- 1Neurology and Epileptology, Hertie Institute for Clinical Brain Research, University of Tübingen, Tübingen, Germany
- 2Department of Epileptology and Neurology, University of Aachen, Aachen, Germany
- 3Department of Molecular and Cellular Therapeutics, Royal College of Surgeons in Ireland, Dublin, Ireland
- 4FutureNeuro Research Centre, Science Foundation Ireland, Dublin, Ireland
- 5Division of Neurology, Beaumont Hospital, Dublin, Ireland
- 6Department of Neurology, Hôpital Erasme, Université Libre de Bruxelles, Brussels, Belgium
- 7Division of Brain Sciences, Imperial College Faculty of Medicine, London, United Kingdom
- 8Department of Genetics, University Medical Center Utrecht, Utrecht, Netherlands
- 9Luxembourg Centre for Systems Biomedicine, University of Luxembourg, Esch-sur-Alzette, Luxembourg
- 10Institute of Experimental Epileptology and Cognition Research and Department of Epileptology, University of Bonn, Bonn, Germany
- 11Department of Molecular and Clinical Pharmacology, Institute of Translational Medicine, University of Liverpool, Liverpool, United Kingdom
- 12The Walton Centre NHS Foundation Trust, Liverpool, United Kingdom
- 13Liverpool Health Partners, Liverpool, United Kingdom
- 14Department of Clinical and Experimental Epilepsy, UCL Queen Square Institute of Neurology, London, United Kingdom
- 15Chalfont Centre for Epilepsy, Chalfont-St-Peter, United Kingdom
- 16Stichting Epilepsie Instellingen Nederland (SEIN), Heemstede, Netherlands
- 17School of Life Sciences, University of Glasgow, Glasgow, United Kingdom
- 18IRCCS "G. Gaslini" Institute, Genova, Italy
- 19Department of Neurosciences, University of Genoa, Genova, Italy
Objective: Resistance to anti-seizure medications (ASMs) presents a significant hurdle in the treatment of people with epilepsy. Genetic markers for resistance to individual ASMs could support clinicians to make better-informed choices for their patients. In this study, we aimed to elucidate whether the response to individual ASMs was associated with common genetic variation.
Methods: A cohort of 3,649 individuals of European descent with epilepsy was deeply phenotyped and underwent single nucleotide polymorphism (SNP)-genotyping. We conducted genome-wide association analyses (GWASs) on responders to specific ASMs or groups of functionally related ASMs, using non-responders as controls. We performed a polygenic risk score (PRS) analyses based on risk variants for epilepsy and neuropsychiatric disorders and ASM resistance itself to delineate the polygenic burden of ASM-specific drug resistance.
Results: We identified several potential regions of interest but did not detect genome-wide significant loci for ASM-specific response. We did not find polygenic risk for epilepsy, neuropsychiatric disorders, and drug-resistance associated with drug response to specific ASMs or mechanistically related groups of ASMs.
Significance: This study could not ascertain the predictive value of common genetic variants for ASM responder status. The identified suggestive loci will need replication in future studies of a larger scale.
Introduction
About one-third of people with epilepsy have seizures that are refractory to anti-seizure medications (ASMs). The International League against Epilepsy (ILAE) defines drug resistance as ongoing seizures despite treatment with at least two well-tolerated and appropriate ASMs (Kwan et al., 2010). With each additional drug trial, the odds to achieve seizure freedom decrease (Brodie et al., 2012). The introduction of new ASMs with alternate mechanisms of action has not significantly changed this situation (Chen et al., 2018). For certain epilepsy syndromes, some ASMs have proven to be more beneficial than others: valproic acid (VPA) and ethosuximide are superior to lamotrigine (LTG) in childhood absence epilepsy (Glauser et al., 2013), VPA is superior to topiramate (TPM) and LTG in genetic generalized epilepsy (GGE) (Marson et al., 2007a; Silvennoinen et al., 2019), and carbamazepine (CBZ) and LTG are superior to TPM and gabapentin (GBP) in focal epilepsy (Marson et al., 2007b). Yet, studies with head-to-head comparisons are sparse (Beyenburg et al., 2010; Androsova et al., 2017). Thus, in clinical practice, ASMs are prescribed based on age, gender, co-morbidities, electroclinical syndrome, seizure type, potential drug interactions, or adverse drug reactions.
