- 1Department of Medical Biotechnology, National Institute of Genetic Engineering and Biotechnology, Tehran, Iran
- 2Department of Systems Biotechnology, National Institute of Genetic Engineering and Biotechnology, Tehran, Iran
Sentrin specific-protease 1 (SENP1) is a protein involved in deSUMOylation that is almost overexpressed in cancer. SENP1 has a determinative role in the activation of transcription programs in the innate immune responses and the development B of and C lymphocytes. We found, SENP1 possibly plays a critical role in immune infiltration and acts as an expression marker in PAAD, ESCA, and THYM. CD4+ T cells, CD8+ T cells, and macrophages were more key-related immune cells, indicating that SENP1 might be introduced as a potential target for cancer immunotherapy. We further showed that dysregulation of SENP1 is powerfully associated with decreased patient survival and clinical stage. Total SENP1 protein also increases in cancer. SENP1 is also controlled by transcription factors (TFs) CREB1, KDM5A, REST, and YY1 that regulates apoptosis, cell cycle, cell proliferation, invasion, tumorigenesis, and metastasis. These TFs were in a positive correlation with SENP1. MiR-138–5p, miR-129-1-3p, and miR-129-2-3p also inhibit tumorigenesis through targeting of SENP1. The SENP1 expression level positively correlated with the expression levels of UBN1, SP3, SAP130, NUP98, NUP153 in 32 tumor types. SENP1 and correlated and binding genes: SAP130, NUP98, and NUP153 activated cell cycle. Consistent with this finding, drug analysis was indicated SENP1 is sensitive to cell cycle, apoptosis, and RTK signaling regulators. In the end, SENP1 and its expression-correlated and functional binding genes were enriched in cell cycle, apoptosis, cellular response to DNA damage stimulus. We found that the cell cycle is the main way for tumorigenesis by SENP1. SENP1 attenuates the effect of inhibitory drugs on the cell cycle. We also introduced effective FDA-Approved drugs that can inhibit SENP1. Therefore in the treatments in which these drugs are used, SENP1 inhibition is a suitable approach. This study supplies a wide analysis of the SENP1 across The Cancer Genome Atlas (CGA) cancer types. These results suggest the potential roles of SENP1 as a biomarker for cancer. Since these drugs and the drugs that cause to resistance are applied to cancer treatment, then these two class drugs can use to inhibition of SENP1.
Introduction
There are seven sentrin specific-protease (SENP) isoforms that operate with SUMO 1–3 (SENP one to three and 5–8). The SENPs are complicated in the deSUMOylation from their substrate proteins and in the maturation of SUMO (Hang and Dasso, 2002). SENP1 (NM_001267594.2 for mRNA or NP_001254523.1 for protein) contains the C-terminal domain that shows catalytic activity and the N-terminal domain that regulates cell localization and substrate specificity.
In mammalian cells, SENPs are differently located. SENP1 (HGNC:17,927) is located at the PML bodies and can commonly act on all the SUMOs one to three precursors (Nayak and Müller, 2014). SENP1 upregulation is a comparatively preliminary event in prostate carcinogenesis. SENP1 enhances the transcriptional activity of AR, eases c-Jun dependent transcription, and induces expression of the cell cycle regulator (Cyclin D1) (Bawa-Khalfe and Yeh, 2010). The overall dynamics of SUMOylation/deSUMOylation may be changed by cell growth, cell cycle conditions, and disease state and SENP proteins might have an important role in cancer growth and be an appropriate target for cancer treatment and therapy. SENP1 deletion has been prevented cell growth by upregulation of CDK inhibitors, such as p21 and p16 in vitro and in vivo growth of colon cancer cells (Xu et al., 2011). Prostate cancer cell growth could be induced, because HIF1α activation and stabilization by SENP1 results in promoted Cyclin D1 and VEGF levels, angiogenesis, and cell growth (Cheng et al., 2006). SENP1 organizes matrix metalloproteinase 2 (MMP2) and MMP9 expressions. This introduces SENP1 in the progression of prostate cancer and suggests SENP1 as a prognostic marker and a therapeutic target for prostate cancer metastasis patients (Wang et al., 2013a). SENP1 can also cause lung, breast, and bladder cancers (Brems-Eskildsen et al., 2010; Wang et al., 2013b; Wang et al., 2016). SENP1 was upregulated in pancreatic ductal adenocarcinoma (PAAD) tissues compared with adjacent normal tissues. The positive dependency of SENP1 with lymph node metastasis and TNM Classification of Malignant Tumors (TNM) stage was exhibited by clinical data. Silencing of SENP1 leads to MMP-9 downregulation, which is fundamental for PAAD cell growth and migration (Ma et al., 2014). SENP1 can be utilized as a molecular target in the discovery of anti-tumor drugs vs. human hepatocellular carcinoma (HCC) metastasis. Zhang et al. indicated SENP1 knockdown leads to inhibition of HGF-induced proliferation and migration of HCC at the same time (Zhang et al., 2016). SENP1 is reported to be involved in hepatocarcinogenesis through the regulation of HIF-1α deSUMOylation in hypoxia conditions. Novel inhibitor development that particularly targets SENP1 may offer a new therapeutic approach to block development, metastasis, and recurrence of HCC (Cui et al., 2017). Increased expression of SENP1 has been also reported in thyroid adenomas (Jacques et al., 2005). These studies suggest SENP1 has a main role in carcinogenesis.
TCGA data collection referred to as the “Pan-Cancer” dataset, presents the scientific community with data on DNA alterations, gene expression, survival status, methylation status, immune infiltration, pharmacogenomics, and protein abundances to detect tumorigenesis effects in different cancer types (Mercatelli et al., 2019). The aim of the present study was the demonstration of SENP1 importance and identification of molecular mechanisms and functions of SENP1 and its interacted proteins in carcinogenesis. There is yet no sufficient Pan-Cancer evidence on the relation between SENP1 and different tumor types based on clinical data. Thus, we conducted a Pan-Cancer study on SENP1 protein. We suggested SENP1 through the effect on cell cycle can result in cancer. The findings of this study indicate the important role of SENP1 in carcinogenesis and supply a potential relationship and a mechanism between SENP1 and tumor-immune interactions. Besides, we represented drugs for SENP1 inhibition. We reported additional references for future experimental studies on SENP1 in cancer.
Material and Methods
Genetic Alterations
In order to study genetic alterations of the SENP1 gene in the Pan-Cancer cohort, including ACC (Adrenocortical carcinoma), BLCA (Bladder Urothelial Carcinoma), BRCA (Breast invasive carcinoma), CESC (Cervical squamous cell carcinoma and endocervical adenocarcinoma), CHOL (Cholangiocarcinoma), COAD (Colon adenocarcinoma), DLBC (Lymphoid Neoplasm Diffuse Large B-cell Lymphoma), ESCA (Esophageal carcinoma), GBM (Glioblastoma multiforme), HNSC (Head and Neck squamous cell carcinoma), KICH (Kidney Chromophobe), KIRC (Kidney renal clear cell carcinoma), KIRP (Kidney renal papillary cell carcinoma), LAML (Acute Myeloid Leukemia), LGG (Brain Lower Grade Glioma), LIHC (Liver hepatocellular carcinoma), LUAD (Lung adenocarcinoma), LUSC (Lung squamous cell carcinoma), MESO (Mesothelioma), OV (Ovarian serous cystadenocarcinoma), PAAD (Pancreatic adenocarcinoma), PCPG (Pheochromocytoma and Paraganglioma), PRAD (Prostate adenocarcinoma), READ (Rectum adenocarcinoma), SARC (Sarcoma), SKCM (Skin Cutaneous Melanoma), STAD (Stomach adenocarcinoma), TGCT (Testicular Germ Cell Tumor), THCA (Thyroid carcinoma), THYM (Thymoma), UCEC (Uterine Corpus Endometrial Carcinoma), UCS (Uterine Carcinosarcoma), and UVM (Uveal Melanoma), we used cBioPortal portal (https://www.cbioportal.org/) (Cerami et al., 2012; Gao et al., 2013) through the “TCGA Pan-Cancer Atlas Studies” in the “Quick select” section and entered “SENP1” as query. The results of the genetic alterations, including mutation type, and CNA (Copy number alteration) in the TCGA tumor samples were identified in the “Cancer Types Summary” module. The “Comparison” module was also applied to gain the survival data on the overall, disease-specific, progression-free, and disease-free survival differences for the TCGA cancers with or without SENP1 genetic alteration. Log-rank p-value < 0.05 was applied.
Gene Expression Analysis
The “Expression analysis-Box Plots” module of the GEPIA2 (Gene Expression Profiling Interactive Analysis, version 2) webserver (http://gepia2.cancer-pku.cn/#analysis) (Tang et al., 2019) was used to determine the expression profile of SENP1 between the tumor tissues (9,664 samples), the normal of tumor tissues (711 samples) from The Cancer Genome Atlas (TCGA), and the normal tissues from the Genotype-Tissue Expression (GTEx) database (4,829 samples) as box plot, under “Match TCGA normal and GTEx data”, log2FC (fold change) cutoff = 1, and p-value cutoff = 0.01 among 33 cancer types.
We also applied the UALCAN portal (http://ualcan.path.uab.edu/analysis-prot.html), to carry out SENP1 expression analysis from the CPTAC (Clinical proteomic tumor analysis consortium) dataset (Chen et al., 2019). The expression level of the total protein between primary tumor and normal tissues was explored through entering “SENP1”. The available CPTAC datasets were included breast cancer, colon cancer, ovarian cancer, clear cell RCC (renal cell carcinoma), UCEC (Uterine corpus endometrial carcinoma), LUAD (Lung adenocarcinoma), and Pediatric Brain Cancer.
We also obtained stage plots of the SENP1 expression in various pathological stages (stage I- IV) of all the TCGA tumors using the “Pathological Stage Plot” module of GEPIA2. The log2 [TPM (Transcripts per million) +1] expression data and AdjP-value<0.05 were used to draw the stage plots.
Regulatory Networks of Sentrin Specific-Protease 1 (Transcription Factors, miRNAs, and Methylation)
Transcription Factors and miRNAs
To study epigenetic alterations of SENP1, TFs with binding ability to the SENP1 promoter were anticipated using Harmonizome (https://maayanlab.cloud/Harmonizome) (Rouillard et al., 2016), including CHEA Transcription Factor Targets, ENCODE Transcription Factor Targets, JASPAR Predicted Transcription Factor Targets, and TRANSFAC Curated Transcription Factor Targets databases. Then for TFs with at least two repetitions, their correlation with SENP1 were calculated using ENCORI (http://starbase.sysu.edu.cn/panCancer.php) (Li et al., 2014) database (p-value < 0.05, R = 1 to −1).
MiRNAs were considered to post-transcriptionally regulate the expression of more than 60% of the human genome through targeting their 3′ untranslated regions (3′UTR) and affect cell proliferation, apoptosis, and invasion in cancer (Yu et al., 2013). MiRNAs that regulate SENP1 were predicted using the miRWalk (http://mirwalk.umm.uni-heidelberg.de/) (Sticht et al., 2018), including miRDB, miRTarBase, and TargetScan databases. Then for miRNAs with at least two repetitions, their correlation with SENP1 was calculated using ENCORI (http://starbase.sysu.edu.cn/panCancer.php) (Li et al., 2014) database (p-value < 0.05, R = 1 to −1).
Methylation
We investigated methylation of SENP1 in the TCGA cancers using the DNMIVD (http://119.3.41.228/dnmivd/index/) (Ding et al., 2020). The correlation between methylation and expression of SENP1 in the TCGA cancers and the correlation of differential survival with methylation state of SENP1 were obtained in the TCGA cancers through the DNMIVD database.
Immune Infiltration Analysis
We also investigated the correlation between SENP1 expression levels and immune cell infiltration levels via TIMER2 (http://timer.cistrome.org/) (Li et al., 2020) across different cancer types. The correlation between SENP1 expression and immune infiltration in 21 immune cell types in the 32 TCGA tumors were visualized using the “Immune-Gene” module of the TIMER2. The TIMER, CIBERSORT, CIBERSORT-ABS, QUANTISEQ, XCELL, MCPCOUNTER, TIDE, and EPIC algorithms were used for immune infiltration estimations. The data were visualized as a heatmap. p-values < 0.05 and −1<R < 1 were obtained viz the Spearman’s rank correlation test and were considered statistically significant.
Survival Prognosis Analysis
The “Survival Map” module of the GEPIA2 (Tang et al., 2019) was applied to assess the OS (Overall survival)and DFS (Disease-free survival) survival map of SENP1 in the 33 TCGA cancers. We classified tumors into two groups: the low-expression and high-expression groups. We also utilized the “Survival Analysis” module of the GEPIA2 for OS and DFS plots using the log-rank test in the hypothesis test. p-value < 0.05 which was considered statistically significant.
Sentrin Specific-Protease 1-Related Gene Enrichment Analysis
We drew a protein-protein interaction network for SENP1 through STRING (Doncheva et al., 2018) App in Cytoscape version 3.8.1 (Kohl et al., 2011; Saito et al., 2012) using the query (“SENP1”) and organism (“Homo sapiens”). In the following, we set the main parameters: minimum required interaction score [“medium confidence (0.4)”], and max additional interactors 100 for obtaining SENP1-binding proteins.
We drew an interactive functional association network for SENP1 using GeneMANIA (Montojo et al., 2010) App in Cytoscape version 3.8.1 (Kohl et al., 2011; Saito et al., 2012) with max resultant genes 100 and max resultant attributes 100. We also applied the “Similar Gene Detection” module of the GEPIA2 to get the top 100 SENP1-correlated targeting genes based on expression in all the TCGA tumor samples. Using 100 correlated genes, we drew an expression-correlated network for SENP1 by STRING (Doncheva et al., 2018) App in Cytoscape version 3.8.1 (Kohl et al., 2011; Saito et al., 2012) that contains members with correlated expression to SENP1.
Then an intersection analysis was conducted using between STRING network and the GeneMANIA network with the correlated network by Venn (http://bioinformatics.psb.ugent.be/webtools/Venn/) and five common genes (UBN1, SP3, SAP130, NUP98, and NUP153) were obtained between two functional networks with expression network. Four genes were common between the correlated network and the GeneMANIA network, two genes were common between the correlated network and STRING network, and one gene was common between three networks. Then correlation of five common genes with SENP1 in the Pan-Cancer cohort were calculated using ENCORI database (http://starbase.sysu.edu.cn/panCancer.php) (Li et al., 2014) (p-value < 0.05, −1<R < 1).
Enrichr (Chen et al., 2013) is a tool for gene enrichment analysis that estimates the importance of overlap between an input list of genes and the gene sets in existing gene libraries. In the end, we uploaded the STRING network, GeneMANIA network, and correlated network to Enrichr for GO ontology and KEGG pathway. We considered p-value < 0.01 statistically significant.
Drug and Pathway Analysis for Sentrin Specific-Protease 1 in the Pan-Cancer Cohort, Virtual Screening, and Molecular Docking
Drug and Pathway Analysis for Sentrin Specific-Protease 1 in the Pan-Cancer Cohort
We also downloaded data for SENP1-associated drugs from PharmacoDB (https://www.pharmacodb.pmgenomics.ca/) (Smirnov et al., 2018; Smirnov et al., 2017), p-value < 0.01 and standardized coefficient −1 to 1. We obtained targets and target pathways of drugs with statistical significance through the Genomics of Drug Sensitivity in Cancer (GDSC) database https://www.cancerrxgene.org/) (Yang et al., 2012). Pathway activity pie chart and heatmap were obtained from GSCALite (http://bioinfo.life.hust.edu.cn/web/GSCALite/) (Liu et al., 2018) in the Pan-Cancer cohort.
