- 1Department of Orthopedics, Peking University Third Hospital, Beijing, China
- 2Drug Clinical Trial Center, Peking University Third Hospital, Beijing, China
- 3HR-Biopharm Technology Co., Ltd., Wuhan, China
- 4Pharmaron Beijing Co., Ltd., Beijing, China
- 5Shanghai Qiangshi Information Technology Co., Ltd., Shanghai, China
To evaluate the biodistribution of hydroxychloroquine (HCQ) in cynomolgus macaques and receive dynamic quantitative relationship between plasma, blood, and lung tissue concentration using the population pharmacokinetic modeling method, seventeen cynomolgus macaques were divided into six groups according to different HCQ dosing regimens over 5 days. The monkeys were euthanized, and blood, plasma, urine, feces and ten tissues were collected. All the samples were prepared by protein precipitation and analyzed by HPLC-MS/MS detection. The population pharmacokinetics of HCQ in the plasma, red blood cells, and lung tissue was conducted and simulated via ADAPT program. Results demonstrated that the maximum concentration (Cmax) of HCQ was 292.33 ng/mL in blood and 36.90 ng/mL in plasma after single dose of 3 mg/kg. The value of area under curve (AUC0–∞) was determined as 5,978.94 and 363.31 h* ng/mL for the blood and plasma, respectively. The descending order of the tissue-to-plasma concentration ratio was liver > spleen > kidney > lung > heart > subcutaneous fat > brain. The tissue-to-plasma concentration ratio and the tissue-to-blood concentration ratio for lung were found to be time-dependent with 267.38 and 5.55 at 120 h postdose, respectively. A five-compartment model with first-order oral absorption and elimination best described the plasma, blood, and lung tissue pharmacokinetics. The estimated elimination rate constant (ke) for a typical monkey was 0.236 h−1. The volume of distribution in central (Vc/F) and other two peripheral compartments (Vb/F and Vl/F) were 114, 2.68, and 5.55 L, respectively. Model-based simulation with PK parameters from cynomolgus macaques showed that the ratio of the blood or plasma to lung tissue was a dynamic change course, which suggested that the rate of HCQ concentration decrease in the blood or plasma was faster than that in the lung tissue. HCQ was found to be accumulated in tissues, especially in the liver, kidney, lung, and spleen. Also, the tissue-to-plasma concentration ratio increased over time. The population pharmacokinetic model developed could allow for the assessment of pharmacokinetics–pharmacodynamics relationships, especially relevant tissue concentration-response for HCQ. Determining appropriate treatment regimens in animals allows translation of these to clinical studies.
Introduction
As a 4-aminoquinoline compound with the hydroxyl group based on structure of chloroquine, hydroxychloroquine (HCQ) has a similar therapeutic effect but less side effects compared with other antimalarial drugs. Several clinical practices confirmed that HCQ has good safety and reliable efficacy in various rheumatic diseases such as systemic lupus erythematosus and rheumatoid arthritis. Furthermore, it has been used as basic treatment for rheumatic immune diseases.
Since the outbreak of the novel coronavirus disease (COVID-19) in December 2019, which is caused by severe acute respiratory syndrome coronavirus 2 (SARS-CoV-2), there are more than 21.2 million confirmed cases of those infected and nearly 770,000 deaths (WHO Situation Report, 2019). It has been confirmed that HCQ has a relatively strong anti-SARS-CoV-2 effect in several nonclinical studies (Liu et al., 2020; Wang et al., 2020; Yao et al., 2020). In addition, clinical research on the treatment of HCQ has been carried out around the world. However, there are differences in the HCQ dosing regimens among these clinical trials, and the effectiveness and safety of HCQ are still controversial (Gao et al., 2020; Gautret et al., 2020; Mehra et al., 2020; Molina et al., 2020). Therefore, the dosing regimen optimization of HCQ has become a key point in its clinical application. Due to the target site for the COVID-19 treatment mainly focus on the lung tissue and the transport mechanism of HCQ is similar to active transport (Xu et al., 2016), the blood concentration or plasma concentration of HCQ does not represent that in lung tissue. In addition, there are a broad range of EC50 values with different cell lines or different sampling times. Thus, it is necessary to conduct additional pharmacokinetic studies to understand how the concentration in the lung tissue relates to its efficacy.
