- 1Department of Analysis and Bioanalysis of Medicines, Faculty of Pharmacy with the Division of Laboratory Medicine, Medical University of Bialystok, Bialystok, Poland
- 2Clinical Research Centre, Medical University of Bialystok, Bialystok, Poland
- 3Department of Clinical Pharmacy and Toxicology, Leiden University Medical Center, Leiden, Netherlands
- 4Leiden Network of Personalized Therapeutics, Leiden, Netherlands
- 5Department of Endocrinology, Diabetology and Internal Medicine, Medical University of Bialystok, Bialystok, Poland
Pharmacogenomics (PGx) studies the use of genetic data to optimize drug therapy. Numerous clinical centers have commenced implementing pharmacogenetic tests in clinical routines. Next-generation sequencing (NGS) technologies are emerging as a more comprehensive and time- and cost-effective approach in PGx. This review presents the main considerations for applying NGS in guiding drug treatment in clinical practice. It discusses both the advantages and the challenges of implementing NGS-based tests in PGx. Moreover, the limitations of each NGS platform are revealed, and the solutions for setting up and management of these technologies in clinical practice are addressed.
Introduction
The Importance of Pharmacogenomics in Modern Medicine
Pharmacogenomics (PGx) utilizes individuals’ genomic profiles to identify those who are at greater risk for adverse drug reactions or ineffectiveness. Many studies clearly indicate that drug-related genes, also referred to as “pharmacogenes,” in the human genome contain extensive functional genetic variations (FGVs) and that different alleles are associated with diverse outcomes of drug treatments (Madian et al., 2012; Guchelaar, 2018; Suarez-Kurtz and Parra, 2018). Around 97–98% of people have at least one actionable FGV in their drug-related genes. In addition, the possibility of the presence of a genetic variant which could result in a loss of function (LOF) variant in pharmacogenes is 93% for every individual (Schärfe et al., 2017). Hence, the identification of the different genetic variants associated with the drug metabolism would impact on the prescription of medication, allowing for the selection of the right drug and dose, thereby reducing the potential adverse effects or the therapeutic inefficacy. For clinical interpretation of PGx tests, the Clinical Pharmacogenetics Implementation Consortium (CPIC) and the Dutch Pharmacogenetics Working Group (DPWG) guidelines are available as well as FDA drug-gene interaction recommendations. CPIC originally started as a shared project between PharmGKB and the Pharmacogenomics Research Network (PGRN) in 2009, and DPWG was launched in 2005 by the Royal Dutch Pharmacists Association. The two consortia have developed and published recommendations for numerous gene-drug interactions (JJ Swen et al., 2011; Relling and Klein, 2011). Both CPIC and DPWG provide updated, evidence-based, free access guidelines to facilitate and accelerate the establishment of a link between the results of PGx tests and specific dose recommendations. Nowadays, an increasing number of specified PGx tests are available in specialized CAP/CLIA approved clinical pharmacology/genome analysis centers around the world and can be found in the genetic testing registry (GTR, https://www.ncbi.nlm.nih.gov/gtr/) (Jiang and You, 2015).
The introduction of next-generation genome sequencing in PGx practice is an interesting and promising, albeit challenging, step. Currently, the field of PGx is moving from reactive testing of a single gene towards scanning an entire panel of genes involved in drug absorption, distribution, metabolism, and excretion (ADME) before prescribing (pre-emptive genotyping) by applying different types of next-generation sequencing (NGS) platforms (Bielinski et al., 2014; van Der Wouden et al., 2019). The results include all the PGx-related genetic variants in the genome which will be utilized to prepare drug dosing recommendations based on the predicted phenotype provided by the sequencing tests (Figure 1).
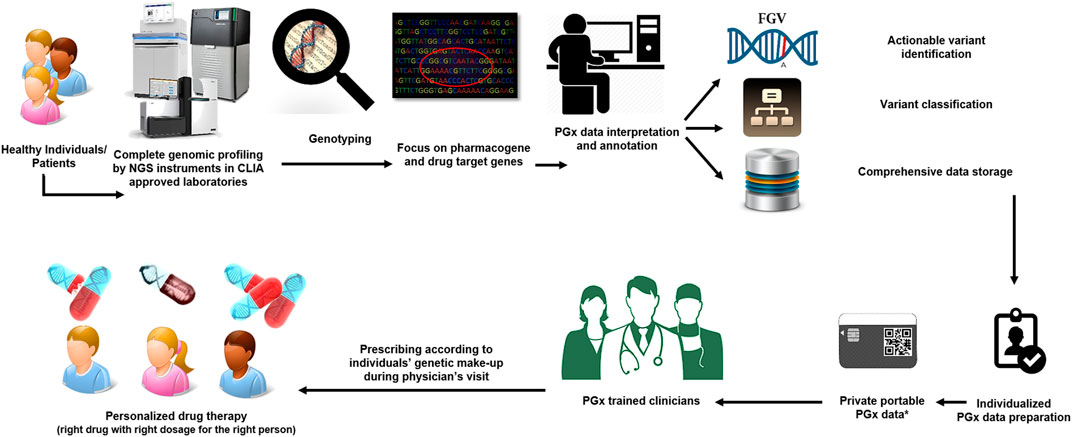
FIGURE 1. A prospective view of the use of pharmacogenomics in modern medicine. Every person (sick or healthy individuals) will undergo comprehensive genomic screening before going to the physician/clinicians. The genetic variations in all pharmacogenes of an individual will be identified through data annotation and visualization by specific bioinformatics tools. The final report for each individual will be available through a private portable PGx electronic card. PGx trained clinicians will use an individual’s genetic make-up report to tailor treatment to the patient’s needs.
While the topic is highly popular and an overview of the current state of the NGS technologies for use in PGx testing has been offered in the literature previously (Schwarz et al., 2019), this article will discuss the challenges of detecting specific types of variants in PGx and interpreting such data in clinical practice. Solutions for the establishment and management of NGS devices in clinical practice are also addressed. A number of useful tables that provide detailed NGS-PGx-related information are also included. To aid with the terminology used throughout this manuscript, we included a concise glossary of NGS-related terminology in Appendix 1.
How Can We Use NGS for PGx Analysis?
In this section, we firstly discuss the SNP-based PGx testing, which is currently the most frequently used test in the clinical PGx profiling of individuals, followed by targeted sequencing and whole-exome and whole-genome sequencing (WES/WGS).
SNP-Based PGx Testing in Clinical Practice
Fast, accurate, and inexpensive genotyping methods are key to the implementation of PGx in clinical practice. Currently, specific genotyping methods which mostly utilize different types of SNP-based genotyping approaches including real-time PCR with TaqMan probes and restriction fragment length polymorphism (RFLP) technique as well as gene panel-based genotyping methods such as ADME arrays are used in everyday clinical practice (Dorado et al., 2005; Johnson et al., 2012; Larsen and Rasmussen, 2017; Rasmussen-Torvik et al., 2017; Lemieux Perreault et al., 2018; Hippman and Nislow, 2019). In principle, genome-wide genotyping arrays such as Infinium Global Screening Array (GSA) could be used for routine PGx testing but are not yet commonly applied for this purpose. While the technology is still developing, the main limitation is that the identification of the structural PGx variants such as Copy Number Variations (CNVs) and hybrid genes as well as CYP2D6/7 is mostly ignored. Moreover, the variants in the pharmacogenes that are tested are limited to currently known and common alleles. Although several versions of arrays are being enriched with more specific PGx variants (thousands of drug-related biomarkers) (Arbitrio et al., 2016; Thermofisher.com/Pharmacoscan, 2018; Illumina, 2020), no phasing information will be obtained through these tests, which makes it more challenging to provide an accurate phenotype prediction.
Hence, the properties of NGS technologies make them an interesting approach to performing clinical PGx testing. In recent years, several investigators have explored different approaches utilizing NGS platforms, namely, targeted sequencing, WES, and WGS in pharmacogenomics. Table 1 shows several studies stratified by different approaches.