Virtual Screening of Potential Compounds Targeting Sentrin Specific-Protease 1
A designing inhibitor for SENP1 can distinguish some clues for the cancer treatment and assist the functional study of SENP1 at the molecular level for experimental biologists. We selected FDA-Approved drugs of the ZINC15 database (http://zinc.docking.org/) (Sterling and Irwin, 2015) as the library. All the drug-like compounds were gotten from the ZINC15 database in SDF format. We used PyRx 0.8 (Dallakyan and Olson, 2015) for structure-based virtual screening (SBVS). We received 2IYC from RCSB Protein Data Bank (https://www.rcsb.org/) (Deshpande et al., 2005) as PDB form. 2IYC converted into PDBQT format. The SDF file was imported in the open babel of PyRx and energy minimization of all the ligands was done. Then all compounds were converted into AutoDock PDBQT format. The search space encompassed with dimensions in Å: center (x, y, z) = (27.9890, −9.5475, −6.1959), dimensions (x, y, z) = (250,000, 250,000, 250,000). Thus, the ligands were docked with SENP1 protein using AutoDock Vina (Trott and Olson, 2010) in PyRx 0.8 (Dallakyan and Olson, 2015).
Molecular Docking by AutoDock Tools
After the virtual screening, we selected twenty top results for molecular docking. Then, before initiating the docking operation, the protein and ligand structure were prepared. Molecular docking of SENP1 was performed with twenty top FDA-Approved compounds from virtual screening results using AutoDock4 software (Morris et al., 2009). Binding position includes the coordinates x center = 33.658 -y center = −16.605 -z center = −0.55 and active site amino acids include TRP465, LEU466, HIS529, GLY531, VAL532, HIS533, TRP534, MET552, GLN596 and CYT603. Polar hydrogen atoms were incorporated by the hydrogen module in AutoDock4 (ADT) for SENP1. Non-polar hydrogens were merged. Gasteiger charges were added. Docking protocol was performed in a grid box consisting of 60 × 60 × 60 (x, y, z) points at the center and with a grid resolution of 0.375 Å to cover SENP1 binding site. Docking was performed with a genetic algorithm. 25 × 105 energy evaluations with a maximum of 27,000 generations number were performed. The population size was fixed at 150 in each run, mutation rate at 0.02, and cross-over the rate at 0.80. For the ligands, the torsions were defined using the “Ligand torsions” menu option of AutoDock Tools. Other parameters were set to default amounts (Taghvaei et al., 2021). In the end, compounds with the lowest binding energy in SBVS and molecular docking by AutoDock4 were visualized using Discovery Studio (Studio, 2008).
Results
Genetic Alterations
At first, we explored genetic alterations of SENP1 in the 33 tumors, including 10,969 tumor samples. The highest genetic alteration rates were for amplification type in DLBC, ACC, and SARC with frequencies of 4.2, 3.3, and 2%, respectively (Figure 1A). Another genetic alteration was mutation with frequencies of 4.5, 4, and 2.7% in UCEC, SKCM, and STAD, respectively (Figure 1A). Among the mutations, missense mutations had the highest frequency (Figure 1B). Deep deletion was also observed with 2% frequency in DLBC. Fusion and multiple alterations were with lower frequency than 1%, (Figure 1A). We observed mutations in the peptidase domain which contains the active site of SENP1 and is essential for SENP1 function (Figure 1B). Generally, across tumor types, the genetic alterations rate was 1.5% and the mutation rate was only 0.8%.
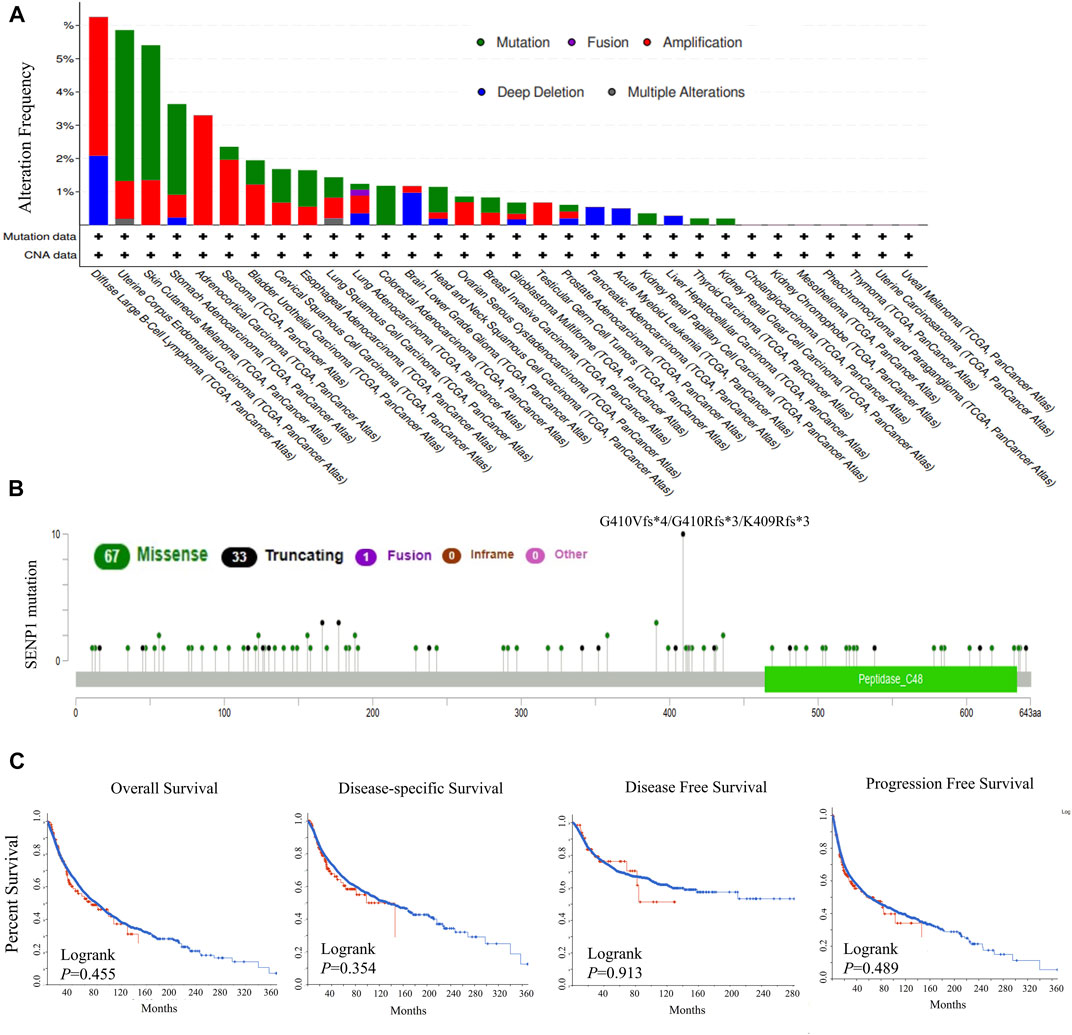
FIGURE 1. Genetic alterations in SENP1, (A) Mutation type and copy number alterations, (B) Mutation types in SENP1 with the most frequency of missense mutations, and (C) OS, DSS, DFS, and PFS in cancers with genetic alterations.
Also, no genetic alteration of SENP1 had effect on the survival prognosis of the TCGA tumors. Because we found disease-free (p = 0.913), disease-specific (p = 0.354), overall (p = 0.355), and progression-free (p = 0.489) survivals were not statistically significant, (Figure 1C). We concluded that the percentage of genetic alterations in the study of SENP1 was negligible.
Differential Expression Analysis Sentrin Specific-Protease 1 Between Tumor and Normal Samples
We carried out differential expression analysis of the 33 TCGA cancers using GEPIA2 (Figure 2A). The results were demonstrated significant differential expression between cancer tissues and para-cancerous tissues. Data of the TCGA shown higher expression of SENP1 in tumor tissues of CHOL, DLBC, ESCA, GBM, PAAD, and THYM than normal tissues, but lower expression of SENP1 in tumor tissues than normal tissues in TGCT (Figure 2A, p-value < 0.01). Other cancers were displayed in Supplementary Figure 1.
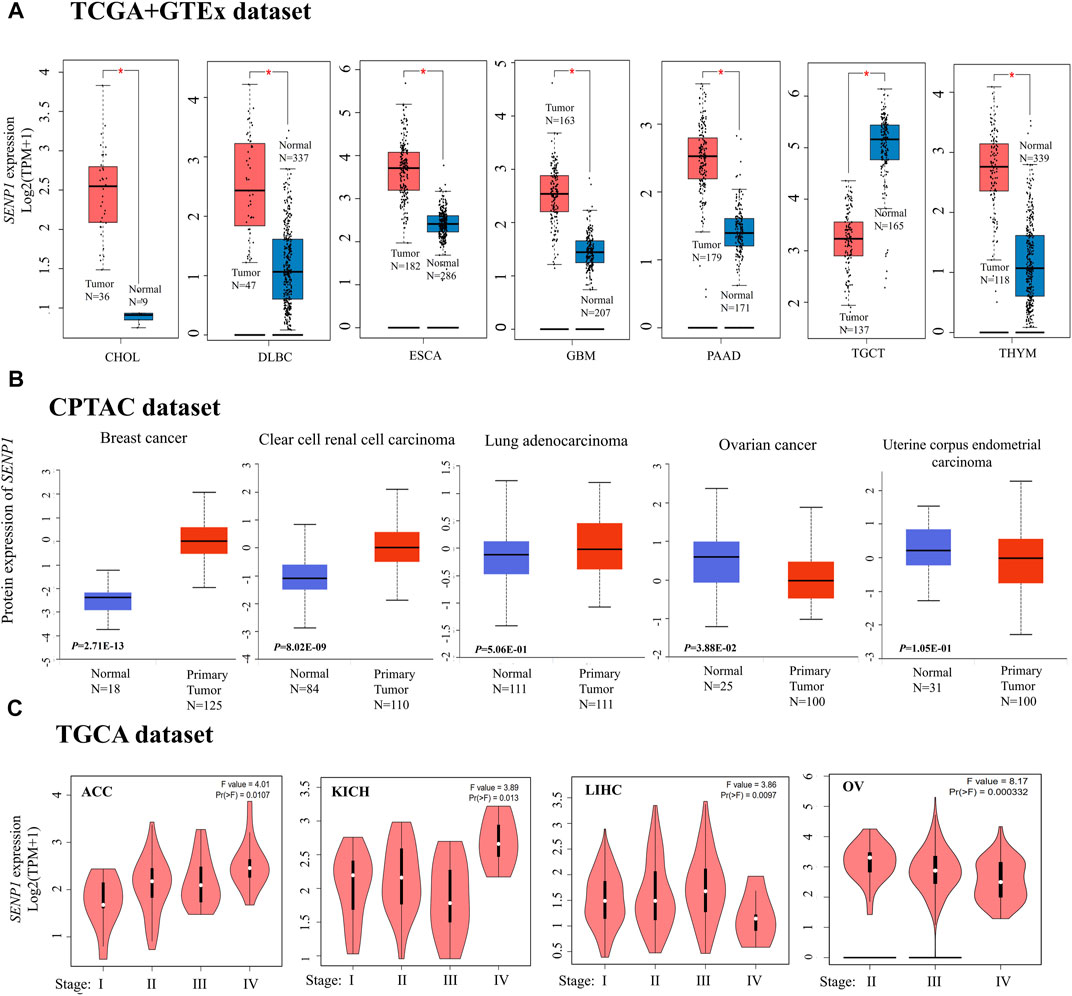
FIGURE 2. SENP1 expression, (A) Box plots of SENP1 expression in tumor tissues compared with normal tissues, (B) SENP1 total protein in tumor tissues compared with normal tissues, and (C)SENP1 expression in the different stages of cancers ACC, KICH, LIHC, and OV.
The CPTAC dataset also indicated higher expression of SENP1 total protein in the primary tissues of breast cancer, clear cell RCC (renal cell carcinoma), and LUAD (Lung adenocarcinoma) (Figure 2B, p < 0. 01) than normal tissues. While we observed higher expression of SENP1 total protein in the normal tissues of ovarian cancer and UCEC (Uterine corpus endometrial carcinoma) compared with primary cancer tissues.
The results of the Pathological Stage Plot of GEPIA2 also displayed the correlation between SENP1 expression and the pathological stages of cancers consisting of only ACC (p < 0.0107), KICH (p < 0.013), LIHC (p < 0.0097), and OV (p < 0.000332) (Figure 2C, p < 0.05). Other cancers were displayed in Supplementary Figure 2.
Transcription Factors and miRNAs Controls Sentrin Specific-Protease 1
Transcription Factors and miRNAs
During mitosis, transcription factors could keep the capacity to bind to their targets and nucleosomal arrays (Festuccia et al., 2019). Due to the significance of SENP1 in cancer, we explored transcription factors and miRNAs that regulate SENP1. We obtained 149 TFs regulating SENP1 in four databases consisting of CHEA, NCODE, JASPAR, and TRANSFAC. Twenty TFs were common in two or more than two databases and were selected. These TFs were displayed as a bubble plot based on correlation in 32 tumors (Figure 3B). CREB1 in THYM (R = 0.903), KDM5A in UVM (R = 0.914), REST in UVM (R = 0.909), and YY1 in UVM (R = 0.908) had the most positive correlation with SENP1. CREB1 regulates apoptosis, cell cycle, cell proliferation, invasion, carcinogenesis, and metastasis and decreases drug sensitivity in vitro (Ye et al., 2017). CREB1 targets the Bcl2 family, Cyclins, and Egr-1 (early growth response 1 (Yan et al., 2018). KDM5A inhibition causes anticancer activity through impaired cell cycle and senescence via regulation of p16 and p27. KDM5A leads to inhibition of pRB function and P53 signaling in the cell cycle. KDM5A can be a target to treat cancer because it triggers tumorigenesis (Shokri et al., 2018). YY1 is an important regulator in tumorigenesis that its expression was disturbed in many tumors. YY1 can cause activation of oncogenes and suppression of tumor suppressors (Arribas et al., 2015). Overexpression and activation of YY1 are related to uncontrollable cell proliferation, resistance to apoptosis stimulators, carcinogenesis, and metastasis (Gordon et al., 2006). REST causes apoptosis, decreasing cell proliferation and Bcl2 expression, and increasing drug sensitivity (Lv et al., 2010). Then SENP1 interacts with these TFs and can through them contribute in the carcinogenesis.