Pharmacogenomics, i.e. the influence of genetic variants on drug response or adverse effects, bear the potential to support the choice of the most suitable ASM (Löscher et al., 2009). Other medical fields have seen the integration of pharmacogenomics in clinical routine (Daly, 2017). For epilepsies, reproducible pharmacogenomic findings are limited to cutaneous adverse reactions caused by aromatic ASMs (Chung et al., 2004; McCormack et al., 2011; McCormack et al., 2018). The utility of these findings in individuals’ care remains a matter of debate (Chen et al., 2014). The endeavor to identify common genetic variants associated with drug response is still elusive, also due to small sample sizes (Heavin et al., 2019; Wolking et al., 2020a). There is some evidence that enrichment of ultra-rare variants in genes associated with pharmacodynamics and pharmacokinetics can modify ASM response, but further replication of these results is needed (Wolking et al., 2020a).
We assessed common variants' role and common variant burden for drug response to common ASMs using genome-wide association studies (GWAS) and polygenic risk score (PRS) analyses in a cohort of 3,649 individuals.
Methods
Ethics Statement
All study participants provided written, informed consent for genetic analyses. Local institutional review boards reviewed and approved study protocols at each contributing site.
Study Design
This cohort was derived from the EpiPGX Consortium established in 2012 to identify genetic biomarkers of epilepsy treatment response and adverse drug reactions. EpiPGX is a European-wide epilepsy research partnership under the European Commission Seventh Framework Protocol (FP7). This case-control study was based on the retrospective evaluation of individual data. Relevant data were extracted from case charts by trained personnel and collected in a standard electronic case report form (eCRF) used at all consortium sites. Our cohorts consisted exclusively of individuals of non-Finnish European ancestry with an established diagnosis of either focal or genetic generalized epilepsy according to current ILAE diagnostic criteria (Scheffer et al., 2017). We tested whether common genetic variants were significantly associated with drug response to one ASM or groups of mechanistically related ASMs (sodium channel-active and calcium channel-active ASMs). We also tested whether the response profile was associated with an increased burden of polygenic variants for risk of epilepsy syndromes, other neuropsychiatric disorders, or whether a burden of risk variants for drug response itself could predict the outcome.
ASMs were selected based on their usage in the EpiPGX cohort. ASM-specific analysis was performed for levetiracetam (LEV), lamotrigine (LTG), valproic acid (VPA) for focal epilepsies and all epilepsies. For focal epilepsies only, we performed additional ASM-specific GWAS for phenytoin (PHT), oxcarbazepine (OXC), and carbamazepine (CBZ). ASM groups comprised T-type calcium channel-active ASMs (valproic acid, ethosuximide, and zonisamide [ZNS]) for focal and all epilepsies; and sodium channel-active ASMs (LTG, lacosamide [LCM], ZNS, PHT, CBZ, OXC, and eslicarbazepine [ESL]) for focal epilepsies only. The breakdown of the sample size per analysis is depicted in Table 1.
Cohorts and Phenotype Definition
The individuals in this study were selected from more than 12.000 individuals that were documented in the EpiPGX eCRF. Thereof, 3,649 individuals fulfilled the inclusion criteria, 2,762 with focal epilepsy, and 887 with generalized genetic epilepsy. The latter group has been part of a previous study (Wolking et al., 2020a). A more detailed cohort description is provided in Table 1.
Individuals were classified as responders or non-responders. The response was defined as seizure freedom under ongoing treatment for at least one year and before initiation of any other treatment; non-response as recurring seizures at ≥ 50% of pretreatment seizure frequency given adequate dosage of the trial drug. Individuals with recurrent non-compliance for ASM intake were excluded. The response or non-response groups' assignment was based on the evaluation of one or more epilepsy specialists at the source center. To harmonize phenotyping procedures a phenotyping manual was created at the start of the EpiPGX project. At the beginning and on a yearly basis throughout the recruitment phase phenotyping workshops were held. To assess cross-center consistency of data interpretation, a cross-center phenotyping validation test was performed at the outset of the EpiPGX project, using anonymized medical records. An overall inter-rater agreement of 74.2% was reached. Stark disagreement, e.g ASM response vs. non-response, occurred in 5.1% of recorded ASM trials.