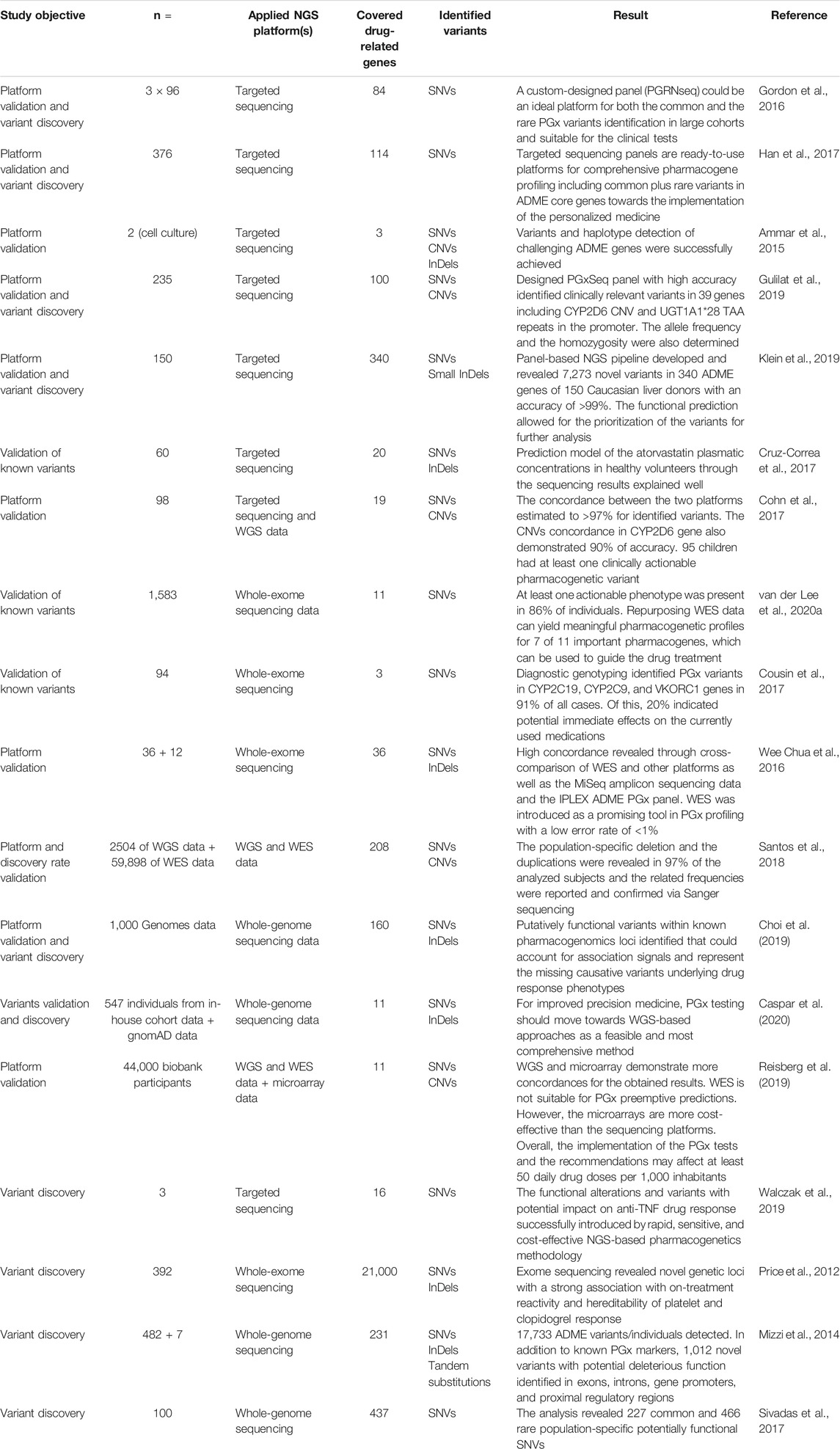
TABLE 1. Summary of the recent studies that used the NGS technologies for functional PGx variant detection.
Targeted Sequencing Panels
Research into PGx over the years has resulted in the identification of numerous genes which may play an essential role in drug metabolism, transport, and targeting in the human body. However, not all of them are strongly associated with drug response phenotypes and therefore CPIC and DPWG only provide clinical recommendations for specific variants in well-known pharmacogenes.
Gordon et al. developed the PGRNSeq panel as a balance between cost, throughput, and depth of coverage. The panel included clinically actionable CPIC genes as well as genes for which little was known, although a primary association with the PGx trait existed. It was concluded that the PGRNSeq panel is suitable for both the clinical investigations and the discovery studies. However, some non-coding parts and complex structural variants for specific pharmacogenes (including CYP2A6, CYP2D6, and HLA-B) alongside better computational resources for data interpretation remain to be developed. In a similar approach, Han et al. developed an unbiased and broad-range NGS panel and suggested that the utilization of such panels may be a valuable tool in the comprehensive study of PGx genes. The selection of genes for inclusion in the panel was based on the pharmaadme and Www.pharmaadme.org database (Gordon et al., 2016; Han et al., 2017).
Customized PGx panels can also serve as a highly accurate approach to variant detection in the clinical PGx testing. Gulilat et al. developed a targeted exome panel, named PGxSeq, for capturing both SNVs and CNVs in pharmacogenes. They demonstrated that PGxSeq could be employed as a reliable tool for common and novel SNVs alongside CNV detection in pharmacogenes in clinical use. However, a limitation of the work was that the validation was restricted to 39 loci in 16 genes in specific population samples. Moreover, pharmacogenetic variants in non-coding and regulatory parts were not included (Gulilat et al., 2019). A comprehensive PGx panel that includes all coding regions, adjacent introns, and 5′ and 3′ UTRs in flanking sequences of 340 ADME genes has recently been developed by investigators in Germany. The identification of genes for inclusion in the panel was based on multiple sources including PharmaADME, PharmGKB, and ADME-related genes from the literature. Compared with other genotyping methods, accuracy was high, with >99% correct calls. The obtained data allowed for the covering of coding and functional non-coding parts and provided related data for both common and rare variants in addition to revealing novel associations. The detection of some limited InDels and integration of rare variants into PGx by the current computational predictors alongside the sample size were reported as limitations of the panel (Klein et al., 2019).
Long-Read Sequencing for Gene Panels
Several PGx genes involve complex variants such as tandem repeats, pseudogenes, and CNVs. Long-read sequencing approaches (on average over 10 kb in one single read) have been used previously in the profiling of different complex genomic loci and have been proposed for the identification of such challenging genomic areas in PGx (Ardui et al., 2017; Mantere et al., 2019; van der Lee et al., 2020a). In this field, Ammar et al. applied long-read sequencers to identify PGx variants and haplotypes in three challenging pharmacogenes: CYP2D6, HLA-A, and HLA-B. The constructed haplotypes were confirmed by HapMap data and statistically phased Complete Genomics (WGS data from the public 69 genomes project) and Sequenom genotypes (for 36 SNP, InDels, and CNVs for CYP2D6). The results demonstrated the potential of long-read sequencing in clinical PGx (Ammar et al., 2015). In addition to haplotyping, variant phasing is also a challenge in PGx. Long-read sequencing has also been employed to resolve phasing issues and provide a solution to the accurate genotyping of complex PGx genes. Yusmiati Liau et al. utilized the GridION platform for sequencing and haplotyping of the entire CYP2D6 gene. Known and new alleles and subvariants plus duplicated alleles were assigned accurately with correct phasing. The approach also demonstrated the capability of processing multiple samples simultaneously and appeared to be a time- and cost-effective method (Liau et al., 2019).
Whole-Exome Sequencing
More comprehensive methods such as WES and WGS identify high numbers of pharmacogenetic biomarkers. In addition, these sequencing approaches may facilitate the discovery of novel loci. While it is possible to reuse WES for PGx purposes for known variants, the application for novel variants is challenging as the investigators would need a confirmative study or extensive in-vitro research to attribute potential, newly identified variants in a particular gene to drug response. This is particularly true if it is not clear what functional effect the genetic variation exerts on protein function and/or expression. Van der Lee et al. investigated the feasibility of repurposing WES data for the extraction of a PGx panel of 42 variants in 11 pharmacogenes to provide a pharmacogenomic profile. Based on the Ubiquitous Pharmacogenomics (U-PGx; www.upgx.eu) panel which includes all the actionable genes and variants in the DPWG guidelines, the authors successfully extracted information regarding 39 variants out of the total 42. At least one actionable phenotype was present in 86% of the analyzed data from the included subjects. Although structural variants (SVs) and copy numbers in some pharmacogenes as well as CYP2C19, UGT1A1, CYP3A5, and CYP2D6 were not detected, and the study suffered from a small number of drug-related genes and a limited sample size, the authors concluded that the WES data can yield meaningful pharmacogenetic profiles for 7 out of 11 important pharmacogenes (van der Lee et al., 2020b). To assess the potential benefits and the limitations of using the clinical WES data for PGx analysis as a secondary finding, Cousin et al. analyzed the clinical WES data for the detection of any FGVs in three important pharmacogenes. PGx variants were extracted from the WES test results of patients and used in addition to their medical history data. A pharmacist interpreted the PGx data based on multiple resources including CPIC, UpToDate, Micromedex, and AskMayoExpert and used the information to perform a genotype-informed medication review. The authors concluded that PGx testing early in life would be helpful for prescribing physicians to make future prescribing decisions (Cousin et al., 2017). The accuracy and the concordance rate for the WES variant calling were also investigated by Wee Chua et al. The researchers performed a cross-comparison between the WES and MiSeq amplicon sequencing data in addition to the WES and iPLEX ADME PGx panel in 36 and 12 samples, respectively. The rate obtained for both comparisons was high (99%), which indicates that WES is a promising tool in PGx profiling of individuals with an estimated error rate of <1% (Chua et al., 2016). However, despite these positive results, an important limitation of WES is that several important PGx variants, including CYP2C19*17 and VKORC1, are located outside of the captured regions of routine whole-exome sequencing.
