- 1Department of Clinical Pharmacology, School of Medicine, Faculty of Health, Witten/Herdecke University, Witten, Germany
- 2Bayer AG, Research and Development, Clinical Pharmacometrics, Wuppertal, Germany
- 3Medical Clinic 1, Division of Nephrology, Helios University Hospital Wuppertal, Wuppertal, Germany
- 4Bayer AG, Research and Development, Systems Pharmacology and Medicine I, Leverkusen, Germany
- 5Philipp Klee-Institute for Clinical Pharmacology, Helios University Hospital Wuppertal, Wuppertal, Germany
This study compared simulations of a physiologically based pharmacokinetic (PBPK) model implemented for cyclosporine with drug levels from therapeutic drug monitoring to evaluate the predictive performance of a PBPK model in a clinical population. Based on a literature search model parameters were determined. After calibrating the model using the pharmacokinetic profiles of healthy volunteers, 356 cyclosporine trough levels of 32 renal transplant outpatients were predicted based on their biometric parameters. Model performance was assessed by calculating absolute and relative deviations of predicted and observed trough levels. The median absolute deviation was 6 ng/ml (interquartile range: 30 to 31 ng/ml, minimum = −379 ng/ml, maximum = 139 ng/ml). 86% of predicted cyclosporine trough levels deviated less than twofold from observed values. The high intra-individual variability of observed cyclosporine levels was not fully covered by the PBPK model. Perspectively, consideration of clinical and additional patient-related factors may improve the model’s performance. In summary, the current study has shown that PBPK modeling may offer valuable contributions for pharmacokinetic research in clinical drug therapy.
Introduction
Physiologically based pharmacokinetic (PBPK) models are mathematical representations of pharmacokinetic processes. In a bottom-up approach all determining physico-chemical and biological interactions between a drug and the body are implemented into virtual compartments, mapping the anatomical architecture and the physiological and biochemical properties of the body. Integration over time then allows for calculation of concentration-time curves of a modeled drug in specific body-compartments in silico (Kuepfer et al., 2016). While PBPK modeling has become an established methodical approach in drug development and latterly in regulatory decision making (Zhao et al., 2012), there is now an emerging use of PBPK modeling to simulate pathological conditions (Radke et al., 2016). Still, the predictive performance of PBPK models in specific clinical settings with heterogeneous and chronically ill patients characterized by numerous unknown individual and clinical factors needs to be further tested.
Cyclosporine is a drug with a high inter- and intraindividual pharmacokinetic variability and a narrow therapeutic window. As a cyclic oligopeptide (Rüegger et al., 1976) cyclosporine shows a poor water-solubility but high intestinal permeability (Amidon et al., 1995). It is predominantly a substrate of cytochrome P450 (CYP) 3A4 (Kronbach et al., 1988) and P-glycoprotein (P-GP) (Saeki et al., 1993). Due to a high protein binding predominantly to lipoproteins (Lemaire and Tillement, 1982), the fraction unbound in blood (fU) is low (1–17%) and depends on measurement methods and examined individuals (Akhlaghi and Trull, 2002). Cyclosporine shows saturable accumulation in blood cells and peripheral tissue resulting in a partially non-linear pharmacokinetic behavior (Foxwell et al., 1988; Tanaka et al., 2000). Moreover it shows a high affinity for fatty and lymphatic tissue (Kahan et al., 1983). Cyclosporine is eliminated almost exclusively in form of its metabolites via bile (Venkataramanan et al., 1985).
Since the early 1980’s cyclosporine has been used for immunosuppression after renal transplantation and can impressively reduce acute rejection episodes early after transplantation. Yet long-term allograft survival is still inadequate with a progressively decreasing allograft function occurring in most patients within 10 years after transplantation (Nankivell et al., 2003). Causes of so called chronic-allograft-injury include, among others, cyclosporine nephrotoxicity and immunological rejection of the transplant which should both be prevented by an optimal immunosuppressive regime. Although dosing and target levels are poorly researched (KDIGO Clinical Practice Guideline for the Kidney Disease Improving Global Outcomes, 2009) and must be adjusted in consideration of individual patient factors and (immunosuppressive) co-medication, a high variability of cyclosporine exposure might correlate with a worse long-term outcome (Kahan et al., 2000). In clinical practice cyclosporine blood levels are measured regularly and invasively via therapeutic drug monitoring (TDM) to avoid side effects, particularly nephrotoxicity, as well as rejection episodes and to adapt dosing. However, details on procedure and the actual benefit of TDM for efficacy and safety of cyclosporine therapy have been studied insufficiently (KDIGO Clinical Practice Guideline for the Kidney Disease Improving Global Outcomes, 2009).
PBPK modeling can serve to further investigate the complex pharmacokinetic of cyclosporine. In the 1990’s there have been several approaches to investigate the non-linear pharmacokinetic behavior of cyclosporine after intravenous application by PBPK modeling (Kawai et al., 1998; Tanaka et al., 1999). One model has been applied to analyze the intravenous pharmacokinetic of cyclosporine in children (Gérard et al., 2010). Attempts to describe the oral absorption of cyclosporine by whole-body PBPK modeling either publish incomplete data (Darwich et al., 2013) or rely on semi-mechanistic approaches only (Gertz et al., 2013). Drug levels from TDM allow to retrospectively evaluate the predictive ability of a PBPK model in clinical care (Polasek et al., 2018).
The aim of this study was to implement a PBPK model for the oral application of cyclosporine and to assess its predictive performance in a clinical setting by comparing model based predictions of trough levels with observed trough levels from TDM of renal transplant outpatients.
Materials and Methods
Development of the Physiologically Based Pharmacokinetic Model
The PBPK model was constructed using PK-Sim® (Open Systems Pharmacology Suite/Leverkusen, Germany). The PK-Sim® workflow, basic algorithm, and differential equations have been detailed previously (Willmann et al., 2003; Willmann et al., 2005). For the identification and quantification of model parameters a comprehensive literature search was conducted. For parameters with a broad range of values or no values found in literature a sensitivity analysis was performed. Finally applied values were estimated by comparing model based predictions with the pharmacokinetic data of single dose healthy volunteer studies published in literature (Table 1). A clinically and pharmacokinetically relevant range of doses was taken into account (for intravenous application: 1.5 mg/kg body weight (BW), 2, 2.1, 2.5, 3.0, and 5.0 mg/kg BW, for oral application: 100, 300, and 600 mg). For the simulations a virtual individual with the biometric values of an average European male human was generated (age: 30 years, BW: 73 kg, body height (BH): 176 cm). Model performance was assessed visually and quantitatively comparing the predicted and observed concentration-time curves in venous blood (Table 2).