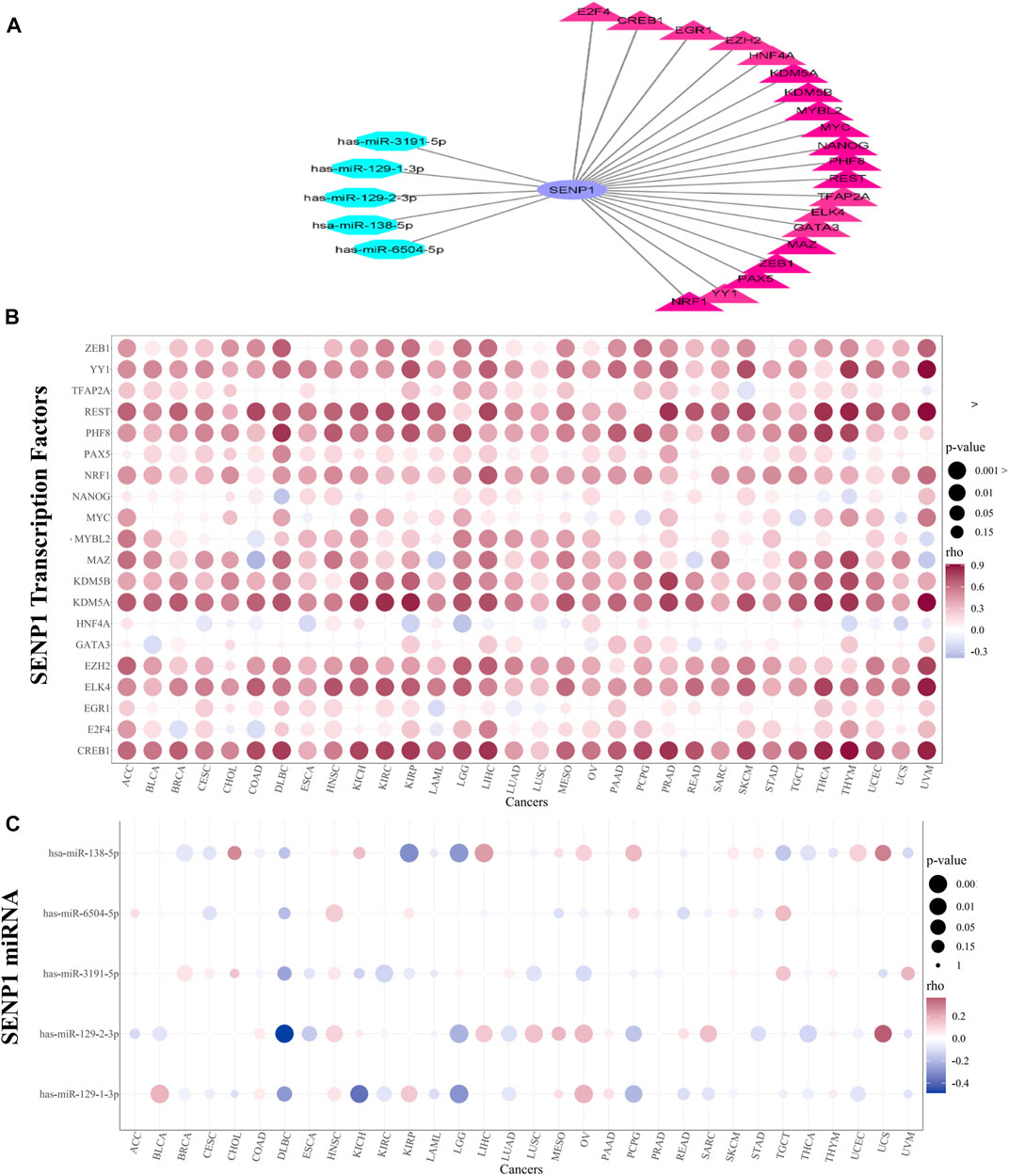
FIGURE 3. TF and miRNA regulatory networks. (A) TF and miRNA network, (B) Bubble plot of TFs alterations in 32 cancers, and (C) Bubble plot of miRNAs alterations in 32 cancers.
miRNAs can regulate gene expression at the post-transcriptional level and affect cell proliferation, apoptosis, and invasion in cancer (Li et al., 2018). MiRNAs that can control SENP1 were also gotten from miRWalk (consisting of miRDB, miRTarBase, and TargetScan). From eight miRNAs, five miRNAs (has-miR-129-1-3p, has-miR-2-3p, has-miR-138–5p, has-miR-6504–5p, and has-miR-3191–5p) were common in two or more of two databases that have been shown as bubble plot in Figure 3C. The TF and miRNA network were drawn in Cytoscape and were demonstrated in Figure 3A. We concluded that miR-138–5p in Kidney Renal Papillary Cell Carcinoma (KIRP) (R = -0.369), miR-129-1-3p in Kidney Chromophobe (KICH) (R = -0.304), and miR-129-2-3p in Lymphoid Neoplasm Diffuse Large B-cell Lymphoma (DLBC) (R = -487) have the most inhibitory effects on SENP1 expression (Figure 3C).
The previous studies reported the miRNA-138–5p can inhibit the progression of lung adenocarcinoma, and (head and neck squamous cell carcinoma (HNSCC), and prostate, colorectal, breast, ovarian, and gastric cancers, (Zhang et al., 2020). The miRNA-129-1-3p can prevent tumor progression in glioblastoma, and colorectal, and gastric cancers. The miRNA-129-2-3p can also inhibit tumor progression in hepatocellular carcinoma (HCC), and glioblastoma, and colorectal, breast, gastric cancers (Yu et al., 2013).
The inhibition of SENP1 by miRNA-129-1-3p and miRNA-129-2-3p were reported in any study. Since these miRNAs inhibit tumorigenesis and affect SENP1 expression, then we can use their overexpression for inhibition of SENP1.
Methylation
Methylation of SENP1 in 32 cancers were also considered in the DNMIVD. SENP1 regulation in cancer was not affected by methylation. Only in BRCA (p-value = 0.0154,259, R = −0.182,279) and COAD (p-value = 0.00935,263, R = 0.103,032) were observed significant correlation that were no significant change in the methylation level, p-value < 0.05, Spearman_r between one and −1. We concluded promoter DNA methylation cannot control the expression of SENP1 in cancer.
Sentrin Specific-Protease 1 Expression is Correlated With Immune Infiltration in Pancreatic Adenocarcinoma, Esophageal Carcinoma, Lymphoid Neoplasm Diffuse Large B-cell Lymphoma, and Thymoma
One of the cancer hallmarks is the immune reaction. Solid tumors are generally infiltrated with immune cells consisting of B and T lymphocytes, macrophages, eosinophils, neutrophils, mast cells, NK-T cells, natural killer (NK) cells, dendritic cells (DCs), etc. Infiltration of cells is responsible for chronic inflammation. Increased data demonstrated that local inflammation powerfully induces cancer development (Pagès et al., 2010).
SENP1 expression was demonstrated significant positive correlations with B cells in 31 cancers, CD4+ T cells in 31 cancers, neutrophils in 31 cancers, macrophage cells in 30 cancers, DC in 29 cancers, CD8+ T cells in 29 cancers, Tregs in 27 cancers, NK cells in 26 cancers, mast cells in 24 cancers, and monocytes in 23 cancers. We observed a general pattern from a positive correlation between SENP1 expression and immune cell infiltration. SENP1 expression level was shown significant positive correlations with abundances of infiltrating immune cells, including B cells, CD4+ T cells, CD8+ T cells, DC cells, NK cells, Tregs, monocytes, and macrophages. Then SENP1 can play an important role in cancer. Also, we obtained the top ten of immune cells with significantly positive correlations that among they, immune cells with correlation≥4 were consisting of CD4+ T cells, CD8+ T cells, monocytes, and macrophages in PAAD, DC cells in ESCA, NK cells in DLBC, and monocytes in THYM according to all the applied algorithms. Among these cells, CD4+ T cells, CD8+ T cells, and macrophages were more key-related immune cells that have been displayed in Figure 4, indicating that SENP1 can increase the tumor-infiltrating immune cell abundance in cancer. Other cells were displayed in Supplementary Figure 2. According to our results, SENP1 expression had significant expression changes and higher immune infiltration in PAAD, ESCA, and THYM.
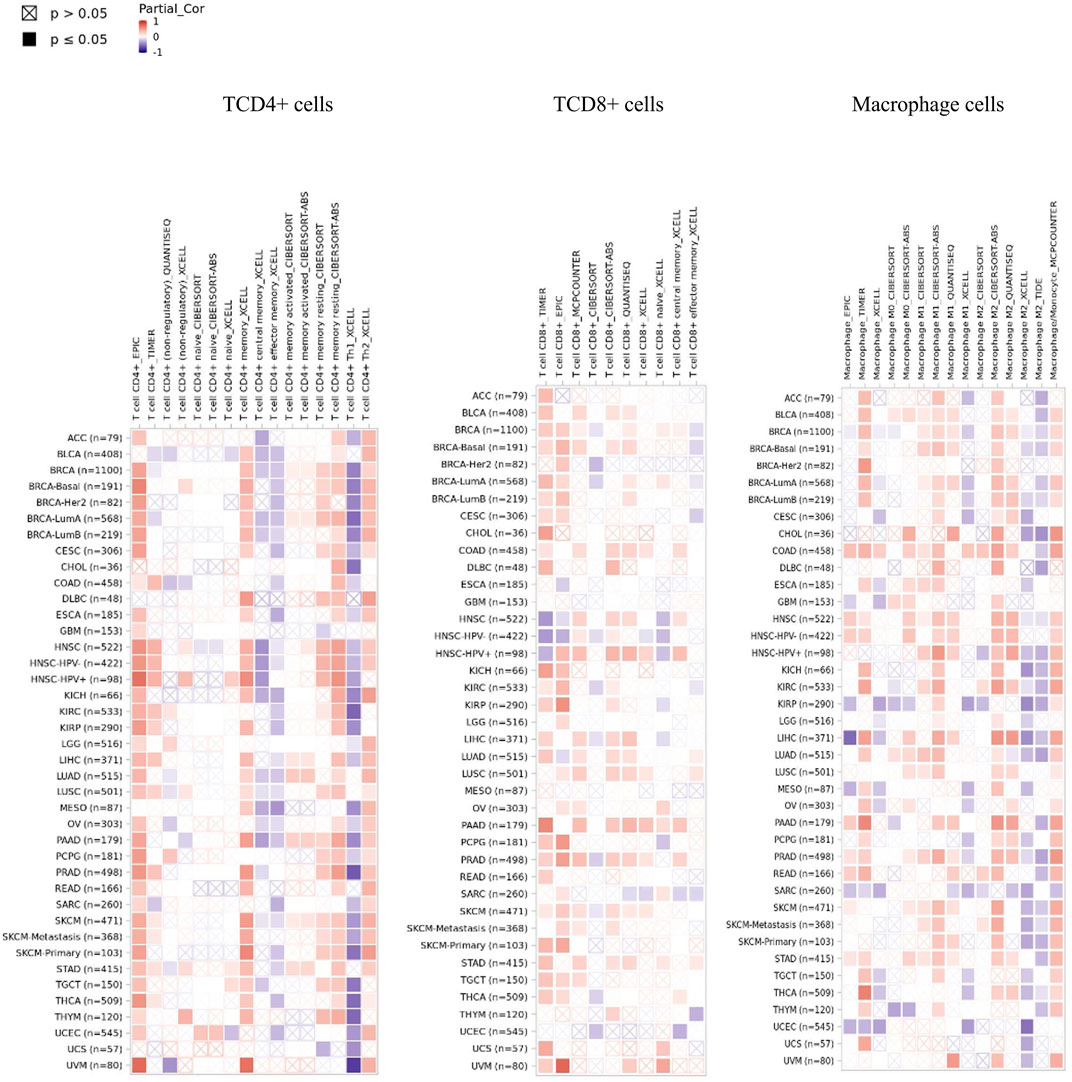
FIGURE 4. Heatmap of immune cells with positive significant correlation with SENP1 in the Pan-Cancer cohort.
Survival Analysis Data
GEPIA2 also was applied to obtain survival of the TCGA tumors. In the survival analysis, the cancer cases were divided into high-expression and low-expression groups based on the expression levels of SENP1 and the correlation of SENP1 expression with the prognosis of patients of various tumors, principally through the TCGA datasets were explored. High expression of SENP1 was associated with a significantly worse prognosis of OS in ACC (p = 0.0081, HR = 2.9), KIRP (p = 0.023, HR = 2), LIHC (p = 0.013, HR = 1.6), and THCA (p = 0.031, HR = 3.2) of the TCGA (Figure 5A). DFS data analysis has represented a correlation between high expression of SENP1 and significantly worse prognosis in the ACC (p = 0.00043, HR = 3.4), KICH (p = 0.028, HR = 4.8), LIHC (p = 0.026, HR = 1.2), and MESO (Figure 5B, p = 0.011, HR = 2.1). Moreover, low SENP1 expression was associated with poor OS prognosis of KIRC (Figure 5B, p = 0.0095, HR = 0.67). These results propose the potential role of SENP1 as a marker for cancer survival. Other tumors with p > 0.05 were shown in the Supplementary Figure 4.
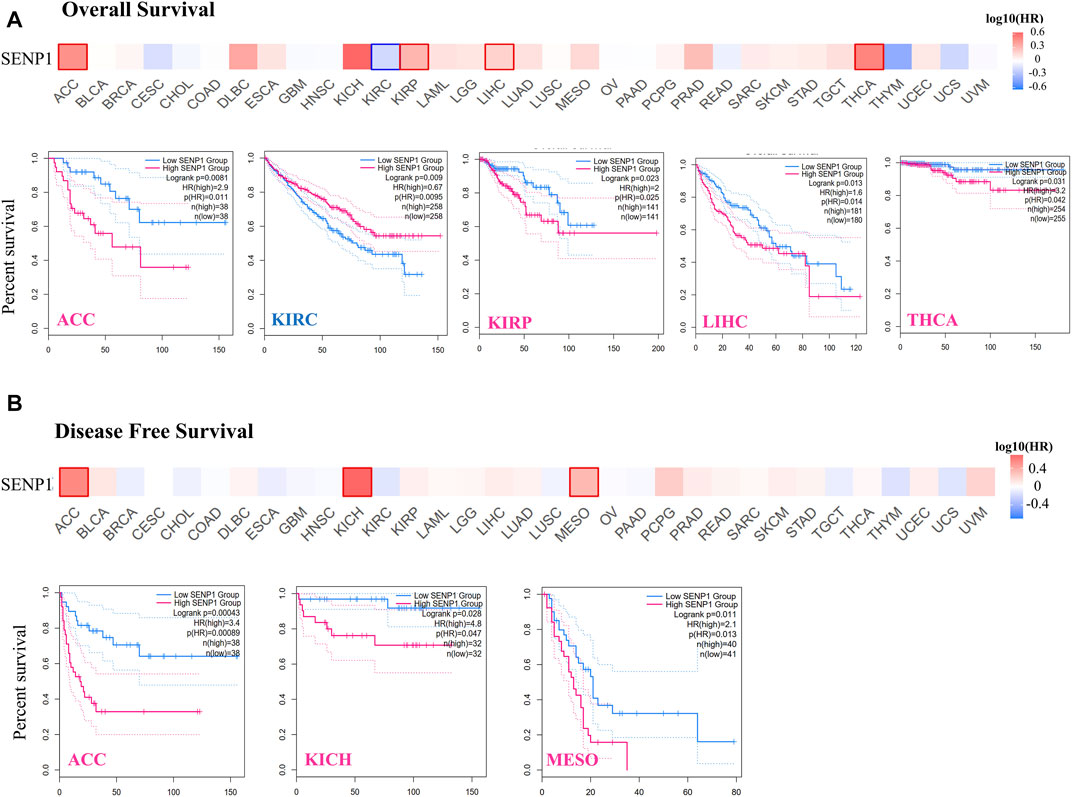
FIGURE 5. Survival of patients with the altered SENP1 expression in various tumors, (A) Overall survival of SENP1 in ACC, KIRC, KIRP, LIHC, and THCA, and (B) Disease-Free Survival of SENP1 in ACC, KICH, and MESO.
Enrichment of Sentrin Specific-Protease 1-Binding Proteins Networks
Due to the importance of SENP1 in cancer. We drew the expression-correlated network for SENP1 (Figure 6A). We also drew functional networks of STRING and GeneMANIA (Supplementary Figure 5 and Supplementary Figure 6). The five genes: UBN1, SP3, SAP130, NUP98, and NUP153 were common between functional networks and expression-correlated networks (Figure 6B, Venn diagram). Correlation heatmap of these genes with SENP1 has been shown in the Pan-Cancer cohort in Figure 6C. We observed a correlation with all tumor samples in 32 TCGA cancers.