Whole-Genome Sequencing
Complete genomic variants (including PGx-related markers) for an individual would be available through the utilization of the WGS approach. Although the big data interpretation of such tests is still challenging, a decrease in sequencing costs alongside the comprehensiveness of WGS may result in the method becoming a standard platform for clinical PGx tests.
Through using the WGS data from phase 1 of the 1,000 Genomes project and subsequent annotation, 69,319 variants including SNVs (94%) and InDels (6%) were revealed in 160 pharmacogenes (127 CPIC genes and 64 VIP genes from PharmGKB). Minor allele frequency for the variants was >1%, of which 8,207 were in strong linkage disequilibrium (LD) (r2 > 0.8) with known PGx variants. The alterations were distributed in various parts of the genome including intronic, coding, and 5′ upstream and 3′ downstream regions. In the end, the authors identified putatively functional variants within known pharmacogenomic loci underlying drug response phenotypes and suggested direct testing instead of relying on LD, which is going to be different among populations. A limited sample size and exclusion of rare variants (MAF <0.01) in addition to a lack of an experimental validation study were reported as the main limitations of the investigation. However, the results from such PGx studies facilitate the translation of the findings of the genomic analysis into clinical practice (Choi et al., 2019). While the known PGx gene panels could be included in the WGS data and considered a source for clinical PGx and drug prescribing, the remainder of the information could still be useful for discovery studies.
The functional CNVs in ADME genes are distributed with significantly different frequencies across diverse populations (He et al., 2011; Martis et al., 2013). The NGS data could also be used for CNV calling in different ethnic backgrounds. The investigators used the integrated WGS and WES data from 1,000 Genome and ExAC repositories for CNV identification in 208 pharmacogenes. Novel CNVs (deletion in 84% and duplications in 91% of genes) across six different populations of non-Finnish Europeans, Africans, Finns, East Asians, South Asians, and admixed Americans were decoded successfully. The final result highlighted the necessity for the comprehensive NGS-based genotyping of the pharmacogenes for the CNV identification alongside their allele frequencies. The assessment of the contribution of such CNVs to the drug response outcomes is also possible through a population-specific analysis of rare variants (Santos et al., 2018). Applying NGS for recognizing the actionable variants in genomic profiles may lead to lifetime utilization of PGx information for related individuals. Furthermore, future bioinformatics tools could potentially be utilized for the NGS data re-analysis and the functional prediction of novel variants (Cousin et al., 2017).
As demonstrated, the targeted sequencing approaches are most suitable for genotyping of known PGx genes, including the low-frequency variants. For the discovery of novel pharmacogenes of interest, WGS and WES are considered better choices (Reisberg et al., 2019). WES and WGS also offer the possibility of data repurposing, which means that the clinicians can benefit from the existing clinical sequencing data to extract a PGx profile to inform drug treatment. Although the NGS data from different platforms offer many potential benefits, there are still several challenges and limitations which are discussed in the following sections.
Challenges in the Application of NGS Platforms for the Decoding of PGx Variants in Specific Pharmacogenes
From the studies presented above, it appears that most types of variants in the coding and non-coding or regulatory parts of drug-related genes including SNVs, InDels, CNVs, and some structural alterations such as tandem substitutions could be identified with NGS, particularly with long-read sequencers and WGS. However, some well-known clinically actionable pharmacogenetic variants still pose a challenge for the NGS methods. Challenging genes include some core ADME genes, such as CYP2D6 which contains many different known (>100 * alleles, www.pharmvar.org) variants in different populations. Moreover, high sequence similarity and genetic recombination between real genes and close pseudogenes, such as CYP2D7 and CYP2D8, structural rearrangement complexities, and high CNVs among individuals present substantial challenges. Here, the routine short-read NGS approaches will not clarify the genetic profile of an individual and offer proper phenotype prediction. Furthermore, difficulties in the alignment procedures make interpretation and translation into clinical use complicated. Although some of these problems can be resolved by high-resolution techniques, including long-read sequencing, such sequencers with lower error rates (as well as PacBio Sequel HiFi II) are only available through highly specialized centers and are not yet applied in routine clinical practice (Yang et al., 2017). In addition, the technology is currently not being considered for the large-scale genome analysis in the PGx studies (van der Lee et al., 2020a).
Another example of a challenging pharmacogene is UGT1A1, with some important variants in the non-coding parts of the gene (TA repeats in the promoter of the gene, particularly UGT1A1*28, which affect the gene transcription and hence enzyme activity) (Bosma et al., 1995; Dalén et al., 1998; Numanagić et al., 2015). The gene harbors more than 113 functionally relevant variants, most of which reduce or enhance enzyme function, in addition to many other variants with unknown significance. The allele frequency is heavily population-specific, too. However, most of the panels focus on commonly known genotypes and could easily miss predictive variants in particular cases. By way of illustration, FDA approved the test for *28 allele but not *6 allele for irinotecan, although the latter is the main cause of the altered activity of the UGT1A enzyme in the Asian populations (Ikediobi et al., 2009). Also, the utilization of more comprehensive platforms such as WES is accompanied by poor and insufficient coverage for non-coding parts, which may result in the lower concordance and weak diplotype and CNV calls for the UGT1A1 gene (van der Lee et al., 2020b).
A third challenging region is the HLA genes. They are characterized by high sequence homology and prone to error in the capturing procedure and possible misalignment in the mapping processes. In addition, more than 21,000 known alleles and several pseudogenes and some InDels in the intronic regions of HLA class I and class II genes require the utilization of a proper platform, and more advanced IT infrastructure for the bioinformatics analysis and the identification of various potential predictive PGx markers, particularly in the newly studied populations (Klasberg et al., 2019). HLA alleles are important not only in PGx but also in other medical fields, including the genomic evaluation of multifactorial disorders and organ transplantation. Unfortunately, most of the HLA variants are rare and population-specific and are not included in routine clinical PGx testing (Nakkam et al., 2018). Today, many bioinformatics tools and algorithms available for HLA variant calling and haplotype phasing based on the WGS, WES, and targeted sequencing results. However, the high coverage of the genomic region is preferred as input for the allelic imputation by most software (Karnes et al., 2017). The available tools and their pros and cons have been discussed comprehensively in the literature (Ka et al., 2017; Kawaguchi et al., 2017; Xie et al., 2017). In general, to overcome the challenges of decoding PGx variants in specific genes, up-to-date knowledge of PGx-related genomics for physicians requesting the test in addition to the selection and utilization of an appropriate platform and interpretation tools for each situation by PGx test centers is required. This may also include previous knowledge of some particular PGx alleles with substrate-specific effects. For example, CYP2D6*17 encodes an enzyme with an increased capacity to metabolize haloperidol but an impaired ability to metabolize codeine (Oscarson et al., 1997; Wennerholm et al., 2002). In addition, occasional discrepancies between guidelines on the classification of genotypes into metabolic groups (which is key to formulating corresponding therapeutic recommendations) must also be considered (Caudle et al., 2020). Table 2 summarizes some challenging pharmacogenes and their main features that need to be taken into consideration during sequencing or panel design.
Challenges and Opportunities for Data Acquisition and Interpretation
The NGS data annotation, in the form of PGx phenotype prediction, is a highly specialized task that requires both molecular knowledge and clinical knowledge. The extraction of actionable, putative, or likely pathogenic variants from large, sophisticated raw data requires considerable time and effort as well as accurate validation methods. The current approaches include newly developed PGx dedicated tools for star allele calling in pharmacogenes (discussed in the following sections). Here, we address the key considerations, discuss some features of the common PGx-related tools, and propose solutions for managing the challenges.
Targeted Sequencing Panels
Unlike with other genotyping approaches, performing a sequencing run always offers the possibility of decoding novel variants in the sequenced part(s). This has also been observed in the targeted sequencing panels of known pharmacogenes, where novel variations appeared in addition to common markers (Gulilat et al., 2019). Indeed, the variants with unknown clinical significance (VUS) in the NGS data and with no clear connection to pharmacogenetics present a real challenge as far as the implementation of such technologies in clinical practice is concerned. Nevertheless, handling VUS as potentially important identified variants is essential since if appropriate approaches to the correct interpretation were not available, the real functional alleles might simply be introduced as non-actionable. Therefore, a prediction is not feasible easily on the functionality of VUS to interpret the potential effects on the drug responses in a patient. However, because of the lower number of such findings in panels, replication and validation studies using other orthogonal genotyping methods, in silico algorithms, genetic screening for first degree relatives of the proband, and use of GWAS, HapMap, or gnomAD datasets for meta-analysis will be faster and more easier with regard to predicting and confirming the negative or neutral functionality of variants and demonstrating the phenotype associations in the targeted sequencing approaches (Svidnicki et al., 2020).
Whole-Exome and Whole-Genome Sequencing
As expected, VUS are more common in WES and WGS. The situation becomes even more complicated when the results involve novel PGx genes. Online tools such as SIFT and PolyPhen2 as well as other algorithms, including CADD and PROVEAN, plus Ensembl based sources with multiple integrated tools like VEP and REVEL, are available for the prediction of the damaging effects of a large number of variants. However, these tools rely primarily on evolutionary conservation and utilize amino acid or nucleotide sequence alignment, which is less applicable to pharmacogenes. Also, low predictive value of these tools has recently been demonstrated (Lee et al., 2019; Zhou et al., 2019).