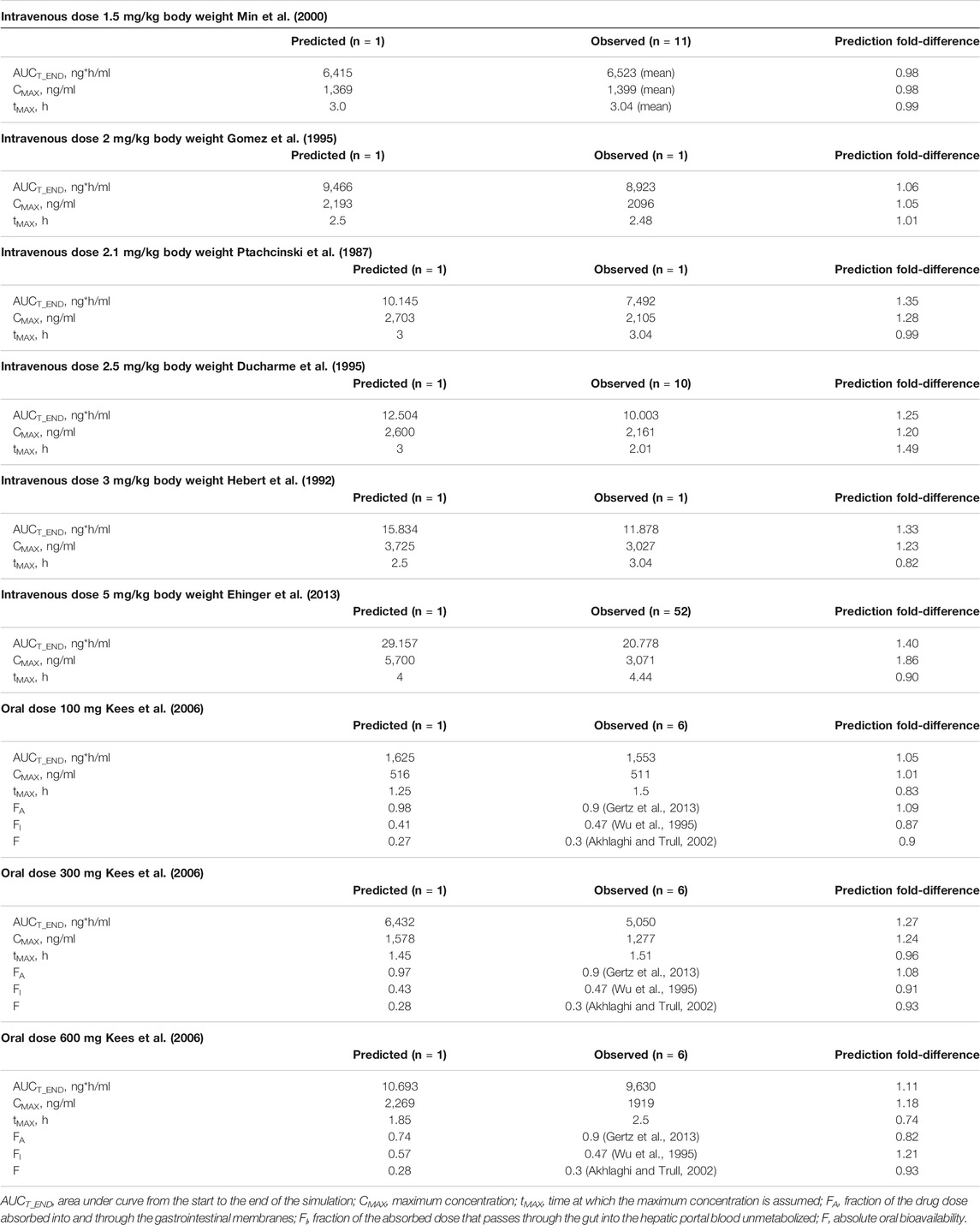
TABLE 2. Evaluation of the developed PBPK model with single dose healthy volunteer studies (Table 1).
Modeling and evaluation were initially performed for the intravenous application of cyclosporine. In a second step the oral absorption process was added. For modeling of the absorption process the fraction of the drug’s dose absorbed into and through the gastrointestinal membranes [FA = 0.9 for cyclosporine (Gertz et al., 2013)], the fraction of the absorbed dose that passes through the gut into the hepatic portal blood without metabolization [FI = 0.47 for cyclosporine (Wu et al., 1995)] and the absolute oral bioavailability [F = 0.3 for cyclosporine (Akhlaghi and Trull, 2002)] were considered while defining the model parameter’s values. That is, solubility, specific intestinal permeability, and intestinal intrinsic clearance were fitted within a range of literature based values in order to meet literature based values for FA, FI, and F.
Model Parameters
Table 3 shows all cyclosporine-specific model parameters and their respective values employed within the model as well as the abundance of binding proteins, transporters and metabolizing enzymes utilized to further characterize binding, transport and biotransformation processes in the virtual individuals.
For the lipophilicity and the solubility of cyclosporine depending on the solvent a broad range of values was found in literature. Applied values were within the range of literature based values and were adapted to finally map literature based concentration-time curves of healthy individuals and a literature based value for FA, respectively, as described above.
Nine specific binding processes to parenchymatous organs and blood cells were defined and quantified according to PBPK modeling for intravenous application of cyclosporine by Kawai and Tanaka et al. (Kawai et al., 1998; Tanaka et al., 1999) that were based on invasive studies with rats (Tanaka et al., 2000). From the same literature source the quantification of fU was set.
The clearance of cyclosporine from the body was represented via CYP3A4 enzymatic activity in the liver, the stomach, the kidney, the muscles and the intestine. The kinetics of systemic biotransformation were again quantified based on Tanaka et al. (Tanaka et al., 1999). While this model was developed for intravenous application and supposed biotransformation in the liver only, a closer agreement with observed data could be achieved with the inclusion of biotransformation in more CYP 3A4-enriched organs as mentioned above. The abundance and relative distribution of CYP 3A4 was quantified based on in vitro data as specified in Table 3.
To reproduce the bioavailability of cyclosporine after oral intake the intestinal biotransformation during absorption was quantified excluding systemic metabolism by fitting the intrinsic intestinal clearance to reach a literature based value for FI.
Ancillary, a P-GP-efflux-transport for the blood-brain-barrier and the intestinal wall was defined. The quantification of the efflux kinetics relied on Tanaka et al. (Tanaka et al., 1999) while the absolute and relative protein abundance for the intestine and brain was set in agreement with observed and in vitro data, respectively.
Since only a negligible amount of cyclosporine is cleared without metabolization and TDM in blood was supposed to be specific, no excretion processes were implemented within the model. Simulations with cyclosporine-intake as a solution (i.e. no drug liberation) showed the best agreement with observed data.