Imputation and Genotyping Quality Controls
GWASs were conducted separately for each ASM-response cohort using imputed genotypes. Genotyping of a subset of samples was performed at deCODE Genetics on Illumina OmniExpress-12 v1.1 and -24 v1.1 single nucleotide polymorphism (SNP) arrays. The remainder of samples were genotyped locally on various Illumina beadchip SNP arrays. Detailed genotyping, imputation and quality control methods have been described previously (McCormack et al., 2018). Population structure was controlled via principal component analysis as reported previously (Wolking et al., 2020a) (Supplementary Figure S1). European ancestry was established by a principal component analysis comparison to 1,000 genomes data (Supplementary Figure S2).
Genome-wide Association Analysis
GWAS power was calculated using PGA (Menashe et al., 2008). Association analysis was performed using SNPTEST in a frequentist model with the top 10 main components, sex, and epilepsy subtype (where appropriate) included as covariates. The statistical threshold for genome-wide significance was set at p < 5 × 10–8. Post-association QC removed SNPs with INFO scores lower than 0.95, missingness rates >0.10, Hardy-Weinberg deviations p < 5 × 10–6, and minor allele frequencies <5%.
Study Power
We estimated that our most extensive analysis for sodium channel-active ASMs had 80% power to detect a genetic predictor of the relative risk of 1.37 with an allele frequency of ≥20%, based on an α level of 5 × 10–8 and given a prevalence of drug-resistance of 30%. The study power for the other analyses is shown in Table 1.
Polygenic Risk Score Analysis for Epilepsy and Neuropsychiatric Disorders
GWAS summary statistics for epilepsy (focal, GGE, and all epilepsies) were downloaded from the ILAE study (Cross-Disorder Group of the Psychiatric Genomics Consortium, 2013) using the EpiGAD server. These statistics were remade with the overlapping samples between the larger ILAE cohort and our EpiPGX samples removed. GWAS results for a broad psychiatric disorder study (covering autism, attention deficit hyperactivity disorder, bipolar disorder, major depression and schizophrenia) were downloaded from the psychiatric genomics consortium (International League Against Epilepsy Consortium on Complex Epilepsies, 2018). PRS for each phenotype were calculated for all samples our study cohorts using PRSice (Euesden et al., 2015), using all SNPs from the base GWAS with p-values ≤0.5. The threshold of ≤0.5 was selected because for most complex traits the most predictive p-thresholds will typically be between 0.3 and 0.5, including epilepsy (Leu et al., 2019). The PRS were then regressed onto responder status using R 3.6, with the top six principle components, sex, and epilepsy subtype (where appropriate) included as covariates.
Polygenic Risk Score Analysis for Drug Response
To test whether responsiveness to individuals ASMs or groups of ASMs had a distinct polygenic component, we split our cohorts into a discovery and replication cohort, depending on recruitment site (Test: 636 cases, 890 controls; discovery: 229 cases, 323 controls). A GWAS was run in the test cohort (following the protocol from above) and used a PRS analysis base in the discovery cohort (same methods as above).
SNP-Heritability Testing
Linkage disequilibrium score-regression (Bulik-Sullivan et al., 2015) was used to calculate SNP-based heritability in the cohort of sodium-channel actives ASM treated study participants. We also used GCTA-GREML to estimate the heritability (Yang et al., 2011).
Results
Cohort Description
After per individual quality check, 3,649 individuals were included in the GWAS analyses. The breakdown of the GWAS cohorts is shown in Table 1. The proportion of individuals with GGE was 25%. For the GWAS for VPA response and in consequence for T-type calcium channel-active ASMs (including VPA, ESX, and ZNS), GGE was overrepresented in the responder group. The mean age of onset tended to be higher for responders than non-responders except for VPA and T-type calcium channel-active ASMs.
Genome-wide Association Studies for Drug Response
We performed GWAS for drug response for specific ASMs and groups of ASMs (as shown in Table 1) for focal epilepsy and all epilepsies. Results for GGE alone have been published previously (Wolking et al., 2020b). We found no evidence for a relevant GWAS p-value inflation (lambda-range between 0.99 and 1.06). We did not find any genome-wide markers that exceeded the significance threshold (5 × 10–8). We identified 30 loci suggestive for an association with ASM response (<5 × 10–6) as shown in Table 2. To exemplify the findings, QQ- and Manhattan plots for the largest GWAS of sodium channel-active ASMs are shown in Figures 1A,B; the results of the other GWAS are depicted in Supplementary Figures S3 to S12.