Furthermore, incidental findings (IFs), referred to as secondary findings in the ACMG recommendations (Kalia et al., 2017), can be expected in different types of high throughput sequencing and genotype screening methods. They are mostly defined as annotated functional variants in major drug-related genes which were not expected in the specified assessment but may be either related or unrelated to the particular medication taken by the patient. This adds to the complexity of reporting findings from PGx profiling, where the DNA variants may alter the drug efficacy or increase the risk of serious adverse drug reactions. Such findings could be reported as variants with potential usage in guiding therapy if they are managed properly through appropriate clinical genomic assays, vigorous genotype-phenotype correlation studies, and utilization of PGx-related sources for data interpretation and variant scoring (Lee et al., 2016). However, the existence of secondary findings would also be associated with some technical issues in the employed NGS platform. These issues include the percentage of coverage and type of sequencing methods as well as the number of evaluated individuals, evaluation of family members or randomly selected patients (Westbrook et al., 2013). Yet, not all secondary findings that are identified need to be reported in the result of a clinical test. The ACMG also declared a policy statement for reporting particular secondary findings in the clinical setting (L Blackburn et al., 2015; Miller et al., 2021). However, the statement is related to non-PGx secondary findings. Moreover, many pharmacogenetic variants are not disease-causing. Therefore, the relevance of reporting secondary findings may not be obvious at the time of submitting the report, particularly when only a specific set of pharmacogenes is tested. For the pharmacogenes connected with disease risk, the secondary findings may be handled in accordance with the current ACMG recommendations; that is, it is not necessary to provide a separate set of recommendations for those genes. Nevertheless, while the purpose of PGx testing is to exhaustively (and pre-emptively) profile genes that may potentially alter the drug response, curating and storing the information relevant to the future drug therapy may indicate that no findings should be considered “secondary,” particularly when untargeted methods as well as WES and WGS are employed.
Recently Developed Bioinformatics Algorithms for PGx Variant Calling
Concentrated efforts have been undertaken to design and develop specific PGx tools for the identification of SNVs, CNVs, structural rearrangements, gene deletion, gene duplication/multiplication, haplotype phasing, diplotype calling, and phenotype prediction out of the NGS data in the clinical setting. The tools as well as Stargazer, PharmCAT, Astrolabe, Aldy, Cypripi, include special algorithms, which were designed for the interpretation of the PGx variants (Numanagić et al., 2015; Twist et al., 2016; Klein and Ritchie, 2018; Numanagić et al., 2018; Lee et al., 2019). Furthermore, some other tools including g-Nomic and PHARMIP were developed for providing recommendations based on the general information obtained from a PGx test (Sabater et al., 2019; Zidan et al., 2020). The advantages and the disadvantages of each of the tools have been demonstrated previously in the literature (Twesigomwe et al., 2020). Table 3 provides a concise overview of the key features of these tools. Stargazer, Astrolabe, and Aldy have been fully analyzed and are widely used in the field. Twesigomwe and colleagues have recently performed a comprehensive and systematic comparison of the functions of these three tools in calling different CYP2D6 variants. The results of the study demonstrate that Aldy and Astrolabe are better common and rare SNV callers compared to Stargazer. Yet, Stargazer outperformed the other tools in rare homozygous allele phasing due to its in-built supplementary algorithm. Calling InDel star alleles in the short-read NGS data and the hybrid rearrangements was challenging for all three algorithms. For other structural variants, gene deletion, duplication, and multiplications, Aldy demonstrated higher concordance in comparison to Stargazer and Astrolabe, respectively. Noticeably, Astrolabe performed weak structural variant calling in comparison to the other two tools. Although Stargazer displayed better performance in CNV calling and the identification of hybrid rearrangements, it simultaneously revealed the highest number of non-genotyped diplotypes for the samples including structural variants. Unfortunately, all three tools had difficulty calling diplotypes with high copy numbers. While these genotypes are very rare, they may still be considered an important variant in some isolated populations. The phenotype prediction and the clinical accuracy of Aldy, Astrolabe, and Stargazer were also evaluated. Remarkably, the concordances were higher than the diplotype concordances as the activity scoring systems may assign the same values as the true function of the wrongly genotyped samples. The impact of the sequencing coverage and the misalignment of InDels on genotyping accuracy was also investigated. The study, however, had some limitations. It used simulated data for most rare and structural variants, did not compare the performances of the three tools across the NGS data from the targeted custom-capture panels, and did not compare the impacts of different aligners on the variant calling processes. Novel SNVs calling was also not analyzed in the study and reliable validation studies were not included (Twesigomwe et al., 2020). Aldy and Stargazer may also result in false-positive/false-negative results in small variant calling, since they rely on initial read alignments. Another major obstacle is that two of the three tools does not support the GRCh38 genome assembly and that the investigators may need to lift their alignments to GRCh37 (i.e., https://genome.ucsc.edu/cgi-bin/hgLiftOver). To address these challenges, Chen et al. developed Cyrius, a novel bioinformatics method for all classes of variants and haplotype calling from CYP2D6 in the WGS data (also included in Table 3). The tool can overcome CYP2D6 and CYP2D7 homology challenges and work with both GRCh37 and 38 to accurately genotype CYP2D6 with a higher overall concordance rate with true genotypes (99.3%). Compared to Aldy and Stargazer, superior genotyping was demonstrated for both GeT-RM and long-read data, and the application of the method led to improved understanding of CYP2D6 genetic diversity within five ethnic groups. The authors are currently extending the method to genotype other pharmacogenes with a paralog, CYP2A6 and CYP2B6, and plan to apply it to more genes in the future (Chen et al., 2021). Overall, it is useful to be aware of the specifications and the features of each of the tools in order to increase their utility while applying such algorithms to calling different PGx variants out of high throughput sequencing results.
Solutions for the Management of Challenges in Applying the NGS-PGx Tests in the Clinic
Here, we present three main problems which may arise during clinical NGS testing for PGx in everyday practice and discuss solutions.
Firstly, based on the type of panel or other selected approaches, the setup and the initiation of NGS tests (covering PGx markers) in every clinic will require a substantial investment and reimbursement by insurance companies, bioinformatics infrastructure, specific software and computational tools, and professional clinical experts for data interpretation. In addition, validation studies to determine and improve the clinical utility and the validity are essential. Once a positive evaluation has been performed by public and private payers, relevant NGS-derived PGx tests could be considered for implementation in routine clinical practice. Estimated costs of PGx profiling may vary substantially depending on the type of test applied. Is the PGx assessment a pre-emptive NGS test or repurposed findings from diagnostic WES/WGS? Currently, the test coverage and reimbursement are still considered major barriers to routine clinical use. Enhancing physicians’ awareness of the type of test to be requested, gaining third-party support, increasing the number of clients through direct-to-consumer genetic testing companies, and decreasing the cost of tests due to advances in diagnostic technologies may play an essential role in bringing the clinical utility of PGx tests to the attention of insurance companies (L Rogers et al., 2020). While many related services are currently limited to reactive single-gene testing, some clinical centers offer routine pre-emptive PGx tests. For example, all patients treated for an active disease at St. Jude Research Hospital are offered PGx testing (www.stjude.org/pg4kds). Recently, Anderson et al. performed a large-scale study in the United States and demonstrated that only a few core pharmacogenes, including CYP2C19, CYP2D6, CYP2C9, VKORC1, UGT1A1, and HLA class I, were covered by the patients’ insurance (Anderson et al., 2020).
Secondly, as mentioned previously, the evolutionary conservation is less applicable to the drug-related genes and therefore the conventional computational algorithms have low predictive accuracy when applied to the pharmacogenetic variants. The difficulties with novel and big data interpretation could be overcome by applying combined and optimized calculation tools and algorithms (at least 6-7 of such bioinformatics tools) for allele imputation (see Appendix 1) of PGx single- or multi-marker signatures, as well as confirming such genetic variants as predictive for the drug response with more accuracy (Zhou et al., 2019; Tafazoli et al., 2021). However, not all pharmacogenes have this limitation. Indeed, some genes appear relatively free of evolutionary constraints and are highly similar to other genes. This is particularly true for the genes that are involved in the transfer of endogenous substances (i.e., OTC1). Whenever a novel PGx variant is identified in evolutionarily conserved positions, such genes may still benefit from routine predictor tools to indicate their functional impact (Shu et al., 2003). However, in the absence of distinct clinical data, both computational and laboratory models are needed for the genotype-guided drug therapy based on previously unreported genomic variants (Shrestha et al., 2018).
