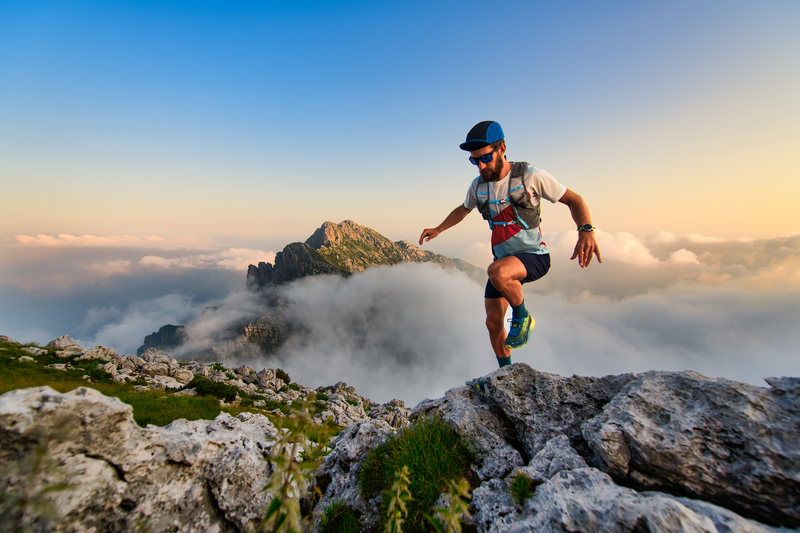
94% of researchers rate our articles as excellent or good
Learn more about the work of our research integrity team to safeguard the quality of each article we publish.
Find out more
REVIEW article
Front. Pharmacol. , 11 March 2021
Sec. Ethnopharmacology
Volume 12 - 2021 | https://doi.org/10.3389/fphar.2021.474267
Amid the establishment and optimization of Connectivity Map (CMAP), the functional relationships among drugs, genes, and diseases are further explored. This biological database has been widely used to identify drugs with common mechanisms, repurpose existing drugs, discover the molecular mechanisms of unknown drugs, and find potential drugs for some diseases. Research on traditional Chinese medicine (TCM) has entered a new era in the wake of the development of bioinformatics and other subjects including network pharmacology, proteomics, metabolomics, herbgenomics, and so on. TCM gradually conforms to modern science, but there is still a torrent of limitations. In recent years, CMAP has shown its distinct advantages in the study of the components of TCM and the synergetic mechanism of TCM formulas; hence, the combination of them is inevitable.
CMAP is a database in which mRNA expression levels of cells treated with different small molecule drugs are stored according to the degree of upregulation or downregulation compared with the control group. After the query signature (mRNA expression level was significantly changed) was found from the drug or disease, the connectivity scores of each drug molecule in the database ranging from −1 to 1 (from completely negative correlation to completely positive correlation) were available by querying the Connectivity Map. Finally, the known molecules were selected to do further research according to the scores (Gao et al., 2019). The five elements of the CMAP database are genomic signatures, cell lines, perturbagens, concentration and duration of treatment, and pattern-matching strategy. According to the principle that a few cells, low cost, high yield, and enough complexity to provide rich description, the first-generation Connectivity Map chose mRNA expression assayed on DNA microarrays as genomic signatures (Lamb et al., 2006). The perturbation in mammalian cell culture is more easy and economic to generate data. 164 disparate small molecules approved by FDA represent different bioactive tools to perturb cells. The dose sensitive to the result was explored through a subset of compounds across a range of concentrations. The duration of treatment is obliged to veritably reflect signals related to the direct mechanism. Instead of the traditional hierarchical clustering (Subramanian et al., 2005), the database employs new pattern-matching strategy based on the Gene Set Enrichment Analysis (GSEA) (Boratyn et al., 2006). In this article, we look back at the process of CMAP optimization in the last 20 years since the emergence of CMAP. In addition, we aim to summarize the articles published in the past ten years on the application of CMAP in traditional Chinese medicine (TCM) research and emphasize the advantages of CMAP in it.
CMAP began with Daniel D. Shoemaker, who searched for the function of open reading frames (ORFs) in yeast by molecular bar-coding strategy using mutant yeast strains tagged with unique sequences and hybridizing with oligonucleotide array to amplify the signal in 1996 (Shoemaker et al., 1996). But few cellular functions possess the corresponding simple phenotypes to be applied. In 2000, Timothy R. Hughes established the genome database of 300 S. cerevisiae (with characteristic gene mutation, uncharacteristic ORF gene mutations, and treatment of known molecular target drugs) for gene function classification and discovery (Hughes et al., 2000). This idea was proposed as a database of reference expression profiles by a simple comparison of the most similar patterns of its gene expression profiles. In the same way, they applied it to discover the function of drugs and states of diseases. The pioneer work could be traced to Justin Lamb who first demonstrated that the Connectivity Map concept was indeed viable through majorization of the potential problem and recovery of known and new connections in 2006 (Lamb et al., 2006).
In recent decades, CMAP has become comprehensive with more drug molecules in the database and further optimized data processing methods. The publicly funded CMAP increased FDA-approved small molecules by 164–1,309. It also provides an interactive website and an online tool to conduct CMAP queries against the chemical reference catalogs (http://www.broadinstitute.org/cmap) (Qu and Rajpal, 2012). SSCMap (statistically significant connections' map) is a Java application designed to make statistically significant connections between a user-supplied gene signature and the 6,100 core reference profiles based on the Broad Institute expanded dataset (Zhang and Gant, 2009). Quite a few new types of CMAP have emerged, such as Functional Module Connectivity Map (FMCM), long noncoding RNA (lncRNA) Connectivity Map, and Drug directionality Map (DMAP). Functional Module Connectivity Map was devised for the discovery of repurposed drug compounds for systems treatment of complex diseases especially cancer (Xue et al., 2014). The core of FMCM is to construct condition-specific function–function networks (FFNs) to identify highly expressed hub genes before querying CMAP. In this way, higher hit rates around 65% on effective drugs against early tumorigenesis in colorectal cancer were presented. Shortly before, mRNA expression was transformed to lncRNAs as genomic signatures to connect small molecules, genes, and diseases, which is called lncRNA Connectivity Map (Yang et al., 2017). Different from traditional CMAP, lncRNAs as potential biomarkers play a key role in various biological processes and mechanisms. Drug directionality Map is an exhaustive drug-protein Connectivity Map constructed in silico, which makes up for the limitation of Connectivity Maps on data coverage. The database not only stores drugs for certain diseases, but contains directed drug-to-protein effects and effect scores to make drug repositioning efforts successful (Huang et al., 2015).