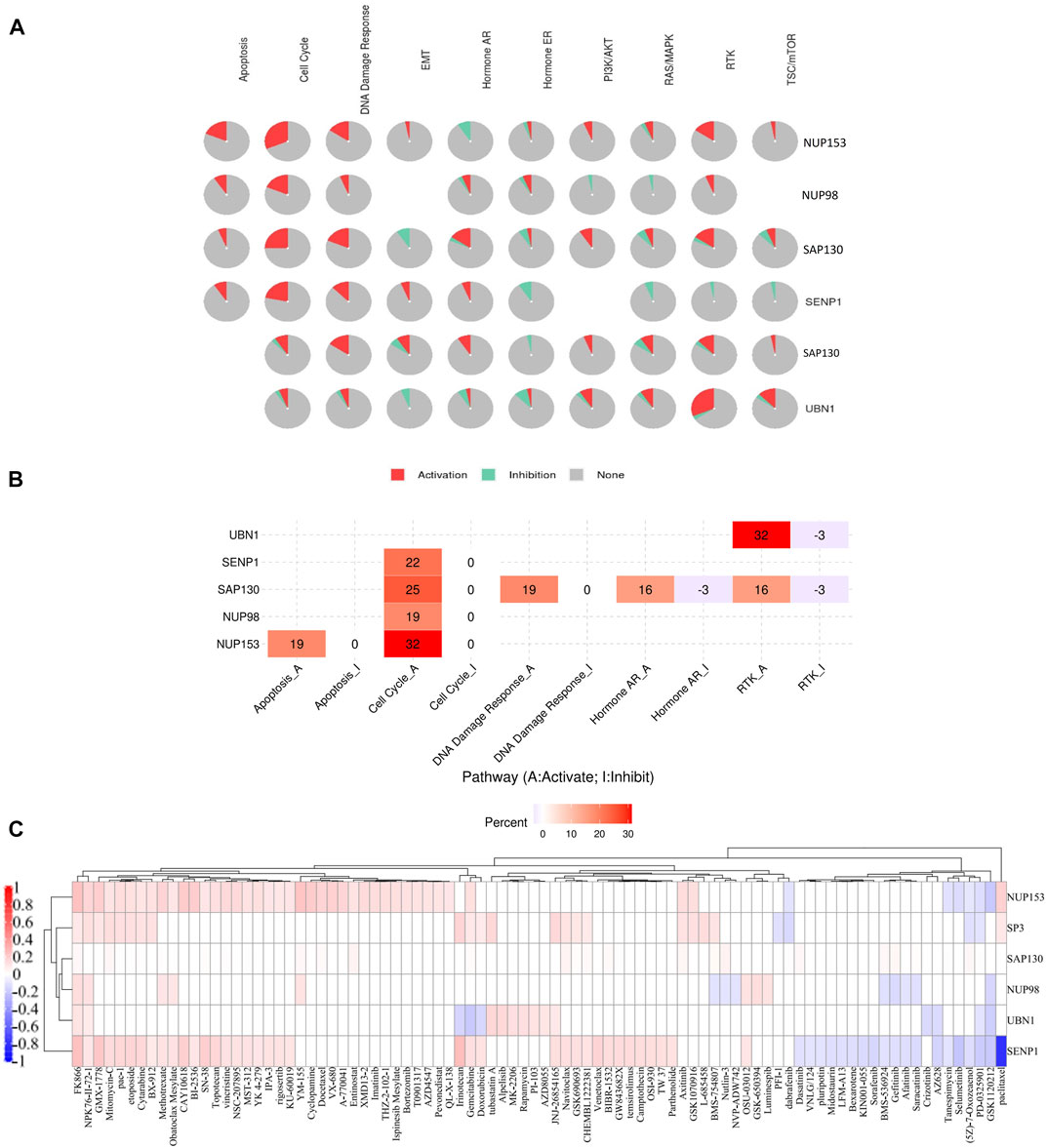
FIGURE 6. SENP1 network. (A) SENP1-correlated network, (B) Venn diagram of SENP1-binding genes of STRING, and GeneMANIA with SENP1-correlated genes, and (C) Correlation heatmap of common five genes with SENP1 in 32 cancers, (D) Go ontology (BP), and KEGG pathway of SENP1-correlated network.
KEGG pathway enrichment results shown “Cell Cycle” and “Apoptosis” might be complicated in the effect of SENP1 on tumorigenesis. The GO enrichment analysis data also represented that these three networks involved in the cellular response to DNA damage stimulus, regulation of the apoptotic process, and regulation of cell cycle (Figure 6D and Supplementary Figure 5 and Supplementary Figure 6). Based on these results, we concluded the potential involvement of SENP1 in tumor progression.
Drug and Pathway Activity Analysis Shown a Role of Sentrin Specific-Protease 1 in the Pan-Cancer Cohort
Drug and Pathway Activity Analysis
In the next step to more study and the survey of SENP1 function during cancer, we explored the correlation between expression of five common genes and SENP1 with activation or inhibition of signaling pathways.
Pathway activity results showed SENP1, SAP130, NUP98, and NUP153 are associated with activation of cell cycle, and SAP130 & UBN1 are associated with activation of RTK signaling. NUP153 is also associated with the strong activation of apoptosis, and SAP130 is associated with the strongest activation of DNA Damage Response, Hormone AR, and RTK signaling (Figures 7A,B).
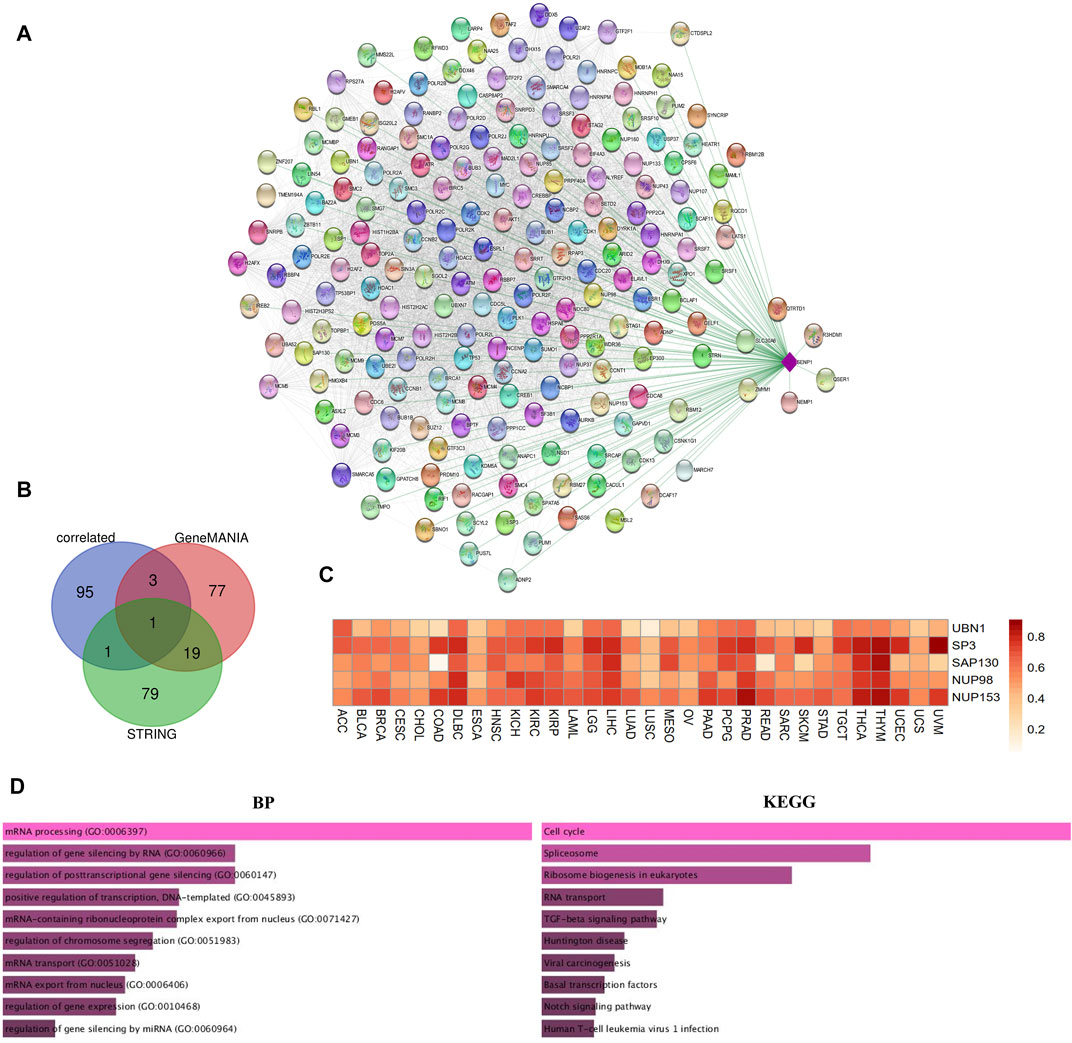
FIGURE 7. The role of SENP1 in famous cancer related-pathways (GSCALite), and the drug resistance analysis of common genes based on GDSC drug data. (A) Pie chart of pathway activity, (B) Heatmap of pathway activity, and (C) Heatmap of drugs correlated with six genes NUP153, SP3, SAP130, NUP98, UBN1, and SENP1.
After receiving a list of drugs related to the SENP1 from the PharmacoDB database, we obtained target and the target pathways of drugs with p < 0.01. After the initial investigation, we deleted drugs without target and target pathway, selected standard coefficient >0.1 and < −0.1, and then we displayed drugs as a heatmap in Figure 7C. Among them, SENP1 was sensitive to thirty-five drugs and was resistant to twenty drugs.
Among these drugs, SENP1 is sensitive to apoptosis regulators: Venetoclax, Navitoclax, pac-1, Obatoclax Mesylate, TW 37, XMD 13–2, and YM-155. Venetoclax (Tse et al., 2008) through Bcl2, Navitoclax through Bcl2, Bcl-XL, and Bcl-W, Obatoclax Mesylate through Bcl2, Bcl-XL, Bcl-W, and Mcl-1, pac-1 through Procaspase-3 and Procaspase-7, TW 37 through Bcl2, Bcl-XL, and Mcl-1, XMD13-2 through RIPK1, and YM-155 through BIRC5 (Saleem et al., 2013) regulate apoptosis. Then SENP1 may regulate apoptosis.
Mutations of RTK-signaling often cause cell transformation which was observed in a widevariety of cancers (Regad, 2015). SENP1 is sensitive to regulators of RTK-signaling pathway: Axitinib, AZD4547, BMS-754807, Crizotinib, LFM-A13, and Sorafenib. Axitinib with targeting PDGFR, KIT, and VEGFR, AZD4547 with targeting FGRF1, FGFR2, and FGFR3, BMS-754807 with targeting IGF1R and IR, Crizotinib with targeting MET, ALK, and ROS1, LFM-A13 with targeting VEGFR1, VEGFR2, VEGFR3, CSF1R, FLT3, and KIT, OSI-930 with targeting KIT, and Sorafenib with targeting PDGFR, KIT, VEGFR, and RAF regulate RTK-signaling pathway. Then SENP1 may regulate the RTK-signaling pathway.
SENP1 is sensitive to cell cycle regulators, including Bi-2536, CAY10618, GW843682X, NSC-207895, and Rigosertib. Bi-2536 with effect on PLK1-3, CAY10618 with effect on PPM1D, GW843682X with effect on PLK1, NSC-207895 with effect on PLK3, and Rigosertib with effect on CDK2, CDK7, and CDK9 regulate the cell cycle. Then we suggested SENP1 also regulates the cell cycle. These findings distinguish that SENP1 is associated with alterations of multiple oncogenic pathways.
SENP1 overexpression also led to resistance to drugs Paclitaxel, GSK1120212, PD-0325901 (5Z)-7-Oxozeaenol, Selumetinib, Tanespimycin, AZ628, Saracatinib, Afatinib, Gefitinib, BMS-536924, Sorafenib, KIN001-055, Bexarotene, LFM-A13, Midostaurin, Pluripotin, VNLG/124, and Dasatinib.
Virtual Screening of Sentrin Specific-Protease 1 With FDA-Approved Drugs
We carried out SBVS using the docking method on FDA-Approved drugs of the ZINC15 database to recognize new SENP1 inhibitors. Molecular docking is the best method to quickly estimate the binding conformations of ligands that are energy-efficient to interact with a pharmacological receptor site and has obtained popularity as a tool to store time and costs in the pipeline of drug discovery and development (Kaushik et al., 2020; Caliskan et al., 2021). ZINC15 joins biological activities of drugs, gene products, and natural products with commercial availability (Irwin and Shoichet, 2005). We supposed that, if we manage to break through the interactions that are activated, we might model a strategy to cure the disease. For this purpose, we considered SENP1 with activated interactions in the tumor state as potential drug targets. After the molecular docking was complete, the top twenty ranked results were docked using autodock4 (Table 1).
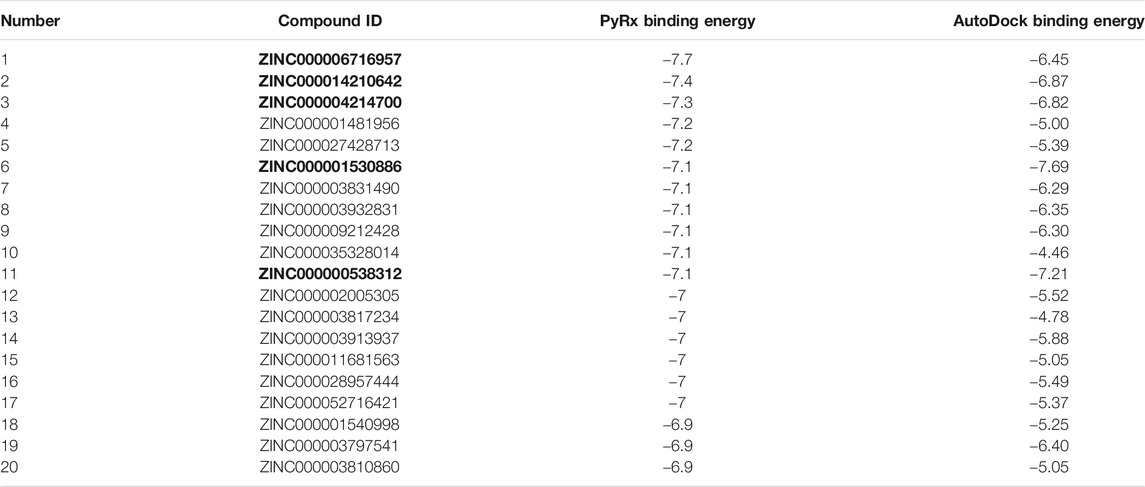
TABLE 1. PyRx binding energy and AutoDock binding energy of twenty top FDA-Approved results of SBVS between FDA-Approved drugs of ZINC15 and SENP1. The bold values are five compounds azilsartan, telmisartan, nilutinib, paliperidone and risperdal.
Molecular Docking via AutoDock Tools
After SBVS, the compounds ranked with the lowest binding energy. Twenty top results of SBVS were used for molecular docking using AutoDock4 (Table 1). Then, among the top Twenty compounds, the compounds with the lowest SBVS binding energy and the lowest AutoDock binding energy were included Nilotinib (ZINC000006716957), Azilsartan medoxomil (ZINC000014210642), Paliperidone (ZINC000004214700), Telmisartan (ZINC000001530886), and Risperdal (ZINC000000538312). Nilotinib is chronic myeloid leukemia (CML) tyrosine kinase inhibitor that was also introduced as an inhibitor of COVID-19 (Rahman et al., 2020; Singh et al., 2020). Paliperidone that can inhibit COVID-19 (Gul et al., 2020), was also demonstrated can use to Huntington treatment. Telmisartan can also be used in COVID-19 treatment (Barage et al., 2020), and Risperidal can be used for MALT1-driven cancer or autoimmune diseases (Zhang et al., 2019).