However, there are still some difficulties in clinical studies, such as the ethical issues and operability of obtaining tissue samples. Physiologically based pharmacokinetic (PBPK) modeling, a powerful means of optimizing the dosing regimen, can integrate the drug physicochemical properties, in vitro experimental data, and human physiological parameters to predict the pharmacokinetic characteristics of the drug in vivo in a mechanistic manner.
In this study, we first established a high-performance liquid chromatography tandem mass spectrometry (HPLC-MS/MS) method for determining the concentration of HCQ and N-desethyl hydroxychloroquine (DHCQ) in cynomolgus monkeys. Then, we performed a biodistribution study following different HCQ dosing regimens in monkeys. We also conducted a population pharmacokinetics study to characterize the time-dependent pharmacokinetic characteristics, and then HCQ concentration in the lung, blood, and plasma were predicted so as to support the verification of HCQ physiologically based pharmacokinetic (PBPK) model to assist clinical dosing regimen optimization.
Materials and Methods
Materials and Reagents
The reference standard of hydroxychloroquine sulfate (100% purity), hydroxychloroquine sulfate-D4 (internal standard, IS), and N-desethyl hydroxychloroquine (DHCQ) bromide were purchased from TLC Pharmaceutical Standards Ltd. (Newmarket, Ontario, Canada). Hydroxychloroquine sulfate (99.90% purity), the experimental drug, was supplied by Jiangsu Shenhua Pharmaceutical Co., Ltd. Ammonium formate of analytical grade was purchased from Sinopharm Chemical Reagent Co., Ltd. (Beijing, China). Methanol, acetonitrile, and formic acid of HPLC grade were purchased from Fisher Scientific (Shanghai, China). Purified water obtained from a PURELAB Option-Q7 (ELGA LabWater, High Wycombe, United Kingdom) system was used throughout the experiment.
Experimental Animals
Cynomolgus macaques (4.13 ± 0.43 kg) were supplied by Shanghai Beijing Institute of Xieerxin Biology Resource (SCXK: 2015-0011). The animal experiments were carried out in accordance with the guidance from the Animal Care and Use Committee of the Pharmaron Beijing Co. Ltd. (IACUC No.16-120).
Equipment and HPLC-MS/MS Conditions
Biological samples were analyzed using an HPLC–MS/MS system. The liquid chromatography system (Shimadzu, Japan) was equipped with an LC-20ADXR binary liquid pump. Chromatographic separation was performed on a Venusil C18 Plus chromatographic column (5 µm, 2.1 × 50 mm, Agela), and the column temperature was maintained at 35 °C. The mobile phase consisted of 0.1% formic acid and 20 mM ammonium formate in water (mobile phase A) and acetonitrile (mobile phase B). The chromatographic separation was achieved using 3-min gradient elution. The gradient was 5% B at 0.0–0.01 min, 5%–30% B at 0.01–1.40 min, 30%–95% B at 1.40–1.50 min, 95% B at 1.50–2.30 min, 95%–5% B at 2.30–2.40 min, and 5% B at 2.40-3.00 min. The flow rate was set to 0.8 mL/min. The injection volume was 2.0 µL for all samples.