To put that in perspective, CMAP has been widely used in pharmacogenomics, including identification of novel phenotypic relations for disease treatment, for drug repurposing and for studying drug combinations (Musa et al., 2018).
On the one hand, exploring the connections between drugs is facile. For example, the agonist and antagonist of same substance can be directly shown through the connectivity score, so that we can identify the drugs with common mechanisms of action, even though structurally diverse hERG (Human Ether-a-go-go-Related Gene) inhibitors are prone to be enriched through transcriptional response similarity (Brody et al., 2013). A class of drugs with common activity can be recovered by CMAP, which can predict the target path and even discover the unknown mechanism for small molecule drugs with unknown characteristics. Andrea M. Brum et al. analyzed gene expression profiles in hMSCs (human bone marrow mesenchymal stem cells) differentiated into osteoblasts and obtained query signature. They found parbendazole matched with the expression changes in osteogenic hMSCs as a candidate of bone stimulating compound. It further revealed the human osteogenic pathway by which parbendazole can induce osteogenic differentiation by combining cytoskeleton changes and the increase of bmp-2 activity (Brum et al., 2015). Withaferin A, calcium folinate, and amylocaine were underlying osteogenic drugs through stimulating both ALP activity and mineralization in hMSCs in light of positive correlation (Brum et al., 2018).
On the other hand, the link between drugs and diseases is equally important. For instance, the particular query signature directly from a disease or indirectly from a known drug efficacious to the disease can be used to filter other potential drugs for the disease through CMAP. Owing to multiple databases with massive gene expression profiles on CRC (colorectal cancer), CMAP analyzed a combined gene with a distinction of 148 genes to filtrate 10 candidate compounds, including irinotecan and etoposide (Wen et al., 2015). Similarly, it has been found that histone deacetylase inhibitors can inhibit the activity of hepatocytes and select the prognostic phenotype of HB cells (hepatoblastoma cells) by changing gene expression, which plays a synergistic role in combination with cisplatin (Beck et al., 2016). Drug candidates to restrain cigarette smoke-induced inflammation were kaempferol and bethanechol via CMAP (Vanderstocken et al., 2018). The difference is that the query signature here is from the contrast between the healthy and the smokers. Beyond that, the CMAP has shown promise in the treatment of cystic fibrosis (CF) (Malcomson et al., 2016), lymphoma (Luo et al., 2018), Huntington's disease (Smalley et al., 2016), breast cancer (Fang and Zhang, 2017), Hirschsprung disease (Xiao et al., 2018), and gastric cancer (Zhang et al., 2018) on account of identifying compounds and combination therapies.
Furthermore, CMAP serves to identify the novel therapies for existing drugs. Leptin has been dismissed as the main treatment for obesity with resistance. Amantadine, an FDA-approved antiviral and anti-Parkinson agent, is associated with a monocyte-macrophage-like differentiation of HL60, U937, and Kasumi-1 myeloid leukemia cell lines by inducing vitamin D receptor (VDR) expression (Manzotti et al., 2015). On account of Celastrol, a small molecule extracted from the roots of Thunder of God Vine plant and found to act as a sensitization of leptin through CMAP, leptin combined with it can be used to treat obesity with leptin deficiency (Liu et al., 2015). Nowadays there are many other staggering applications. It is critical to recover apoptosis subnetwork of the generalizable cell death pathways triggered by drug. CMAP can help restore the network (Yu et al., 2015). CMAP succeeds to carve out early prediction models of adverse drug reactions (ADRs) rendering the potential safety risks visible (Wang et al., 2015). What's more surprising is that RNAi and CRISPR technologies are evaluated by CMAP (Smith et al., 2017).
So far, the CMAP technology has been applied in a wide range of TCM studies. The application can be categorized in regard to their purpose of study. Specifically, CMAP in TCM has been used to discover the molecular mechanism of TCM, to interpret synergistic mechanism of TCM formula, to elucidate the veracity of some TCM for certain disease treatment, and even for TCM repurposing (Zhang et al., 2016).
Although more and more meaningful results proved the necessity of CMAP for TCM research, only a few small molecule drugs approved by FDA in CMAP are components of TCM. There is a lack of systematic TCM-CMAP. Over the past few years, Weidong Zhang's laboratory has performed microarray analysis and fluorescence quantitative detection on MCF7 cells treated by 102 representative small molecules of TCM. In addition, each group was treated with DMSO as control, and the two groups were divided during the algorithm treatment to correct the results caused by the discrepancy in gene chip. Finally, the differential expression probe was selected as the query signature and input CMAP. The query result was obtained according to the same principle as the usual CMAP (Lv et al., 2017). On the basis of them, Minjae Yoo et al. query the 102 TCM components with gene expression signatures using the latest connectivity graph CLUE web application (https://clue.io/). The query results comprise 84 CMAP classes, and the MoAs (the mechanism of actions) for these TCM components are divided into seven categories based upon the classification of the inhibitors or activators as well as clustering analysis. TCM component clusters are four correspondingly. These results are stored to establish TCM Hub (Yoo et al., 2018). TCM Hub that is a connection diagram resource is freely available in http://tanlab.ucdenver.edu/TCMHub. It functions as a TCM-CMAP to query the MoAs of TCM, active ingredients, and Chinese medicine molecules associated with certain diseases (Figure 1).
FIGURE 1. Simplified overview of the principle and route of Connectivity Map applied in TCM. In the first step, cells treated with 102 representative Chinese medicines molecularly were calibrated with the control group, and gene expression profiles were obtained by microarray technology. Then, different expressed genes were available to query the latest CMAP so connection scores were acquired with certain algorithm. Based on the results above, comparability between TCM and corresponding small molecules was the starting point. With that, the relationship between TCM, genes, and diseases has chance to be further analyzed. Finally, a TCM Hub database is established by integrating the results.
