthy older individuals with 80% sensitivity (Hampel et al., 2008). These biomarkers cannot be applied in an early diagnosis of AD because they are detected only when brain functionality is compromised irreversibly (Contino et al., 2013).
CSF and plasma contain the richest source of biomarkers. CSF is an ideal source of various biomarkers for events occurring in AD because it: 1) communicates directly with superficial portions of the brain; 2) it does not pass through the tightly regulated blood–brain barrier (BBB); 3) reflects the biochemical changes that occur in the brain (Aluise et al., 2008; Blennow et al., 2010). However, CSF collection is invasive because it involves a lumbar puncture, which requires patient compliance and can be a challenge in older people with arthritic spines (Henriksen et al., 2014) as well as risking the complications of headaches and meningitis (de Almeida et al., 2011). In addition, patients with low CSF pressure/volume can have a high failure rate of CSF collection (≥20%) and a higher risk of sequelae (Hansson et al., 2006). Besides, CSF collection requires significant clinical skills and use of a sterile method to minimize the risk of headaches, meningitis, epidural abscess, subdural hematoma, and death (Kuntz et al., 1992; Pandian et al., 2004; Martín-Millán et al., 2005). Therefore, biomarker-based brain research and clinical applications for the early diagnosis of AD are limited.
Identification of relevant biomarkers in blood samples has attracted attention in AD research because collection of blood samples is noninvasive, inexpensive, rapid, and influenced less by external factors. Besides, a blood sample is a stable source for repeat measurements. Nevertheless, there is evidence that BBB function is disrupted with aging and that the degree of cognitive impairment results, ultimately, in increased permeability (Baird et al., 2015). This observation reveals the close relationship between the brain and blood. Approximately 500 ml of CSF is absorbed into blood each day, and the small-sized metabolites (e.g., peptides) present in the brain can be detected in plasma or serum (Zipser et al., 2007; Voyle et al., 2016).
Biomarkers Based in Brain Samples in Clinical Research
Lipids have key roles in maintaining normal physiological functions in the body, such as energy storage, signal transduction, maintenance of cell membrane structure, and cell transport (Brügger, 2014). Disordered lipid metabolism is closely related to neurological diseases (e.g., AD and Parkinson’s disease) and affects cognitive function (Gaamouch et al., 2016). Lipid metabolites and pathways strategy (LIPID MAPS; www.lipidmaps.og/) can be used to study the role of lipids. In this way, lipids can be classified into eight main categories: fatty acids, glycerolipids, glycerophospholipids, sphingolipids, saccharolipids, polyketides, prenol lipids, and sterols (Fahy et al., 2009). Glycerophospholipids, sphingolipids, and cholesterol are localized mainly to neuronal membranes and myelin.
Recent studies have indicated that disruption of the metabolism of cholesterol and lipids in the brain is closely related to the generation, deposition, and clearance of Aβ and, finally, leads to neuronal dysfunction (Wellington, 2004; Han, 2010; Song et al., 2012; Wong et al., 2017). A series of studies has claimed that APP processing may occur in cholesterol-rich regions known as “lipid rafts.” Intracellular cholesterol may modulate APP processing directly by regulating secretase activity or affecting the trafficking of secretase or APP (Francis et al., 2002; Edbauer et al., 2003; Li et al., 2003; Cordy et al., 2006). Studies have suggested that intracellular cholesterol regulates the metabolism of Aβ peptides by shifting APP from α- to β-cleavage products, which accumulate as amyloid plaques in the brains of AD patients (Panza et al., 2006). Statins (inhibitors of cholesterol biosynthesis) can reduce the morbidity of AD by ≤70% (Jick et al., 2000; Wolozin et al., 2000). There is strong evidence that AD pathogenesis implicates a genetic variant of apolipoprotein E (ApoE). Apo4 (encoded by the Apoε4 allele) is the highest genetic risk factor for late-onset AD (Corder et al., 1993). In the brain, ApoE is the main cholesterol-carrier protein, which promotes the transfer of cholesterol from astrocytes to neurons. ApoE can also bind Aβ in a genotype-dependent manner. ApoE3 has a higher affinity and greater ability to clear Aβ than that of ApoE4 (Castellano et al., 2011). ApoE2 has a protective effect against AD (Conejero-Goldberg et al., 2014).
Varma and colleagues undertook a study on quantitation of targeted metabolomics in brain tissue using an AbsoluteIDQ® p180 kit (Biocrates Life Sciences). The study cohort was 15 AD patients, 14 healthy controls (HCs), and 15 individuals with asymptomatic Alzheimer’s disease. Two main classes of sphingolipids and glycerophospholipids with 26 metabolites were documented, including sphingomyelin (SM) with acyl residue sum C16:0, C18:1, and C16:1 and hydroxy-sphingomyelin with acyl residue sum C14:1 (SM (OH) C14:1), which could be used to discriminate AD patients and HCs with 83.33% accuracy. Sphingolipids were implicated in biologically relevant pathways in AD: p-tau, Aβ metabolism, calcium homeostasis, acetylcholine biosynthesis, and apoptosis (Varma et al., 2018). Toledo and colleagues reported that increase in levels of SM C16:0 and SM (OH) C14:1 in the temporal cortex of AD patients was related to brain atrophy, cognitive decline, and conversion from MCI to AD (Toledo et al., 2017). Another study of SMs in the brain tissue of AD patients found that carrying ApoE ε4 alleles could lead to increase in levels of ceramide (C22:0) and sulfatide but lower ceramide (C24:0) levels. ApoE ε4 carriers may be important when investigating lipid levels in CSF (Bandaru et al., 2009). On an average of 4.5-year follow-up, longitudinal cohorts of aging and dementia studies found that higher baseline of 3 glycerophospholipids [PC aa C30:0, PC ae C34:0, and PC ae C36:1] and 1 acylcarnitine (C14:2) was present in both the postmortem brain and antemortem blood, which could predict a lower risk for AD. However, only C14:2 was associated with protection against AD, and three glycerophospholipids showed opposite results between brain tissue and blood (Huo et al., 2020). Kaddurah-Daouk and colleagues studied metabolomic changes in autopsy-confirmed AD with 15 AD patients and 15 people not suffering from dementia. Analyses of CSF samples suggested that the level of norepinephrine (NE) and dopamine pathway–related metabolites was reduced significantly in AD cases. In addition, reduced levels of tryptophan, NE, and indoleacetic acid in the CSF of AD cases could be used to distinguish between different groups with 90% accuracy (Kaddurah-Daouk et al., 2011). However, whether a disorder in the NE pathway is unique to AD needs clarification. Some of those studies are shown in Table 2.
The biomarker studies described above suggest that AD research using brain tissue or CSF has made considerable progress. However, obtaining samples is invasive and carries risks for older patients. Even though such samples may offer direct information on brain status, such samples are unlikely to be used for the clinical diagnosis.
Biomarkers Based on Blood Samples in Clinical Research
Use of blood-based biomarkers has garnered increasing attention because blood collection is noninvasive, rapid, and carries little risk compared with that based on CSF collection (Humpel, 2011). Blood is a valid source for repeat measurements, thereby making blood-derived biomarkers for AD is highly sought after (Enche Ady et al., 2017). As stated above, the BBB is disrupted with aging and cognitive impairment in AD development, which results in increased permeability and strengthens the communication between blood and the brain (Baird et al., 2015). CSF is absorbed into the blood circulation each day and small-sized peptides (or even proteins) can be detected in blood upon BBB weakening (Zipser et al., 2007; Henriksen et al., 2014). Even if the lesion occurred in the brain, the blood biomarker also could represent the change in AD (Thambisetty and Lovestone, 2010). Additionally, the smaller metabolites have a more chance pass through a weakened BBB (Voyle et al., 2016).