Drug Levels From Therapeutic Drug Monitoring
Available drug levels from TDM consisted of 356 cyclosporine trough levels of 32 renal transplant outpatients [male/female: 16/16, median age: 47 years (range: 22–66 years), median time after transplantation: 4 years (range: 1–20 years)] attended at the nephrological outpatient clinic of university hospital Marburg, Germany. Patients received a maintenance immunosuppressive medication regime consisting of cyclosporine (median drug dose: 87.5 mg every 12 h, range: 25–225 mg), additionally mycophenolate mofetil or azathioprine and, if necessary, corticosteroids. All patients showed a chronic elevation of serum creatinine levels and/or proteinuria. TDM was conducted over 6 years with an interval of one month or longer between each sample. Due to individual dose adjustments over time, each patient had received between 1 and 11 different doses of cyclosporine (median: 2) during the observation period. Cyclosporine levels were determined as whole blood measurements.
Comparison of Model Based Predictions With Drug Levels From Therapeutic Drug Monitoring
To compare clinically observed cyclosporine trough levels (C0_obs) with predictions made by the PBPK model (C0_pred) for each patient a corresponding virtual patient was created. Patient-specific parameters taken therefore into account were sex, age (as the median value of the observation period) and BW (as the median value of the observation period) (Table 4). For BH no values were documented in the retrospectively used clinical data. After assessing the resulting bias as negligible (data not shown) the parameter BH was quantified with the German average in correspondence to age and sex (Statistisches Bundesamt, 2013).
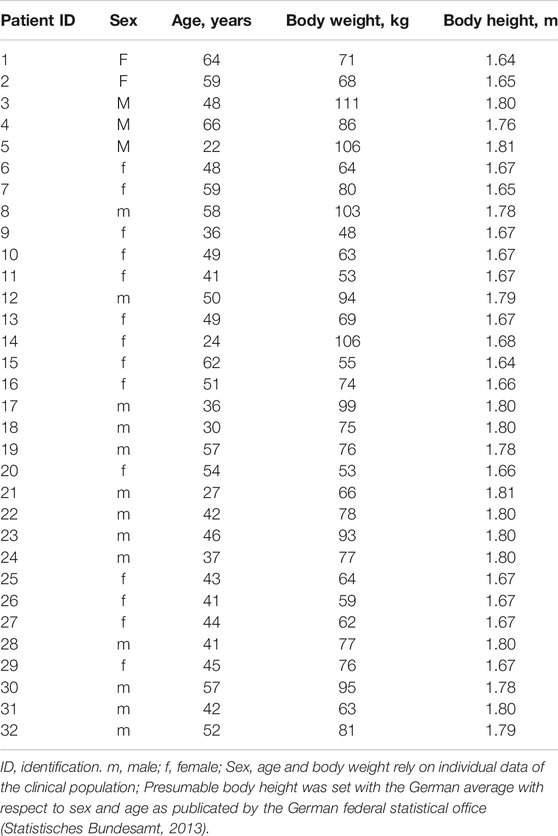
TABLE 4. Biometric parameters used to characterize each clinical patient virtually for modeling and simulation.
Trough levels were predicted for each patient and each dose with an application scheme of administrating the corresponding dose every 12 h over a period of 4 days to reach steady state.
Comparison was conducted by calculating the absolute deviation
and ratio
for matched pairs of trough levels. For the ratio a scatter-plot was mapped and the residuum R
was calculated, where a value of −0.30 < R < 0.30 represents a deviation of C0_pred and C0_obs of less than factor two and a value of −0.48 < R < 0.48 represents a deviation of C0_pred and C0_obs of less than factor three. The residuum of each matched pair of trough levels was then stratified for BW normalized dose and patient to reveal a potential relationship between prediction error and dose or prediction error and the specific patient, respectively.
To further characterize the predictive performance of the PBPK model the bias B
the precision p
the mean relative deviation MRD
and the mean percentage error MPE
were calculated (Sheiner and Beal, 1981; Edginton et al., 2006; Khalil and Läer, 2014).
Numerical calculations and graphical illustrations were conducted using Microsoft Excel® (Washington, United States of America).
Results
Evaluation of the Developed Physiologically Based Pharmacokinetic Model Using Single Dose Healthy Volunteer Studies
Results of the quantitative comparison of predicted and observed concentration-time curves with respect to FA, FI and F for healthy individuals represented by AUCT_END, CMAX, and tMAX are shown in Table 2. Particularly for low doses a high accordance could be achieved. For higher doses the predicted values showed a trend to exceed the observed values. For high oral dosing the model showed a solubility based restriction of absorption indicated by a fall of FA. This led to a raise of FI resulting in a constant value of absolute oral bioavailability (F).
Comparison of Model Based Predictions With Drug Levels From Therapeutic Drug Monitoring
C0_pred for renal transplant patients was 114 ng/ml in the median [interquartile range (IQR): 84–141 ng/ml, minimum (MIN) = 31 ng/ml, maximum (MAX) = 231 ng/ml] while C0_obs came to 111 ng/ml (IQR: 79–152 ng/ml, MIN = 5 ng/ml, MAX = 504 ng/ml). Comparing each corresponding pair of specific patient and dose the absolute deviation of C0_pred and C0_obs was 6 ng/ml in the median (IQR: −30–31 ng/ml, MIN = −379 ng/ml, MAX = 139 ng/ml). The ratios of C0_pred and C0_obs are shown as a scatterplot in Figure 1 in logarithmic and linear scale. 307 matched pairs (86%) are located on the light gray area between the dashed lines and differ thus less than twofold. 40 pairs (11%) are located on the dark gray area between dashed and dotted lines and thus differ at least twofold but less than threefold. 9 pairs (3%) exhibited a threefold or even higher deviation as indicated by location beyond the gray areas.
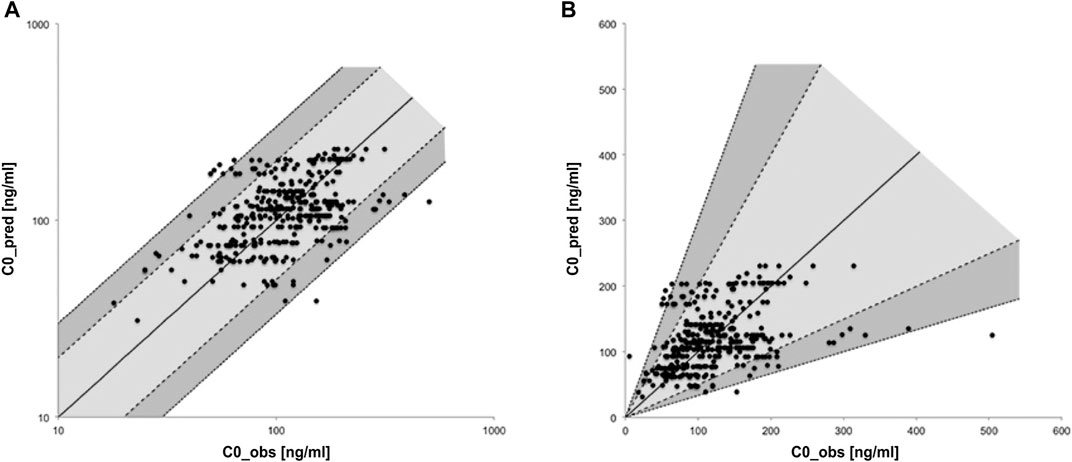
FIGURE 1. Scatter-plot of relative deviation between predicted and observed trough levels in logarithmic (A) and linear (B) scale.