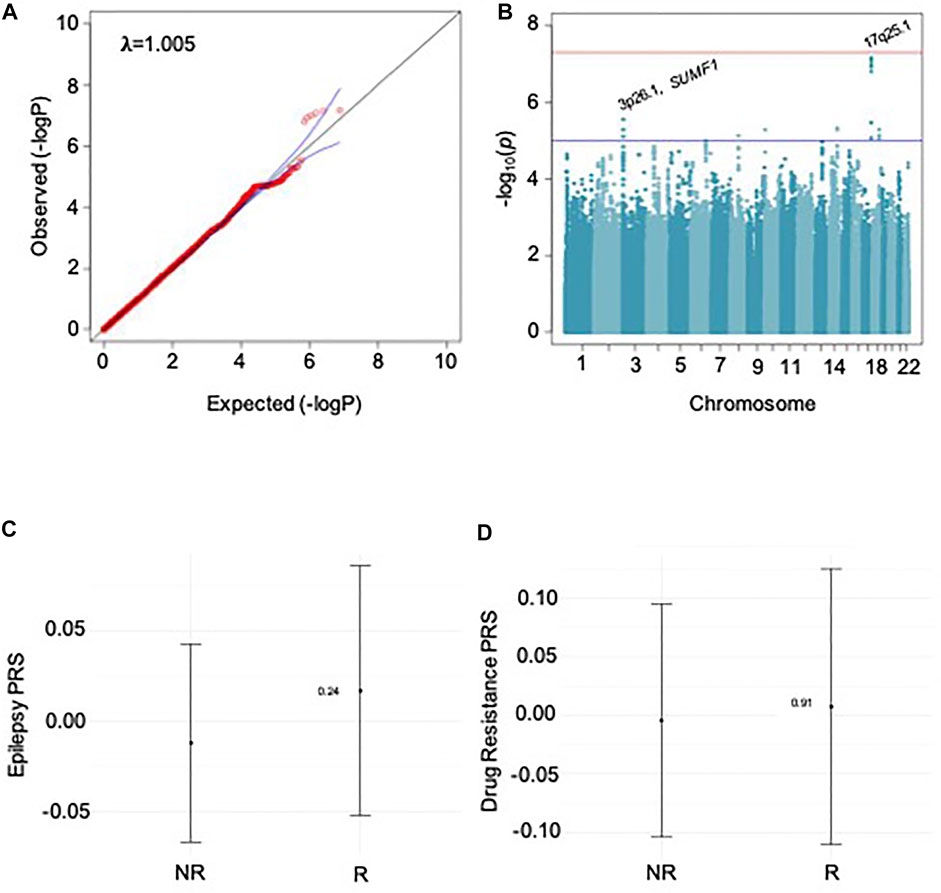
FIGURE 1. Results for sodium channel-active ASMs. A and B show results for GWAS, C and D for PRS analysis. A: QQ plot shows no relevant p-value inflation; lambda-value = 1.005. B: Manhattan plot of GWAS; locations of SNPs with p-value ≤ 5 × 10–6 are marked. C: Results for PRS-analysis PRS for epilepsy risk based on the ILAE 2018 metadata. D: Results for PRS-analysis for PRS for risk of ASM-specific drug response.
SNP-Heritability Testing
We calculated SNP-based heritability [SNP-h2] as a measure of the proportion of variance in ASM response status, which could be attributed to common genetic variants for the largest cohort of samples treated with sodium-blocking ASMs. The result was not significant, SNP-h2 was estimated to be 0.3108, with a standard error of 0.2868 (Lower CI: −0.252, Upper CI: 0.873). Using GCTA-GREML to calculate h2, the result was not significant [h2 = 0.000002, standard error = 0.178,925, p = 0.5].