Other PGx specific computational models and algorithms with a high sensitivity and specificity have also been developed for the prediction of the loss of function and/or the functionally neutral variations. The scores obtained with the models could provide quantitative estimation of the impact of different variants on the gene function. A comprehensive analysis of the computational prediction methods and evaluation of the recent progress in the functional interpretation of non-coding variants for drug-metabolizing enzymes and transporters is provided by Zhou and colleagues (Zhou et al., 2018). Once the functionality of a variant is known, the effect on drug pharmacology needs to be estimated. For this, pathway analysis databases as well as DAVID, Human Metabolome Database, String-db, and KEGG could be used to identify the molecular connections between the altered allele(s) in specific genes and the other related genes in the cell. Moreover, newly developed PGx specific tools such as Aldy, Stargazer, Astrolabe, and Cyrius can also help with NGS data processing in the PGx analysis (Klein and Ritchie, 2018; Lee et al., 2019). Table 4 lists some databases which are useful in interpreting the results of the clinical PGx analysis. We have also recently reviewed the software and the algorithms dedicated to the functional prediction alongside the related mechanism of action in such tools while using the PGx functional analysis (Tafazoli et al., 2021). After finding a potentially strong relationship between the identified variant(s) and the drug response, particular in-vitro assessments as well as cell line modifications may be considered for exploring the functional consequences of the altered alleles and diplotypes on the activity of the related protein. However, the latter is not appropriate in clinical use as it increases the turnaround time considerably. As the final step, the clinical association analysis will confirm the connection between the novel variants and the drug response phenotypes in the patients. Needless to say, it is suitable solely for the patient data analysis and not pre-emptive PGx profiling of a healthy individual with no clinically observable phenotype (Ji et al., 2013).
Finally, while well-known and annotated PGx variant(s) can be used immediately in patient care, the clinical translation and utilization of newly introduced variants requires substantial evidence and records of gene-drug interaction as well as phenotyping data. Nevertheless, such data would be stored primarily for the research purposes and the patient may be recontacted for further investigations. Since the prediction of an individual’s metabolic status is very important for drug dosage modifications in a clinic, the translation of the sequencing results into phenotype assignment must follow the universal standardized test interpretation approaches. A gene continuum activity score system has been introduced to deal with such situations and may be accepted by reference laboratories and medical centers for converting the genotype data to the clinically actionable recommendations (Hicks et al., 2014). However, to facilitate the incorporation of the high throughput derived PGx reports in the clinical setting, it is necessary to provide the healthcare professionals with more applicable, evidence-based results and employ standardized and updated cohort and case reports (Giri et al., 2019; Krebs and Milani, 2019).
Conclusion
The NGS technologies have been used in the PGx research studies for a decade. The rapid development in accessories and supporting bioinformatics tools in addition to the reduced cost and the technological advancement that will allow for testing of a larger number of drug-related genes and biomarkers will result in the widespread use of such methods in various clinical settings. The main challenges are management of identified VUS, a lack of specific variant caller software, poor haplotype phasing, insufficient coverage of some parts of the genome by different platforms, limited capacity to assess variant functionality in-vitro, and limited ability to assess functionality through computational approaches. Nevertheless, the application of NGS in PGx testing in the clinical practice is continually increasing, paving the way for new PGx variant discovery and a bright future for pharmacogenomics-guided drug treatment.
Author Contributions
AT designed the study, conducted the search for literature, and wrote the entire manuscript. H-JG and JS supervised the study thoroughly and revised and edited the manuscript. WM performed the search for literature and modified the text as well. AK provided the idea and introduced the topic for the manuscript. All authors have read and agreed to the current version of the manuscript.
Funding
This article was conducted within the projects which have received funding from the European Union’s Horizon 2020 research and innovation program under the Marie Skłodowska-Curie grant, agreement no. 754432, and the Polish Ministry of Science and Higher Education and from financial resources for science in 2018–2023 granted for the implementation of an international co-financed project.
Conflict of Interest
The authors declare that the research was conducted in the absence of any commercial or financial relationships that could be construed as a potential conflict of interest.
Publisher’s Note
All claims expressed in this article are solely those of the authors and do not necessarily represent those of their affiliated organizations, or those of the publisher, the editors and the reviewers. Any product that may be evaluated in this article, or claim that may be made by its manufacturer, is not guaranteed or endorsed by the publisher.
Abbreviations
ACMG, American College of Medical Genetics and Genomics; CADD, Combined Annotation-Dependent Depletion; CAP, College of American Pathologists; CLIA, Clinical Laboratory Improvement Amendments; CNV, Copy Number Variation; CYP, cytochrome P450; ExAC, Exome Aggregation Consortium; FDA, US Food and Drug Administration; GWAS, Genome-Wide Association Study; InDel, Insertion/Deletion; MAF, minor allele frequency; PROVEAN, Protein Variation Effect Analyzer; REVEL, Rare Exome Variant Ensemble Learner; SNP, Single Nucleotide Polymorphism; SNV, Single Nucleotide Variation; SV, structural variant; VCF, variant calling format; VEP, Variant Effect Predictor; VIP, Very Important Pharmacogenes.
References
Ammar, R., Paton, T. A., Torti, D., Shlien, A., and Bader, G. D. (2015). Long Read Nanopore Sequencing for Detection of HLA and CYP2D6 Variants and Haplotypes. F1000Res 4, 17. doi:10.12688/f1000research.6037.2
Anderson, H. D., Crooks, K. R., Kao, D. P., and Aquilante, C. L. (2020). The Landscape of Pharmacogenetic Testing in a US Managed Care Population. Genet. Med. 22, 1247–1253. 10.1038/s41436-020-0788-3.
Arbitrio, M., Di Martino, M., Scionti, F., Agapito, G., Hiram Guzzi, P., and Cannataro, M. (2016). DMETTM (Drug Metabolism Enzymes and Transporters): a Pharmacogenomic Platform for Precision Medicine. Oncotarget 5 (33), 54028–54050. doi:10.18632/oncotarget.9927
Ardui, S., Race, V., Zablotskaya, A., Hestand, M. S., Van Esch, H., Devriendt, K., et al. (2017). Detecting AGG Interruptions in Male and Female FMR1 Premutation Carriers by Single-Molecule Sequencing. Hum. Mutat. 38, 324–331. doi:10.1002/humu.23150
Barbarino, J. M., Haidar, C. E., Klein, T. E., and Altman, R. B. (2014). PharmGKB Summary. Pharmacogenetics and genomics 24, 177–183. doi:10.1097/FPC.0000000000000024
Barbarino, J. M., Whirl-Carrillo, M., Altman, R. B., and Klein, T. E. (2018). PharmGKB: a Worldwide Resource for Pharmacogenomic Information. Wires Syst. Biol. Med. 10, e1417. doi:10.1002/wsbm.1417