Figure 2 shows the relative deviation of predicted and observed cyclosporine levels represented as the residual and stratified by BW-normalized dose and by patient, respectively. Stratifying the residua by BW-normalized dose revealed a trend toward increasingly positive residua for higher BW-normalized doses, that is the PBPK model predicted higher drug levels for higher doses than clinically observed.
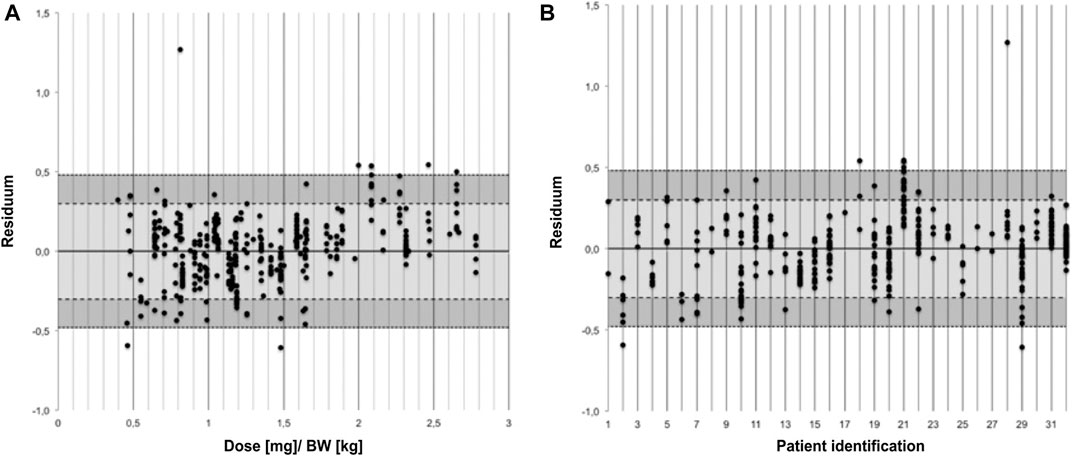
FIGURE 2. Residua of predicted and observed trough levels stratified by body weight normalized dose (A) and by patient (B).
For some patients (for example patient ID numbers 2 and 21) deviations between C0_pred and C0_obs were scattered around a high absolute residuum while predictions for other patients seemed to be scattered around a residuum of 0 (for example patient number 16). Yet others spread around a residuum of 0 and deviated only meanwhile in the direction of a higher residuum (for example patient number 29). The apparent outlier (R = 1.27 for patient number 28) resulted from a trough level of a 41 year old patient with a BW of 77 kg 19 years after transplantation. C0_obs was 5 ng/ml for a dose of 62.5 mg while C0_pred was 93 ng/ml.
The developed PBPK model showed a slightly negative systematic bias (−0.9 ng/ml) and a small standard deviation (precision 58.3 ng/ml). The mean relative deviation was 1.6 and the mean percentage error was 19.3%.
Discussion
The aim of this study was to test the predictive performance of a PBPK model in real-life clinical care by comparing predicted trough levels of a PBPK model developed for orally administered cyclosporine with observed trough levels measured by TDM in renal transplant outpatients. Up to now, PBPK models are hardly applied in clinical routine. Our work has shown, that taking into account patient's biometric parameter a PBPK model shows a satisfying accuracy with a small systematic bias and a reasonable precision for a heterogenous and chronically ill group of patients.
While in most patients predicted trough levels were in good accordance with observed drug levels, a relevant deviation (more than threefold) was found in some patients at least for a few cyclosporine trough levels. Prediction errors were more pronounced in patients receiving higher cyclosporine doses showing an overprediction in most of these cases. In the literature, factors influencing the absorption process are discussed as being substantial for the pharmacokinetic variability of cyclosporine. These factors include diet, intestinal motility and notably factors with an impact on the intestinal cytochrome P450 system.
Comedications inducing or inhibiting CYP3A4 have a strong impact on cyclosporine’s pharmacokinetics by lowering plasma levels up to one third or doubling of oral bioavailability, respectively (Hebert et al., 1992; Gomez et al., 1995). In our study, documented comedication data were limited to a few concomitantly taken immunosuppressive compounds (azathioprine, mycophenolate mofetil, and corticosteroids) which might be considered as a potential source for bias. Whereas conflicting results have been reported for corticosteroids, no clinically relevant influence on cyclosporine pharmacokinetics have been reported for azathioprine and mycofenolate mofetil (Kuypers, 2008; Lam et al., 2008). Hence, a limited impact of the immunosuppressive agents taken by the patients on the cyclosporine pharmacokinetics cannot be fully excluded. However, we did not found relevant discrepancies regarding the predictive accuracy of the developed cyclosporine PBPK model after stratification by corticosteroid intake (data not shown). Due to the limited documentation of comedications, we cannot fully exclude an intake of other comedications influencing the pharmacokinetics of cyclosporine to a relevant extent. Inclusion of those interacting (non-) immunosuppressive comedications may further improve the predictive accuracy of cyclosporine PBPK models.