Mass spectrometric detection was performed on an AB Triple Quad 4500 mass spectrometer, equipped with an electrospray ionization (ESI) source (Turbo Spray ESI source) and Analyst 1.6.3 quantitative analysis software (American Applied Biosystems company). The ESI source was set in the positive mode, with a spray voltage of 5500 V. The ion source temperature was set to 600 °C. The pressure of ion source gas 1 (N2) and ion source gas 2 (N2) was both set to 50 psi. The air curtain gas (N2) pressure was 30 psi. Optimal multiple reaction monitoring (MRM) was selected, and the dwell time for each analyte was 150 ms. The ion pairs used for quantitative analysis were m/z 336.1→247.0 (hydroxychloroquine), m/z 308.2→247.0 (N-desethylhydroxychloroquine), and m/z 340.1→247.0 (IS, hydroxychloroquine-D4). The collision energy (CE) was set to 30 eV for each analyte.
Sample Preparation
For blood samples, an aliquot of 50 µL whole blood or plasma was mixed with 25 µL IS (500 ng/mL) solution and 50 µL zinc sulfate solution (0.2 mol/L). After thorough vortex mixing for 1 min, 400 µL acetonitrile containing 2% formic acid was added for protein precipitation. After thorough vortex mixing for 1 min, the samples were centrifuged at 1700g for 15 min, an aliquot containing 100 µL of the supernatant was diluted with 200 µL 90% acetonitrile (acetonitrile: H2O, 9:1, v/v), and the diluent was collected for HPLC-MS/MS analysis after thorough vortex mixing for 1 min. While for the plasma samples, the preparation method was the same as the blood samples, but without zinc sulfate solution.
Cynomolgus macaque (male, 7–8 weeks old, weight: 4.0 ± 0.8 kg) tissue samples were removed from the -70 °C refrigerator and placed on the ice tray to be cut into pieces. Tissues were placed in the homogenate tubes, and 10 times equivalent volume of 50% methanol (methanol: H2O, 1: 1, v/v) was added. The mixture was homogenized with a high-speed homogenizer, and the produced homogenate was stored at -70 °C until analysis. Similar to the preparation of the plasma, the tissue samples (50 µL) were mixed with 25 µL of IS (500 ng/mL) and 400 µL of acetonitrile containing 2% formic acid, then vortex mixed for 1 min, and centrifuged at 14,000 rpm for 5 min. An aliquot of 100 µL of the supernatant was diluted with 200 µL 90% acetonitrile (acetonitrile: H2O, 9:1, v/v), and the diluent was then analyzed by LC-MS/MS.
Method Validation
The selectivity, linearity, accuracy, precision, extraction recovery, and stability of the LC–MS/MS method used in the present study were evaluated as follows. The selectivity of this method was examined by analyzing the MRM chromatograms of blank monkey blood/plasma/tissue homogenate, blank monkey blood/plasma/tissue homogenate spiked with HCQ, DHCQ and IS, and monkey blood/plasma/tissue homogenate collected at indicated time after intragastric administration of hydroxychloroquine sulfate and spiked with IS.
The calibration samples of analytes were prepared by adding a series of different concentrations of working solution and IS working solution to monkey blood, plasma, or tissue homogenate to determine the linearity and LLOQ, then processed as described in “Section 2.4.” The calibration concentrations used for HCQ/DHCQ were 5.00/2.50, 10.00/5.00, 25.00/12.50, 50.00/25.00, 150.00/75.00, 500.00/150.00, 900.00/450.00, and 1,000.00/500.00 ng/mL in blood samples and 2.00/0.20, 4.00/0.40, 10.00/1.00, 20.00/2.00, 50.00/5.00, 100.00/10.00, 180.00/18.00, and 200.00/20.00 ng/mL in plasma samples. Additionally, the calibration concentrations of HCQ and DHCQ in tissue homogenate were 0.40, 0.80, 2.00, 5.00, 20.00, 180.00, and 200.00 ng/mL. Carryover of the tested analytes was assessed by injection of blank plasma samples after injection of the highest level calibration standard. The accuracy and precision assay for intraday and interday were evaluated by the analysis of three QC samples (n = 6) on the same day and on three consecutive days. The precision is expressed by the relative standard deviation (RSD).