Several studies have shown that sphingolipids in plasma may be important biomarkers for AD. Ceramides in plasma have been related to memory loss and reduction of hippocampal volume. Mielke and colleagues showed that plasma levels of the ceramides C22:0 and C24:0 in patients with MCI were lower than those of HCs and AD cases. A higher baseline level of C22:0 and C24:0 could predict memory impairment and volume loss of the right hippocampus for MCI patients 1 year later (Mielke et al., 2010). In that study, longitudinal follow-up revealed that, compared with people with the lowest serum ceramide level, a higher baseline serum ceramide level of C16:0 and C24:0 was associated with a higher risk of AD, whereas the SM level was unchanged. In particular, individuals with the lowest ceramide level did not suffer AD (Mielke et al., 2012). Those findings indicated that blood levels of sphingolipids and ceramides could be related to the extent of cognitive decline, disease severity, and brain atrophy. Han and colleagues undertook a cross-sectional study based on 26 AD cases and 26 HCs. They took serum samples and measured changes in sphingolipids levels. The levels of eight sphingolipids in AD patients were lower than those in HCs. However, the levels of two ceramides (C16:0 and C22:0) were higher in AD patients than those in HCs, and the ratio of ceramides-to-sphingolipids was higher than that in HCs (Han et al., 2011). It’s indicated that the disorder levels and constituents of sphingolipids in CSF could be reflected in the blood (Mielke et al., 2010). In addition, the levels of sphingolipids and ceramides changed with aging.
Changes in levels of phospholipids have also been investigated. Whiley and colleagues used MA based on liquid chromatography–mass spectrometry and nuclear magnetic resonance on serum samples from 70 individuals. They found that in AD, levels of three PCs (PC 16:0/20:5, PC 16:0/22:6, and PC 18:0/22:6) were lower than those in HCs, and could be diminished in AD cases (Whiley et al., 2014). That finding was consistent with a subsequent validation study involving a larger cohort (n = 141). After conducting analyses of receiver operating characteristic (ROC) curves, the three PCs, combined with ApoE status, gave an area under the ROC curve of 0.828. The authors did not mention changes in PCs with similar side chains or changes in the choline metabolic pathway, which suggested that a specific type of lipid was destroyed in AD patients. Some scholars have postulated that the same PCs are related to poor memory performance in older individuals without dementia and that phospholipid metabolism is a common factor in AD and age-related cognitive decline (Simpson et al., 2016). Klavins and collaborators reported lower concentrations of 10 phospholipids (PC aa C36:6, PC aa C38:0, PC aa C38:6, PC aa C40:1, PC aa C40:2, PC aa C40:6, PC ae C40:6, lyso PC aa C18:2, propionyl acylcarnitine (C3), and C16:1-OH) in AD patients, MCI cases, and HCs over a 5-year period (Li et al., 2016). They reported a group of lipid metabolites that could be used to differentiate MCI patients or AD cases from HCs within 2–3 years. Similarly, a lower concentration of PC aa C36:6 in MCI and dementia and the ratio of PC aa 34:4/lyso PC C18:2 could clearly distinguish AD cases or MCI patients from HCs with an accuracy of 82–85% (Klavins et al., 2015). Casanova and colleagues could not replicate those findings using a larger cohort with identical methods (Casanova et al., 2016), which emphasizes the importance of reproducibility in different cohorts.
Changes in levels of some low-molecular-weight metabolites (e.g., amino acids and biogenic amines) have been implicated in AD or MCI. Reduction in levels of amino acids with antioxidant properties (Fonteh et al., 2007), changes in concentrations of metabolites associated with mitochondrial function (Maruszak and Żekanowski, 2011), and reduction in the metabolism of medium-chain fatty acids may participate in neurodegenerative hypometabolism, and there may be a supplementary pathway for impaired carbohydrate catabolism or the tricarboxylic acid (TCA) cycle (Ferreira et al., 2010). Also, biomarkers associated with vascular disorders have been detected in the serum of AD patients, such as homocysteine-cysteine disulfide, asymmetric dimethyl-arginine, and phenylalanyl-phenylalanine (Breteler, 2000). Also, disturbed glutamate neurotransmission has been postulated to be a characteristic feature of AD (Lin et al., 2003).
In summary, analyses of the CSF or blood samples of AD patients suggest that amino-acid metabolism, mitochondrial function, neurotransmitter metabolism, and lipid biosynthesis are changed (Trushina et al., 2013). Studies on these low-molecular-weight metabolites (Table 2) may provide valuable biomarkers for AD diagnosis.
Analyses of the Metabolic Pathways Associated With Alzheimer’s Disease
Pathway analyses based on brain and blood samples using MetaboAnalyst 5.0 are shown in Figure 4. Ten metabolic pathways were disordered: glycerophospholipid metabolism; metabolism of linoleic acid; metabolism of alpha-Linolenic acid; sphingolipid metabolism; glycerolipid metabolism; citrate cycle; arginine and proline metabolism; fatty-acid biosynthesis and glutathione metabolism; and purine metabolism. Pathway analyses suggest that lipid metabolism and amino acid metabolism are changed significantly, but purine metabolism is altered only slightly, in AD patients. Recently, studies based on whole-transcript expression on brain tissue indicated stage- and region-dependent deregulation of purine metabolism in AD (González-Domínguez et al., 2014). In addition to intracellular signaling, purines and their products can function as extracellular signals between neurons or neurons and glial cells equipped with appropriate receptors (Ipata et al., 2011). Adenosine (an important neuroprotective factor) is responsible for regulating, integrating, and “fine tuning” neuronal activities and influencing relevant brain functions (sleep and arousal, cognition and memory, and neuronal damage and degeneration) by acting as an extracellular molecule via specific adenosine receptors (Anisur, 2009).
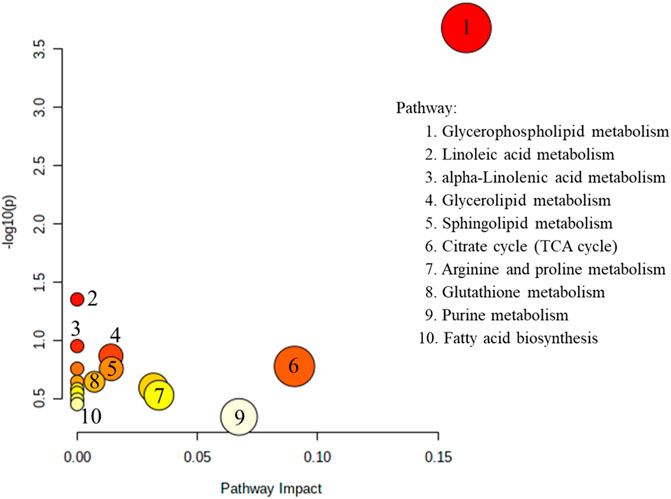
FIGURE 4. The metabolites reported in the brain and blood (Tables 2, 3, excepted small molecular amino acid) view analyzed by MetaboAnalyst 5.0.