Berberine (BBR) is an isoquinoline alkaloid known for its anti-inflammatory, lipid-regulating, anticancer, antidiabetic, antibacterial, antiparasitic, and fungicidal activities (Ayati et al., 2017). The multiple pharmacological effects of BBR are derived from its diverse molecular targets, so it takes a lot of work to study its MoAs (mechanism of action) by traditional methods, while CMAP can efficiently locate its various MoAs as a whole. Lee K. H et al. obtained molecules with high connectivity score by querying CMAP, top of which were protein synthesis inhibitors, mTOR, or HDAC inhibitor. With further querying the STTICH database and experimental verification, they found that potential MoAs of BBR were inhibition of global protein synthesis and the basic activity of AKT, induction of endoplasmic reticulum stress, and autophagy and acetylation of HDAC6 substrate a-tubulin (Kuhn et al., 2014; Lee et al., 2014). This provides a direction for further research on the specific pharmacological mechanism of BBR. Likewise, Yuan Quan et al. also predict the pharmacological mechanisms of curcumin (a typical isolated natural drug), including anti-inflammatory, anti-infective, and neuroregulation effect. Most of them were supported by experimental observations (Quan et al., 2014). Cinobufotalin is a compound extracted from the skin of dried toads and has a broad spectrum of antitumor activity, but the molecular mechanism is unknown. Jie Li et al. searched for matches in CMAP and found that cinobufotalin and miconazole had similar molecular mechanisms, with neuroactive ligand-receptor interactions and calcium signaling pathways being the key to their effects (Li et al., 2019).
The most comprehensive TCM researched through CMAP is probably Salvia miltiorrhiza Bunge. As a medicinal model plant, Danshen Transcriptional Resource Database (DsTRD) has been constructed for the reference of other researches (Shao et al., 2016). And compound Danshen dropping pill (DSP) is of great benefit in the treatment of atherosclerosis. Wei Zhou et al. first identified 10 candidates for active ingredients in it (Zhou et al., 2016a). And then Chao Lv found that tanshinone IIA (Tan IIA) (the main active ingredient derived from Danshen) and mTOR inhibitors, Hsp90 inhibitors, PKC inhibitors, and PI3K inhibitors have positive correlation in regard to gene expression characteristics, thus further demonstrating the antitumor mechanism of Tan IIA. Overall, Tan IIA may restrain activity of total protein kinase C (PKC) and Ras/MAPK pathway. In addition, it also significantly inhibited the PI3K/Akt/mTOR signaling pathway and induced cell cycle arrest and autophagy (Lv et al., 2018).
The efficacy of TCM formula that consists of two or more herbs is the result of the synergistic effect of various natural compounds (Yuan et al., 2017). However, there is no strong evidence to fully prove the synergistic effect of Chinese herbal medicine. Some opponents assert that TCM formula is more likely to work as a placebo than to have more active ingredients. Inversely, most clinical cases show that TCM formula is more effective than single drug in the treatment of some chronic diseases or diseases with complicated causes (Zhou et al., 2016b). CMAP technology can appropriately reveal the synergistic effect of some TCM formula based on multiactive components and multitarget mechanism. At the same time, it can account for the magic that TCM formula suitable for a certain “ZHENG” is also efficacious for other different diseases in the perspective of western medicine (Zhang and Wang, 2014). Through the analysis of difference in the RNA expression of leukocyte in patients with qi-deficiency-blood-stasis syndrome before and after treatment with Fuzheng Huayu capsule, CMAP query found that Fuzheng Huayu capsule possesses antihyperglycemia, antihyperlipidemia, antihypotension, anti-inflammatory, and other effects. Its potential mechanism may involve Ca2+ related pathway (Yu et al., 2012). The four main active components of Salvia miltiorrhiza Bunge are tanshinone IIA, salvianic acid A sodium, protocatechuic aldehyde, and salvianolic acid B. Lv et al. applied the algorithm of random walk with restart (RWR) to compute the cardiovascular effect scores of the mixture of the four components and each single component separately. The mixture of the four components obtained a high effect score of 0.72 and the Z-score of 4.958, which was much higher than that of any single component. It suggested that the synergy among the four components is significantly associated with the effects of Danshen on cardiovascular disease (Lv et al., 2017). QiShenYiQi (QSYQ), a Chinese medicine, is composed of Astragalus propinquus Schischkin (Huangqi), Salvia miltiorrhiza (Danshen), Panax notoginseng (Sanqi), and Dalbergia odorifera (Jiangxiang). Query labels were identified from the rat model of myocardial infarction treated with QSYQ, and complex pathways of QSYQ's synergistic effect on myocardial infarction were delineated through compound-target-pathway network (Li et al., 2014).
The ultimate goal of all research is to help the treatment of clinical diseases. The link between diseases and herbal medicines has been a focus of research. Recently, Xuetong Chen et al. took 189 disease gene expression profiles as pathological characteristics and 502 herbal medicine profiles as disturbance information and integrated the data to obtain the optimal combination of diseases based on this strategy (Chen et al., 2018). When CMAP was mentioned above to identify candidate drug for certain diseases, the obesity drug candidate Celastrol (the root extract of Thunder of God Vine) was introduced (Liu et al., 2015). Biochemical and pharmacological experiments indicated that the water-soluble polysaccharide MDG-1 extracted from Radix Ophiopogonis had strong effect of hypoglycemic and weight loss and was also a candidate drug for obesity-related metabolic diseases (Wang et al., 2014). In the same way, we could use CMAP to confirm the connection of TCM and corresponding diseases at the genetic level. For example, the gene expression profile of MCF7 cells treated with Si-Wu-Tang was put into CMAP, and the result disclosed that the score of estrogens was high, and the score of estrogen receptor antagonist was low. This falls into line with the fact that Si-Wu-Tang does well in gynecological diseases (Wen et al., 2011). In addition, CMAP and functional network approach using in vitro cell lines can be used to evaluate the pharmacological effects of drugs with in vivo systems. Kim et al. analyzed the microarray information of HepG2 cells treated with ESB (an extract of Scutellaria baicalensis) and input it into CMAP to obtain pharmaceutical profile, namely, the correlation set of small molecular drugs in CMAP. The pharmaceutical profiles of db/db mouse liver and human diabetic liver were obtained by the same method. Enrichment scores from human diabetic liver correlated positively with those of db/db mouse liver but inversely with those of ESB-treated HepG2 cells. Then common functional modules were established through functional network analysis to predict therapeutic effect of ESB on human diabetes finally (Kim et al., 2017). It is hoped that this method is of service to predict the pharmacological effects of herbs in vivo system by using cell lines in vitro.