The extraction recovery was determined by comparing the peak areas of the QC samples prespiked in blank blood, plasma, and tissue homogenates with those in the mobile phase (n = 6). The matrix effect was evaluated by comparing the peak areas of the QC samples postspiked in the blank blood, plasma, and tissue homogenate with those in the mobile phase (n = 6). The extraction recovery and matrix effect of the IS were determined in the same way. The stability of the tested analytes was evaluated using the QC samples spiked in blank monkey biological samples (n = 3) under the following conditions: (1) store at room temperature for 24 h; (2) three freeze-thaw cycles from −80 °C to room temperature; and (3) store at−80 °C for 30 days.
Pharmacokinetics Analysis
Cynomolgus macaques (male, weight: 4.13 ± 0.13 kg) were randomly divided into six groups from A to F. In group A, monkeys were given with 3 mg/kg hydroxychloroquine. In group B, monkeys were treated with 3 mg/kg hydroxychloroquine twice at D1 and D2, with an interval of 4 h. In group C, 2 mg/kg hydroxychloroquine was administered twice at D1, with an interval of 4 h, and then 1 mg/kg hydroxychloroquine twice daily was treated at D2 and D3, with an interval of 4 h. In group D, 6 mg/kg hydroxychloroquine was administered twice at D1, with an interval of 4 h, and then 2 mg/kg hydroxychloroquine twice daily was treated at D2 and D3, with an interval of 4 h. In group E, 21 mg/kg hydroxychloroquine was administered twice at D1, with an interval of 4 h. In group F, 21 mg/kg hydroxychloroquine was administered twice at D1, with an interval of 4 h, and then 7 mg/kg hydroxychloroquine twice daily was treated at D2∼D5, with an interval of 4 h. Hydroxychloroquine was administered intragastrically in all groups.
Blood samples (approximately 2 mL) were collected before and after dosing from the hind limb vein in polyethylene tubes at 1, 2, 4, 8, 24, 48, and 72 h with K2-EDTA as anticoagulant in groups A ∼ D. About 2 mL blood samples were collected at predose and 1, 2, 4, 8, 24, 48, 72, 144, 192, 240, and 264 h postdose with the same anticoagulant in group E. And about 2 mL blood samples were collected at predose and 1, 2, 4, 8, 24, 48, 72, 96, 97, 98, 100, 104, and 120 h postdose with the same anticoagulant in group F. Then, 2 mL blood sample was divided equally into two parts, one of which was stored at -70 °C until analysis without any treatment, while the other one was centrifuged at 2000 g for 10 min at 4 °C, and then, the harvested plasma samples were stored at -70 °C until analysis. The pharmacokinetic parameters t1/2 (the biological half-life), CLz (total body clearance), and AUC0–t (area under curve)were calculated from the plasma concentration–time data according to noncompartmental methods by WinNonlin (Version 8.1, Pharsight, Mountain View, CA) in a noncompartmental manner.
Tissue Distribution
Cynomolgus macaques (male, weight: 4.13 ± 0.13 kg) were randomly divided into six groups (n = 3 for each group, except group F (n = 2)). All individuals in each group were euthanized at 504, 336, 192, 240, 264, and 120 h following administration of hydroxychloroquine. Tissues (including the lung, bronchoalveolar fluid, liver, kidney, heart, spleen, brain, subcutaneous fat, and thymus) were harvested and homogenized by the methods described in “Section 2.4.” Each monkey was given 50 mL of normal saline irrigation for both lungs. All these samples were then stored at -70 °C until analysis.
Population PK Model Development
Subjects and Study Design
Total 17 cynomolgus macaques were included in this analysis. The dose regimen administered including a) 11.82–11.97 mg (3 individuals with dose time: 0, 4, 24, and 28 h), b) the first two loading doses in the range of 8.12–9.4 mg, following the 4 dose in the range of 4.06–4,72 mg (3 individuals with dose time: 0, 4, 24, 28, 48, and 52 h), c) first two loading doses in range of 24.72–27.78 mg, following the 4 dose in the range of 8.24–9.26 mg (3 individuals with dose times 0, 4, 24, 28, 48, and 52 h). Intensive plasma (141) and blood sampling (149) were performed. Fourteen of 17 cynomolgus macaques with one sample from the lung were collected at 120, 198, 240, 264, and 480 h.