A mechanism based on brain biomarkers for AD is shown in Figure 5. In the normal brain, APP is hydrolyzed by α-secretase produced by neurotrophic metabolism to produce sAPPα and P3. However, with aging of the brain, activation of β-secretase and γ-secretase produces Aβ aggregates, which results in neuritic plaques and mitochondrial dysfunction. In addition, disordered metabolism of lipid rafts and Ca2+ imbalance accelerate the phosphorylation of tau protein, and β-secretase is activated through glycogen synthase kinase 3 beta and protein kinase B. These actions result in brain atrophy, neurofibrillary tangles, and, ultimately, AD.
Future Prospects
Current diagnostic methods for AD are slow, expensive, and require several clinical disciplines. The first step is a neuropsychological test, which is suitable only for symptomatic patients. These diagnostic approaches are not suitable in early-stage AD because they only detect if brain function has been damaged irreversibly (Contino et al., 2013). Moreover, AD treatment aims to relieve symptoms by improving neurotransmission and involves use of cholinesterase inhibitors and antagonists of N-Methyl-D-aspartic acid receptors. However, these drugs treat symptoms and do not prevent AD progression. The preclinical stage of AD provides an opportunity to reduce the risk of developing AD. Earlier diagnosis using CSF samples (invasive, expensive, and risk of sequelae) and brain imaging (expensive and limited availability) is difficult in most clinical settings. Nevertheless, biomarkers in CSF samples are used commonly in clinical research. Although using biomarkers in CSF and brain imaging are helpful, a primary screening tool is needed for AD because of the aging societies found worldwide. Therefore, patients who could benefit from these strategies must be identified and treated in a timely manner. Blood-based biomarkers for early screening would be very advantageous because sample collection is easy and cost-effective. MA could have huge potential in AD-biomarker investigation and the early diagnosis of AD. A blood sample has also been increasingly explored for AD biomarker over the last 10 years, due to a reliable panel of biomarker given much hope. The biomarker researches should conduct a longitudinal investigation of subjects, who subsequently then convert to clinical AD. Lipids and amino acids could be candidate biomarkers for the early diagnosis of AD.
The inconsistent results obtained from different studies are a major limitation in the development of AD biomarkers. This problem arises due to a lack of standardized protocols in terms of the collection, storage, preparation of samples, and analytical methodologies (Arnerić et al., 2017). In addition, heterogeneity of participants among different trials is another challenge, which is usually due to differences in age, sex, demographic characteristics, neuropathology, and the different underlying genetics in each individual. A standardized guideline for preanalytical processing of samples and validation of potential biomarkers are needed to overcome these problems. Finally, a clear definition of the preclinical phenotype is necessary for research on modifying the course of AD.
Author Contributions
Y-YW drafted the manuscript; Y-PS, D-HP, XL, and B-YY revised the manuscript; H-XK and Q-HW approved the final manuscript.
Conflict of Interest
The authors declare that the research was conducted in the absence of any commercial or financial relationships that could be construed as a potential conflict of interest.
References
Aizenstein, H. J., Nebes, R. D., Saxton, J. A., Price, J. C., Mathis, C. A., Tsopelas, N. D., et al. (2008). Frequent Amyloid Deposition Without Significant Cognitive Impairment Among the Elderly. Arch. Neurol. 65, 1509–1517. doi:10.1001/archneur.65.11.1509
Ala, T. A., Hughes, L. F., Kyrouac, G. A., Ghobrial, M. W., and Elble, R. J. (2002). The Mini-Mental State Exam May Help in the Differentiation of Dementia With Lewy Bodies and Alzheimer’s Disease. Int. J. Geriat. Psychiatry 17, 503–509. doi:10.1002/gps.550
Albert, M. S., DeKosky, S. T., Dickson, D., Dubois, B., Feldman, H. H., Fox, N. C., et al. (2011). The Diagnosis of Mild Cognitive Impairment Due to Alzheimer’s Disease: Recommendations From the National Institute on Aging-Alzheimer’s Association Workgroups on Diagnostic Guidelines for Alzheimer’s Disease. Alzheimers Dement. 7, 270–279. doi:10.1016/j.jalz.2011.03.008
Aluise, C. D., Sowell, R. A., and Butterfield, D. A. (2008). Peptides and Proteins in Plasma and Cerebrospinal Fluid as Biomarkers for the Prediction, Diagnosis, and Monitoring of Therapeutic Efficacy of Alzheimer’s Disease. Biochim. Biophys. Acta 1782, 549–558. doi:10.1016/j.bbadis.2008.07.008
Alzheimer’s Association (2020). 2020 Alzheimer’s Disease Facts and Figures. Alzheimers Demen. 16, 391–460. doi:10.1002/alz.12068
Andrade-Moraes, C. H., Oliveira-Pinto, A. V., Castro-Fonseca, E., da Silva, C. G., Guimarães, D. M., Szczupak, D., et al. (2013). Cell Number Changes in Alzheimer's Disease Relate to Dementia, Not to Plaques and Tangles. Brain 136, 3738–3752. doi:10.1093/brain/awt273
Anisur, R. (2009). The Role of Adenosine in Alzheimers Disease. Curr. Neuropharmacol. 7, 207–216. doi:10.2174/157015909789152119
Ansoleaga, B., Jové, M., Schlüter, A., Garcia-Esparcia, P., Moreno, J., Pujol, A., et al. (2015). Deregulation of Purine Metabolism in Alzheimer’s Disease. Neurobiol. Aging 36, 68–80. doi:10.1016/j.neurobiolaging.2014.08.004
Arnerić, S. P., Batrla-Utermann, R., Beckett, L., Bittner, T., Blennow, K., Carter, L., et al. (2017). Cerebrospinal Fluid Biomarkers for Alzheimer’s Disease: A View of the Regulatory Science Qualification Landscape from the Coalition Against Major Diseases CSF Biomarker Team. J. Alzheimers Dis. 55, 19–35. doi:10.3233/JAD-160573