It is known that expression of CYP3A4 can change in chronic kidney disease (Rowland Yeo et al., 2011; Zhao et al., 2012) which mostly develops in the clinical course after kidney transplantation and was present in our clinical population too. We stratified the model’s prediction by patient’s GFR (data not shown) and found no correlation between GFR and accuracy of model prediction. This is in accordance with the available evidence. Correspondingly no dose adjustments are recommended for patients with impaired kidney function (Novartis Pharma, 2011) implicating that changes of CYP3A4 expression in chronic kidney disease are of minor pharmacokinetic relevance for cyclosporine. Still, modeling of cyclosporine metabolism in chronic kidney disease seems to be an interesting spot for further research. The representation of intestinal cyclosporine metabolism within the model is based on literature based local CYP3A4 expression and on an intrinsic clearance fitted to match a literature based value for FI of 0.47. Other attempts to derive the intestinal extraction rate for cyclosporine from in vitro-data by PBPK modeling resulted in a 5-fold underestimation of the supposed value for FI (Gertz et al., 2011). Considerable variations in local expression influencing clearance in vivo are well known for CYP3A4 (Paine et al., 1997). Moreover, for cyclosporine its binding not only to plasmatic but also to cytosolic lipoproteins could be of particular relevance for intestinal and systemic metabolism. Lipoproteins are involved in many highly regulated physiological and pathophysiological processes which might also concern lipoprotein-bound cyclosporine (Gupta and Benet, 1990). Distribution of cyclosporine into lipoproteins shows an extensive inter- and intraindividual variation (Sgoutas et al., 1986) and lipoproteins vary greatly according to the current metabolic state in general, during cyclosporine therapy and with concurrent chronic kidney disease in particular. Depending on the method for measurement, pre-analytics and examined individuals values for fu vary therefore from 1 to 17% (Akhlaghi and Trull, 2002) while the fraction unbound was a very sensitive parameter in the current modeling with a distinct influence on simulated concentration-time curves. Concerning this aspect the developed PBPK model might not cover all physiological and pathophysiological conditions relevant for a mechanistic representation and mapping of cyclosporine pharmacokinetic variability but might offer prospects for further research.
Intestinal P-GP is discussed as another potential cause for the high variability of cyclosporine absorption (Fricker et al., 1996; Lown et al., 1997). The quantification of the intestinal P-GP efflux transport kinetics in the current model is based on the efflux transport of cyclosporine at the blood-brain-barrier of rats in vivo (Tanaka et al., 1999) which is attributed to P-GP (Goralski et al., 2006). Using numerical values for the P-GP efflux transport kinetics from in vitro studies (Saeki et al., 1993; Fricker et al., 1996) resulted in a fraction absorbed of ∼0.01, i.e. almost no absorption. Difficulties in translating P-GP in vitro data to in vivo observations are well known and might be at least partially caused by the complex interplay of intestinal solubility, intestinal permeability and intestinal metabolism. Modeling and simulation in the current work confirmed the assumption that a P-GP-efflux-transport in the small intestinum might be of minor importance for the pharmacokinetic profile of cyclosporine since a large amount of drug must be absorped to enable the extensive intestinal metabolism known for cyclosporine. P-GP might be of particular relevance to prevent absorption from the large intestine thereby forming an absorption window for cyclosporine in jejunum and ileum (Fricker et al., 1996). Moreover, a relevance of P-GP-efflux for the systemic distribution of cyclosporine was discussed before (Schinkel et al., 1995), but not considered in the current model.
The intravenous pharmacokinetics of cyclosporine within the model are marked by nine specific binding processes in blood cells and in parenchymatous organs leading to the high volume of distribution and a long half-life. While the accumulation of cyclosporine in blood cells due to specific and high-affinity-binding to cyclophilins is well studied, binding of cyclosporine in peripheral tissue and its quantification was hypothesized based on tissue concentrations in rates and estimations made by PBPK-modeling (Tanaka et al., 1999; Tanaka et al., 2000). An actual physiological correlate is unknown or at least presumably nonspecific (Ryffel, 1993). The validity of an interspecies translation between rats and humans was not studied.
Rather than specific binding processes, biotransformation and specific transports, physico-chemical parameters, that is solubility and intestinal permeability, appeared to be very sensitive parameters having a strong impact on predicted concentration-time curves and trough levels in the developed PBPK model and the conducted simulations by influencing absorption. Depending on the solvent, values for cyclosporine solubility range from 7.3 μg/ml in water (Ismailos et al., 1991) up to 250 μg/ml in postprandial human intestinal fluid (Persson et al., 2005). A solubility of 190 μg/ml as used in the current work seems to be a reasonable parameter value resulting in a solubility limit and a decline of the fraction absorbed at doses above 300 mg per os. This is in accordance with observed data and the reduction of dose has been discussed as a possible reason for the increase of oral bioavailability that can be observed in the course of cyclosporine immunosuppressive therapy early after transplantation (Ptachcinski et al., 1986). With respect to the fact that the absorption of cyclosporine seems to be food-dependent (Gupta and Benet, 1990), enhanced mechanisms of digestion prior to absorption might be involved in the absorption process. The prediction of drug levels while considering food intake and adapting solubility in the PBPK model might help to map very low and very high drug levels.
To the best of our knowledge, two other whole-body PBPK models depicting the absorption process of cyclosporine can be found in the literature. The first model (Darwich et al., 2013) relies on the Simcyp® compound file for cyclosporine, available in the Simcyp® Simulator compound library. Only few model parameter values are reported within the publication itself. FI is supposed to be much higher and FA to be considerably smaller than in the current work but no references for these assumptions are published. In the second model (Gertz et al., 2013) a drug liberation process is implied and the intestinal permeability is set higher than in our model. No P-GP kinetics are considered and the intestinal metabolism is represented semi-mechanistically only.
Considering solubility, intestinal metabolism and P-GP-efflux transport mechanistically our model can be used to examine the influence of clinical aspects on cyclosporine dosing (such as non-immunosuppressive co-medication, nutrition, dyslipidemia and chronic kidney disease). This might help to further understand inter- and intraindividual variability and to improve clinical outcome. While drug levels from TDM are a valuable starting point for testing the predictive performance of a PBPK model in a clinical context, further research should evaluate the PBPK model prospectively using full pharmacokinetic profiles.
In summary, the current study has shown that PBPK modeling offers valuable contributions for pharmacokinetic research in clinical populations.
Data Availability Statement
The raw data supporting the conclusion of this article will be made available by the authors, without undue reservation.
Ethics Statement
Prior to this study, an approval was given by the institutional review board of Philipps-University Marburg/Germany to use patients’ clinical data in an anonymized manner for scientific purposes.
Author Contributions
All authors contributed significantly to the design of this study. SZ analyzed data, developed the PBPK model for cyclosporine under supervision of SS, PT, SW, KM and drafted a first version of the manuscript. S-OG obtained the clinical data and was responsible for clinical interpretation of findings. SS and PT were responsible for the project plan and supervised all steps of the project. All authors contributed significantly to the final version of the manuscript.
Funding
This study was financially supported by an internal research funding of the Witten/Herdecke University.
Conflict of Interest
SW was employed by the company Bayer AG, Research and Development, Clinical Pharmacometrics, Wuppertal, Germany. KM was employed by the company Bayer AG, Research and Development, Systems Pharmacology and Medicine I, Leverkusen, Germany. For this study, the software PK-Sim® (Open Systems Pharmacology Suite/Leverkusen, Germany) was used.