Assessing the Polygenic Risk of Epilepsy and Neuropsychiatric Disorders for Drug Response
First, we tested whether the responder status to individual ASMs and the groups of sodium channel active-, and T-type calcium channel-active ASMs correlated with the genetic load for epilepsy (focal, generalized, and combined), Figure 1C. Second, we tested whether the responder status correlated with the genetic load for five neuropsychiatric disorders was associated with responder status. In both cases, we found no significant association of polygenic risk scores with any ASM drug-responder status.
Third, we assumed that the responder status itself harbored a polygenic component, which is largely distinct from the polygenic component for epilepsy risk. We split the ASM cohorts in half to calculate a GWAS for the first half. This discovery cohort was used to calculate PRS for individual ASM responder status in the second half. We also did not find a significant association for drug response PRS with responder status (Figure 1D).
Discussion
We tested whether common genetic variation could predict drug response to various commonly used ASMs. We identified several loci of potential interest for ASM response but found no significant genome-wide association. Our analysis was underpowered to detect small effect size variants, but the results suggest that there are no large-effect size variants associated with drug response. We further tested whether the polygenic burden for epilepsy risk, risk for various neuropsychiatric disorders, or drug-resistance itself had a predictive value for the drug response phenotype. We could not show that polygenic risk scores were significantly associated with ASM response within the limits of study size. Other methods of PRS calculation also exist, such as LDpred (Vilhjálmsson et al., 2015), which may prove more successful at finding polygenic signals associated with drug response to ASMs and could be further explored in future studies.
This study was limited to the sample size of the sub-analyses. This study does not prove that drug response is without genetic influence. The results could imply that drug response is a far more complex trait with multiple influencing parameters beyond genomic factors alone. While PRS for epilepsy is a reliable predictor for the risk of epilepsy and epilepsy sub-phenotypes itself (Leu et al., 2019; Moreau et al., 2020), this approach was not beneficial to predict drug response within this study's limitations.
The results align with our previous studies that found no common genetic variants in association to VPA, LTG, and LEV response in genetic generalized epilepsy (Wolking et al., 2020b) or for the response to lacosamide in focal epilepsy (Heavin et al., 2019). One previous study suggests that rare genetic variants in genes related to drug targets and pharmacokinetics might be involved (Wolking et al., 2020b). Given that many individuals with epilepsy exhibit a broad pharmacoresistance, regardless of the drugs’ mechanism of action, other factors are probably at play (Löscher et al., 2020). Epigenetic mechanisms such as altered DNA methylation (Kobow et al., 2013), seizure-induced alterations of neural networks (Fang et al., 2011), or intrinsic factors mediating disease severity (Rogawski, 2013) should be further explored.
Conclusion
No genome-wide significant variants could be identified in association with drug response to various widely used ASMs. We identified several suggestive risk loci. Future hypothesis-driven association studies should attempt to reproduce our findings.
EpiPGX-CONSORTIUM
Andreja Avbersek, Costin Leu, Kristin Heggeli, Rita Demurtas, Joseph Willis, Douglas Speed, Narek Sargsyan, Krishna Chinthapalli, Mojgansadat Borghei, Antonietta Coppola, Antonio Gambardella, Stefan Wolking, Felicitas Becker, Sarah Rau, Christian Hengsbach, Yvonne G. Weber, Bianca Berghuis, Wolfram S. Kunz, Mark McCormack, Norman Delanty, Ellen Campbell, Lárus J. Gudmundsson, Andres Ingason, Kári Stefánsson, Reinhard Schneider, Rudi Balling, Pauls Auce, Ben Francis, Andrea Jorgensen, Andrew Morris, Sarah Langley, Prashant Srivastava, Martin Brodie, Marian Todaro, Slave Petrovski, Jane Hutton, Fritz Zimprich, Martin Krenn, Hiltrud Muhle, Karl Martin Klein, Rikke S Møller, Marina Nikanorova, Sarah Weckhuysen, Zvonka Rener-Primec, Gianpiero L. Cavalleri, John Craig, Chantal Depondt, Michael R. Johnson, Bobby P. C. Koeleman, Roland Krause, Holger Lerche, Anthony G. Marson, Terence J. O’Brien, Slave Petrovski, Samuel F. Berkovic, Josemir W. Sander, Graeme J. Sills, Hreinn Stefansson, Pasquale Striano, Federico Zara, and Sanjay M. Sisodiya
Data Availability Statement
The original contributions presented in the study are included in the article/Supplementary Material. The raw SNP datasets presented in this article are not readily available due to ethical and privacy restrictions. Further inquiries should be directed to the corresponding author.