In the end, the best pose of the five best AutoDock results was imported into Discovery Studio (Studio, 2008) to be visualized as a 2D structure (Figure 8). We observed which Azilsartan medoxomil constitutes the most bonds especially hydrogen bonds with the active site of SENP1. Telmisartan also constitutes the most bonds with the active site of SENP1 especially van der Waals bonds (Figure 8).
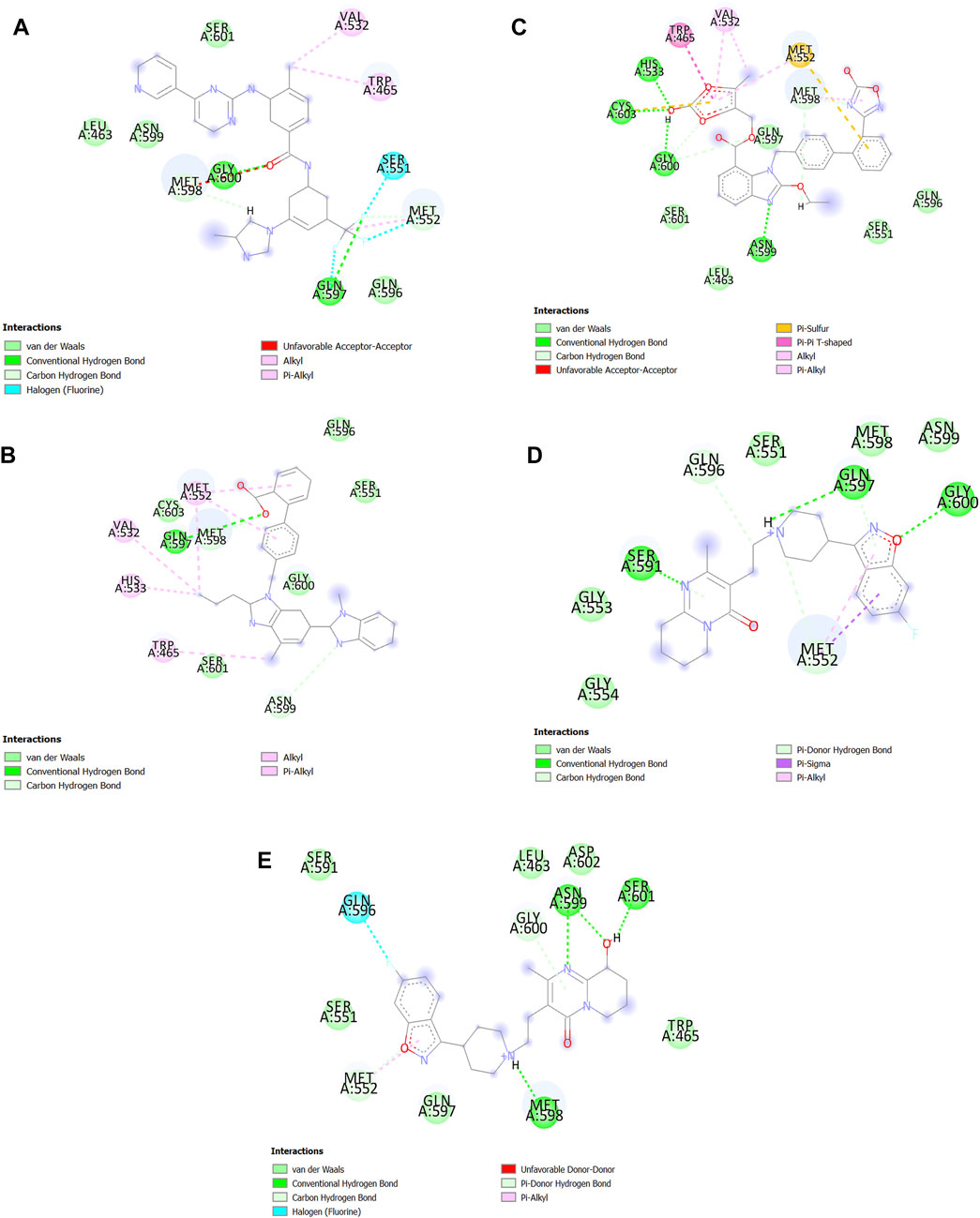
FIGURE 8. 2D structure of the best drugs for SENP1 inhibition. (A) Nilotinib, (B) Telmisartan, (C) Azilsartan medoxomil, (D) Risperdal, and (E) Paliperidone.
Discussion
Cancer is the second cause of death universally. Cancer has been led to approximately 9,958 133 million deaths in 2020 according to statistics by the Global Cancer Observatory. Numerous important advances in cancer research have manifested in the genetics and pathologies of malignant tumors, which, in turn, assist the development of new anticancer agents (Dong et al., 2019). SENP1 is located on the chromosomal position 12q13.11 (Kim and Baek, 2009). Activation of transcription factors by SUMOylation and their inactivation by deSUMOylation are performed (Chang et al., 2012). DeSUMOylation of HIF1-α by SENP1 under conditions of hypoxia is needed for the stabilization of HIF1-α and the expression of HIF1-α target genes. In the mitotic cells, the knockdown of SENP1 delays sister chromatid separation at metaphase (Nayak and Müller, 2014). The findings indicated RNA interference via SENP1 repression leads to a global increase in SUMOylated proteins and in the number of nuclear PML bodies plus P53-mediated transcription activity that results in premature senescence (Andreou and Tavernarakis, 2010). Overexpression of SENP1 was reported in many cancers (Brems-Eskildsen et al., 2010; Xu et al., 2011; Wang et al., 2013a; Wang et al., 2013b; Ma et al., 2014; Wang et al., 2016; Zhang et al., 2016).
The study of expression, functions and molecular mechanisms of SENP1 in carcinogenesis for prognosis and treatment in cancers with abnormal SENP1 expression is significant. Our study indicates the use of computational biology methods to explore and clarify new molecular biology mechanisms of SENP1 in tumorigenesis. In this study, we provided evidence of gene expression, survival status, immune infiltration, transcription factors and miRNAs, pharmacogenomics, and relevant cellular pathway for SENP1 as a biomarker in cancer across the Pan-Cancer cohort.
In this study, genetic alterations were not important in the investigation of SENP1 carcinogenesis mechanism in the Pan-Cancer cohort. Immune control maintains potentially metastatic or invading cancer cells and supplies new prognostic markers and novel therapeutic targets (Fridman et al., 2010). Inducing lymphocytic infiltration in the primitive tumor usually joins with a preferred clinical outcome in patients with cancer (Pagès et al., 2010). Increasing evidences showed immune cell infiltration plays a key role in cancer progression and metastasis and could affect the prognosis of cancer patients (Bremnes et al., 2016; Zeng et al., 2020). SENP1 through control of the SUMOylation status of STAT5 plays a role in lymphocytes of B and T development (Van Nguyen et al., 2012). Macrophage activation led to a severe decrease in the amount of SUMOylated IRF8 and promotion of SENP1 in activated macrophages that trigger innate immune responses (Chang et al., 2012). CD4+ T cells have a key role in making the immune response to cancer. CD8+ T cells are also cytotoxic T lymphocytes that identify specific tumor-associated antigens on MHC class I molecules on the cancer cell and can destroy cancer cells straightly (Hiraoka et al., 2006). We observed SENP1 has higher expression and higher immune infiltration in PAAD, ESCA, and THYM. CD4+ T cells, CD8+ T cells, and macrophages were more key-related immune cells. These are our novel findings. Then we suggest that SENP1 could affect cancer prognosis by increasing immune infiltration.
Results of the Pathological Stage Plot displayed SENP1 plays a strong role in the pathological stages in ACC, KICH, LIHC, and OV. Whereas the results of survival showed high expression of SENP1 was associated to poor prognosis of OS in the ACC, KIRP, LIHC, and THCA, and DFS in the ACC, KICH, LIHC, and MESO. Such cancer type-dependent differences in the regulation of the SENP1 could be important to the development of therapies that target SENP1.
Our study also found TFs with the highest positive correlation in UVM, and THYM, whereas miRNAs with the most inhibitory effect in KIRP, KICH, and DLBC. These TFs were involved in cell cycle and apoptosis regulation, senescence, and carcinogenesis. For example, KDM5A causes cancer through interference in the cell cycle and senescence via regulation of p16 and p27 and prevents pRB function and P53 signaling in the cell cycle (Shokri et al., 2018). YY1 is an important regulator in tumorigenesis that its expression was disturbed in many tumors (Arribas et al., 2015). Since, SENP1 is regulated by these TFs, then these can be representative a carcinogenic role of the SENP1 in cancer.
With the pathway activity study, we observed SENP1 activates cell cycle. Consistent with the pathway activity, enrichment analysis indicated SENP1-correlated genes and SENP1-binding genes were primarily complicated in the cell cycle. This was more verified by the pharmacogenomic data from pharmacoDB and GDSC that SENP1 could widely affect anti-cancer drug sensitivity across TCGA cancer types. Because SENP1 is sensitive to drugs complicated in the cell cycle. Cell cycle disorder participates in aberrant proliferation, decreased apoptosis, invasion, and metastasis (Zaretsky et al., 2016). Then, SENP1 with intervening in the cell cycle cause cancer. These findings may be protection for drug-targeted therapy in cancer.
Pathway activity also showed SENP1-correlated and SENP1-binding genes: SAP130, NUP98, and NUP153 are associated with activation of the cell cycle, UBN1 is associated with the strongest activation of RTK signaling, NUP153 is associated with the strongest activation of apoptosis, and SAP130 is associated the strongest activation of DNA Damage Response, and Hormone AR. SENP1 is also sensitive to the apoptosis and RTK signaling regulators. As well as, SENP1-correlated and SENP1-binding genes facilitate tumorigenesis by interfering in apoptosis, and DNA Damage stimulus. Then SENP1, and SENP1-associated genes increase carcinogenesis via different mechanisms.
We first reported increased SENP1 expression in ESCA, DLBC, THYM, and CHOL. We also shown SENP1 overexpression cause resistance to drugs Paclitaxel, GSK1120212 (Trametinib), PD-0325901, (5Z)-7-Oxozeaenol, Selumetinib, Tanespimycin, AZ628, Saracatinib, Afatinib, Gefitinib, BMS-536924, Sorafenib, KIN001-055, Bexarotene, LFM-A13, Midostaurin, Pluripotin, VNLG/124, and Dasatinib. The studies shown among these drugs, Paclitaxel can apply to treat B-cell lymphoma (Nevala et al., 2017), GBM (Zhan et al., 2010), PAAD (Ma and Hidalgo, 2013), ESCA (Gong et al., 2009), CHOL (Hirose et al., 2013; Cadamuro et al., 2016; Sahai et al., 2018), and THYM (Umemura et al., 2002). Other drugs also include GSK1120212 to treat CHOL (Loaiza-Bonilla et al., 2014), and PAAD (Walters et al., 2013; Estrada-Bernal et al., 2015), PD-0325901 to treat PAAD (van Geel et al., 2020), (5Z)-7-Oxozeaenol to treat DLBC (Bhalla et al., 2011), Selumetinib to treat CHOL (Prado et al., 2012), Afatinib to treat GBM (Alshami et al., 2015), CHOL (Zhang et al., 2018), PAAD (Ioannou et al., 2011; Ioannou et al., 2013), and ESCA (Wong et al., 2015), Gefitinib to treat PAAD (Li et al., 2004), GBM (Aljohani et al., 2015; Mu et al., 2016), CHOL (Yang et al., 2015), and ESCA (Guo et al., 2006), BMS-536924 to treat GBM (Zhou, 2015), and ESCA (Adachi et al., 2014), Sorafenib to treat CHOL (Huether et al., 2007), GBM (Jo et al., 2018), ESCA (Delgado et al., 2008), PAAD (Siu et al., 2006; Rausch et al., 2010), and DLBC (Greenwald et al., 2013), Bexarotene to treat GBM (Heo et al., 2016), and Dasatinib to treat DLBC (Cann et al., 2019; Scuoppo et al., 2019), THYM (Chuah et al., 2006), PAAD (Chang et al., 2008), and ESCA (Chen et al., 2015). Then SENP1 inhibition while using these drugs can be a suitable therapy strategy.
On the other hand, among the FDA-approved drugs we found inhibitors for the SENP1 including Nilotinib, Telmisartan, Azilsartan medoxomil, Risperdal, and Paliperidone. The studies indicated Telmisartan can be used to treat ESCA (Matsui et al., 2019), EAC (Fujihara et al., 2017), CHOL (Samukawa et al., 2017), and hematologic malignancies (Kozako et al., 2016). It has also been reported that Telmisartan can apply in the treatment of endometrial (Koyama et al., 2014), lung cancers (Rasheduzzaman et al., 2018), bladder and urological (Matsuyama et al., 2010), ovarian (Pu et al., 2016), colon (Lee et al., 2014), renal (de Araújo Júnior et al., 2015), prostate (Funao et al., 2008), gastric (Fujita et al., 2020), and breast (Kociecka et al., 2010) cancers, hepatocellular carcinoma (Oura et al., 2017), and GBM (Wang et al., 2021). Nilotinib can also be used to treat CHOL (Marin et al., 2018), DLBC (Robak and Robak, 2012; Cai et al., 2019), and THYM (Kelly, 2013; Simonelli et al., 2015). Moreover, Nilotinib is also used to treat other cancers including ovarian (Weigel et al., 2014), gastric (Onoyama et al., 2013), liver (Frolov, 2017) cancers, ALL, AML, and CML (Bleeker and Bardelli, 2007), GBM (Au et al., 2015; Frolov et al., 2016), melanoma (Guo et al., 2017), and gastrointestinal stromal tumors (Blay et al., 2015). Risperdal is also being used to treat DLBC (Gallagher et al., 2008), and GBM (Lee et al., 2001). Paliperidone and Azilsartan also were used to GBM (Kast, 2010), and hepatocellular carcinoma (Ahmadian et al., 2018), respectively.
Therefore, these FDA-approved drugs can be used alone to treat cancer, which shows they can be applied to treat cancer and to inhibit SENP1. So, these inhibitors can use with drugs that cause drug resistance of SENP1.
This study presents evidence of the associations between the expression of SENP1 and cancer immunity. Consistent with this finding, we have seen that SENP1 correlates with immune infiltration and several TFs increase the SENP1 expression in cancer. We also exhibited that SENP1 is highly correlated with sensitivity and resistance to anti-cancer drugs and drug-targeted genes across cancer cell lines. Our results provide a new document about the role of SENP1 in tumorigenesis and new insights into cancer therapy targets. The functions of SENP1 and associated genes were primarily complicated in the tumor-related functions and pathways that show SENP1 may mediate the progression and tumorigenesis of cancer. The FDA-Approved drugs concomitant to chemotherapy drugs do better treatment.
These findings propose the clinical value of evaluating SENP1 for specific cancer diagnosis and treatment decisions. Experimental work is necessary to further analyze and validate these findings.
Data Availability Statement
The raw data supporting the conclusion of this article will be made available by the authors, without undue reservation.
Author Contributions
ST wrote the manuscript and analyzed the data. FS and ZM conducted the research. ST and ZN conceived or designed the studies. ZM participated in the manuscript edition. All authors read and approved the final manuscript.
Funding
We received funding from the national institute of genetic engineering and biotechnology with Grant Number 660 for this study.
Conflict of Interest
The authors declare that the research was conducted in the absence of any commercial or financial relationships that could be construed as a potential conflict of interest.