Arriagada, P. V., Growdon, J. H., Hedley-Whyte, E. T., and Hyman, B. T. (1992). Neurofibrillary Tangles but Not Senile Plaques Parallel Duration and Severity of Alzheimer's Disease. Neurology 42, 631. doi:10.1212/wnl.42.3.631
Baird, A. L., Westwood, S., and Lovestone, S. (2015). Blood-Based Proteomic Biomarkers of Alzheimer’s Disease Pathology. Front. Neurol. 6, 236. doi:10.3389/fneur.2015.00236
Bandaru, V. V. R., Troncoso, J., Wheeler, D., Pletnikova, O., Wang, J., Conant, K., et al. (2009). ApoE4 Disrupts Sterol and Sphingolipid Metabolism in Alzheimer’s But Not Normal Brain. Neurobiol. Aging 30, 591–599. doi:10.1016/j.neurobiolaging.2007.07.024
Beckonert, O., Keun, H. C., Ebbels, T. M. D., Bundy, J., Holmes, E., Lindon, J. C., et al. (2007). Metabolic Profiling, Metabolomic and Metabonomic Procedures for NMR Spectroscopy of Urine, Plasma, Serum and Tissue Extracts. Nat. Protoc. 2, 2692–2703. doi:10.1038/nprot.2007.376
Blennow, K., and Hampel, H. (2003). CSF Markers for Incipient Alzheimer’s Disease. Lancet Neurol. 2, 605–613. doi:10.1016/s1474-4422(03)00530-1
Blennow, K., Hampel, H., Weiner, M., and Zetterberg, H. (2010). Cerebrospinal Fluid and Plasma Biomarkers in Alzheimer Disease. Nat. Rev. Neurol. 6, 131–144. doi:10.1038/nrneurol.2010.4
Blennow, K., Vanmechelen, E., and Hampel, H. (2001). CSF Total Tau, Aβ42 and Phosphorylated Tau Protein as Biomarkers for Alzheimer’s Disease. Mol. Neurobiol. 24, 087–098. doi:10.1385/mn:24:1-3:087
Borson, S., Scanlan, J. M., Chen, P., and Ganguli, M. (2003). The Mini-Cog as a Screen for Dementia: Validation in a Population-Based Sample. J. Am. Geriatr. Soc. 51, 1451–1454. doi:10.1046/j.1532-5415.2003.51465.x
Breteler, M. M. B. (2000). Vascular Risk Factors for Alzheimer’s Disease: Neurobiol. Aging 21, 153–160. doi:10.1016/s0197-4580(99)00110-4
Brink-Jensen, K., Bak, S., Jørgensen, K., and Ekstrøm, C. T. (2013). Integrative Analysis of Metabolomics and Transcriptomics Data: A Unified Model Framework to Identify Underlying System Pathways. PLoS One 8, e72116. doi:10.1371/journal.pone.0072116
Brügger, B. (2014). Lipidomics: Analysis of the Lipid Composition of Cells and Subcellular Organelles by Electrospray Ionization Mass Spectrometry. Annu. Rev. Biochem. 83, 79–98. doi:10.1146/annurev-biochem-060713-035324
Bruno, D., Feldman, H. H., Jacova, C., Cummings, J. L., Dekosky, S. T., Barberger-Gateau, P., et al. (2010). Revising the Definition of Alzheimer’s Disease: A New Lexicon. Lancet. Neurol. 9, 1118–1127. doi:10.1016/S1474-4422(10)70223-4
Casanova, R., Varma, S., Simpson, B., Kim, M., An, Y., Saldana, S., et al. (2016). Blood Metabolite Markers of Preclinical Alzheimer’s Disease in Two Longitudinally Followed Cohorts of Older Individuals. Alzheimers Demen. 12, 815–822. doi:10.1016/j.jalz.2015.12.008
Caselli, R. J., Beach, T. G., Knopman, D. S., and Graff-Radford, N. R. (2017). Alzheimer Disease. Mayo Clinic Proc. 92, 978–994. doi:10.1016/j.mayocp.2017.02.011
Castellano, J. M., Kim, J., Stewart, F. R., Jiang, H., DeMattos, R. B., Patterson, B. W., et al. (2011). Human apoE Isoforms Differentially Regulate Brain Amyloid-β Peptide Clearance. Sci. Transl. Med. 3, 89ra57. doi:10.1126/scitranslmed.3002156
Cedazo-Minguez, A., and Winblad, B. (2010). Biomarkers for Alzheimers Disease and Other Forms of Dementia: Clinical Needs, Limitations and Future Aspects. Exp. Gerontol. 45, 5–14. doi:10.1016/j.exger.2009.09.008
Conejero-Goldberg, C., Gomar, J. J., Bobes-Bascaran, T., Hyde, T. M., Kleinman, J. E., Herman, M. M., et al. (2014). APOE2 Enhances Neuroprotection Against Alzheimer’s Disease through Multiple Molecular Mechanisms. Mol. Psychiatry 19, 1243–1250. doi:10.1038/mp.2013.194
Contino, M., Cantore, M., Leopoldo, M., and Colabufo, N. A. (2013). Biomarkers for the Early Diagnosis of Alzheimer’s Disease: The Challenge of XXI Century. Adv. Alzheimers Dis. 2, 13–30. doi:10.4236/aad.2013.21003
Corder, E., Saunders, A., Strittmatter, W., Schmechel, D., Gaskell, P., Small, G., et al. (1993). Gene Dose of Apolipoprotein E Type 4 Allele and the Risk of Alzheimer’s Disease in Late Onset Families. Science 261, 921–923. doi:10.1126/science.8346443
Cordy, J. M., Cordy, J. M., Hooper, N. M., and Turner, A. J. (2006). The Involvement of Lipid Rafts in Alzheimer’s Disease (Review). Mol. Membr. Biol. 23, 111–122. doi:10.1080/09687860500496417
Costa, A. C., Joaquim, H. P. G., Forlenza, O. V., Gattaz, W. F., and Talib, L. L. (2020). Three Plasma Metabolites in Elderly Patients Differentiate Mild Cognitive Impairment and Alzheimer’s Disease: a Pilot Study. Eur. Arch. Psychiatry Clin. Neurosci. 270, 483–488. doi:10.1007/s00406-019-01034-9
Cummings, J. L. (2011). Biomarkers in Alzheimer’s Disease Drug Development. Alzheimer’s Demen. 7, e13–e44. doi:10.1016/j.jalz.2010.06.004
de Almeida, S. M., Shumaker, S. D., LeBlanc, S. K., Delaney, P, Marquie-Beck, J., Ueland, S., et al. (2011). Incidence of Post-Dural Puncture Headache in Research Volunteers. Headache 51, 1503–1510. doi:10.1111/j.1526-4610.2011.01959.x
de la Monte, S. M., and Tong, M. (2014). Brain Metabolic Dysfunction at the Core of Alzheimer’s Disease. Biochem. Pharmacol. 88, 548–559. doi:10.1016/j.bcp.2013.12.012
DeCarli, C. (2003). Mild Cognitive Impairment: Prevalence, Prognosis, Aetiology, and Treatment. Lancet Neurol. 2, 15–21. doi:10.1016/s1474-4422(03)00262-x
Eckhart, A. D., Beebe, K., and Milburn, M. (2012). Metabolomics as a Key Integrator for “Omic” Advancement of Personalized Medicine and Future Therapies. Clin. Transl. Sci. 5, 285–288. doi:10.1111/j.1752-8062.2011.00388.x
Edbauer, D., Winkler, E., Regula, J. T., Pesold, B., Steiner, H., and Haass, C. (2003). Reconstitution of γ-Secretase Activity. Nat. Cell Biol. 5, 486–488. doi:10.1038/ncb960
Enche Ady, C. N. A., Lim, S. M., Teh, L. K., Salleh, M. Z., Chin, A.-V., Tan, M. P., et al. (2017). Metabolomic-Guided Discovery of Alzheimer’s Disease Biomarkers From Body Fluid. J. Neuro. Res. 95, 2005–2024. doi:10.1002/jnr.24048