Ethics Statement
The studies involving human participants were reviewed and approved by Ethics Comittee, University Hospital Tübingen and other ethics comittees of recruitment sites. Written informed consent to participate in this study was provided by the participants' legal guardian/next of kin.
Author Contributions
SW, CC, GLC, and HL conceived the study concept and design. SW, CC, HL, SS, JS, PS wrote the manuscript. SW, CC, CS, GLC, and MM performed the analyses. SW, ND, CD, MJ, BK, RK, WK, AM, JS, GS, PS, FZ, SS, GC, and HL acquired the data and oversaw phenotyping. All authors interpreted the data. HL, SS, and GC supervised the study.
Funding
The EpiPGX Consortium was funded by FP7 grant 279062 “EpiPGX” from the European Commission. SW received funding from the German Research Foundation (DFG) (WO 2385/1-1).
Conflict of Interest
The authors declare that the research was conducted in the absence of any commercial or financial relationships that could be construed as a potential conflict of interest.
Supplementary Material
The Supplementary Material for this article can be found online at: https://www.frontiersin.org/articles/10.3389/fphar.2021.688386/full#supplementary-material
References
Androsova, G., Krause, R., Borghei, M., Wassenaar, M., Auce, P., Avbersek, A., et al. (2017). Comparative Effectiveness of Antiepileptic Drugs in Patients with Mesial Temporal Lobe Epilepsy with Hippocampal Sclerosis. Epilepsia 58 (10), 1734–1741. doi:10.1111/epi.13871
Beyenburg, S., Stavem, K., and Schmidt, D. (2010). Placebo-corrected Efficacy of Modern Antiepileptic Drugs for Refractory Epilepsy: Systematic Review and Meta-Analysis. Epilepsia 51 (1), 7–26. doi:10.1111/j.1528-1167.2009.02299.x
Brodie, M. J., Barry, S. J. E., Bamagous, G. A., Norrie, J. D., and Kwan, P. (2012). Patterns of Treatment Response in Newly Diagnosed Epilepsy. Neurology 78 (20), 1548–1554. doi:10.1212/wnl.0b013e3182563b19
Bulik-Sullivan, B. K., Loh, P-R., Loh, P.-R., Finucane, H. K., Ripke, S., Yang, J., et al. (2015). LD Score Regression Distinguishes Confounding from Polygenicity in Genome-wide Association Studies. Nat. Genet. 47 (3), 291–295. doi:10.1038/ng.3211
Chen, Z., Liew, D., and Kwan, P. (2014). Effects of a HLA-B*15:02 Screening Policy on Antiepileptic Drug Use and Severe Skin Reactions. Neurology 83 (22), 2077–2084. doi:10.1212/wnl.0000000000001034
Chen, Z., Brodie, M. J., Liew, D., and Kwan, P. (2018). Treatment Outcomes in Patients with Newly Diagnosed Epilepsy Treated with Established and New Antiepileptic Drugs. JAMA Neurol. 75 (3), 279–286. doi:10.1001/jamaneurol.2017.3949
Chung, W.-H., Hung, S.-I., Hong, H.-S., Hsih, M.-S., Yang, L.-C., Ho, H.-C., et al. (2004). A Marker for Stevens-Johnson Syndrome. Nature 428 (6982), 486. doi:10.1038/428486a
Cross-Disorder Group of the Psychiatric Genomics Consortium (2013). Identification of Risk Loci with Shared Effects on Five Major Psychiatric Disorders: a Genome-wide Analysis. Lancet 381 (9875), 1371–1379. doi:10.1016/S0140-6736(12)62129-1
Daly, A. K. (2017). Pharmacogenetics: a General Review on Progress to Date. Br. Med. Bull., 124, 1–15. doi:10.1093/bmb/ldx035
Euesden, J., Lewis, C. M., and O’Reilly, P. F. (2015). PRSice: Polygenic Risk Score Software. Bioinformatics 31 (9), 1466–1468. doi:10.1093/bioinformatics/btu848
Fang, M., Xi, Z.-Q., Wu, Y., and Wang, X.-F. (2011). A New Hypothesis of Drug Refractory Epilepsy: Neural Network Hypothesis. Med. Hypotheses 76 (6), 871–876. doi:10.1016/j.mehy.2011.02.039
Glauser, T. A., Cnaan, A., Shinnar, S., Hirtz, D. G., Dlugos, D., Masur, D., et al. (2013). Ethosuximide, Valproic Acid, and Lamotrigine in Childhood Absence Epilepsy: Initial Monotherapy Outcomes at 12 Months. Epilepsia 54 (1), 141–155. doi:10.1111/epi.12028
Heavin, S. B., McCormack, M., Wolking, S., Slattery, L., Walley, N., Avbersek, A., et al. (2019). Genomic and Clinical Predictors of Lacosamide Response in Refractory Epilepsies. Available:at: https://onlinelibrary.wiley.com/doi/abs/10.1002/epi4.12360.