Acknowledgments
We also thank Miss Zahra Nayeri for his scientific support.
Supplementary Material
The Supplementary Material for this article can be found online at: https://www.frontiersin.org/articles/10.3389/fphar.2021.700454/full#supplementary-material
References
Adachi, Y., Ohashi, H., Imsumran, A., Yamamoto, H., Matsunaga, Y., Taniguchi, H., et al. (2014). The Effect of IGF-I Receptor Blockade for Human Esophageal Squamous Cell Carcinoma and Adenocarcinoma. Tumor Biol. 35 (2), 973–985. doi:10.1007/s13277-013-1131-2
Ahmadian, E., Khosroushahi, A. Y., Eftekhari, A., Farajnia, S., Babaei, H., and Eghbal, M. A. (2018). Novel Angiotensin Receptor Blocker, Azilsartan Induces Oxidative Stress and NFkB-Mediated Apoptosis in Hepatocellular Carcinoma Cell Line HepG2. Biomed. Pharmacother. 99, 939–946. doi:10.1016/j.biopha.2018.01.117
Aljohani, H., Koncar, R. F., Zarzour, A., Park, B. S., Lee, S. H., and Bahassi, E. M. (2015). ROS1 Amplification Mediates Resistance to Gefitinib in Glioblastoma Cells. Oncotarget 6 (24), 20388–20395. doi:10.18632/oncotarget.3981
Alshami, J., Guiot, M.-C., Owen, S., Kavan, P., Gibson, N., Solca, F., et al. (2015). Afatinib, an Irreversible ErbB Family Blocker, with Protracted Temozolomide in Recurrent Glioblastoma: a Case Report. Oncotarget 6 (32), 34030–34037. doi:10.18632/oncotarget.5297
Andreou, A. M., and Tavernarakis, N. (2010). Roles for SUMO Modification during Senescence. Protein Metabolism and Homeostasis in Aging, 694. 160–171. doi:10.1007/978-1-4419-7002-2_12
Arribas, J., Castellví, J., Marcos, R., Zafón, C., and Velázquez, A. (2015). Expression of YY1 in Differentiated Thyroid Cancer. Endocr. Pathol. 26 (2), 111–118. doi:10.1007/s12022-015-9359-6
Au, K., Singh, S. K., Burrell, K., Sabha, N., Hawkins, C., Huang, A., et al. (2015). A Preclinical Study Demonstrating the Efficacy of Nilotinib in Inhibiting the Growth of Pediatric High-Grade Glioma. J. Neurooncol. 122 (3), 471–480. doi:10.1007/s11060-015-1744-y
Barage, S., Karthic, A., Bavi, R., Desai, N., Kumar, R., Kumar, V., et al. (2020). Identification and Characterization of Novel RdRp and Nsp15 Inhibitors for SARS-COV2 Using Computational Approach. J. Biomol. Struct. Dyn., 1–18. doi:10.1080/07391102.2020.1841026
Bawa-Khalfe, T., and Yeh, E. T. H. (2010). SUMO Losing Balance: SUMO Proteases Disrupt SUMO Homeostasis to Facilitate Cancer Development and Progression. Genes & Cancer 1 (7), 748–752. doi:10.1177/1947601910382555
Bhalla, S., Evens, A. M., Dai, B., Prachand, S., Gordon, L. I., and Gartenhaus, R. B. (2011). The Novel Anti-MEK Small Molecule AZD6244 Induces BIM-dependent and AKT-independent Apoptosis in Diffuse Large B-Cell Lymphoma. J. Am. Soc. Hematol. 118 (4), 1052–1061. doi:10.1182/blood-2011-03-340109
Blay, J.-Y., Shen, L., Kang, Y.-K., Rutkowski, P., Qin, S., Nosov, D., et al. (2015). Nilotinib versus Imatinib as First-Line Therapy for Patients with Unresectable or Metastatic Gastrointestinal Stromal Tumours (ENESTg1): a Randomised Phase 3 Trial. Lancet Oncol. 16 (5), 550–560. doi:10.1016/s1470-2045(15)70105-1
Bleeker, F. E., and Bardelli, A. (2007). Genomic Landscapes of Cancers: Prospects for Targeted Therapies. Pharmacogenomics. 8(12). 1629-1633. doi:10.2217/14622416.8.12.1629
Bremnes, R. M., Busund, L.-T., Kilvær, T. L., Andersen, S., Richardsen, E., Paulsen, E. E., et al. (2016). The Role of Tumor-Infiltrating Lymphocytes in Development, Progression, and Prognosis of Non-small Cell Lung Cancer. J. Thorac. Oncol. 11 (6), 789–800. doi:10.1016/j.jtho.2016.01.015
Brems-Eskildsen, A. S., Zieger, K., Toldbod, H., Holcomb, C., Higuchi, R., Mansilla, F., et al. (2010). Prediction and Diagnosis of Bladder Cancer Recurrence Based on Urinary Content of hTERT, SENP1, PPP1CA, and MCM5 Transcripts. BMC cancer 10 (1), 646. doi:10.1186/1471-2407-10-646
Cadamuro, M., Spagnuolo, G., Sambado, L., Indraccolo, S., Nardo, G., Rosato, A., et al. (2016). Low-dose Paclitaxel Reduces S100A4 Nuclear Import to Inhibit Invasion and Hematogenous Metastasis of Cholangiocarcinoma. Cancer Res. 76 (16), 4775–4784. doi:10.1158/0008-5472.can-16-0188
Cai, Z., Liu, S., Zi, J., Ma, J., and Ge, Z. (2019). A Case of Primary Gastric Diffuse Large B-Cell Lymphoma Occurring in Chronic Myeloid Leukemia. Ott Vol. 12, 5917–5923. doi:10.2147/ott.s212838
Caliskan, A., Gulfidan, G., Sinha, R., and Arga, K. Y. (2021). Differential Interactome Proposes Subtype-specific Biomarkers and Potential Therapeutics in Renal Cell Carcinomas. Jpm 11 (2), 158. doi:10.3390/jpm11020158
Cann, M. L., Herring, L. E., Haar, L. L., Gilbert, T. S. K., Goldfarb, D., Richards, K. L., et al. (2019). Dasatinib Is Preferentially Active in the Activated B-Cell Subtype of Diffuse Large B-Cell Lymphoma. J. Proteome Res. 18 (1), 522–534. doi:10.1021/acs.jproteome.8b00841
Cerami, E., Gao, J., Dogrusoz, U., Gross, B. E., Sumer, S. O., Aksoy, B. A., et al. (2012). The cBio Cancer Genomics portal: an Open Platform for Exploring Multidimensional Cancer Genomics Data. Cancer Discov. 2 (5), 404. doi:10.1158/2159-8290.CD-12-0095
Chang, Q., Jorgensen, C., Pawson, T., and Hedley, D. W. (2008). Effects of Dasatinib on EphA2 Receptor Tyrosine Kinase Activity and Downstream Signalling in Pancreatic Cancer. Br. J. Cancer 99 (7), 1074–1082. doi:10.1038/sj.bjc.6604676
Chang, T.-H., Xu, S., Tailor, P., Kanno, T., and Ozato, K. (2012). The Small Ubiquitin-like Modifier-Deconjugating Enzyme Sentrin-specific Peptidase 1 Switches IFN Regulatory Factor 8 from a Repressor to an Activator during Macrophage Activation. J.I. 189 (7), 3548–3556. doi:10.4049/jimmunol.1201104
Chen, E. Y., Tan, C. M., Kou, Y., Duan, Q., Wang, Z., Meirelles, G. V., et al. (2013). Enrichr: Interactive and Collaborative HTML5 Gene List Enrichment Analysis Tool. BMC bioinformatics 14 (1), 1–14. doi:10.1186/1471-2105-14-128
Chen, F., Chandrashekar, D. S., Varambally, S., and Creighton, C. J. (2019). Pan-cancer Molecular Subtypes Revealed by Mass-Spectrometry-Based Proteomic Characterization of More Than 500 Human Cancers. Nat. Commun. 10 (1), 1–15. doi:10.1038/s41467-019-13528-0
Chen, J., Lan, T., Zhang, W., Dong, L., Kang, N., Fu, M., et al. (2015). Dasatinib Enhances Cisplatin Sensitivity in Human Esophageal Squamous Cell Carcinoma (ESCC) Cells via Suppression of PI3K/AKT and Stat3 Pathways. Arch. Biochem. Biophys. 575, 38–45. doi:10.1016/j.abb.2014.11.008
Cheng, J., Bawa, T., Lee, P., Gong, L., and Yeh, E. T. H. (2006), Role of Desumoylation in the Development of Prostate Cancer. Neoplasia.8. 667–676. New York, NY. doi:10.1593/neo.06445
Chuah, C., Lim, T. H., Lim, A. S. T., Tien, S. L., Lim, C. H., Soong, R., et al. (2006). Dasatinib Induces a Response in Malignant Thymoma. Jco 24 (34), e56–e58. doi:10.1200/jco.2006.08.8963
Cui, C.-P., Wong, C. C.-L., Kai, A. K.-L., Ho, D. W.-H., Lau, E. Y.-T., Tsui, Y.-M., et al. (2017). SENP1 Promotes Hypoxia-Induced Cancer Stemness by HIF-1α deSUMOylation and SENP1/HIF-1α Positive Feedback Loop. Gut 66 (12), 2149–2159. doi:10.1136/gutjnl-2016-313264
Dallakyan, S., and Olson, A. J. (2015). Small-Molecule Library Screening by Docking with PyRx. Chemical Biology. 1263, 243–250. doi:10.1007/978-1-4939-2269-7_19
de Araújo Júnior, R. F., Leitão Oliveira, A. L. C., de Melo Silveira, R. F., de Oliveira Rocha, H. A., de França Cavalcanti, P., and de Araújo, A. A. (2015). Telmisartan Induces Apoptosis and Regulates Bcl-2 in Human Renal Cancer Cells. Exp. Biol. Med. (Maywood) 240 (1), 34–44. doi:10.1177/1535370214546267
Delgado, J.-S., Mustafi, R., Yee, J., Cerda, S., Chumsangsri, A., Dougherty, U., et al. (2008). Sorafenib Triggers Antiproliferative and Pro-apoptotic Signals in Human Esophageal Adenocarcinoma Cells. Dig. Dis. Sci. 53 (12), 3055–3064. doi:10.1007/s10620-008-0294-y
Deshpande, N., Addess, K. J., Bluhm, W. F., Merino-Ott, J. C., Townsend-Merino, W., Zhang, Q., et al. (2005). The RCSB Protein Data Bank: a Redesigned Query System and Relational Database Based on the mmCIF Schema. Nucleic Acids Res. 33 (Suppl. l_1), D233–D237. doi:10.1093/nar/gki057
Ding, W., Chen, J., Feng, G., Chen, G., Wu, J., Guo, Y., et al. (2020). DNMIVD: DNA Methylation Interactive Visualization Database. Nucleic Acids Res. 48 (D1), D856–D862. doi:10.1093/nar/gkz830
Doncheva, N. T., Morris, J. H., Gorodkin, J., and Jensen, L. J. (2018). Cytoscape StringApp: Network Analysis and Visualization of Proteomics Data. J. Proteome Res. 18 (2), 623–632. doi:10.1021/acs.jproteome.8b00702
Dong, Y., Qiu, P., Zhu, R., Zhao, L., Zhang, P., Wang, Y., et al. (2019). A Combined Phytochemistry and Network Pharmacology Approach to Reveal the Potential Antitumor Effective Substances and Mechanism of Phellinus Igniarius. Front. Pharmacol. 10, 266. doi:10.3389/fphar.2019.00266
Estrada-Bernal, A., Chatterjee, M., Haque, S. J., Yang, L., Morgan, M. A., Kotian, S., et al. (2015). MEK Inhibitor GSK1120212-Mediated Radiosensitization of Pancreatic Cancer Cells Involves Inhibition of DNA Double-Strand Break Repair Pathways. Cell Cycle 14 (23), 3713–3724. doi:10.1080/15384101.2015.1104437
Festuccia, N., Owens, N., Papadopoulou, T., Gonzalez, I., Tachtsidi, A., Vandoermel-Pournin, S., et al. (2019). Transcription Factor Activity and Nucleosome Organization in Mitosis. Genome Res. 29 (2), 250–260. doi:10.1101/gr.243048.118
Fridman, W. H., Galon, J., Dieu-Nosjean, M.-C., Cremer, I., Fisson, S., Damotte, D., et al. (2010). Immune Infiltration in Human Cancer: Prognostic Significance and Disease Control. Cancer Immunology and Immunotherapy. 1–24. doi:10.1007/82_2010_46
Frolov, A., Evans, I. M., Li, N., Sidlauskas, K., Paliashvili, K., Lockwood, N., et al. (2016). Imatinib and Nilotinib Increase Glioblastoma Cell Invasion via Abl-independent Stimulation of p130Cas and FAK Signalling. Scientific Rep. 6 (1), 1–12. doi:10.1038/srep27378
Frolov, A. (2017). Mechanisms of Glioblastoma Cell Migration and Invasion: Insights from the Use of Tyrosine Kinase Inhibitors: UCL. London: University College London.
Fujihara, S., Morishita, A., Ogawa, K., Tadokoro, T., Chiyo, T., Kato, K., et al. (2017). The Angiotensin II Type 1 Receptor Antagonist Telmisartan Inhibits Cell Proliferation and Tumor Growth of Esophageal Adenocarcinoma via the AMPKα/mTOR Pathway In Vitro and In Vivo. Oncotarget 8 (5), 8536–8549. doi:10.18632/oncotarget.14345
Fujita, N., Fujita, K., Iwama, H., Kobara, H., Fujihara, S., Chiyo, T., et al. (2020). Antihypertensive Drug Telmisartan Suppresses the Proliferation of Gastric Cancer Cells In Vitro and In Vivo. Oncol. Rep. 44 (1), 339–348. doi:10.3892/or.2020.7607
Funao, K., Matsuyama, M., Kawahito, Y., Sano, H., Chargui, J., Touraine, J. L., et al. (2008). Telmisartan Is a Potent Target for Prevention and Treatment in Human Prostate Cancer. Oncol. Rep. 20 (2), 295–300.