Drug repurposing refers to seeking out undiscovered roles for existing drugs by dint of certain computational methods (De Bastiani et al., 2018). There are two mainstream methods: one is that structure determines properties paradigm; the other is based on omics data, especially the whole genome expression database (Sun et al., 2017). CMAP belongs to the latter, which makes it possible to reposition drugs by systematically comparing drug-related gene expression profiles. Dudly et al. compared the expression characteristics of IBD (inflammatory bowel disease) genes from public microarray data with those of 164 drug compounds to infer novel therapeutic relationships between drug–disease changes. Among the top-ranked drugs, in addition to the corticosteroid prednisone for traditional treatment of IBD, there was the antiepileptic drug topiramate, which was found to be more effective in subsequent IBD rat experiments (Dudley et al., 2011). In the same way, TCM has an array of functions to be excavated. For example, the most recent study of snake venom used functional genomics and concatenation mapping to recharacterize the biological activity of the Bothrops jararaca venom of the South American pit viper, suggesting that it may be useful in the treatment of neuropsychiatric and cardiovascular diseases (Nicolau et al., 2018). Drug developers can further research and develop drugs with potential clinical benefits. It takes billions of investment dollars and an average of about 9–12 years to bring a new drug to the market (Dickson and Gagnon, 2004). Investment in drug development has risen steadily, while the number of new drug approvals has stagnated. Compared to new drug discovery, CMAP as a computational drug repositioning may reduce the time and cost of drug discovery in preclinical and phase I trials, although repositioning of drugs may still present challenges after phase II trials (Li et al., 2016).
Zhou Zhongying, a master of Chinese medicine, once said that “the recession of traditional Chinese physician could be put down to traditional Chinese medicine.” It is foundational and crucial to pore through the cause of TCM. At present, the trend of TCM research is to extract single compound for further molecular biology study. The key to this approach is to find the effective single compound. As mentioned above, CMAP can be used to find the active ingredients of TCM, so it is of benefit to provide a direction for the extraction of single compound. However, traditional medical concept of TCM and modern pharmacology give us quite different feeling. Providing that we make use of the idea of single target and single component of western medicine, it is difficult to reflect the systematicness and synergism of TCM (Jiang, 2005). The synergistic therapeutic effect of TCM is derived from the complex interactions between a variety of bioactive ingredients in herbal medicines or herbal formulations. CMAP can reflect the synergistic effect of TCM through changes in gene expression. Moreover, compared with the low throughput of pharmacology and toxicology, CMAP has the advantages of being comprehensive, of high throughput, and accurate. The Broad Institute released the Library of Integrated Network-based Cellular Signatures (LINCS) which cover a vastly broader range of cell types and perturbagens (20,413 small molecules and 22,119 genetic perturbagens), along with the inferred expression of a further 21,305 probes (Keenan et al., 2018). Confronting with these challenges, new technologies stand out from the crowd, such as TCM proteomics, network pharmacology, and herbgenomics.
TCM proteomics seeks for potential targets through the differences in protein expression after the treatment of TCM or analyzes the differences in proteins from different parts of Chinese herbal medicine to find active components (Le et al., 2016). But most remain in the differentially expressed protein (DEP) levels, not clarifying internal mechanism (Suo et al., 2016). CMAP is committed to explore the MoAs of TCM. Wei Liu et al. used WGCNA to analyze the CMAP microarray dataset and isolated drugs into useful modules. They focused on the redundancy of gene function and gene-to-gene interactions that help predict drug behavior and identify MoAs (Liu et al., 2018).
After network pharmacology was firstly introduced into medical research by professor Andrew Hopkins L. (Hopkins, 2007), the researchers of Chinese medicine render it promising in collaboration with TCM, which broke the traditional model of “the single ingredient-the single target-the single disease” and made “disease-gene-targets-drug” integrate a network through the computer technology. Actually, it is quite a complex database with diverse genes, proteins, diseases, and drugs mutually crosslinked to form a whole (Li and Zhang, 2013). But network pharmacology of TCM lacks complex network pharmacology model to carry out plenty of work (Zhang et al., 2019). Beyond that, certain TCM with single active compound may well become complicated under guidance of network pharmacology theory. CMAP can simplify the study of TCM with complicated components and mechanisms and will not complicate the study of simple drugs.
The concept of herbgenomics was first proposed by Chen Shilin et al. (Hu et al., 2019). Herbgenomics in a narrow sense refers to beginning from the whole genome sequencing of TCM, gene mapping, and gene function analysis. It intends to establish systematic research of the original plant genome, with affirmative effect in distinguishing species of Chinese herbal medicine, looking for patterns, explaining biology essence of trueborn herb, and controlling quality of TCM. These are all basic studies of Chinese herbal medicine and do not involve disease or clinic. CMAP could confirm the connection of TCM and corresponding diseases at the genetic level from above. Cheng et al. compiled a set of 890 true drug-disease pairs from two different sources as a benchmarking standard. The disease signatures were generated using Gene Logic BioExpressTM system and the compound profiles were derived from the CMAP. The similarity scoring algorithm called eXtreme Sum (XSum) can achieve a fourfold enrichment at 0.01 false positive rate level, which confirms the high accuracy of CMAP in predicting association of drug and disease (Cheng et al., 2014).