International League Against Epilepsy Consortium on Complex Epilepsies (2018). Genome-wide Mega-Analysis Identifies 16 Loci and Highlights Diverse Biological Mechanisms in the Common Epilepsies. Nat. Commun. 9 (1), 5269. doi:10.1038/s41467-018-07524-z
Kobow, K., El-Osta, A., and Blümcke, I. (2013). The Methylation Hypothesis of Pharmacoresistance in Epilepsy. Epilepsia 54 (Suppl. 2), 41–47. doi:10.1111/epi.12183
Kwan, P., Arzimanoglou, A., Berg, A. T., Brodie, M. J., Allen Hauser, W., Mathern, G., et al. (2010). Definition of Drug Resistant Epilepsy: Consensus Proposal by the Ad Hoc Task Force of the ILAE Commission on Therapeutic Strategies. Epilepsia 51 (6), 1069–1077. doi:10.1111/j.1528-1167.2009.02397.x
Leu, C., Stevelink, R., Smith, A. W., Goleva, S. B., Kanai, M., Ferguson, L., et al. (2019). Polygenic burden in Focal and Generalized Epilepsies. Available:at: https://academic.oup.com/brain/advance-article/doi/10.1093/brain/awz292/5585821.
Löscher, W., Klotz, U., Zimprich, F., and Schmidt, D. (2009). The Clinical Impact of Pharmacogenetics on the Treatment of Epilepsy. Epilepsia 50 (1), 1–23. doi:10.1111/j.1528-1167.2008.01716.x
Löscher, W., Potschka, H., Sisodiya, S. M., and Vezzani, A. (2020). Drug Resistance in Epilepsy: Clinical Impact, Potential Mechanisms, and New Innovative Treatment Options. Pharmacol. Rev. 72 (3), 606–638. doi:10.1124/pr.120.019539
Marson, A. G., Al-Kharusi, A. M., Alwaidh, M., Appleton, R., Baker, G. A., Chadwick, D. W., et al. (2007a). The SANAD Study of Effectiveness of Carbamazepine, Gabapentin, Lamotrigine, Oxcarbazepine, or Topiramate for Treatment of Partial Epilepsy: an Unblinded Randomised Controlled Trial. The Lancet 369 (9566), 1000–1015. doi:10.1016/s0140-6736(07)60460-7
Marson, A. G., Al-Kharusi, A. M., Alwaidh, M., Appleton, R., Baker, G. A., Chadwick, D. W., et al. (2007b). The SANAD Study of Effectiveness of Valproate, Lamotrigine, or Topiramate for Generalised and Unclassifiable Epilepsy: an Unblinded Randomised Controlled Trial. The Lancet 369 (9566), 1016–1026. doi:10.1016/s0140-6736(07)60461-9
McCormack, M., Alfirevic, A., Bourgeois, S., Farrell, J. J., Kasperavičiūtė, D., Carrington, M., et al. (2011). HLA-A*3101 and Carbamazepine-Induced Hypersensitivity Reactions in Europeans. N. Engl. J. Med. 364 (12), 1134–1143. doi:10.1056/nejmoa1013297
McCormack, M., Gui, H., Ingason, A., Speed, D., Wright, G. E. B., Zhang, E. J., et al. (2018). Genetic Variation in CFH Predicts Phenytoin-Induced Maculopapular Exanthema in European-descent Patients. Neurology 90 (4), e332–e341. doi:10.1212/wnl.0000000000004853
Menashe, I., Rosenberg, P. S., and Chen, B. E. (2008). PGA: Power Calculator for Case-Control Genetic Association Analyses. BMC Genet. 9, 36. doi:10.1186/1471-2156-9-36
Moreau, C., Rébillard, R.-M., Wolking, S., Michaud, J., Tremblay, F., Girard, A., et al. (2020). Polygenic Risk Scores of Several Subtypes of Epilepsies in a Founder Population. Neurol. Genet. 6 (3), e416. doi:10.1212/nxg.0000000000000416