Bielinski, S. J., Olson, J. E., Pathak, J., Weinshilboum, R. M., Wang, L., Lyke, K. J., et al. (2014). Preemptive Genotyping for Personalized Medicine: Design of the Right Drug, Right Dose, Right Time-Using Genomic Data to Individualize Treatment Protocol. Mayo Clinic Proc. 89, 25–33. doi:10.1016/j.mayocp.2013.10.021
Bosma, P. J., Chowdhury, J. R., Bakker, C., Gantla, S., De Boer, A., Oostra, B. A., et al. (1995). The Genetic Basis of the Reduced Expression of Bilirubin UDP-Glucuronosyltransferase 1 in Gilbert's Syndrome. N. Engl. J. Med. 333, 1171–1175. doi:10.1056/NEJM199511023331802
Caspar, S. M., Schneider, T., Meienberg, J., and Matyas, G. (2020). Added Value of Clinical Sequencing: WGS-Based Profiling of Pharmacogenes. Ijms 21, 2308. doi:10.3390/ijms21072308
Caudle, K. E., Sangkuhl, K., Whirl‐Carrillo, M., Swen, J. J., Haidar, C. E., Klein, T. E., et al. (2020). Standardizing CYP 2D6 Genotype to Phenotype Translation: Consensus Recommendations from the Clinical Pharmacogenetics Implementation Consortium and Dutch Pharmacogenetics Working Group. Clin. Transl Sci. 13, 116–124. doi:10.1111/cts.12692
Chen, X., Shen, F., Gonzaludo, N., Malhotra, A., Rogert, C., Taft, R. J., et al. (2021). Cyrius: Accurate CYP2D6 Genotyping Using Whole-Genome Sequencing Data. Pharmacogenomics J. 21, 251–261. doi:10.1038/s41397-020-00205-5
Choi, J., Tantisira, K. G., and Duan, Q. L. (2019). Whole Genome Sequencing Identifies High-Impact Variants in Well-Known Pharmacogenomic Genes. Pharmacogenomics J. 19, 127–135. doi:10.1038/s41397-018-0048-y
Chua, E. W., Cree, S. L., Ton, K. N. T., Lehnert, K., Shepherd, P., Helsby, N., et al. (2016). Cross-Comparison of Exome Analysis, Next-Generation Sequencing of Amplicons, and the iPLEX ADME PGx Panel for Pharmacogenomic Profiling. Front. Pharmacol. 7, 1. doi:10.3389/fphar.2016.00001
Chumnumwat, S., Lu, Z. H., Sukasem, C., Winther, M. D., Capule, F. R., Abdul Hamid, A. A. a. t., et al. (2019). Southeast Asian Pharmacogenomics Research Network (SEAPharm): Current Status and Perspectives. Public health genomics 22, 132–139. doi:10.1159/000502916
Cohn, I., Paton, T. A., Marshall, C. R., Basran, R., Stavropoulos, D. J., Ray, P. N., et al. (2017). Genome Sequencing as a Platform for Pharmacogenetic Genotyping: a Pediatric Cohort Study. Npj Genomic Med. 2, 19. doi:10.1038/s41525-017-0021-8
Cousin, M. A., Matey, E. T., Blackburn, P. R., Boczek, N. J., Mcallister, T. M., Kruisselbrink, T. M., et al. (2017). Pharmacogenomic Findings from Clinical Whole Exome Sequencing of Diagnostic Odyssey Patients. Mol. Genet. Genomic Med. 5, 269–279. doi:10.1002/mgg3.283
Cruz-Correa, O. F., León-Cachón, R. B. R., Barrera-Saldaña, H. A., and Soberón, X. (2017). Prediction of Atorvastatin Plasmatic Concentrations in Healthy Volunteers Using Integrated Pharmacogenetics Sequencing. Pharmacogenomics 18, 121–131. doi:10.2217/pgs-2016-0072
Dalén, P., Dahl, M.-L., Ruiz, M. L. B., Nordin, J., and Bertilsson, L. (1998). 10-hydroxylation of Nortriptyline in white Persons with 0, 1, 2, 3, and 13 Functional CYP2D6 Genes*. Clin. Pharmacol. Ther. 63, 444–452. doi:10.1016/S0009-9236(98)90040-6
Dorado, P., Cáceres, M. C., Pozo-Guisado, E., Wong, M.-L., Licinio, J., and Llerena, A. (2005). Development of a PCR-Based Strategy forCYP2D6genotyping Including Gene Multiplication of Worldwide Potential Use. Biotechniques 39, S571–S574. doi:10.2144/000112044
Flockhart, D. A., and Oesterheld, J. R. (2000). Cytochrome P450-Mediated Drug Interactions. Child. Adolescent Psychiatric Clinics North. America 9, 43–76. doi:10.1016/s1056-4993(18)30135-4
Gaedigk, A., Ingelman-Sundberg, M., Miller, N. A., Leeder, J. S., Whirl-Carrillo, M., Klein, T. E., et al. (2018). The Pharmacogene Variation (PharmVar) Consortium: Incorporation of the Human Cytochrome P450 (CYP ) Allele Nomenclature Database. Clin. Pharmacol. Ther. 103, 399–401. doi:10.1002/cpt.910
Giri, J., Moyer, A. M., Bielinski, S. J., and Caraballo, P. J. (2019). Concepts Driving Pharmacogenomics Implementation into Everyday Healthcare. Pgpm Vol. 12, 305–318. doi:10.2147/PGPM.S193185
Gordon, A. S., Fulton, R. S., Qin, X., Mardis, E. R., Nickerson, D. A., and Scherer, S. (2016). PGRNseq. Pharmacogenetics and genomics 26, 161–168. doi:10.1097/FPC.0000000000000202
Guchelaar, H.-J. (2018). Pharmacogenomics, a Novel Section in the European Journal of Human Genetics. Eur. J. Hum. Genet. 26, 1399–1400. doi:10.1038/s41431-018-0205-4
Gulilat, M., Lamb, T., Teft, W. A., Wang, J., Dron, J. S., Robinson, J. F., et al. (2019). Targeted Next Generation Sequencing as a Tool for Precision Medicine. BMC Med. genomics 12, 81. doi:10.1186/s12920-019-0527-2
Han, S., Park, J., Lee, J., Lee, S., Kim, H., Han, H., et al. (2017). Targeted Next-Generation Sequencing for Comprehensive Genetic Profiling of Pharmacogenes. Clin. Pharmacol. Ther. 101, 396–405. doi:10.1002/cpt.532
He, Y., Hoskins, J. M., and Mcleod, H. L. (2011). Copy Number Variants in Pharmacogenetic Genes. Trends Molecular Medicine 17, 244–251. doi:10.1016/j.molmed.2011.01.007
Hicks, J., Swen, J., and Gaedigk, A. (2014). Challenges in CYP2D6 Phenotype Assignment from Genotype Data: a Critical Assessment and Call for Standardization. Cdm 15, 218–232. doi:10.2174/1389200215666140202215316
Hippman, C., and Nislow, C. (2019). Pharmacogenomic Testing: Clinical Evidence and Implementation Challenges. Jpm 9, 40. doi:10.3390/jpm9030040
Huang, L., Fernandes, H., Zia, H., Tavassoli, P., Rennert, H., Pisapia, D., et al. (2017). The Cancer Precision Medicine Knowledge Base for Structured Clinical-Grade Mutations and Interpretations. J. Am. Med. Inform. Assoc. 24, 513–519. doi:10.1093/jamia/ocw148
Ikediobi, O., Shin, J., Nussbaum, R., Phillips, K., Translational, U. C. F., Medicine, P. R. O. P., et al. (2009). Addressing the Challenges of the Clinical Application of Pharmacogenetic Testing. Clin. Pharmacol. Ther. 86, 28–31. doi:10.1038/clpt.2009.30
Illing, P. T., Purcell, A. W., and Mccluskey, J. (2017). The Role of HLA Genes in Pharmacogenomics: Unravelling HLA Associated Adverse Drug Reactions. Immunogenetics 69, 617–630. doi:10.1007/s00251-017-1007-5
Illumina, (2020). Infinium® Global Screening Array-24 v3.0 BeadChip. Availableat: https://emea.illumina.com/content/dam/illumina-marketing/documents/products/datasheets/infinium-global-screening-array-data-sheet-370-2016-016.pdf
Ji, Y., Biernacka, J. M., Hebbring, S., Chai, Y., Jenkins, G. D., Batzler, A., et al. (2013). Pharmacogenomics of Selective Serotonin Reuptake Inhibitor Treatment for Major Depressive Disorder: Genome-wide Associations and Functional Genomics. Pharmacogenomics J. 13, 456–463. doi:10.1038/tpj.2012.32
Jiang, M., and You, J. H. (2015). Review of Pharmacoeconomic Evaluation of Genotype-Guided Antiplatelet Therapy. Expert Opin. Pharmacother. 16, 771–779. doi:10.1517/14656566.2015.1013028
Johnson, J. A., Burkley, B. M., Langaee, T. Y., Clare-Salzler, M. J., Klein, T. E., and Altman, R. B. (2012). Implementing Personalized Medicine: Development of a Cost-Effective Customized Pharmacogenetics Genotyping Array. Clin. Pharmacol. Ther. 92, 437–439. doi:10.1038/clpt.2012.125
Ka, S., Lee, S., Hong, J., Cho, Y., Sung, J., Kim, H.-N., et al. (2017). HLAscan: Genotyping of the HLA Region Using Next-Generation Sequencing Data. BMC bioinformatics 18, 1–11. doi:10.1186/s12859-017-1671-3
Kalia, S. S., Adelman, K., Adelman, K., Bale, S. J., Chung, W. K., Eng, C., et al. (2017). Recommendations for Reporting of Secondary Findings in Clinical Exome and Genome Sequencing, 2016 Update (ACMG SF v2.0): a Policy Statement of the American College of Medical Genetics and Genomics. Genet. Med. 19, 249–255. doi:10.1038/gim.2016.190