In the wake of the emergence of microarray technology and the wide application of gene expression profile in pharmaceutical research, CMAP, as a database connecting genes, drugs, and diseases, has been extensively used to elucidate the mechanism of action, discover potential drugs for diseases, and relocate new drugs (Musa et al., 2018). TCM as the gem of China is an indispensable part of people's healthy life. Compared with traditional pharmacology, toxicology, Chinese medicine chemistry, and other research methods, CMAP has the characteristics of high throughput and being automatic, rapid, and simple. Besides modern methods such as herbgenomics and network pharmacology, it is also outstanding relying on the advantage of being more comprehensive and efficient (Liu et al., 2018). For instance, network pharmacology is only appropriate for the analysis of complicated TCM, whereas some simple ones tend to be complicated and not effectively distinguished (Liang et al., 2014). CMAP can identify multicomponent and multitarget drugs from the gene level and can easily research simple drugs as well. In addition, gene expression profiles of 102 TCM ingredients were recently studied, and a small CMAP database of TCM was established for the first time as a general template for TCM research (Lv et al., 2017). Minjae Yoo et al. further classified the molecular mechanisms of these TCMs (Yoo et al., 2018). CMAP has succeeded in analyzing the molecular functions of TCM, the synergistic mechanism of TCM components, the multiple functions of some compounds, and the detoxification theory of TCM (Zhang et al., 2016). Nevertheless, CMAP still demonstrates the issues. One is the limitations of the database itself. For example, we need to distinguish between widely activated conserved genes and widely expressed genes from those that are authentically regulated by drugs. In particular, some low-expressed genes are susceptible to detection noise (Zou et al., 2017). Otherwise, many unrelated genes involved in the construction of “connectivity” may be ambiguous, so OneComp was applied in advance to screening for differentially expressed genes (DEGs) related to treatment (He et al., 2017). Furthermore, combining the data of different cell lines through the probabilistic model can basically ignore the influence of different cell line gene data (Parkkinen and Kaski, 2014). The other is the limitation of the drugs in question. Certain drugs are not enough to be studied through CMAP, such as ingredients that work only after long-term exposure in vivo and components whose target genes are not expressed in all types of cells, etc. In particular, established gene expression profiles for the study of TCM components are only 102 molecules (Lv et al., 2017). This method can only pore through the connections from the known corresponding relationship between TCM molecules and genes in the database, but it fails to find new targets or pathways. In future studies, more investigators will further expand the number of small molecules. These data will provide more possibilities for the study of the molecular mechanism of TCM and eventually establish its own database. A previous study has illustrated that about 5% of the tested hypothesis of drug–gene interaction is verified in Connectivity Map (Mears et al., 2017). In a word, this way of using CMAP to study TCM is promising and tortuous and arduous, which entails people to keep on studying with the help of bioinformatics and other modern scientific technologies. Connectivity Map is doomed to be a sword to promote the development of TCM.
MC designed the review. HJ and CH collected data from publications. HJ developed the database, wrote the manuscript, and edited the final text. MC provided financial support.
This work was supported by research grants from the National Nature Science Foundation of China (No.81503374), the Open Projects of the Discipline of Chinese Medicine of Nanjing University of Chinese Medicine Supported by the Subject of Academic Priority Discipline of Jiangsu Higher Education Institutions (PAPD, ZYX03KF047) and Traditional Chinese and Western Medicine Clinical Medicine Brand Construction Project of Jiangsu Higher Education Institutions (Phase II); No:2020PPZXL261.
The authors declare that the research was conducted in the absence of any commercial or financial relationships that could be construed as a potential conflict of interest.
Ayati, S. H., Fazeli, B., Momtazi-Borojeni, A. A., Cicero, A. F. G., Pirro, M., and Sahebkar, A. (2017). Regulatory effects of berberine on microRNome in cancer and other conditions. Crit. Rev. Oncol. Hematol. 116, 147–158. doi:10.1016/j.critrevonc.2017.05.008
Beck, A., Eberherr, C., Hagemann, M., Cairo, S., Haberle, B., Vokuhl, C., et al. (2016). Connectivity map identifies HDAC inhibition as a treatment option of high-risk hepatoblastoma. Cancer Biol. Ther. 17, 1168–1176. doi:10.1080/15384047.2016.1235664
Boratyn, G. M., Datta, S., and Datta, S. (2006). Biologically supervised hierarchical clustering algorithms for gene expression data. Conf. Proc. IEEE Eng. Med. Biol. Soc. 1, 5515–5518. doi:10.1109/iembs.2006.260308
Brody, J. P., Babcock, J. J., Du, F., Xu, K., Wheelan, S. J., and Li, M. (2013). Integrated analysis of drug-induced gene expression profiles predicts novel hERG inhibitors. PLoS One 8, e69513. doi:10.1371/journal.pone.0069513
Brum, A. M., van de Peppel, J., Nguyen, L., Aliev, A., Schreuders-Koedam, M., Gajadien, T., et al. (2018). Using the Connectivity Map to discover compounds influencing human osteoblast differentiation. J. Cell. Physiol. 233, 4895–4906. doi:10.1002/jcp.26298