Gallagher, D. A., Schott, J. M., Childerhouse, A., Wilhelm, T., Gale, A. N., and Schrag, A. (2008). Reversible “Applause Sign” Secondary to Diffuse Large B Cell Lymphoma. Mov. Disord. 23 (16), 2426–2428. doi:10.1002/mds.22311
Gao, J., Aksoy, B. A., Dogrusoz, U., Dresdner, G., Gross, B., Sumer, S. O., et al. (2013). Integrative Analysis of Complex Cancer Genomics and Clinical Profiles Using the cBioPortal. Sci. signaling 6 (269), pl1. doi:10.1126/scisignal.2004088
Gong, Y., Ren, L., Zhou, L., Zhu, J., Huang, M., Zhou, X., et al. (2009). Phase II Evaluation of Nedaplatin and Paclitaxel in Patients with Metastatic Esophageal Carcinoma. Cancer Chemother. Pharmacol. 64 (2), 327–333. doi:10.1007/s00280-008-0874-8
Gordon, S., Akopyan, G., Garban, H., and Bonavida, B. (2006). Transcription Factor YY1: Structure, Function, and Therapeutic Implications in Cancer Biology. Oncogene 25 (8), 1125–1142. doi:10.1038/sj.onc.1209080
Greenwald, D. R., Li, H., Luger, S. M., Go, R. S., King, D., Patel, T., et al. (2013). A Phase II Study of Sorafenib (BAY 43–9006) in Recurrent Diffuse Large B Cell Lymphoma: an Eastern Cooperative Oncology Group Study (E1404). J. Hematol. Oncol. 6 (1), 1–5. doi:10.1186/1756-8722-6-46
Gul, S., Ozcan, O., Asar, S., Okyar, A., Barıs, I., and Kavakli, I. H. (2020). In Silico identification of Widely Used and Well-Tolerated Drugs as Potential SARS-CoV-2 3C-like Protease and Viral RNA-dependent RNA Polymerase Inhibitors for Direct Use in Clinical Trials. J. Biomol. Struct. Dyn., 1–20. doi:10.1080/07391102.2020.1802346
Guo, J., Carvajal, R. D., Dummer, R., Hauschild, A., Daud, A., Bastian, B. C., et al. (2017). Efficacy and Safety of Nilotinib in Patients with KIT-Mutated Metastatic or Inoperable Melanoma: Final Results from the Global, Single-Arm, Phase II TEAM Trial. Ann. Oncol. 28 (6), 1380–1387. doi:10.1093/annonc/mdx079
Guo, M., Liu, S., and Lu, F. (2006). Gefitinib-sensitizing Mutations in Esophageal Carcinoma. N. Engl. J. Med. 354 (20), 2193–2194. doi:10.1056/nejmc052698
Hang, J., and Dasso, M. (2002). Association of the Human SUMO-1 Protease SENP2 with the Nuclear Pore. J. Biol. Chem. 277 (22), 19961–19966. doi:10.1074/jbc.m201799200
Heo, J.-C., Jung, T.-H., Lee, S., Kim, H. Y., Choi, G., Jung, M., et al. (2016). Effect of Bexarotene on Differentiation of Glioblastoma Multiforme Compared with ATRA. Clin. Exp. Metastasis 33 (5), 417–429. doi:10.1007/s10585-016-9786-x
Hiraoka, K., Miyamoto, M., Cho, Y., Suzuoki, M., Oshikiri, T., Nakakubo, Y., et al. (2006). Concurrent Infiltration by CD8+ T Cells and CD4+ T Cells Is a Favourable Prognostic Factor in Non-small-cell Lung Carcinoma. Br. J. Cancer 94 (2), 275–280. doi:10.1038/sj.bjc.6602934
Hirose, A., Tajima, H., Ohta, T., Tsukada, T., Okamoto, K., Nakanuma, S., et al. (2013). Low-dose Paclitaxel Inhibits the Induction of Epidermal-Mesenchymal Transition in the Human Cholangiocarcinoma CCKS-1 Cell Line. Oncol. Lett. 6 (4), 915–920. doi:10.3892/ol.2013.1494
Huether, A., Höpfner, M., Baradari, V., Schuppan, D., and Scherübl, H. (2007). Sorafenib Alone or as Combination Therapy for Growth Control of Cholangiocarcinoma. Biochem. Pharmacol. 73 (9), 1308–1317. doi:10.1016/j.bcp.2006.12.031
Ioannou, N., Seddon, A. M., Dalgleish, A., Mackintosh, D., and Modjtahedi, H. (2013). Treatment with a Combination of the ErbB (HER) Family Blocker Afatinib and the IGF-IR Inhibitor, NVP-Aew541 Induces Synergistic Growth Inhibition of Human Pancreatic Cancer Cells. BMC cancer 13 (1), 1–12. doi:10.1186/1471-2407-13-41
Ioannou, N., Dalgleish, A. G., Seddon, A. M., Mackintosh, D., Guertler, U., Solca, F., et al. (2011). Anti-tumour Activity of Afatinib, an Irreversible ErbB Family Blocker, in Human Pancreatic Tumour Cells. Br. J. Cancer 105 (10), 1554–1562. doi:10.1038/bjc.2011.396
Irwin, J. J., and Shoichet, B. K. (2005). ZINC − A Free Database of Commercially Available Compounds for Virtual Screening. J. Chem. Inf. Model. 45 (1), 177–182. doi:10.1021/ci049714+
Jacques, C., Baris, O., Prunier-Mirebeau, D., Savagner, F., Rodien, P., Rohmer, V., et al. (2005). Two-step Differential Expression Analysis Reveals a New Set of Genes Involved in Thyroid Oncocytic Tumors. J. Clin. Endocrinol. Metab. 90 (4), 2314–2320. doi:10.1210/jc.2004-1337
Jo, Y., Kim, E., Sai, S., Kim, J., Cho, J.-M., Kim, H., et al. (2018). Functional Biological Activity of Sorafenib as a Tumor-Treating Field Sensitizer for Glioblastoma Therapy. Ijms 19 (11), 3684. doi:10.3390/ijms19113684
Kast, R. (2010). Glioblastoma Chemotherapy Adjunct via Potent Serotonin Receptor-7 Inhibition Using Currently Marketed High-Affinity Antipsychotic Medicines. Br. J. Pharmacol. 161 (3), 481–487. doi:10.1111/j.1476-5381.2010.00923.x
Kaushik, A. C., Mehmood, A., Dai, X., and Wei, D-Q. (2020). Pan-cancer Analysis and Drug Formulation for GPR139 and GPR142. Front. Pharmacol. 11, 2154.
Kelly, R. J. (2013). Thymoma versus Thymic Carcinoma: Differences in Biology Impacting Treatment. J. Natl. Compr. Canc Netw. 11 (5), 577–583. doi:10.6004/jnccn.2013.0073
Kim, J. H., and Baek, S. H. (2009). Emerging Roles of Desumoylating Enzymes. Biochim. Biophys. Acta (Bba) - Mol. Basis Dis. 1792 (3), 155–162. doi:10.1016/j.bbadis.2008.12.008
Kociecka, B., Surazynski, A., Miltyk, W., and Palka, J. (2010). The Effect of Telmisartan on Collagen Biosynthesis Depends on the Status of Estrogen Activation in Breast Cancer Cells. Eur. J. Pharmacol. 628 (1-3), 51–56. doi:10.1016/j.ejphar.2009.11.043
Kohl, M., Wiese, S., and Warscheid, B. (2011). Cytoscape: Software for Visualization and Analysis of Biological Networks. Data Mining in Proteomics. 696, 291–303. doi:10.1007/978-1-60761-987-1_18
Koyama, N., Nishida, Y., Ishii, T., Yoshida, T., Furukawa, Y., and Narahara, H. (2014). Telmisartan Induces Growth Inhibition, DNA Double-Strand Breaks and Apoptosis in Human Endometrial Cancer Cells. PLoS One 9 (3), e93050. doi:10.1371/journal.pone.0093050
Kozako, T., Soeda, S., Yoshimitsu, M., Arima, N., Kuroki, A., Hirata, S., et al. (2016). Angiotensin II Type 1 Receptor Blocker Telmisartan Induces Apoptosis and Autophagy in Adult T‐cell Leukemia Cells. FEBS Open Bio 6 (5), 442–460. doi:10.1002/2211-5463.12055
Lee, L. D., Mafura, B., Lauscher, J. C., Seeliger, H., Kreis, M. E., and Gröne, J. (2014). Antiproliferative and Apoptotic Effects of Telmisartan in Human colon Cancer Cells. Oncol. Lett. 8 (6), 2681–2686. doi:10.3892/ol.2014.2592
Lee, M. A., Leng, M. E. F., and Tiernan, E. J. J. (2001). Risperidone: a Useful Adjunct for Behavioural Disturbance in Primary Cerebral Tumours. Palliat. Med. 15 (3), 255–256. doi:10.1191/026921601678576248
Li, J., Kleeff, J., Giese, N., Büchler, M. W., Korc, M., and Friess, H. (2004). Gefitinib (‘Iressa', ZD1839), a Selective Epidermal Growth Factor Receptor Tyrosine Kinase Inhibitor, Inhibits Pancreatic Cancer Cell Growth, Invasion, and colony Formation. Int. J. Oncol. 25 (1), 203–210.
Li, J.-H., Liu, S., Zhou, H., Qu, L.-H., and Yang, J.-H. (2014). starBase v2.0: Decoding miRNA-ceRNA, miRNA-ncRNA and Protein-RNA Interaction Networks from Large-Scale CLIP-Seq Data. Nucl. Acids Res. 42 (D1), D92–D97. doi:10.1093/nar/gkt1248
Li, T., Fu, J., Zeng, Z., Cohen, D., Li, J., Chen, Q., et al. (2020). TIMER2.0 for Analysis of Tumor-Infiltrating Immune Cells. Nucleic Acids Res. 48 (W1), W509–W514. doi:10.1093/nar/gkaa407
Li, Z., Lin, C., Zhao, L., Zhou, L., Pan, X., Quan, J., et al. (2018). Oncogene miR-187-5p Is Associated with Cellular Proliferation, Migration, Invasion, Apoptosis and an Increased Risk of Recurrence in Bladder Cancer. Biomed. Pharmacother. 105, 461–469. doi:10.1016/j.biopha.2018.05.122
Liu, C.-J., Hu, F.-F., Xia, M.-X., Han, L., Zhang, Q., and Guo, A.-Y. (2018). GSCALite: a Web Server for Gene Set Cancer Analysis. Bioinformatics 34 (21), 3771–3772. doi:10.1093/bioinformatics/bty411
Loaiza-Bonilla, A., Clayton, E., Furth, E., O'Hara, M., and Morrissette, J. (2014). Dramatic Response to Dabrafenib and Trametinib Combination in a BRAF V600E-Mutated Cholangiocarcinoma: Implementation of a Molecular Tumour Board and Next-Generation Sequencing for Personalized Medicine. Ecancermedicalscience 8, 479. doi:10.3332/ecancer.2014.479
Lv, H., Pan, G., Zheng, G., Wu, X., Ren, H., Liu, Y., et al. (2010). Expression and Functions of the Repressor Element 1 (RE-1)-silencing Transcription Factor (REST) in Breast Cancer. J. Cel. Biochem. 110 (4), 968–974. doi:10.1002/jcb.22610
Ma, C., Wu, B., Huang, X., Yuan, Z., Nong, K., Dong, B., et al. (2014). SUMO-specific Protease 1 Regulates Pancreatic Cancer Cell Proliferation and Invasion by Targeting MMP-9. Tumor Biol. 35 (12), 12729–12735. doi:10.1007/s13277-014-2598-1
Ma, W. W., and Hidalgo, M. (2013). The Winning Formulation: the Development of Paclitaxel in Pancreatic Cancer. Clin. Cancer Res. 19 (20), 5572–5579. doi:10.1158/1078-0432.ccr-13-1356
Marin, J. J. G., Lozano, E., Herraez, E., Asensio, M., Di Giacomo, S., Romero, M. R., et al. (2018). Chemoresistance and Chemosensitization in Cholangiocarcinoma. Biochim. Biophys. Acta (Bba) - Mol. Basis Dis. 1864 (4), 1444–1453. doi:10.1016/j.bbadis.2017.06.005
Matsui, T., Chiyo, T., Kobara, H., Fujihara, S., Fujita, K., Namima, D., et al. (2019). Telmisartan Inhibits Cell Proliferation and Tumor Growth of Esophageal Squamous Cell Carcinoma by Inducing S-phase Arrest In Vitro and In Vivo. Ijms 20 (13), 3197. doi:10.3390/ijms20133197
Matsuyama, M., Funao, K., Kuratsukuri, K., Tanaka, T., Kawahito, Y., Sano, H., et al. (2010). Telmisartan Inhibits Human Urological Cancer Cell Growth through Early Apoptosis. Exp. Ther. Med. 1 (2), 301–306. doi:10.3892/etm_00000046
Mercatelli, D., Ray, F., and Giorgi, F. M. (2019). Pan-Cancer and Single-Cell Modeling of Genomic Alterations through Gene Expression. Front. Genet. 10, 671. doi:10.3389/fgene.2019.00671
Montojo, J., Zuberi, K., Rodriguez, H., Kazi, F., Wright, G., Donaldson, S. L., et al. (2010). GeneMANIA Cytoscape Plugin: Fast Gene Function Predictions on the Desktop. Bioinformatics 26 (22), 2927–2928. doi:10.1093/bioinformatics/btq562
Morris, G. M., Huey, R., Lindstrom, W., Sanner, M. F., Belew, R. K., Goodsell, D. S., et al. (2009). AutoDock4 and AutoDockTools4: Automated Docking with Selective Receptor Flexibility. J. Comput. Chem. 30 (16), 2785–2791. doi:10.1002/jcc.21256
Mu, L., Wang, T., Chen, Y., Tang, X., Yuan, Y., and Zhao, Y. (2016). β-Elemene Enhances the Efficacy of Gefitinib on Glioblastoma Multiforme Cells through the Inhibition of the EGFR Signaling Pathway. Int. J. Oncol. 49 (4), 1427–1436. doi:10.3892/ijo.2016.3626
Nayak, A., and Müller, S. (2014). SUMO-specific Proteases/isopeptidases: SENPs and beyond. Genome Biol. 15 (7), 422. doi:10.1186/s13059-014-0422-2
Nevala, W. K., Butterfield, J. T., Sutor, S. L., Knauer, D. J., and Markovic, S. N. (2017). Antibody-targeted Paclitaxel Loaded Nanoparticles for the Treatment of CD20+ B-Cell Lymphoma. Scientific Rep. 7 (1), 1–9. doi:10.1038/srep45682
Onoyama, M., Kitadai, Y., Tanaka, Y., Yuge, R., Shinagawa, K., Tanaka, S., et al. (2013). Combining Molecular Targeted Drugs to Inhibit Both Cancer Cells and Activated Stromal Cells in Gastric Cancer. Neoplasia 15 (12), 1391–1399. doi:10.1593/neo.131668
Oura, K., Tadokoro, T., Fujihara, S., Morishita, A., Chiyo, T., Samukawa, E., et al. (2017). Telmisartan Inhibits Hepatocellular Carcinoma Cell Proliferation In Vitro by Inducing Cell Cycle Arrest. Oncol. Rep. 38 (5), 2825–2835. doi:10.3892/or.2017.5977
Pagès, F., Galon, J., Dieu-Nosjean, M.-C., Tartour, E., Sautès-Fridman, C., and Fridman, W.-H. (2010). Immune Infiltration in Human Tumors: a Prognostic Factor that Should Not Be Ignored. Oncogene 29 (8), 1093–1102. doi:10.1038/onc.2009.416
Prado, C. M. M., Bekaii-Saab, T., Doyle, L. A., Shrestha, S., Ghosh, S., Baracos, V. E., et al. (2012). Skeletal Muscle Anabolism Is a Side Effect of Therapy with the MEK Inhibitor: Selumetinib in Patients with Cholangiocarcinoma. Br. J. Cancer 106 (10), 1583–1586. doi:10.1038/bjc.2012.144
Pu, Z., Zhu, M., and Kong, F. (2016). Telmisartan Prevents Proliferation and Promotes Apoptosis of Human Ovarian Cancer Cells through Upregulating PPARγ and Downregulating MMP-9 Expression. Mol. Med. Rep. 13 (1), 555–559. doi:10.3892/mmr.2015.4512
Rahman, M. M., Saha, T., Islam, K. J., Suman, R. H., Biswas, S., Rahat, E. U., et al. (2020). Virtual Screening, Molecular Dynamics and Structure-Activity Relationship Studies to Identify Potent Approved Drugs for Covid-19 Treatment. J. Biomol. Struct. Dyn., 1–11. doi:10.1080/07391102.2020.1794974
Rasheduzzaman, M., Moon, J.-H., Lee, J.-H., Nazim, U. M., and Park, S.-Y. (2018). Telmisartan Generates ROS-dependent Upregulation of Death Receptor 5 to Sensitize TRAIL in Lung Cancer via Inhibition of Autophagy Flux. Int. J. Biochem. Cel Biol. 102, 20–30. doi:10.1016/j.biocel.2018.06.006
Rausch, V., Liu, L., Kallifatidis, G., Baumann, B., Mattern, J., Gladkich, J., et al. (2010). Synergistic Activity of Sorafenib and Sulforaphane Abolishes Pancreatic Cancer Stem Cell Characteristics. Cancer Res. 70 (12), 5004–5013. doi:10.1158/0008-5472.can-10-0066
Regad, T. (2015). Targeting RTK Signaling Pathways in Cancer. Cancers 7 (3), 1758–1784. doi:10.3390/cancers7030860
Robak, T., and Robak, E. (2012). Tyrosine Kinase Inhibitors as Potential Drugs for B-Cell Lymphoid Malignancies and Autoimmune Disorders. Expert Opin. Investig. Drugs 21 (7), 921–947. doi:10.1517/13543784.2012.685650
Rouillard, A. D., Gundersen, G. W., Fernandez, N. F., Wang, Z., Monteiro, C. D., McDermott, M. G., et al. (2016). The Harmonizome: A Collection of Processed Datasets Gathered to Serve and Mine Knowledge about Genes and Proteins, Database. 3, 2016.