The remaining authors declare that the research was conducted in the absence of any commercial or financial relationships that could be construed as a potential conflict of interest.
Acknowledgments
We thank Witten/Herdecke University for supporting this study by an internal research funding.
Abbreviation
PBPK model, physiologically based pharmacokinetic model; CYP, cytochrome P450; P-GP, P-glycoprotein; fU, fraction nbound; TDM, therapeutic drug monitoring; BW, body weight; BH, body height; FA, fraction of the drug’s dose absorbed into and through the gastrointestinal membranes; FI, fraction of the absorbed dose that passes through the gut into the hepatic portal blood unmetabolized; F, absolute oral bioavailability; C0_obs, clinically observed cyclosporine trough levels; C0_pred, cyclosporine trough levels predicted by the PBPK model; R, residuum; B, bias; P, precision; MRD, mean relative deviation; MPE, mean percentage error; IQR, interquartile range; MIN, minimum; MAX, maximum.
References
Akhlaghi, F., and Trull, A. K. (2002). Distribution of Cyclosporin in Organ Transplant Recipients. Clin. Pharmacokinet. 41 (9), 615–637. doi:10.2165/00003088-200241090-00001
Amidon, G. L., Lennernäs, H., Shah, V. P., and Crison, J. R. (1995). A Theoretical Basis for a Biopharmaceutic Drug Classification: the Correlation of In Vitro Drug Product Dissolution and In Vivo Bioavailability. Pharm. Res. 12 (3), 413–420. doi:10.1023/a:1016212804288
Bruyère, A., Declèves, X., Bouzom, F., Ball, K., Marques, C., Treton, X., et al. (2010). Effect of Variations in the Amounts of P-Glycoprotein (ABCB1), BCRP (ABCG2) and CYP3A4 along the Human Small Intestine on PBPK Models for Predicting Intestinal First Pass. Mol. Pharm. 7 (5), 1596–1607. doi:10.1021/mp100015x
Darwich, A. S., Pade, D., Rowland-Yeo, K., Jamei, M., Asberg, A., Christensen, H., et al. (2013). Evaluation of an In Silico PBPK Post-Bariatric Surgery Model through Simulating Oral Drug Bioavailability of Atorvastatin and Cyclosporine. CPT Pharmacometrics Syst. Pharmacol. 2 (6), e47. doi:10.1038/psp.2013.23
Ducharme, M. P., Warbasse, L. H., and Edwards, D. J. (1995). Disposition of Intravenous and Oral Cyclosporine after Administration with Grapefruit Juice. Clin. Pharmacol. Ther. 57 (5), 485–491. doi:10.1016/0009-9236(95)90032-2
Edginton, A. N., Schmitt, W., and Willmann, S. (2006). Development and Evaluation of a Generic Physiologically Based Pharmacokinetic Model for Children. Clin. Pharmacokinet. 45 (10), 1013–1034. doi:10.2165/00003088-200645100-00005
Ehinger, K. H. J., Hansson, M. J., Sjövall, F., and Elmér, E. (2013). Bioequivalence and Tolerability Assessment of a Novel Intravenous Ciclosporin Lipid Emulsion Compared to Branded Ciclosporin in Cremophor EL. Clin. Drug Invest. 33 (1), 25–34. doi:10.1007/s40261-012-0029-x
Foxwell, B. M. J., Frazer, G., Winters, M., Hiestand, P., Wenger, R., and Ryffel, B. (1988). Identification of Cyclophilin as the Erythrocyte Ciclosporin-Binding Protein. Biochim. Biophys. Acta—Biomembranes 938 (3), 447–455. doi:10.1016/0005-2736(88)90142-3
Fricker, G., Drewe, J., Huwyler, J., Gutmann, H., and Beglinger, C. (1996). Relevance of P-Glycoprotein for the Enteral Absorption of Cyclosporin A: In Vitro-In Vivo Correlation. Br. J. Pharmacol. 118 (7), 1841–1847. doi:10.1111/j.1476-5381.1996.tb15612.x
Gérard, C., Bleyzac, N., Girard, P., Freyer, G., Bertrand, Y., and Tod, M. (2010). Influence of Dosing Schedule on Organ Exposure to Cyclosporin in Pediatric Hematopoietic Stem Cell Transplantation: Analysis with a PBPK Model. Pharm. Res. 27 (12), 2602–2613. doi:10.1007/s11095-010-0252-1
Gertz, M., Cartwright, C. M., Hobbs, M. J., Kenworthy, K. E., Rowland, M., Houston, J. B., et al. (2013). Cyclosporine Inhibition of Hepatic and Intestinal CYP3A4, Uptake and Efflux Transporters: Application of PBPK Modeling in the Assessment of Drug-Drug Interaction Potential. Pharm. Res. 30 (3), 761–780. doi:10.1007/s11095-012-0918-y
Gertz, M., Houston, J. B., and Galetin, A. (2011). Physiologically Based Pharmacokinetic Modeling of Intestinal First-Pass Metabolism of CYP3A Substrates with High Intestinal Extraction. Drug Metab. Dispos. 39 (9), 1633–1642. doi:10.1124/dmd.111.039248
Gomez, D. Y., Wacher, V. J., Tomlanovich, S. J., Hebert, M. F., and Benet, L. Z. (1995). The Effects of Ketoconazole on the Intestinal Metabolism and Bioavailability of Cyclosporine. Clin. Pharmacol. Ther. 58 (1), 15–19. doi:10.1016/0009-9236(95)90067-5
Goralski, K. B., Acott, P. D., Fraser, A. D., Worth, D., and Sinal, C. J. (2006). Brain Cyclosporin A Levels Are Determined by Ontogenic Regulation of Mdr1a Expression. Drug Metab. Dispos. 34 (2), 288–295. doi:10.1124/dmd.105.007427
Gupta, S. K., and Benet, L. Z. (1990). High-fat Meals Increase the Clearance of Cyclosporine. Pharm. Res. 07 (1), 46–48. doi:10.1023/a:1015831408425
Hebert, M. F., Roberts, J. P., Prueksaritanont, T., and Benet, L. Z. (1992). Bioavailability of Cyclosporine with Concomitant Rifampin Administration Is Markedly Less Than Predicted by Hepatic Enzyme Induction. Clin. Pharmacol. Ther. 52 (5), 453–457. doi:10.1038/clpt.1992.171
Ismailos, G., Reppas, C., Dressman, J. B., and Macheras, P. (1991). Unusual Solubility Behaviour of Cyclosporin A in Aqueous Media. J. Pharm. Pharmacol. 43 (4), 287–289. doi:10.1111/j.2042-7158.1991.tb06688.x
Kahan, B. D., Welsh, M., Urbauer, D. L., Mosheim, M. B., Beusterien, K. M., Wood, M. R., et al. (2000). Low Intraindividual Variability of Cyclosporin A Exposure Reduces Chronic Rejection Incidence and Health Care Costs. J. Am. Soc. Nephrol. 11 (6), 1122–1131. doi:10.1681/ASN.V1161122
Kahan, B. D., Van Buren, C. T., Boileau, M., Ried, M., Payne, W. D., Flechner, S., et al. (1983). Cyclosporin A Tissue Levels in a Cadaveric Renal Allograft Recipient. Transplantation 35 (1), 96–99. doi:10.1097/00007890-198301000-00018
Kawai, R., Mathew, D., Tanaka, C., and Rowland, M. (1998). Physiologically Based Pharmacokinetics of Cyclosporine A: Extension to Tissue Distribution Kinetics in Rats and Scale-Up to Human. J. Pharmacol. Exp. Ther. 287 (2), 457–468.