Brum, A. M., van de Peppel, J., van der Leije, C. S., Schreuders-Koedam, M., Eijken, M., van der Eerden, B. C. J., et al. (2015). Connectivity Map-based discovery of parbendazole reveals targetable human osteogenic pathway. Proc. Natl. Acad. Sci. U.S.A. 112, 12711–12716. doi:10.1073/pnas.1501597112
Chen, X., Zheng, C., Wang, C., Guo, Z., Gao, S., Ning, Z., et al. (2018). Systems-mapping of herbal effects on complex diseases using the network-perturbation signatures. Front. Pharmacol. 9, 1174. doi:10.3389/fphar.2018.01174
Cheng, J., Yang, L., Kumar, V., and Agarwal, P. (2014). Systematic evaluation of connectivity map for disease indications. Genome Med. 6, 540. doi:10.1186/s13073-014-0095-1
De Bastiani, M. A., Pfaffenseller, B., and Klamt, F. (2018). Master regulators connectivity map: a transcription factors-centered approach to drug repositioning. Front. Pharmacol. 9, 697. doi:10.3389/fphar.2018.00697
Dickson, M., and Gagnon, J. P. (2004). Key factors in the rising cost of new drug discovery and development. Nat. Rev. Drug Discov. 3, 417–429. doi:10.1038/nrd1382
Dudley, J. T., Sirota, M., Shenoy, M., Pai, R. K., Roedder, S., Chiang, A. P., et al. (2011). Computational repositioning of the anticonvulsant topiramate for inflammatory bowel disease. Sci. Transl. Med. 3, 96ra76. doi:10.1126/scitranslmed.3002648
Fang, E., and Zhang, X. (2017). Identification of breast cancer hub genes and analysis of prognostic values using integrated bioinformatics analysis. Cancer Biomark. 21, 373–381. doi:10.3233/CBM-170550
Gao, Y., Kim, S., Lee, Y. I., and Lee, J. (2019). Cellular stress-modulating drugs can potentially be identified by in silico screening with connectivity map (CMap). Int. J. Mol. Sci. 20, 5601–5656. doi:10.3390/ijms20225601
He, J., Yan, H., Cai, H., Li, X., Guan, Q., Zheng, W., et al. (2017). Statistically controlled identification of differentially expressed genes in one-to-one cell line comparisons of the CMAP database for drug repositioning. J. Transl. Med. 15, 198–211. doi:10.1186/s12967-017-1302-9
Hopkins, A. L. (2007). Network pharmacology. Nat. Biotechnol. 25, 1110–1111. doi:10.1038/nbt1007-1110
Hu, H., Shen, X., Liao, B., Luo, L., Xu, J., and Chen, S. (2019). Herbgenomics: a stepping stone for research into herbal medicine. Sci. China Life Sci. 62, 913–920. doi:10.1007/s11427-018-9472-y
Huang, H., Nguyen, T., Ibrahim, S., Shantharam, S., Yue, Z., and Chen, J. Y. (2015). DMAP: a connectivity map database to enable identification of novel drug repositioning candidates. BMC Bioinform. 16, 1–11. doi:10.1186/1471-2105-16-S13-S4
Hughes, T. R., Marton, M. J., Jones, A. R., Roberts, C. J., Stoughton, R., Armour, C. D., et al. (2000). Functional discovery via a compendium of expression profiles. Cell 102, 109–126. doi:10.1016/s0092-8674(00)00015-5
Jiang, W. Y. (2005). Therapeutic wisdom in traditional Chinese medicine: a perspective from modern science. Trends Pharmacol. Sci. 5, 455–461. doi:10.1016/j.tips.2005.09.006
Keenan, A. B., Jenkins, S. L., Jagodnik, K. M., Koplev, S., He, E., Torre, D., et al. (2018). The library of integrated network-based cellular signatures NIH program: system-level cataloging of human cells response to perturbations. Cell Syst. 6, 13–24. doi:10.1016/j.cels.2017.11.001
Kim, B. Y., Song, K. H., Lim, C. Y., and Cho, S. I. (2017). Therapeutic properties of Scutellaria baicalensis in db/db mice evaluated using Connectivity Map and network pharmacology. Sci. Rep. 7, 41711. doi:10.1038/srep41711
Kuhn, M., Szklarczyk, D., Pletscher-Frankild, S., Blicher, T. H., von Mering, C., Jensen, L. J., et al. (2014). Stitch 4: integration of protein-chemical interactions with user data. Nucl. Acids Res. 42, D401–D407. doi:10.1093/nar/gkt1207
Lamb, J., Crawford, E. D., Peck, D., Modell, J. W., Blat, I. C., Wrobel, M. J., et al. (2006). The Connectivity Map: using gene-expression signatures to connect small molecules, genes, and disease. Science 313 (5795), 1929–1935. doi:10.1126/science.1132939
Le, L., Jiang, B. P., Xu, J., Hu, K. P., and Chen, S. L. (2016). Research strategy of traditional Chinese medicine proxemics. Zhongguo Zhong Yao Za Zhi 41, 4096–4102. doi:10.4268/cjcmm20162203
Lee, K. H., Lo, H. L., Tang, W. C., Hsiao, H. H., and Yang, P. M. (2014). A gene expression signature-based approach reveals the mechanisms of action of the Chinese herbal medicine berberine. Sci. Rep. 4, 6394. doi:10.1038/srep06394
Li, J., Rong, M. H., Dang, Y. W., He, R. Q., Lin, P., Yang, H., et al. (2019). Differentially expressed gene profile and relevant pathways of the traditional Chinese medicine cinobufotalin on MCF-7 breast cancer cells. Mol. Med. Rep. 19, 4256–4270. doi:10.3892/mmr.2019.10062
Li, J., Zheng, S., Chen, B., Butte, A. J., Swamidass, S. J., and Lu, Z. (2016). A survey of current trends in computational drug repositioning. Brief. Bioinform. 17 (1), 2–12. doi:10.1093/bib/bbv020
Li, S., and Zhang, B. (2013). Traditional Chinese medicine network pharmacology: theory, methodology and application. Chin. J. Nat. Med. 11, 110–120. doi:10.1016/s1875-5364(13)60037-0
Li, X., Wu, L., Liu, W., Jin, Y., Chen, Q., Wang, L., et al. (2014). A network pharmacology study of Chinese medicine QiShenYiQi to reveal its underlying multi-compound, multi-target, multi-pathway mode of action. PLoS One 9, e95004. doi:10.1371/journal.pone.0095004
Liang, X., Li, H., and Li, S. (2014). A novel network pharmacology approach to analyse traditional herbal formulae: the Liu-Wei-Di-Huang pill as a case study. Mol. Biosyst. 10, 1014–1022. doi:10.1039/c3mb70507b