Sahai, V., Catalano, P. J., Zalupski, M. M., Lubner, S. J., Menge, M. R., Nimeiri, H. S., et al. (2018). Nab-Paclitaxel and Gemcitabine as First-Line Treatment of Advanced or Metastatic Cholangiocarcinoma. JAMA Oncol. 4 (12), 1707–1712. doi:10.1001/jamaoncol.2018.3277
Saito, R., Smoot, M. E., Ono, K., Ruscheinski, J., Wang, P.-L., Lotia, S., et al. (2012). A Travel Guide to Cytoscape Plugins. Nat. Methods 9 (11), 1069–1076. doi:10.1038/nmeth.2212
Saleem, M., Qadir, M. I., Perveen, N., Ahmad, B., Saleem, U., Irshad, T., et al. (2013). Inhibitors of Apoptotic Proteins: New Targets for Anticancer Therapy. Chem. Biol. Drug Des. 82 (3), 243–251. doi:10.1111/cbdd.12176
Samukawa, E., Fujihara, S., Oura, K., Iwama, H., Yamana, Y., Tadokoro, T., et al. (2017). Angiotensin Receptor Blocker Telmisartan Inhibits Cell Proliferation and Tumor Growth of Cholangiocarcinoma through Cell Cycle Arrest. Int. J. Oncol. 51 (6), 1674–1684. doi:10.3892/ijo.2017.4177
Scuoppo, C., Wang, J., Persaud, M., Mittan, S. K., Basso, K., Pasqualucci, L., et al. (2019). Repurposing Dasatinib for Diffuse Large B Cell Lymphoma. Proc. Natl. Acad. Sci. USA 116 (34), 16981–16986. doi:10.1073/pnas.1905239116
Shokri, G., Doudi, S., Fathi-Roudsari, M., Kouhkan, F., and Sanati, M.-H. (2018). Targeting Histone Demethylases KDM5A and KDM5B in AML Cancer Cells: A Comparative View. Leuk. Res. 68, 105–111. doi:10.1016/j.leukres.2018.02.003
Simonelli, M., Zucali, P. A., Suter, M. B., Lorenzi, E., Rubino, L., Fatuzzo, G., et al. (2015). Targeted Therapy for Thymic Epithelial Tumors: a New Horizon? Review of the Literature and Two Cases Reports. Future Oncol. 11 (8), 1223–1232. doi:10.2217/fon.14.318
Singh, G., Mishra, R., Goel, G., and Chaudhuri, T. (2020). Old Arsenal to Combat New Enemy: Repurposing of Commercially Available FDA Approved Drugs against Main Protease of SARS-CoV2. ChemRxiv [Preprint]. doi:10.26434/chemrxiv.13032578.v1
Siu, L. L., Awada, A., Takimoto, C. H., Piccart, M., Schwartz, B., Giannaris, T., et al. (2006). Phase I Trial of Sorafenib and Gemcitabine in Advanced Solid Tumors with an Expanded Cohort in Advanced Pancreatic Cancer. Clin. Cancer Res. 12 (1), 144–151. doi:10.1158/1078-0432.ccr-05-1571
Smirnov, P., Kofia, V., Maru, A., Freeman, M., Ho, C., El-Hachem, N., et al. (2017). PharmacoDB: An Integrative Database for Mining in Vitro Drug Screening Studies. bioRxiv [Preprint], 195149.
Smirnov, P., Kofia, V., Maru, A., Freeman, M., Ho, C., El-Hachem, N., et al. (2018). PharmacoDB: an Integrative Database for Mining In Vitro Anticancer Drug Screening Studies. Nucleic Acids Res. 46 (D1), D994–D1002. doi:10.1093/nar/gkx911
Sterling, T., and Irwin, J. J. (2015). ZINC 15-Ligand Discovery for Everyone. J. Chem. Inf. Model. 55 (11), 2324–2337. doi:10.1021/acs.jcim.5b00559
Sticht, C., De La Torre, C., Parveen, A., and Gretz, N. (2018). miRWalk: An Online Resource for Prediction of microRNA Binding Sites. PloS one 13 (10), e0206239. doi:10.1371/journal.pone.0206239
Taghvaei, S., Sabouni, F., Minuchehr, Z., and Taghvaei, A. (2021). Identification of Novel Anti-cancer Agents, Applying In Silico Method for SENP1 Protease Inhibition. J. Biomol. Struct. Dyn., 1–15. doi:10.1080/07391102.2021.1880480
Tang, Z., Kang, B., Li, C., Chen, T., and Zhang, Z. (2019). GEPIA2: an Enhanced Web Server for Large-Scale Expression Profiling and Interactive Analysis. Nucleic Acids Res. 47 (W1), W556–W560. doi:10.1093/nar/gkz430
Trott, O., and Olson, A. J. (2010). AutoDock Vina: Improving the Speed and Accuracy of Docking with a New Scoring Function, Efficient Optimization, and Multithreading. J. Comput. Chem. 31 (2), 455–461. doi:10.1002/jcc.21334
Tse, C., Shoemaker, A. R., Adickes, J., Anderson, M. G., Chen, J., Jin, S., et al. (2008). ABT-263: a Potent and Orally Bioavailable Bcl-2 Family Inhibitor. Cancer Res. 68 (9), 3421–3428. doi:10.1158/0008-5472.CAN-07-5836
Umemura, S., Segawa, Y., Fujiwara, K., Takata, I., Seki, N., Tokuda, Y., et al. (2002). A Case of Recurrent Metastatic Thymoma Showing a Marked Response to Paclitaxel Monotherapy. Jpn. J. Clin. Oncol. 32 (7), 262–265. doi:10.1093/jjco/hyf059
van Geel, R. M. J. M., van Brummelen, E. M. J., Eskens, F. A. L. M., Huijberts, S. C. F. A., de Vos, F. Y. F. L., Lolkema, M. P. J. K., et al. (2020). Phase 1 Study of the Pan-HER Inhibitor Dacomitinib Plus the MEK1/2 Inhibitor PD-0325901 in Patients with KRAS-Mutation-Positive Colorectal, Non-small-cell Lung and Pancreatic Cancer. Br. J. Cancer 122 (8), 1166–1174. doi:10.1038/s41416-020-0776-z
Van Nguyen, T., Angkasekwinai, P., Dou, H., Lin, F. M., Lu, L. S., Cheng, J., et al. (2012). SUMO-specific Protease 1 Is Critical for Early Lymphoid Development through Regulation of STAT5 Activation. Mol. Cel 45 (2), 210–221. doi:10.1016/j.molcel.2011.12.026
Walters, D. M., Lindberg, J. M., Adair, S. J., Newhook, T. E., Cowan, C. R., Stokes, J. B., et al. (2013). Inhibition of the Growth of Patient-Derived Pancreatic Cancer Xenografts with the MEK Inhibitor Trametinib Is Augmented by Combined Treatment with the Epidermal Growth Factor receptor/HER2 Inhibitor Lapatinib. Neoplasia 15 (2), 143–IN10. doi:10.1593/neo.121712
Wang, Q., Xia, N., Li, T., Xu, Y., Zou, Y., Zuo, Y., et al. (2013). SUMO-specific Protease 1 Promotes Prostate Cancer Progression and Metastasis. Oncogene 32 (19), 2493–2498. doi:10.1038/onc.2012.250
Wang, R.-T., Zhi, X.-Y., Zhang, Y., and Zhang, J. (2013). Inhibition of SENP1 Induces Radiosensitization in Lung Cancer Cells. Exp. Ther. Med. 6 (4), 1054–1058. doi:10.3892/etm.2013.1259
Wang, Y., Zhang, T., Li, C., Guo, J., Xu, B., and Xue, L. (2021). Telmisartan Attenuates Human Glioblastoma Cells Proliferation and Oncogenicity by Inducing the Lipid Oxidation. Asia‐Pacific J. Clin. Oncol. 1, 1–7. doi:10.1111/ajco.13574
Wang, Z., Jin, J., Zhang, J., Wang, L., and Cao, J. (2016). Depletion of SENP1 Suppresses the Proliferation and Invasion of Triple-Negative Breast Cancer Cells. Oncol. Rep. 36 (4), 2071–2078. doi:10.3892/or.2016.5036
Weigel, M. T., Rath, K., Alkatout, I., Wenners, A. S., Schem, C., Maass, N., et al. (2014). Nilotinib in Combination with Carboplatin and Paclitaxel Is a Candidate for Ovarian Cancer Treatment. Oncology 87 (4), 232–245. doi:10.1159/000363656
Wong, C. H., Ma, B. B., Hui, C. W., Tao, Q., and Chan, A. T. (2015). Preclinical Evaluation of Afatinib (BIBW2992) in Esophageal Squamous Cell Carcinoma (ESCC). Am. J. Cancer Res. 5 (12), 3588–3599.
Xu, Y., Li, J., Zuo, Y., Deng, J., Wang, L.-S., and Chen, G.-Q. (2011). SUMO-specific Protease 1 Regulates the In Vitro and In Vivo Growth of colon Cancer Cells with the Upregulated Expression of CDK Inhibitors. Cancer Lett. 309 (1), 78–84. doi:10.1016/j.canlet.2011.05.019
Yan, L., You, W.-Q., Sheng, N.-Q., Gong, J.-F., Hu, L.-D., Tan, G.-W., et al. (2018). A CREB1/miR-433 Reciprocal Feedback Loop Modulates Proliferation and Metastasis in Colorectal Cancer. Aging 10 (12), 3774–3793. doi:10.18632/aging.101671
Yang, S.-H., Lin, H.-Y., Chang, V. H. S., Chen, C.-C., Liu, Y.-R., Wang, J., et al. (2015). Lovastatin Overcomes Gefitinib Resistance through TNF-α Signaling in Human Cholangiocarcinomas with Different LKB1 Statuses In Vitro and In Vivo. Oncotarget 6 (27), 23857–23873. doi:10.18632/oncotarget.4408
Yang, W., Soares, J., Greninger, P., Edelman, E. J., Lightfoot, H., Forbes, S., et al. (2012). Genomics of Drug Sensitivity in Cancer (GDSC): a Resource for Therapeutic Biomarker Discovery in Cancer Cells. Nucleic Acids Res. 41 (D1), D955–D961. doi:10.1093/nar/gks1111
Ye, Q., Su, L., Chen, D., Zheng, W., and Liu, Y. (2017). Astragaloside IV Induced miR-134 Expression Reduces EMT and Increases Chemotherapeutic Sensitivity by Suppressing CREB1 Signaling in Colorectal Cancer Cell Line SW-480. Cell Physiol Biochem 43 (4), 1617–1626. doi:10.1159/000482025
Yu, X., Song, H., Xia, T., Han, S., Xiao, B., Luo, L., et al. (2013). Growth Inhibitory Effects of Three miR-129 Family Members on Gastric Cancer. Gene 532 (1), 87–93. doi:10.1016/j.gene.2013.09.048
Zaretsky, J. M., Garcia-Diaz, A., Shin, D. S., Escuin-Ordinas, H., Hugo, W., Hu-Lieskovan, S., et al. (2016). Mutations Associated with Acquired Resistance to PD-1 Blockade in Melanoma. N. Engl. J. Med. 375 (9), 819–829. doi:10.1056/nejmoa1604958
Zeng, Q., Sun, S., Li, Y., Li, X., Li, Z., and Liang, H. (2020). Identification of Therapeutic Targets and Prognostic Biomarkers Among CXC Chemokines in the Renal Cell Carcinoma Microenvironment. Front. Oncol. 9, 1555. doi:10.3389/fonc.2019.01555
Zhan, C., Gu, B., Xie, C., Li, J., Liu, Y., and Lu, W. (2010). Cyclic RGD Conjugated Poly(ethylene Glycol)-Co-Poly(lactic Acid) Micelle Enhances Paclitaxel Anti-glioblastoma Effect. J. Controlled Release 143 (1), 136–142. doi:10.1016/j.jconrel.2009.12.020
Zhang, C., Xu, H., Zhou, Z., Tian, Y., Cao, X., Cheng, G., et al. (2018). Blocking of the EGFR-STAT3 Signaling Pathway through Afatinib Treatment Inhibited the Intrahepatic Cholangiocarcinoma. Exp. Ther. Med. 15 (6), 4995–5000. doi:10.3892/etm.2018.6038
Zhang, J., Ren, L., Wang, Y., and Fang, X. (2019). In Silico study on Identification of Novel MALT1 Allosteric Inhibitors. RSC Adv. 9 (67), 39338–39347. doi:10.1039/c9ra07036b
Zhang, W., Sun, H., Shi, X., Wang, H., Cui, C., Xiao, F., et al. (2016). SENP1 Regulates Hepatocyte Growth Factor-Induced Migration and Epithelial-Mesenchymal Transition of Hepatocellular Carcinoma. Tumor Biol. 37 (6), 7741–7748. doi:10.1007/s13277-015-4406-y
Zhang, Y., Ai, H., Fan, X., Chen, S., Wang, Y., and Liu, L. (2020). Knockdown of Long Non-coding RNA HOTAIR Reverses Cisplatin Resistance of Ovarian Cancer Cells through Inhibiting miR-138-5p-Regulated EZH2 and SIRT1. Biol. Res. 53, 1–10. doi:10.1186/s40659-020-00286-3
Keywords: SENP1, immune infiltration, cell cycle, tumorigenesis, FDA- approved drugs, TCGA
Citation: Taghvaei S, Sabouni F and Minuchehr Z (2021) Evidence of Omics, Immune Infiltration, and Pharmacogenomic for SENP1 in the Pan-Cancer Cohort. Front. Pharmacol. 12:700454. doi: 10.3389/fphar.2021.700454
Received: 26 April 2021; Accepted: 14 June 2021;
Published: 01 July 2021.
Edited by:
Jiang-Jiang Qin, Institute of Cancer and Basic Medicine, (CAS), ChinaReviewed by:
Jingyu Kuang, National University of Defense Technology, ChinaInamul Hasan Madar, Korea University, South Korea
Copyright © 2021 Taghvaei, Sabouni and Minuchehr. This is an open-access article distributed under the terms of the Creative Commons Attribution License (CC BY). The use, distribution or reproduction in other forums is permitted, provided the original author(s) and the copyright owner(s) are credited and that the original publication in this journal is cited, in accordance with accepted academic practice. No use, distribution or reproduction is permitted which does not comply with these terms.
*Correspondence: Farzaneh Sabouni, c2Fib3VuaUBuaWdlYi5hYy5pcg==; Zarrin Minuchehr, bWludWNoZWhyQG5pZ2ViLmFjLmly
†Present address: Zarrin Minuchehr, National Institute of Genetic Engineering and Biotechnology, Tehran, Iran