Kees, F., Bucher, M., Schweda, F., Gschaidmeier, H., Burhenne, J., Mikus, G., et al. (2006). Comparative Bioavailability of the Microemulsion Formulation of Cyclosporine (Neoral) with a Generic Dispersion Formulation (Cicloral) in Young Healthy Male Volunteers. Ther. Drug Monit. 28 (3), 312–320. doi:10.1097/01.ftd.0000211804.89440.74
Khalil, F., and Läer, S. (2014). Physiologically Based Pharmacokinetic Models in the Prediction of Oral Drug Exposure over the Entire Pediatric Age Range-Sotalol as a Model Drug. AAPS J. 16 (2), 226–239. doi:10.1208/s12248-013-9555-6
Kidney Disease Improving Global Outcomes (2009). KDIGO Clinical Practice Guideline for the Care of Kidney Transplant Recipients. Am. J. Transplant. 9, S1–S155. doi:10.1111/j.1600-6143.2009.02834.x
Kronbach, T., Fischer, V., and Meyer, U. A. (1988). Cyclosporine Metabolism in Human Liver: Identification of a Cytochrome P-450III Gene Family as the Major Cyclosporine-Metabolizing Enzyme Explains Interactions of Cyclosporine with Other Drugs. Clin. Pharmacol. Ther. 43 (6), 630–635. doi:10.1038/clpt.1988.87
Kuepfer, L., Niederalt, C., Wendl, T., Schlender, J. F., Willmann, S., Lippert, J., et al. (2016). Applied Concepts in PBPK Modeling: How to Build a PBPK/PD Model. CPT Pharmacometrics Syst. Pharmacol. 5 (10), 516–531. doi:10.1002/psp4.12134
Kuypers, D. R. (2008). Influence of Interactions between Immunosuppressive Drugs on Therapeutic Drug Monitoring. Ann. Transpl. 13 (3), 11–18.
Lam, S., Partovi, N., Ting, L. S., and Ensom, M. H. (2008). Corticosteroid Interactions with Cyclosporine, Tacrolimus, Mycophenolate, and Sirolimus: Fact or Fiction? Ann. Pharmacother. 42 (7), 1037–1047. doi:10.1345/aph.1k628
Lemaire, M., and Tillement, J. P. (1982). Role of Lipoproteins and Erythrocytes in the In Vitro Binding and Distribution of Cyclosporin A in the Blood. J. Pharm. Pharmacol. 34 (11), 715–718. doi:10.1111/j.2042-7158.1982.tb06206.x
Lown, K. S., Mayo, R. R., Leichtman, A. B., Hsiao, H.-l., Turgeon, D. K., Schmiedlin-Ren, P., et al. (1997). Role of Intestinal P-Glycoprotein (Mdr1) in Interpatient Variation in the Oral Bioavailability of Cyclosporine. Clin. Pharmacol. Ther. 62 (3), 248–260. doi:10.1016/s0009-9236(97)90027-8
Min, D., Lee, M., Ku, Y. M., and Flanigan, M. (2000). Gender-dependent Racial Difference in Disposition of Cyclosporine Among Healthy African American and White Volunteers. Clin. Pharmacol. Ther. 68 (5), 478–486. doi:10.1067/mcp.2000.111255
Nankivell, B. J., Borrows, R. J., Fung, C. L.-S., O'Connell, P. J., Allen, R. D. M., and Chapman, J. R. (2003). The Natural History of Chronic Allograft Nephropathy. N. Engl. J. Med. 349 (24), 2326–2333. doi:10.1056/nejmoa020009
Novartis Pharma (2011). Sandimmun® Optoral. Available at: https://klinischeforschung.novartis.de/dokumente/fachinformationen-sandimmun-optoral-100-mg-ml-loesung-zum-einnehmen/.
Paine, M. F., Khalighi, M., Fisher, J. M., Shen, D. D., Kunze, K. L., Marsh, C. L., et al. (1997). Characterization of Interintestinal and Intraintestinal Variations in Human CYP3A-dependent Metabolism. J. Pharmacol. Exp. Ther. 283 (3), 1552–1562.