Liu, J. L., Lee, J., Hernandez, M. A. S., Mazitschek, R., and Ozcan, U. (2015). Treatment of obesity with celastrol. Cell 161, 999–1011. doi:10.1016/j.cell.2015.05.011
Liu, W., Tu, W., Li, L., Liu, Y., Wang, S., Li, L., et al. (2018). Revisiting Connectivity Map from a gene co-expression network analysis. Exp. Ther. Med. 16, 493–500. doi:10.3892/etm.2018.6275
Luo, B., Gu, Y. Y., Wang, X. D., Chen, G., and Peng, Z. G. (2018). Identification of potential drugs for diffuse large b-cell lymphoma based on bioinformatics and connectivity map database. Pathol. Res. Pract. 214, 1854–1867. doi:10.1016/j.prp.2018.09.013
Lv, C., Wu, X., Wang, X., Su, J., Zeng, H., Zhao, J., et al. (2017). The gene expression profiles in response to 102 traditional Chinese medicine (TCM) components: a general template for research on TCMs. Sci. Rep. 7, 352. doi:10.1038/s41598-017-00535-8
Lv, C., Zeng, H. W., Wang, J. X., Yuan, X., Zhang, C., Fang, T., et al. (2018). The antitumor natural product tanshinone IIA inhibits protein kinase C and acts synergistically with 17-AAG. Cell Death Dis. 9, 165. doi:10.1038/s41419-017-0247-5
Malcomson, B., Wilson, H., Veglia, E., Thillaiyampalam, G., Barsden, R., Donegan, S., et al. (2016). Connectivity mapping (ssCMap) to predict A20-inducing drugs and their antiinflammatory action in cystic fibrosis. Proc. Natl. Acad. Sci. U.S.A. 113, E3725–E3734. doi:10.1073/pnas.1520289113
Manzotti, G., Parenti, S., Ferrari-Amorotti, G., Soliera, A. R., Cattelani, S., Montanari, M., et al. (2015). Monocyte-macrophage differentiation of acute myeloid leukemia cell lines by small molecules identified through interrogation of the connectivity map database. Cell Cycle 14, 2578–2589. doi:10.1080/15384101.2015.1033591
Mears, A. J., Schock, S. C., Hadwen, J., Putos, S., Dyment, D., Boycott, K. M., et al. (2017). Mining the transcriptome for rare disease therapies: a comparison of the efficiencies of two data mining approaches and a targeted cell-based drug screen. NPJ Genom Med. 2, 14–22. doi:10.1038/s41525-017-0018-3
Musa, A., Ghoraie, L. S., Zhang, S. D., Glazko, G., Yli-Harja, O., Dehmer, M., et al. (2018). A review of connectivity map and computational approaches in pharmacogenomics. Brief. Bioinform. 19, 506–523. doi:10.1093/bib/bbw112
Nicolau, C. A., Prorock, A., Bao, Y., Neves-Ferreira, A., Valente, R. H., and Fox, J. W. (2018). Revisiting the therapeutic potential of Bothrops jararaca venom: screening for novel activities using connectivity mapping. Toxins 10, 69. doi:10.3390/toxins10020069
Parkkinen, J. A., and Kaski, S. (2014). Probabilistic drug connectivity mapping. BMC Bioinform. 15, 113. doi:10.1186/1471-2105-15-113
Qu, X. A., and Rajpal, D. K. (2012). Applications of Connectivity Map in drug discovery and development. Drug Discov. Today 17, 1289–1298. doi:10.1016/j.drudis.2012.07.017
Quan, Y., Li, B., Sun, Y. M., and Zhang, H. Y. (2014). Elucidating pharmacological mechanisms of natural medicines by biclustering analysis of the gene expression profile: a case study on curcumin and Si-Wu-Tang. Int. J. Mol. Sci. 16, 510–520. doi:10.3390/ijms16010510
Shao, Y., Wei, J., Wu, F., Zhang, H., Yang, D., Liang, Z., et al. (2016). DsTRD: Danshen transcriptional resource database. PloS One 11, e0149747. doi:10.1371/journal.pone.0149747
Shoemaker, D. D., Lashkari, D. A., Morris, D., Mittmann, M., and Davis, R. W. (1996). Quantitative phenotypic analysis of yeast deletion mutants using a highly parallel molecular bar-coding strategy. Nat. Genet. 14, 450–456. doi:10.1038/ng1296-450
Smalley, J. L., Breda, C., Mason, R. P., Kooner, G., Luthi-Carter, R., Gant, T. W., et al. (2016). Connectivity mapping uncovers small molecules that modulate neurodegeneration in Huntington's disease models. J. Mol. Med. 94, 235–24545. doi:10.1007/s00109-015-1344-5
Smith, I., Greenside, P. G., Natoli, T., Lahr, D. L., Wadden, D., Tirosh, I., et al. (2017). Evaluation of RNAi and CRISPR technologies by large-scale gene expression profiling in the connectivity map. PLoS Biol. 15, e2003213. doi:10.1371/journal.pbio.2003213
Subramanian, A., Tamayo, P., Mootha, V. K., Mukherjee, S., Ebert, B. L., Gillette, M. A., et al. (2005). Gene set enrichment analysis: a knowledge-based approach for interpreting genome-wide expression profiles. Proc. Natl. Acad. Sci. U.S.A. 102, 15545–15550. doi:10.1073/pnas.0506580102
Sun, P., Guo, J., Winnenburg, R., and Baumbach, J. (2017). Drug repurposing by integrated literature mining and drug–gene–disease triangulation. Drug Discov. Today 22, 615–619. doi:10.1016/j.drudis.2016.10.008
Suo, T., Wang, H., and Li, Z. (2016). Application of proxemics in research on traditional Chinese medicine. Expert Rev. Proteomics 13, 873–881. doi:10.1080/14789450.2016.1220837
Vanderstocken, G., Dvorkin-Gheva, A., Shen, P., Brandsma, C. A., Obeidat, M., Bossé, Y., et al. (2018). Identification of drug candidates to suppress cigarette smoke-induced inflammation via connectivity map analyses. Am. J. Respir. Cell Mol. Biol. 58, 727–735. doi:10.1165/rcmb.2017-0202OC
Wang, K., Weng, Z., Sun, L., Sun, J., Zhou, S.-F., and He, L. (2015). Systematic drug safety evaluation based on public genomic expression (connectivity map) data: myocardial and infectious adverse reactions as application cases. Biochem. Biophys. Res. Commun. 457, 249–255. doi:10.1016/j.bbrc.2014.12.096
Wang, Y., Zhu, Y., Ruan, K., Wei, H., and Feng, Y. (2014). MDG-1, a polysaccharide from Ophiopogon japonicus, prevents high fat diet-induced obesity and increases energy expenditure in mice. Carbohydr. Polym. 114, 183–189. doi:10.1016/j.carbpol.2014.08.013