Fahy, E., Subramaniam, S., Murphy, R. C., Nishijima, M., Raetz, C. R. H., Shimizu, T., et al. (2009). Update of the LIPID MAPS Comprehensive Classification System for Lipids. J. Lipid Res. 50, S9–S14. doi:10.1194/jlr.r800095-jlr200
Ferreira, I. L., Resende, R., Ferreiro, E., Rego, A. C., and Pereira, C. F. (2010). Multiple Defects in Energy Metabolism in Alzheimer’s Disease. Curr. Drug Targets 11, 1193–1206. doi:10.2174/1389450111007011193
Fiehn, O. (2002). Metabolomics - The Link Between Genotypes and Phenotypes. Plant Mol. Biol. 48, 155–171. doi:10.1007/978-94-010-0448-0_11
Fonteh, A. N., Harrington, R. J., Tsai, A., Liao, P., and Harrington, M. G. (2007). Free Amino Acid and Dipeptide Changes in the Body Fluids from Alzheimer’s Disease Subjects. Amino Acids 32, 213–224. doi:10.1007/s00726-006-0409-8
Franceschi, C., Bonafè, M., Valensin, S., Olivieri, F., De Luca, M., Ottaviani, E., et al. (2000). Inflamm-Aging. An Evolutionary Perspective on Immunosenescence. Ann. N. Y Acad. Sci. 908, 244–254. doi:10.1111/j.1749-6632.2000.tb06651.x
Francis, R., McGrath, G., Zhang, J., Ruddy, D. A., Sym, M., Apfeld, J., et al. (2002). Aph-1 and Pen-2 are Required for Notch Pathway Signaling, γ-Secretase Cleavage of βAPP, and Presenilin Protein Accumulation. Dev. Cell 3, 85–97. doi:10.1016/s1534-5807(02)00189-2
Fuller, S. J., Carrigan, N., Sohrabi, H. R., and Martins, R. N. (2019). “Current and Developing Methods for Diagnosing Alzheimer’s Disease,” in Neurodegeneration and Alzheimer’s Disease. Editors R. N. Martins, C. S. Brennan, W. B. Fernando, M. A. Brennan, and S. J. Fuller 43–87. doi:10.1002/9781119356752.ch3
Gaamouch, F. E., Jing, P., Xia, J., and Cai, D. (2016). Alzheimer’s Disease Risk Genes and Lipid Regulators. J. Alzheimers Dis. 53, 15–29. doi:10.3233/JAD-160169
González-Domínguez, R., García, A., García-Barrera, T., Barbas, C., and Gómez-Ariza, J. L. (2014). Metabolomic Profiling of Serum in the Progression of Alzheimer's Disease by Capillary Electrophoresis-Mass Spectrometry. Electrophoresis 35, 3321–3330. doi:10.1002/elps.201400196
Gordon, B. A., Blazey, T. M., Su, Y., Hari-Raj, A., Dincer, A., Flores, S., et al. (2018). Spatial Patterns of Neuroimaging Biomarker Change in Individuals from Families with Autosomal Dominant Alzheimer’s Disease: A Longitudinal Study. Lancet Neurol. 17, 241–250. doi:10.1016/s1474-4422(18)30028-0
Graham, S. F., Holscher, C., and Green, B. D. (2014). Metabolic Signatures of Human Alzheimer's Disease (AD): 1H NMR Analysis of the Polar Metabolome of post-mortem Brain Tissue. Metabolomics 10, 744–753. doi:10.1007/s11306-013-0610-1
Haass, C., and Selkoe, D. J. (1993). Cellular Processing of β-amyloid Precursor Protein and the Genesis of Amyloid β-Peptide. Cell 75, 1039–1042. doi:10.1016/0092-8674(93)90312-e
Hampel, H., Bürger, K., Teipel, S. J., Bokde, A. L. W., Zetterberg, H., and Blennow, K. (2008). Core Candidate Neurochemical and Imaging Biomarkers of Alzheimer’s Disease. Alzheimers Demen. 4, 38–48. doi:10.1016/j.jalz.2007.08.006
Han, X., Rozen, S., Boyle, S. H., Hellegers, C., Cheng, H., Burke, J. R., et al. (2011). Metabolomics in Early Alzheimer's Disease: Identification of Altered Plasma Sphingolipidome Using Shotgun Lipidomics. PLoS One 6, e21643. doi:10.1371/journal.pone.0021643
Han, X. (2010). Multi-dimensional Mass Spectrometry-Based Shotgun Lipidomics and the Altered Lipids at the Mild Cognitive Impairment Stage of Alzheimer’s Disease. Biochim. Biophys. Acta 1801, 774–783. doi:10.1016/j.bbalip.2010.01.010
Hanseeuw, B. J., Betensky, R. A., Jacobs, H. I. L., Schultz, A. P., Sepulcre, J., Becker, J. A., et al. (2019). Association of Amyloid and Tau With Cognition in Preclinical Alzheimer Disease. JAMA Neurol. 76, 915–924. doi:10.1001/jamaneurol.2019.1424
Hansson, O., Zetterberg, H., Buchhave, P., Londos, E., Blennow, K., and Minthon, L. (2006). Association Between CSF Biomarkers and Incipient Alzheimer’s Disease in Patients With Mild Cognitive Impairment: A Follow-Up Study. Lancet Neurol. 5, 228–234. doi:10.1016/s1474-4422(06)70355-6
Hardy, J., and Selkoe, D. J. (2002). The Amyloid Hypothesis of Alzheimer’s Disease: Progress and Problems on the Road to Therapeutics. Science 297, 353–356. doi:10.1126/science.1072994
Hassan-Smith, G., Wallace, G. R., Douglas, M. R., and Sinclair, A. J. (2012). The Role of Metabolomics in Neurological Disease. J. Neuroimmunol. 248, 48–52. doi:10.1016/j.jneuroim.2012.01.009
Henriksen, K., O’Bryant, S. E., Hampel, H., Trojanowski, J. Q., Montine, T. J., Jeromin, A., et al. (2014). The Future of Blood-Based Biomarkers for Alzheimer’s Disease. Alzheimers Demen. 10, 115–131. doi:10.1016/j.jalz.2013.01.013
Hughes, C. P., Berg, L., Danziger, W., Coben, L. A., and Martin, R. L. (1982). A New Clinical Scale for the Staging of Dementia. Br. J. Psychiatry 140, 566–572. doi:10.1192/bjp.140.6.566
Hulstaert, F., Blennow, K., Ivanoiu, A., Schoonderwaldt, H. C., Riemenschneider, M., Deyn, P. P. D., et al. (1999). Improved Discrimination of AD Patients Using β-amyloid(1-42) and Tau Levels in CSF. Neurology 52, 1555. doi:10.1212/wnl.52.8.1555
Humpel, C. (2011). Identifying and Validating Biomarkers for Alzheimer’s Disease. Trends Biotechnol. 29, 26–32. doi:10.1016/j.tibtech.2010.09.007
Huo, Z., Yu, L., Yang, J., Zhu, Y., Bennett, D. A., and Zhao, J. (2020). Brain and Blood Metabolome for Alzheimer’s Dementia: Findings from a Targeted Metabolomics Analysis. Neurobiol. Aging 86, 123–133. doi:10.1016/j.neurobiolaging.2019.10.014
Hyman, B. T., and Trojanowski, J. Q. (1997). Editorial on Consensus Recommendations for the Postmortem Diagnosis of Alzheimer Disease from the National Institute on Aging and the Reagan Institute Working Group on Diagnostic Criteria for the Neuropathological Assessment of Alzheimer Disease. J. Neuropathol. Exp. Neurol. 56, 1095–1097. doi:10.1097/00005072-199710000-00002