Karnes, J. H., Shaffer, C. M., Bastarache, L., Gaudieri, S., Glazer, A. M., Steiner, H. E., et al. (2017). Comparison of HLA Allelic Imputation Programs. PLoS One 12, e0172444. doi:10.1371/journal.pone.0172444
Kawaguchi, S., Higasa, K., Shimizu, M., Yamada, R., and Matsuda, F. (2017). HLA‐HD: An Accurate HLA Typing Algorithm for Next‐generation Sequencing Data. Hum. Mutat. 38, 788–797. doi:10.1002/humu.23230
Klasberg, S., Surendranath, V., Lange, V., and Schöfl, G. (2019). Bioinformatics Strategies, Challenges, and Opportunities for Next Generation Sequencing-Based HLA Genotyping. Transfus. Med. Hemother 46, 312–325. doi:10.1159/000502487
Klein, K., Tremmel, R., Winter, S., Fehr, S., Battke, F., Scheurenbrand, T., et al. (2019). A New Panel-Based Next-Generation Sequencing Method for ADME Genes Reveals Novel Associations of Common and Rare Variants with Expression in a Human Liver Cohort. Front. Genet. 10, 7. doi:10.3389/fgene.2019.00007
Klein, T. E., and Ritchie, M. D. (2018). PharmCAT: a Pharmacogenomics Clinical Annotation Tool. Clin. Pharmacol. Ther. 104, 19–22. doi:10.1002/cpt.928
Krebs, K., and Milani, L. (2019). Translating Pharmacogenomics into Clinical Decisions: Do Not Let the Perfect Be the Enemy of the Good. Hum. Genomics 13, 1–13. doi:10.1186/s40246-019-0229-z
L Rogers, S., Keeling, N. J., Giri, J., Gonzaludo, N., Jones, J. S., Glogowski, E., et al. (2020). PARC Report: a Health-Systems Focus on Reimbursement and Patient Access to Pharmacogenomics Testing. Pharmacogenomics 21, 785–796. doi:10.2217/pgs-2019-0192
Lam, Y. W. F. (2013). Scientific Challenges and Implementation Barriers to Translation of Pharmacogenomics in Clinical Practice. ISRN Pharmacol. 2013, 1–17. doi:10.1155/2013/641089
Larsen, J. B., and Rasmussen, J. B. (2017). Pharmacogenetic Testing Revisited: 5' Nuclease Real-Time Polymerase Chain Reaction Test Panels for Genotyping CYP2D6 and CYP2C19. Pharmgenomics Pers Med. 10, 115–128. doi:10.2147/PGPM.S131580
L. Blackburn, H., Schroeder, B., Turner, C., D. Shriver, C., L. Ellsworth, D., and E. Ellsworth, R. (2015). Management of Incidental Findings in the Era of Next-Generation Sequencing. Cg 16, 159–174. doi:10.2174/1389202916666150317232930
Lee, E. M. J., Xu, K., Mosbrook, E., Links, A., Guzman, J., Adams, D. R., et al. (2016). Pharmacogenomic Incidental Findings in 308 Families: The NIH Undiagnosed Diseases Program Experience. Genet. Med. 18, 1303–1307. doi:10.1038/gim.2016.47
Lee, S.-B., Wheeler, M. M., Patterson, K., Mcgee, S., Dalton, R., Woodahl, E. L., et al. (2019). Stargazer: a Software Tool for Calling star Alleles from Next-Generation Sequencing Data Using CYP2D6 as a Model. Genet. Med. 21, 361–372. doi:10.1038/s41436-018-0054-0
Lemieux Perreault, L.-P., Zaïd, N., Cameron, M., Mongrain, I., and Dubé, M.-P. (2018). Pharmacogenetic Content of Commercial Genome-wide Genotyping Arrays. Pharmacogenomics 19, 1159–1167. doi:10.2217/pgs-2017-0129
Liau, Y., Maggo, S., Miller, A. L., Pearson, J. F., Kennedy, M. A., and Cree, S. L. (2019). Nanopore Sequencing of the Pharmacogene CYP2D6 Allows Simultaneous Haplotyping and Detection of Duplications. Pharmacogenomics 20, 1033–1047. doi:10.1101/57628010.2217/pgs-2019-0080
Madian, A. G., Wheeler, H. E., Jones, R. B., and Dolan, M. E. (2012). Relating Human Genetic Variation to Variation in Drug Responses. Trends Genetics 28, 487–495. doi:10.1016/j.tig.2012.06.008
Mantere, T., Kersten, S., and Hoischen, A. (2019). Long-read Sequencing Emerging in Medical Genetics. Front. Genet. 10, 426. doi:10.3389/fgene.2019.00426
Marques, S. C., and Ikediobi, O. N. (2010). The Clinical Application of UGT1A1pharmacogenetic Testing: Gene-Environment Interactions. Hum. Genomics 4, 1–12. doi:10.1186/1479-7364-4-4-238
Martis, S., Mei, H., Vijzelaar, R., Edelmann, L., Desnick, R. J., and Scott, S. A. (2013). Multi-ethnic Cytochrome-P450 Copy Number Profiling: Novel Pharmacogenetic Alleles and Mechanism of Copy Number Variation Formation. Pharmacogenomics J. 13, 558–566. doi:10.1038/tpj.2012.48
Miller, D. T., Lee, K., Chung, W. K., Gordon, A. S., Herman, G. E., Klein, T. E., et al. (2021). ACMG SF v3. 0 List for Reporting of Secondary Findings in Clinical Exome and Genome Sequencing: a Policy Statement of the American College of Medical Genetics and Genomics (ACMG). Genet. Med., 1–10. doi:10.1038/s41436-021-01278-8
Mizzi, C., Peters, B., Mitropoulou, C., Mitropoulos, K., Katsila, T., Agarwal, M. R., et al. (2014). Personalized Pharmacogenomics Profiling Using Whole-Genome Sequencing. Pharmacogenomics 15, 1223–1234. doi:10.2217/pgs.14.102
Nakkam, N., Konyoung, P., Kanjanawart, S., Saksit, N., Kongpan, T., Khaeso, K., et al. (2018). HLA Pharmacogenetic Markers of Drug Hypersensitivity in a Thai Population. Front. Genet. 9, 277. doi:10.3389/fgene.2018.00277
Numanagić, I., Malikić, S., Pratt, V. M., Skaar, T. C., Flockhart, D. A., and Sahinalp, S. C. (2015). Cypiripi: Exact Genotyping of CYP2D6 Using High-Throughput Sequencing Data. Bioinformatics 31, i27–34. doi:10.1093/bioinformatics/btv232
Numanagić, I., Malikić, S., Ford, M., Qin, X., Toji, L., Radovich, M., et al. (2018). Allelic Decomposition and Exact Genotyping of Highly Polymorphic and Structurally Variant Genes. Nat. Commun. 9, 1–11. doi:10.1038/s41467-018-03273-1
Oscarson, M., Hidestrand, M., Johansson, I., and Ingelman-Sundberg, M. (1997). A Combination of Mutations in the CYP2D6*17(CYP2D6Z) Allele Causes Alterations in Enzyme Function. Mol. Pharmacol. 52, 1034–1040. doi:10.1124/mol.52.6.1034
Owen, R. P., Gong, L., Sagreiya, H., Klein, T. E., and Altman, R. B. (2010). VKORC1 Pharmacogenomics Summary. Pharmacogenetics and genomics 20, 642–644. doi:10.1097/FPC.0b013e32833433b6
Preissner, S., Kroll, K., Dunkel, M., Senger, C., Goldsobel, G., Kuzman, D., et al. (2010). SuperCYP: a Comprehensive Database on Cytochrome P450 Enzymes Including a Tool for Analysis of CYP-Drug Interactions. Nucleic Acids Res. 38, D237–D243. doi:10.1093/nar/gkp970
Price, M. J., Carson, A. R., Murray, S. S., Phillips, T., Janel, L., Tisch, R., et al. (2012). First Pharmacogenomic Analysis Using Whole Exome Sequencing to Identify Novel Genetic Determinants of Clopidogrel Response Variability: Results of the Genotype Information and Functional Testing (GIFT) Exome Study. J. Am. Coll. Cardiol. 59, E9. doi:10.1016/S0735-1097(12)60010-2
Rasmussen-Torvik, L. J., Almoguera, B., Doheny, K. F., Freimuth, R. R., Gordon, A. S., Hakonarson, H., et al. (2017). Concordance between Research Sequencing and Clinical Pharmacogenetic Genotyping in the eMERGE-PGx Study. J. Mol. Diagn. 19, 561–566. doi:10.1016/j.jmoldx.2017.04.002
Reisberg, S., Krebs, K., Lepamets, M., Kals, M., Mägi, R., Metsalu, K., et al. (2019). Translating Genotype Data of 44,000 Biobank Participants into Clinical Pharmacogenetic Recommendations: Challenges and Solutions. Genet. Med. 21, 1345–1354. doi:10.1038/s41436-018-0337-5
Relling, M. V., and Klein, T. E. (2011). CPIC: Clinical Pharmacogenetics Implementation Consortium of the Pharmacogenomics Research Network. Clin. Pharmacol. Ther. 89, 464–467. doi:10.1038/clpt.2010.279
Sabater, A., Ciudad, C., Cendros, M., Dobrokhotov, D., and Sabater-Tobella, J. (2019). G-Nomic: a New Pharmacogenetics Interpretation Software. Pgpm Vol. 12, 75–85. doi:10.2147/PGPM.S203585
Saminathan, R., Bai, J., Sadrolodabaee, L., Karthik, G. M., Singh, O., Subramaniyan, K., et al. (2010). VKORC1 Pharmacogenetics and Pharmacoproteomics in Patients on Warfarin Anticoagulant Therapy: Transthyretin Precursor as a Potential Biomarker. PLoS One 5, e15064. doi:10.1371/journal.pone.0015064