Wen, Q., O'Reilly, P., Dunne, P. D., Lawler, M., Van Schaeybroeck, S., Salto-Tellez, M., et al. (2015). Connectivity mapping using a combined gene signature from multiple colorectal cancer datasets identified candidate drugs including existing chemotherapies. BMC Syst. Biol. 9 (Suppl. 5), S4. doi:10.1186/1752-0509-9-s5-s4
Wen, Z., Wang, Z., Wang, S., Ravula, R., Yang, L., Xu, J., et al. (2011). Discovery of molecular mechanisms of traditional Chinese medicinal formula Si-Wu-Tang using gene expression microarray and connectivity map. PLoS One 6, e1878. doi:10.1371/journal.pone.0018278
Xiao, S. J., Zhu, X. C., Deng, H., Zhou, W. P., and Xia, H. M. (2018). Gene expression profiling coupled with Connectivity Map database mining reveals potential therapeutic drugs for Hirschsprung disease. J. Pediatr. Surg. 53, 49380–49394. doi:10.1016/j.jpedsurg.2018.02.060
Xue, Y., Chung, F.-H., Chiang, Y.-R., Tseng, A.-L., Sung, Y.-C., Lu, J., et al. (2014). Functional Module Connectivity Map (FMCM): a framework for searching repurposed drug compounds for systems treatment of cancer and an application to colorectal adenocarcinoma. PLoS One 9, e86299. doi:10.1371/journal.pone.0086299
Yang, H., Shang, D., Xu, Y., Zhang, C., Feng, L., Sun, Z., et al. (2017). The LncRNA Connectivity Map: using lncRNA signatures to connect small molecules, lncRNAs, and diseases. Sci. Rep. 7, 1–6. doi:10.1038/s41598-017-06897-3
Yoo, M., Shin, J., Kim, H., Kim, J., Kang, J., and Tan, A. C. (2018). Exploring the molecular mechanisms of Traditional Chinese Medicine components using gene expression signatures and connectivity map. Comput. Methods Progr. Biomed. 17, S0169–S2607. doi:10.1016/j.cmpb.2018.04.002
Yu, J., Putcha, P., and Silva, J. M. (2015). Recovering drug-induced apoptosis subnetwork from Connectivity Map data. Biomed. Res. Int. 2015, 1–11. doi:10.1155/2015/708563
Yu, S., Guo, Z., Guan, Y., Lu, Y. Y., Hao, P., Li, Y., et al. (2012). Combining ZHENG theory and high-throughput expression data to predict new effects of Chinese herbal formulae. Evid Based Compl. Alternat. Med. 2012, 986427. doi:10.1155/2012/986427
Yuan, H., Ma, Q., Cui, H., Liu, G., Zhao, X., Li, W., et al. (2017). How can synergism of traditional medicines benefit from network pharmacology?. Molecules 22, 1135. doi:10.3390/molecules22071135
Zhang, H. J., and Wang, Z. X. (2014). Yin-yang and zheng: exported from Chinese medicine. Chin. J. Integr. Med. 20, 250–255. doi:10.1007/s11655-014-1777-z
Zhang, L., Kang, W., Lu, X., Ma, S., Dong, L., and Zou, B. (2018). Weighted gene co-expression network analysis and connectivity map identifies lovastatin as a treatment option of gastric cancer by inhibiting HDAC2. Gene 681, 15–25. doi:10.1016/j.gene.2018.09.040
Zhang, S. D., and Gant, T. W. (2009). sscMap: an extensible java application for connecting small-molecule drugs using gene-expression signatures. BMC Bioinform. 10, 236. doi:10.1186/1471-2105-10-236
Zhang, W., Huai, Y., Miao, Z., Qian, A., and Wang, Y. (2019). Systems pharmacology for investigation of the mechanisms of action of traditional Chinese medicine in drug discovery. Front. Pharmacol. 10, 743. doi:10.3389/fphar.2019.00743
Zhang, X., Kang, Y., Su, J., and Kong, X. (2016). Application of connectivity map technology in traditional Chinese medicines. J. Zhejiang Univ. Agri. Life Sci. 42, 543–550. doi:10.3785/j.isn.1008-9209.2016.03.041
Zhou, W., Yuan, W. F., Chen, C., Wang, S. M., and Liang, S. W. (2016a). Study on material base and action mechanism of compound Danshen dripping pills for treatment of atherosclerosis based on modularity analysis. J. Ethnopharmacol. 193, 36–44. doi:10.1016/j.jep.2016.07.014
Zhou, X., Seto, S. W., Chang, D., Kiat, H., Razmovski-Naumovski, V., Chan, K., et al. (2016b). Synergistic effects of Chinese herbal medicine: a comprehensive review of methodology and current research. Front. Pharmacol. 7, 201. doi:10.3389/fphar.2016.00201
Keywords: connectivity map, traditional Chinese medicine, Chinese herbal compound, synergetic mechanism, TCM repurposing
Citation: Jiang H, Hu C and Chen M (2021) The Advantages of Connectivity Map Applied in Traditional Chinese Medicine. Front. Pharmacol. 12:474267. doi: 10.3389/fphar.2021.474267
Received: 06 June 2019; Accepted: 11 January 2021;
Published: 11 March 2021.
Edited by:
Lie-Fen Shyur, Agricultural Biotechnology Research Center, TaiwanReviewed by:
Hong-Yu Zhang, Huazhong Agricultural University, ChinaCopyright © 2021 Jiang, Hu and Chen. This is an open-access article distributed under the terms of the Creative Commons Attribution License (CC BY). The use, distribution or reproduction in other forums is permitted, provided the original author(s) and the copyright owner(s) are credited and that the original publication in this journal is cited, in accordance with accepted academic practice. No use, distribution or reproduction is permitted which does not comply with these terms.
*Correspondence: Meijuan Chen, bWpjaGVuQG5qdWNtLmVkdS5jbg==
Disclaimer: All claims expressed in this article are solely those of the authors and do not necessarily represent those of their affiliated organizations, or those of the publisher, the editors and the reviewers. Any product that may be evaluated in this article or claim that may be made by its manufacturer is not guaranteed or endorsed by the publisher.
Research integrity at Frontiers
Learn more about the work of our research integrity team to safeguard the quality of each article we publish.