Rogawski, M. A. (2013). The Intrinsic Severity Hypothesis of Pharmacoresistance to Antiepileptic Drugs. Epilepsia 54, 33–40. doi:10.1111/epi.12182
Scheffer, I. E., Berkovic, S., Capovilla, G., Connolly, M. B., French, J., Guilhoto, L., et al. (2017). ILAE Classification of the Epilepsies: Position Paper of the ILAE Commission for Classification and Terminology. Epilepsia 58 (4), 512–521. doi:10.1111/epi.13709
Silvennoinen, K., Lange, N., Zagaglia, S., Balestrini, S., Androsova, G., Wassenaar, M., et al. (2019). Comparative Effectiveness of Antiepileptic Drugs in Juvenile Myoclonic Epilepsy. Available:at: https://onlinelibrary.wiley.com/doi/abs/10.1002/epi4.12349.
Vilhjálmsson, B. J., Yang, J., Finucane, H. K., Gusev, A., Lindström, S., Ripke, S., et al. (2015). Modeling Linkage Disequilibrium Increases Accuracy of Polygenic Risk Scores. Am. J. Hum. Genet. 97 (4), 576–592. doi:10.1016/j.ajhg.2015.09.001
Wolking, S., Schulz, H., Nies, A. T., McCormack, M., Schaeffeler, E., Auce, P., et al. (2020a). Pharmacoresponse in Genetic Generalized Epilepsy: a Genome-wide Association Study. Available:at: https://www.futuremedicine.com/doi/10.2217/pgs-2019-0179.
Wolking, S., Moreau, C., Nies, A. T., Schaeffeler, E., McCormack, M., Auce, P., et al. (2020b). Testing Association of Rare Genetic Variants with Resistance to Three Common Antiseizure Medications. Available:at: https://onlinelibrary.wiley.com/doi/abs/10.1111/epi.16467.
Keywords: drug-resistant epilepsies, polygenic risk score (PRS), GWAS, anti-seizure medication (ASM), single nucelotide polymorphisms
Citation: Wolking S, Campbell C, Stapleton C, McCormack M, Delanty N, Depondt C, Johnson MR, Koeleman BPC, Krause R, Kunz WS, Marson AG, Sander JW, Sills GJ, Striano P, Zara F, Sisodiya SM, Cavalleri GL and Lerche H (2021) Role of Common Genetic Variants for Drug-Resistance to Specific Anti-Seizure Medications. Front. Pharmacol. 12:688386. doi: 10.3389/fphar.2021.688386
Received: 30 March 2021; Accepted: 10 May 2021;
Published: 09 June 2021.
Edited by:
Heng Xu, Sichuan University, ChinaReviewed by:
Adrian Isaac Campos Gonzalez, The University of Queensland, AustraliaLifeng Tian, University of Pennsylvania, United States
Copyright © 2021 Wolking, Campbell, Stapleton, McCormack, Delanty, Depondt, Johnson, Koeleman, Krause, Kunz, Marson, Sander, Sills, Striano, Zara, Sisodiya, Cavalleri, Lerche and EpiPGX Consortium. This is an open-access article distributed under the terms of the Creative Commons Attribution License (CC BY). The use, distribution or reproduction in other forums is permitted, provided the original author(s) and the copyright owner(s) are credited and that the original publication in this journal is cited, in accordance with accepted academic practice. No use, distribution or reproduction is permitted which does not comply with these terms.
*Correspondence: Holger Lerche, SG9sZ2VyLmxlcmNoZUB1bmktdHVlYmluZ2VuLmRl
†These authors contributed equally to this work