Ibáñez, C., Simó, C., Barupal, D. K., Fiehn, O., Kivipelto, M., Cedazo-Mínguez, A., et al. (2013). A New Metabolomic Workflow for Early Detection of Alzheimer’s Disease. J. Chromatogr. A 1302, 65–71. doi:10.1016/j.chroma.2013.06.005
Ipata, P. L., Camici, M., Micheli, V., and Tozz, M. G. (2011). Metabolic Network of Nucleosides in the Brain. Curr. Top. Med. Chem. 11, 909–922. doi:10.2174/156802611795347555
Iwatsubo, T., Odaka, A., Suzuki, N., Mizusawa, H., Nukina, N., and Ihara, Y. (1994). Visualization of Aβ42(43) and Aβ40 in Senile Plaques with End-Specific Aβ Monoclonals: Evidence that an Initially Deposited Species is Aβ42(43). Neuron 13, 45–53. doi:10.1016/0896-6273(94)90458-8
Jack, C. R., Albert, M. S., Knopman, D. S., McKhann, G. M., Sperling, R. A., Carrillo, M. C., et al. (2011). Introduction to the Recommendations from the National Institute on Aging-Alzheimer’s Association Workgroups on Diagnostic Guidelines for Alzheimer’s Disease. Alzheimers Demen. 7, 257–262. doi:10.1016/j.jalz.2011.03.004
Jarrett, J. T., and Lansbury, P. T. (1993). Seeding “One-Dimensional Crystallization” of Amyloid: A Pathogenic Mechanism in Alzheimer’s Disease and Scrapie? Cell 73, 1055–1058. doi:10.1016/0092-8674(93)90635-4
Jefferson, A. L., Cosentino, S. A., Ball, S. K., Bogdanoff, B., Leopold, N., Kaplan, E., et al. (2002). Errors Produced on the Mini-Mental State Examination and Neuropsychological Test Performance in Alzheimer’s Disease, Ischemic Vascular Dementia, and Parkinson's Disease. J. Neuropsychiatry Clin. Neurosci. 14, 311–320. doi:10.1176/jnp.14.3.311
Jick, H., Zornberg, G., Jick, S., Seshadri, S., and Drachman, D. (2000). Statins and the Risk of Dementia. Lancet 356, 1627–1631. doi:10.1016/s0140-6736(00)03155-x
Kaddurah-Daouk, R., and Krishnan, K. R. R. (2009). Metabolomics: A Global Biochemical Approach to the Study of Central Nervous System Diseases. Neuropsychopharmacol 34, 173–186. doi:10.1038/npp.2008.174
Kaddurah-Daouk, R., Rozen, S., Matson, W., Han, X., Hulette, C. M., Burke, J. R., et al. (2011). Metabolomic Changes in Autopsy-Confirmed Alzheimer’s Disease. Alzheimers Demen. 7, 309–317. doi:10.1016/j.jalz.2010.06.001
Kiehntopf, M., Nin, N., and Bauer, M. (2013). Metabolism, Metabolome, and Metabolomics in Intensive Care: Is it Time to Move beyond Monitoring of Glucose and Lactate? Am. J. Respir. Crit. Care Med. 187, 906–907. doi:10.1164/rccm.201303-0414ed
Klavins, K., Koal, T., Dallmann, G., Marksteiner, J., Kemmler, G., and Humpel, C. (2015). The Ratio of Phosphatidylcholines to Lysophosphatidylcholines in Plasma Differentiates Healthy Controls From Patients With Alzheimer’s Disease and Mild Cognitive Impairment. Alzheimers Demen. Diagn. Assess. Dis. Monit. 1, 295–302. doi:10.1016/j.dadm.2015.05.003
Kuntz, K. M., Md, E. K., Stevens, J. C., Rn, P. M., Offord, K. P., and Ho, M. M. (1992). Post-Lumbar Puncture Headaches: Experience in 501 Consecutive Procedures. Neurology 42, 1884. doi:10.1212/wnl.42.10.1884
Li, D., Misialek, J. R., Boerwinkle, E., Gottesman, R. F., Sharrett, A. R., Mosley, T. H., et al. (2016). Plasma Phospholipids and Prevalence of Mild Cognitive Impairment and/or Dementia in the ARIC Neurocognitive Study (ARIC‐NCS). Alzheimers Demen. 3, 73–82. doi:10.1016/j.dadm.2016.02.008
Li, T., Ma, G., Cai, H., Price, D. L., and Wong, P. C. (2003). Nicastrin is Required for Assembly of Presenilin/γ-Secretase Complexes to Mediate Notch Signaling and for Processing and Trafficking of β-Amyloid Precursor Protein in Mammals. J. Neurosci. 23, 3272–3277. doi:10.1523/jneurosci.23-08-03272.2003
Lin, A. P., Shic, F., Enriquez, C., and Ross, B. D. (2003). Reduced Glutamate Neurotransmission in Patients with Alzheimer's Disease? An In Vivo 13C Magnetic Resonance Spectroscopy Study. MAGMA 16, 29–42. doi:10.1007/s10334-003-0004-x
Lin, C. N., Huang, C. C., Huang, K. L., Lin, K. J., Yen, T. C., and Kuo, H. C. (2019). A Metabolomic Approach to Identifying Biomarkers in Blood of Alzheimer’s Disease. Ann. Clin. Transl Neurol. 6, 537–545. doi:10.1002/acn3.726
Mamas, M., Dunn, W. B., Neyses, L., and Goodacre, R. (2011). The Role of Metabolites and Metabolomics in Clinically Applicable Biomarkers of Disease. Arch. Toxicol. 85, 5–17. doi:10.1007/s00204-010-0609-6
Mann, D. M. A., Iwatsubo, T., Cairns, N. J., Lantos, P. L., Nochlin, D., Sumi, S. M., et al. (1996). Amyloid β Protein (Aβ) Deposition in Chromosome 14-Linked Alzheimer’s Disease: Predominance of Aβ42(43). Ann. Neurol. 40, 149–156. doi:10.1002/ana.410400205
Mapstone, M., Cheema, A. K., Fiandaca, M. S., Zhong, X., Mhyre, T. R., MacArthur, L. H., et al. (2014). Plasma Phospholipids Identify Antecedent Memory Impairment in Older Adults. Nat. Med. 20, 415–418. doi:10.1038/nm.3466
Martín-Millán, M., Hernández, J. L., Matorras, P., Oterino, A., and González-Macías, J. (2005). Multiple Subdural Haematomas Following Lumbar Puncture. Eur. Neurol. 53, 159–160. doi:10.1159/000086126
Maruszak, A., and Żekanowski, C. (2011). Mitochondrial Dysfunction and Alzheimer's Disease. Prog. Neuropsychopharmacol. Biol. Psychiatry 35, 320–330. doi:10.1016/j.pnpbp.2010.07.004
Matteau, E., Dupré, N., Langlois, M., Jean, L., Thivierge, S., Provencher, P., et al. (2011). Mattis Dementia Rating Scale 2. Am. J. Alzheimers Dis. Other Demen. 26, 389–398. doi:10.1177/1533317511412046
McCrimmon, R. J., Ryan, C. M., and Frier, B. M. (2012). Diabetes and Cognitive Dysfunction. Lancet 379, 2291–2299. doi:10.1016/s0140-6736(12)60360-2
McKhann, G. M., Knopman, D. S., Chertkow, H., Hyman, B. T., Jack, C. R., Kawas, C. H., et al. (2011). The Diagnosis of Dementia Due to Alzheimer’s Disease: Recommendations from the National Institute on Aging-Alzheimer’s Association Workgroups on Diagnostic Guidelines for Alzheimer’s Disease. Alzheimers Demen. 7, 263–269. doi:10.1016/j.jalz.2011.03.005