Persson, E. M., Gustafsson, A.-S., Carlsson, A. S., Nilsson, R. G., Knutson, L., Forsell, P., et al. (2005). The Effects of Food on the Dissolution of Poorly Soluble Drugs in Human and in Model Small Intestinal Fluids. Pharm. Res. 22 (12), 2141–2151. doi:10.1007/s11095-005-8192-x
Polasek, T. M., Tucker, G. T., Sorich, M. J., Wiese, M. D., Mohan, T., Rostami-Hodjegan, A., et al. (2018). Prediction of Olanzapine Exposure in Individual Patients Using Physiologically Based Pharmacokinetic Modelling and Simulation. Br. J. Clin. Pharmacol. 84 (3), 462–476. doi:10.1111/bcp.13480
Ptachcinski, R. J., Venkataramanan, R., and Burckart, G. J. (1986). Clinical Pharmacokinetics of Cyclosporin. Clin. Pharmacokinet. 11 (2), 107–132. doi:10.2165/00003088-198611020-00002
Ptachcinski, R. J., Venkataramanan, R., Burckart, G. J., Gray, J. A., Van Thiel, D. H., Sanghvi, A., et al. (1987). Cyclosporine Kinetics in Healthy Volunteers. J. Clin. Pharmacol. 27 (3), 243–248. doi:10.1002/j.1552-4604.1987.tb02193.x
Radke, C., Horn, D., Lanckohr, C., Ellger, B., Meyer, M., Eissing, T., et al. (2016). Development of a Physiologically Based Pharmacokinetic Modelling Approach to Predict the Pharmacokinetics of Vancomycin in Critically Ill Septic Patients. Clin. Pharmacokinet. 56(7). 759–779. doi:10.1007/s40262-016-0475-3
Rodrigues, A. D. (1999). Integrated Cytochrome P450 Reaction Phenotyping: Attempting to Bridge the Gap between cDNA-Expressed Cytochromes P450 and Native Human Liver Microsomes. Biochem. Pharmacol. 57 (5), 465–480. doi:10.1016/s0006-2952(98)00268-8
Rowland Yeo, K., Aarabi, M., Jamei, M., and Rostami-Hodjegan, A. (2011). Modeling and Predicting Drug Pharmacokinetics in Patients with Renal Impairment. Expert Rev. Clin. Pharmacol. 4 (2), 261–274. doi:10.1586/ecp.10.143
Rüegger, A., Kuhn, M., Lichti, H., Loosli, H.-R., Huguenin, R., Quiquerez, C., et al. (1976). Cyclosporin A, ein immunsuppressiv wirksamer Peptidmetabolit ausTrichoderma polysporum(LINKex PERS.)Rifai. Hca 59 (4), 1075–1092. doi:10.1002/hlca.19760590412
Ryffel, B. (1993). Cyclosporin Binding Proteins. Biochem. Pharmacol. 46 (1), 1–12. doi:10.1016/0006-2952(93)90341-s
Saeki, T., Ueda, K., Tanigawara, Y., Hori, R., and Komano, T. (1993). Human P-Glycoprotein Transports Cyclosporin A and FK506. J. Biol. Chem. 268 (9), 6077–6080. doi:10.1016/s0021-9258(18)53221-x
Schinkel, A. H., Wagenaar, E., van Deemter, L., Mol, C. A., and Borst, P. (1995). Absence of the Mdr1a P-Glycoprotein in Mice Affects Tissue Distribution and Pharmacokinetics of Dexamethasone, Digoxin, and Cyclosporin A. J. Clin. Invest. 96 (4), 1698–1705. doi:10.1172/jci118214
Sgoutas, D., MacMahon, W., Love, A., and Jerkunica, I. (1986). Interaction of Cyclosporin A with Human Lipoproteins. J. Pharm. Pharmacol. 38 (8), 583–588. doi:10.1111/j.2042-7158.1986.tb03085.x
Sheiner, L. B., and Beal, S. L. (1981). Some Suggestions for Measuring Predictive Performance. J. Pharmacokinet. Biopharmaceutics 9 (4), 503–512. doi:10.1007/bf01060893
Statistisches Bundesamt (Hrsg.) (2013). Mikrozensus - Fragen zur Gesundheit - Körpermaße der Bevölkerung. Wiesbaden: Statistisches Bundesamt.
Tanaka, C., Kawai, R., and Rowland, M. (2000). Dose-Dependent Pharmacokinetics of Cyclosporin A in Rats: Events in Tissues. Drug Metab. Dispos 28 (5), 582–589.
Tanaka, C., Kawai, R., and Rowland, M. (1999). Physiologically Based Pharmacokinetics of Cyclosporine A: Reevaluation of Dose-Nonlinear Kinetics in Rats. J. Pharmacokinet. Biopharm. 27 (6), 597–623. doi:10.1023/a:1020978509566
Venkataramanan, R., Starzl, T. E., Yang, S., Burckart, G. J., Ptachcinski, R. J., Shaw, B. W., et al. (1985). Biliary Excretion of Cyclosporine in Liver Transplant Patients. Transpl. Proc 17 (1), 286–289.
Wagner, C., Thelen, K., Willmann, S., Selen, A., and Dressman, J. B. (2013). Utilizing In Vitro and PBPK Tools to Link ADME Characteristics to Plasma Profiles: Case Example Nifedipine Immediate Release Formulation. J. Pharm. Sci. 102 (9), 3205–3219. doi:10.1002/jps.23611
Willmann, S., Lippert, J., and Schmitt, W. (2005). From Physicochemistry to Absorption and Distribution: Predictive Mechanistic Modelling and Computational Tools. Expert Opin. Drug Metab. Toxicol. 1 (1), 159–168. doi:10.1517/17425255.1.1.159
Willmann, S., Lippert, J., Sevestre, M., Solodenko, J., Fois, F., and Schmitt, W. (2003). PK-sim: a Physiologically Based Pharmacokinetic “whole-Body” Model. Biosilico 1 (4), 121–124. doi:10.1016/s1478-5382(03)02342-4
Wu, C.-Y., Benet, L. Z., Hebert, M. F., Gupta, S. K., Rowland, M., Gomez, D. Y., et al. (1995). Differentiation of Absorption and First-Pass Gut and Hepatic Metabolism in Humans: Studies with Cyclosporine. Clin. Pharmacol. Ther. 58 (5), 492–497. doi:10.1016/0009-9236(95)90168-x
Zhao, P., Rowland, M., and Huang, S.-M. (2012a). Best Practice in the Use of Physiologically Based Pharmacokinetic Modeling and Simulation to Address Clinical Pharmacology Regulatory Questions. Clin. Pharmacol. Ther. 92 (1), 17–20. doi:10.1038/clpt.2012.68
Zhao, P., Vieira, M., Grillo, J. A., Song, P., Wu, T.-C., Zheng, J. H., et al. (2012b). Evaluation of Exposure Change of Nonrenally Eliminated Drugs in Patients with Chronic Kidney Disease Using Physiologically Based Pharmacokinetic Modeling and Simulation. J. Clin. Pharmacol. 52 (1 Suppl. l), 91S–108S. doi:10.1177/0091270011415528
Keywords: cyclosporine, pharmacokinetics, therapeutic drug monitoring, PBPK, modeling and simulation
Citation: Zapke SE, Willmann S, Grebe S-O, Menke K, Thürmann PA and Schmiedl S (2021) Comparing Predictions of a PBPK Model for Cyclosporine With Drug Levels From Therapeutic Drug Monitoring. Front. Pharmacol. 12:630904. doi: 10.3389/fphar.2021.630904
Received: 19 November 2020; Accepted: 27 April 2021;
Published: 14 May 2021.
Edited by:
Xiaodong Liu, China Pharmaceutical University, ChinaReviewed by:
Sagnik Chatterjee, Syngene International, IndiaConstantin Mircioiu, Carol Davila University of Medicine and Pharmacy, Romania
Copyright © 2021 Zapke, Willmann, Grebe, Menke, Thürmann and Schmiedl. This is an open-access article distributed under the terms of the Creative Commons Attribution License (CC BY). The use, distribution or reproduction in other forums is permitted, provided the original author(s) and the copyright owner(s) are credited and that the original publication in this journal is cited, in accordance with accepted academic practice. No use, distribution or reproduction is permitted which does not comply with these terms.
*Correspondence: Sven Schmiedl, sven.schmiedl@helios-gesundheit.de