Sangkuhl, K., Whirl‐Carrillo, M., Whaley, R. M., Woon, M., Lavertu, A., Altman, R. B., et al. (2020). Pharmacogenomics Clinical Annotation Tool (Pharm CAT ). Clin. Pharmacol. Ther. 107, 203–210. doi:10.1002/cpt.1568
Santos, M., Niemi, M., Hiratsuka, M., Kumondai, M., Ingelman-Sundberg, M., Lauschke, V. M., et al. (2018). Novel Copy-Number Variations in Pharmacogenes Contribute to Interindividual Differences in Drug Pharmacokinetics. Genet. Med. 20, 622–629. doi:10.1038/gim.2017.156
Schärfe, C. P. I., Tremmel, R., Schwab, M., Kohlbacher, O., and Marks, D. S. (2017). Genetic Variation in Human Drug-Related Genes. Genome Med. 9, 117. doi:10.1186/s13073-017-0502-5
Schwarz, U. I., Gulilat, M., and Kim, R. B. (2019). The Role of Next-Generation Sequencing in Pharmacogenetics and Pharmacogenomics. Cold Spring Harb Perspect. Med. 9, a033027. doi:10.1101/cshperspect.a033027
Shrestha, S., Zhang, C., Jerde, C. R., Nie, Q., Li, H., Offer, S. M., et al. (2018). Gene-Specific Variant Classifier (DPYD-Varifier) to Identify Deleterious Alleles of Dihydropyrimidine Dehydrogenase. Clin. Pharmacol. Ther. 104, 709–718. doi:10.1002/cpt.1020
Shu, Y., Leabman, M. K., Feng, B., Mangravite, L. M., Huang, C. C., Stryke, D., et al. (2003). Evolutionary Conservation Predicts Function of Variants of the Human Organic Cation Transporter, OCT1. Proc. Natl. Acad. Sci. 100, 5902–5907. doi:10.1073/pnas.0730858100
Sivadas, A., Salleh, M. Z., Teh, L. K., and Scaria, V. (2017). Genetic Epidemiology of Pharmacogenetic Variants in South East Asian Malays Using Whole-Genome Sequences. Pharmacogenomics J. 17, 461–470. doi:10.1038/tpj.2016.39
Suarez-Kurtz, G., and Parra, E. J. (2018). “Population Diversity in Pharmacogenetics: a Latin American Perspective,” in Advances in Pharmacology (Elsevier), 133–154. doi:10.1016/bs.apha.2018.02.001
Svidnicki, M. C. C. M., Zanetta, G. K., Congrains-Castillo, A., Costa, F. F., and Saad, S. T. O. (2020). Targeted Next-Generation Sequencing Identified Novel Mutations Associated with Hereditary Anemias in Brazil. Ann. Hematol. 99, 955–962. doi:10.1007/s00277-020-03986-8
Swen, J. J., Nijenhuis, M., de Boer, A., Grandia, L., Maitland-van der Zee, A. H., Mulder, H., et al. (2011). Pharmacogenetics: From Bench to Byte- an Update of Guidelines. Clin. Pharmacol. Ther. 89, 662–673. doi:10.1038/clpt.2011.34
Tafazoli, A., Wawrusiewicz-Kurylonek, N., Posmyk, R., and Miltyk, W. (2021). Pharmacogenomics, How to Deal with Different Types of Variants in Next Generation Sequencing Data in the Personalized Medicine Area. Jcm 10, 34. doi:10.3390/jcm10010034
Taylor, C., Crosby, I., Yip, V., Maguire, P., Pirmohamed, M., and Turner, R. M. (2020). A Review of the Important Role of CYP2D6 in Pharmacogenomics. Genes 11, 1295. doi:10.3390/genes11111295
Thermofisher.Com/Pharmacoscan (2018). Verification of Buccal Swab and Saliva Sample Types for PharmacoScan Solution.
Twesigomwe, D., Wright, G. E., Drögemöller, B. I., Da Rocha, J., Lombard, Z., and Hazelhurst, S. (2020). A Systematic Comparison of Pharmacogene star Allele Calling Bioinformatics Algorithms: a Focus on CYP2D6 Genotyping. NPJ Genomic Med. 5, 1–11. doi:10.1038/s41525-020-0135-2
Twist, G. P., Gaedigk, A., Miller, N. A., Farrow, E. G., Willig, L. K., Dinwiddie, D. L., et al. (2016). Constellation: a Tool for Rapid, Automated Phenotype Assignment of a Highly Polymorphic Pharmacogene, CYP2D6, from Whole-Genome Sequences. Npj Genomic Med. 1, 1–10. doi:10.1038/npjgenmed.2015.7
Twist, G. P., Gaedigk, A., Miller, N. A., Farrow, E. G., Willig, L. K., Dinwiddie, D. L., et al. (2017). Erratum: Constellation: a Tool for Rapid, Automated Phenotype Assignment of a Highly Polymorphic Pharmacogene, CYP2D6, from Whole-Genome Sequences. Npj Genomic Med. 2, 16039. doi:10.1038/npjgenmed.2016.39
van der Lee, M., Allard, W. G., Bollen, S., Santen, G. W. E., Ruivenkamp, C. A. L., Hoffer, M. J. V., et al. (2020a). Repurposing of Diagnostic Whole Exome Sequencing Data of 1,583 Individuals for Clinical Pharmacogenetics. Clin. Pharmacol. Ther. 107, 617–627. doi:10.1002/cpt.1665
Van Der Lee, M., Kriek, M., Guchelaar, H.-J., and Swen, J. J. (2020b). Technologies for Pharmacogenomics: A Review. Genes 11, 1456. doi:10.3390/genes11121456
Walczak, M., Skrzypczak-Zielinska, M., Plucinska, M., Zakerska-Banaszak, O., Marszalek, D., Lykowska-Szuber, L., et al. (2019). Long-range PCR Libraries and Next-Generation Sequencing for Pharmacogenetic Studies of Patients Treated with Anti-TNF Drugs. Pharmacogenomics J. 19, 358–367. doi:10.1038/s41397-018-0058-9
Wennerholm, A., Dandara, C., Sayi, J., Svensson, J. O., Abdi, Y. A., Ingelman‐Sundberg, M., et al. (2002). The African-specific CYP2D6*17 Allele Encodes an Enzyme with Changed Substrate Specificity. Clin. Pharmacol. Ther. 71, 77–88. doi:10.1067/mcp.2002.120239
Westbrook, M. J., Wright, M. F., Van Driest, S. L., Mcgregor, T. L., Denny, J. C., Zuvich, R. L., et al. (2013). Mapping the Incidentalome: Estimating Incidental Findings Generated through Clinical Pharmacogenomics Testing. Genet. Med. 15, 325–331. doi:10.1038/gim.2012.147
Wouden, C. H., Van Rhenen, M. H., Jama, W. O. M., Ingelman‐Sundberg, M., Lauschke, V. M., Konta, L., et al. (2019). Development of the PG x‐Passport: A Panel of Actionable Germline Genetic Variants for Pre‐Emptive Pharmacogenetic Testing. Clin. Pharmacol. Ther. 106, 866–873. doi:10.1002/cpt.1489
Xie, C., Yeo, Z. X., Wong, M., Piper, J., Long, T., Kirkness, E. F., et al. (2017). Fast and Accurate HLA Typing from Short-Read Next-Generation Sequence Data with xHLA. Proc. Natl. Acad. Sci. USA 114, 8059–8064. doi:10.1073/pnas.1707945114
Yang, Y., Botton, M. R., Scott, E. R., and Scott, S. A. (2017). Sequencing theCYP2D6gene: from Variant Allele Discovery to Clinical Pharmacogenetic Testing. Pharmacogenomics 18, 673–685. doi:10.2217/pgs-2017-0033
Zhou, Y., Fujikura, K., Mkrtchian, S., and Lauschke, V. M. (2018). Computational Methods for the Pharmacogenetic Interpretation of Next Generation Sequencing Data. Front. Pharmacol. 9, 1437. doi:10.3389/fphar.2018.01437
Zhou, Y., Mkrtchian, S., Kumondai, M., Hiratsuka, M., and Lauschke, V. M. (2019). An Optimized Prediction Framework to Assess the Functional Impact of Pharmacogenetic Variants. Pharmacogenomics J. 19, 115–126. doi:10.1038/s41397-018-0044-2
Zidan, A. M., Saad, E. A., Ibrahim, N. E., Mahmoud, A., Hashem, M. H., and Hemeida, A. A. (2020). PHARMIP: An Insilico Method to Predict Genetics that Underpin Adverse Drug Reactions. MethodsX 7, 100775. doi:10.1016/j.mex.2019.100775
Appendix 1: mini-glossary of the ngs terminologies used in this article
Keywords: pharmacogenomics, clinical implementation, next generation sequencing, clinical practice, PGx testing
Citation: Tafazoli A, Guchelaar H-J, Miltyk W, Kretowski AJ and Swen JJ (2021) Applying Next-Generation Sequencing Platforms for Pharmacogenomic Testing in Clinical Practice. Front. Pharmacol. 12:693453. doi: 10.3389/fphar.2021.693453
Received: 11 April 2021; Accepted: 26 July 2021;
Published: 25 August 2021.
Edited by:
Martin A. Kennedy, University of Otago, New ZealandReviewed by:
Eng Wee Chua, Universiti Kebangsaan, MalaysiaChiara Fabbri, King’s College London, United Kingdom
Copyright © 2021 Tafazoli, Guchelaar, Miltyk, Kretowski and Swen. This is an open-access article distributed under the terms of the Creative Commons Attribution License (CC BY). The use, distribution or reproduction in other forums is permitted, provided the original author(s) and the copyright owner(s) are credited and that the original publication in this journal is cited, in accordance with accepted academic practice. No use, distribution or reproduction is permitted which does not comply with these terms.
*Correspondence: Jesse J. Swen, ai5qLnN3ZW5AbHVtYy5ubA==