Meulen, E. F., Schmand, B., van Campen, J. P., de Koning, S. J., Ponds, R. W., Scheltens, P., et al. (2004). The Seven Minute Screen: a Neurocognitive Screening Test Highly Sensitive to Various Types of Dementia. J. Neurol. Neurosurg. Psychiatry 75, 700–705. doi:10.1136/jnnp.2003.021055
Mielke, M. M., Bandaru, V. V. R., Haughey, N. J., Xia, J., Fried, L. P., Yasar, S., et al. (2012). Serum Ceramides Increase the Risk of Alzheimer Disease: The Women’s Health and Aging Study II. Neurology 79, 633–641. doi:10.1212/wnl.0b013e318264e380
Mielke, M. M., Haughey, N. J., Bandaru, V. V. R., Schech, S., Carrick, R., Carlson, M. C., et al. (2010). Plasma Ceramides Are Altered in Mild Cognitive Impairment and Predict Cognitive Decline and Hippocampal Volume Loss. Alzheimers Demen. 6, 378–385. doi:10.1016/j.jalz.2010.03.014
Moms, J. C., Heyman, A., Mohs, R. C., Hughes, J. P., van Belle, G., Fillenbaum, G., et al. (1989). The Consortium to Establish a Registry for Alzheimer’s Disease (CERAD). Part I. Clinical and Neuropsychological Assesment of Alzheimer’s Disease. Neurology 39, 1159. doi:10.1212/wnl.39.9.1159
Naqvi, R. M., Haider, S., Tomlinson, G., and Alibhai, S. (2015). Cognitive Assessments in Multicultural Populations Using the Rowland Universal Dementia Assessment Scale: a Systematic Review and Meta-Analysis. CMAJ 187, E169–E175. doi:10.1503/cmaj.140802
Niedzwiecki, M. M., Walker, D. I., Howell, J. C., Watts, K. D., Jones, D. P., Miller, G. W., et al. (2020). High‐Resolution Metabolomic Profiling of Alzheimer’s Disease in Plasma. Ann. Clin. Transl. Neurol. 7, 36–45. doi:10.1002/acn3.50956
Orešič, M., Hyötyläinen, T., Herukka, S. K., Sysi-Aho, M., Mattila, I., Seppänan-Laakso, T., et al. (2011). Metabolome in Progression to Alzheimer’s Disease. Transl. Psychiatry 1, e57. doi:10.1038/tp.2011.55
Paajanen, T., Hänninen, T., Tunnard, C., Hallikainen, M., Mecocci, P., Sobow, T., et al. (2014). CERAD Neuropsychological Compound Scores are Accurate in Detecting Prodromal Alzheimer's Disease: a Prospective AddNeuroMed Study. J. Alzheimers Dis. 39, 679–690. doi:10.3233/jad-122110
Palmqvist, S., Hansson, O., Minthon, L., and Londos, E. (2009). Practical Suggestions on How to Differentiate Dementia With Lewy Bodies From Alzheimer’s Disease With Common Cognitive Tests. Int. J. Geriat. Psychiatry 24, 1405–1412. doi:10.1002/gps.2277
Pandian, J. D., Sarada, C., Radhakrishnan, V. V., and Kishore, A. (2004). Iatrogenic Meningitis after Lumbar Puncture-A Preventable Health hazard. J. Hosp. Infect. 56, 119–124. doi:10.1016/j.jhin.2003.09.023
Panza, F., D'Introno, A., Colacicco, A. M., Capurso, C., Pichichero, G., Capurso, S. A., et al. (2006). Lipid Metabolism in Cognitive Decline and Dementia. Brain Res. Rev. 51, 275–292. doi:10.1016/j.brainresrev.2005.11.007
Peña-Bautista, C., Roca, M., López-Cuevas, R., Baquero, M., Vento, M., and Cháfer-Pericás, C. (2020). Metabolomics Study to Identify Plasma Biomarkers in Alzheimer Disease: ApoE Genotype Effect. J. Pharm. Biomed. Anal. 180, 113088. doi:10.1016/j.jpba.2019.113088
Peña-Bautista, C., Roca, M., Hervás, D., Cuevas, A., López-Cuevas, R., Vento, M., et al. (2019). Plasma Metabolomics in Early Alzheimer’s Disease Patients Diagnosed With Amyloid Biomarker. J. Proteomics 200, 144–152. doi:10.1016/j.jprot.2019.04.008
Peña-Casanova, J. (1997). Alzheimer's Disease Assessment Scale-Cognitive in Clinical Practice. Int. Psychogeriatr. 9, 105–114. doi:10.1017/s1041610297004778
Perrin, R. J., Fagan, A. M., and Holtzman, D. M. (2009). Multimodal Techniques for Diagnosis and Prognosis of Alzheimer’s Disease. Nature 461, 916–922. doi:10.1038/nature08538
Reiman, E. M., and Jagust, W. J. (2012). Brain Imaging in the Study of Alzheimer’s Disease. Neuroimage 61, 505–516. doi:10.1016/j.neuroimage.2011.11.075
Román, G., and Pascual, B. (2012). Contribution of Neuroimaging to the Diagnosis of Alzheimer’s Disease and Vascular Dementia. Arch. Med. Res. 43, 671–676. doi:10.1016/j.arcmed.2012.10.018
Rossetti, H. C., Lacritz, L. H., Cullum, C. M., and Weiner, M. F. (2011). Normative Data for the Montreal Cognitive Assessment (MoCA) in a Population-Based Sample. Neurology 77, 1272–1275. doi:10.1212/wnl.0b013e318230208a
Sato, C., Barthélemy, N. R., Mawuenyega, K. G., Patterson, B. W., Gordon, B. A., Jockel-Balsarotti, J., et al. (2018). Tau Kinetics in Neurons and the Human Central Nervous System. Neuron 98, 861–864. doi:10.1016/j.neuron.2018.04.035
Serrano-Pozo, A., Frosch, M. P., Masliah, E., and Hyman, B. T. (2011). Neuropathological Alterations in Alzheimer Disease. Cold Spring Harbor Perspect. Med. 1, a006189. doi:10.1101/cshperspect.a006189
Sevigny, J., Chiao, P., Bussière, T., Weinreb, P. H., Williams, L., Maier, M., et al. (2016). The Antibody Aducanumab Reduces Aβ Plaques in Alzheimer’s Disease. Nature 537, 50–56. doi:10.1038/nature19323
Shaw, L. M., Vanderstichele, H., Knapik-Czajka, M., Clark, C. M., Aisen, P. S., Petersen, R. C., et al. (2009). Cerebrospinal Fluid Biomarker Signature in Alzheimer’s Disease Neuroimaging Initiative Subjects. Ann. Neurol. 65, 403–413. doi:10.1002/ana.21610
Simpson, B. N., Kim, M., Chuang, Y.-F., Beason-Held, L., Kitner-Triolo, M., Kraut, M., et al. (2016). Blood Metabolite Markers of Cognitive Performance and Brain Function in Aging. J. Cereb. Blood Flow Metab. 36, 1212–1223. doi:10.1177/0271678x15611678
Sisodia, S. S. (1992). Beta-amyloid Precursor Protein Cleavage by a Membrane-Bound Protease. Proc. Natl. Acad. Sci. 89, 6075–6079. doi:10.1073/pnas.89.13.6075
Snyder, H. M., Carrillo, M. C., Grodstein, F., Henriksen, K., Jeromin, A., Lovestone, S., et al. (2014). Developing Novel Blood-Based Biomarkers for Alzheimer’s Disease. Alzheimers Demen. 10, 109–114. doi:10.1016/j.jalz.2013.10.007
Song, F., Poljak, A., Crawford, J., Kochan, N. A., Wen, W., Cameron, B., et al. (2012). Plasma Apolipoprotein Levels are Associated with Cognitive Status and Decline in a Community Cohort of Older Individuals. PLoS One 7, e34078. doi:10.1371/journal.pone.0034078
Sperling, R. A., Aisen, P. S., Beckett, L. A., Bennett, D. A., Craft, S., Fagan, A. M., et al. (2011). Toward Defining the Preclinical Stages of Alzheimer's Disease: Recommendations From the National Institute on Aging-Alzheimer's Association Workgroups on Diagnostic Guidelines for Alzheimer's Disease. Alzheimers Demen. 7, 280–292. doi:10.1016/j.jalz.2011.03.003
Stamate, D., Kim, M., Proitsi, P., Westwood, S., Baird, A., Nevado‐Holgado, A., et al. (2019). A Metabolite‐Based Machine Learning Approach to Diagnose Alzheimer‐Type Dementia in Blood: Results From the European Medical Information Framework for Alzheimer Disease Biomarker Discovery Cohort. Alzheimers Dement. 5, 933–938. doi:10.1016/j.trci.2019.11.001
Storey, J. E., Rowland, J. T. J., Conforti, D. A., and Dickson, H. G. (2004). The Rowland Universal Dementia Assessment Scale (RUDAS): A Multicultural Cognitive Assessment Scale. Int. Psychogeriatr. 16, 13–31. doi:10.1017/s1041610204000043
Sun, C., Gao, M., Wang, F., Yun, Y., Sun, Q., Guo, R., et al. (2020). Serum Metabolomic Profiling in Patients with Alzheimer Disease and Amnestic Mild Cognitive Impairment by GC/MS. Biomed. Chromatogr. 34, e4875. doi:10.1002/bmc.4875
Thambisetty, M., and Lovestone, S. (2010). Blood-Based Biomarkers of Alzheimer’s Disease: Challenging but Feasible. Biomarkers Med. 4, 65–79. doi:10.2217/bmm.09.84
Toledo, J. B., Arnold, M., Kastenmüller, G., Chang, R., Baillie, R. A., Han, X., et al. (2017). Metabolic Network Failures in Alzheimer's Disease: A Biochemical Road Map. Alzheimers Demen. 13, 965–984. doi:10.1016/j.jalz.2017.01.020
Trushina, E., Dutta, T., Persson, X-M. T., Mielke, M. M., and Petersen, R. C. (2013). Identification of Altered Metabolic Pathways in Plasma and CSF in Mild Cognitive Impairment and Alzheimer’s Disease Using Metabolomics. PLoS One 8, e63644. doi:10.1371/journal.pone.0063644
Tynkkynen, J., Chouraki, V., van der Lee, S. J., Hernesniemi, J., Yang, Q., Li, S., et al. (2018). Association of Branched-Chain Amino Acids and Other Circulating Metabolites With Risk of Incident Dementia and Alzheimer's Disease: A Prospective Study in Eight Cohorts. Alzheimers Demen. 14, 723–733. doi:10.1016/j.jalz.2018.01.003
Varma, V. R., Oommen, A. M., Varma, S., Casanova, R., An, Y., Andrews, R. M., et al. (2018). Brain and Blood Metabolite Signatures of Pathology and Progression in Alzheimer Disease: A Targeted Metabolomics Study. PLoS Med. 15, e1002482. doi:10.1371/journal.pmed.1002482
Villemagne, V. L., Burnham, S., Bourgeat, P., Brown, B., Ellis, K. A., Salvado, O., et al. (2013). Amyloid β Deposition, Neurodegeneration, and Cognitive Decline in Sporadic Alzheimer’s Disease: A Prospective Cohort Study. Lancet Neurol. 12, 357–367. doi:10.1016/s1474-4422(13)70044-9
Voyle, N., Kim, M., Kim, M., Proitsi, P., Ashton, N. J., Baird, A. L., et al. (2016). Blood Metabolite Markers of Neocortical Amyloid-β burden: Discovery and Enrichment Using Candidate Proteins. Transl. Psychiatry 6, e719. doi:10.1038/tp.2015.205
Wang, G., Zhou, Y., Huang, F.-J., Tang, H.-D., Xu, X.-H., Liu, J.-J., et al. (2014). Plasma Metabolite Profiles of Alzheimer’s Disease and Mild Cognitive Impairment. J. Proteome Res. 13, 2649–2658. doi:10.1021/pr5000895
Wellington, C. (2004). Cholesterol at the Crossroads: Alzheimer’s Disease and Lipid Metabolism. Clin. Genet. 66, 1–16. doi:10.1111/j.0009-9163.2004.00280.x
Whiley, L., Sen, A., Heaton, J., Proitsi, P., García-Gómez, D., Leung, R., et al. (2014). Evidence of Altered Phosphatidylcholine Metabolism in Alzheimer’s Disease. Neurobiol. Aging 35, 271–278. doi:10.1016/j.neurobiolaging.2013.08.001
Winblad, B., and Lönneborg, A. (2014). Blood Biomarkers for Alzheimer’s Disease. Eur. Neurol. Rev. 3, 28–30.
Wolozin, B., Kellman, W., Ruosseau, P., Celesia, G. G., and Siegel, G. (2000). Decreased Prevalence of Alzheimer Disease Associated With 3-Hydroxy-3-Methyglutaryl Coenzyme A Reductase Inhibitors. Arch. Neurol. 57, 1439–1443. doi:10.1001/archneur.57.10.1439
Wong, M. W., Braidy, N., Poljak, A., Pickford, R., Thambisetty, M., and Sachdev, P. S. (2017). Dysregulation of Lipids in Alzheimer’s Disease and Their Role as Potential Biomarkers. Alzheimers Demen. 13, 810–827. doi:10.1016/j.jalz.2017.01.008
Zhang, A., Sun, H., Wang, P., Han, Y., and Wang, X. (2012). Modern Analytical Techniques in Metabolomics Analysis. Analyst 137, 293–300. doi:10.1039/c1an15605e
Keywords: alzheimer’s disease, candidate diagnosis biomarker, metabolomics, brain and blood samples, clinical screening
Citation: Wang Y-Y, Sun Y-P, Luo Y-M, Peng D-H, Li X, Yang B-Y, Wang Q-H and Kuang H-X (2021) Biomarkers for the Clinical Diagnosis of Alzheimer’s Disease: Metabolomics Analysis of Brain Tissue and Blood. Front. Pharmacol. 12:700587. doi: 10.3389/fphar.2021.700587
Received: 26 April 2021; Accepted: 08 June 2021;
Published: 21 July 2021.
Edited by:
Francisco Lopez-Munoz, Camilo José Cela University, SpainReviewed by:
Peiyuan Yin, Dalian Medical University, ChinaGustavo Cahli, Federal University of Rio de Janeiro, Brazil
Julie Ann Reisz, University of Colorado, United States
Copyright © 2021 Wang, Sun, Luo, Peng, Li, Yang, Wang and Kuang. This is an open-access article distributed under the terms of the Creative Commons Attribution License (CC BY). The use, distribution or reproduction in other forums is permitted, provided the original author(s) and the copyright owner(s) are credited and that the original publication in this journal is cited, in accordance with accepted academic practice. No use, distribution or reproduction is permitted which does not comply with these terms.
*Correspondence: Hai-Xue Kuang, aHhrdWFuZ0BobGp1Y20ubmV0; Qiu-Hong Wang, cWh3YW5nQGhsanVjbS5uZXQ=