Structural PK Model
Initially, integrated models were established by considering four and five compartment models with and without an absorption lag phase or with and without peripheral compartment (model selection based on the likelihood ratio test) using measurements from the plasma, blood, and lung simultaneously. A model of the plasma, blood, and lung tissue was final developed by fitting a five-compartment model to represent the relationship between the plasma, blood concentrations, and lung tissue. The five-compartment model with first-order oral absorption (Ka, 1/h) and elimination (Ke, 1/h) was evaluation simultaneously to describe the time course of HCQ in the plasma (Figure 1). The parameters estimated in this structural PK model included Ka, volume of distribution for central compartment (Vc, L), two volumes of distribution for two peripheral compartments (Vb for blood and Vl for the lung tissue, respectively). Model parameters were assumed to follow a multivariate log-normal distribution, with stage 1 random error taken to be normally distributed with a proportional plus additive error variance.
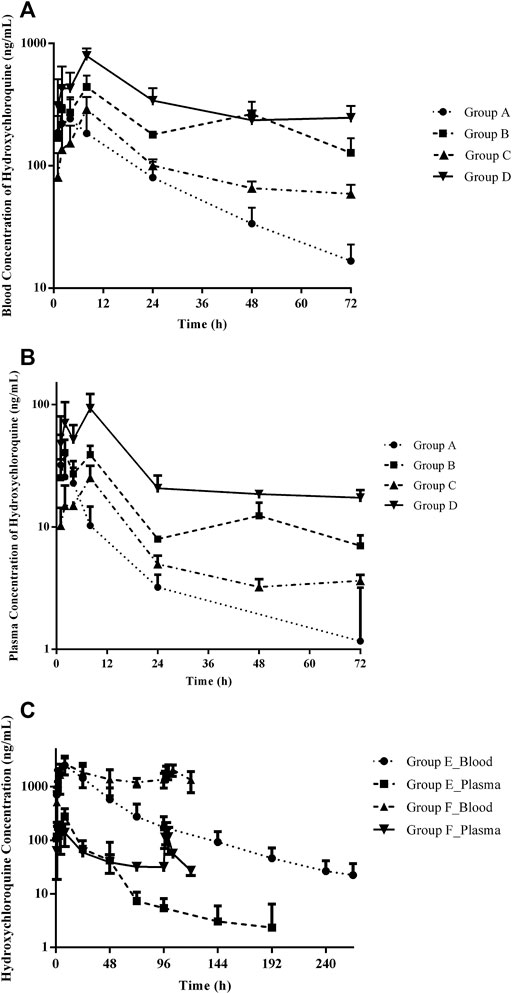
FIGURE 1. Summary of observed HCQ concentrations in the blood and plasma after different treatment regimens. Group A: 6 mg/kg twice (day 1) following 2 mg/kg twice daily (days 2–3); group B: 2 mg/kg twice (day 1) following 1 mg/kg twice daily (day 2–3); group C: 3 mg/kg twice (day 1) following 3 mg/kg twice daily (day 2–3); group D: 3 mg/kg (day 1); group E: 21 mg/kg twice (day 1); group F: 21 mg/kg twice (day 1) following 7 mg/kg twice daily (day 2–5). (A) Blood HCQ concentrations in groups A–D, (B) plasma HCQ concentrations in groups A–D, and (C) HCQ concentration in the blood and plasma, respectively, in groups E and F.
Following Eqs (1)–(8) represent the PK model for HCQ in cynomolgus macaques:
where, A1, A2, A3, A4, and A5 denote the amount (mg) of concentration in the measured plasma compartment (central), absorption compartment, blood compartment, lung tissue compartment, and peripheral compartment, respectively. Kcbmax and Kclmax denote the maximum rate of transfer from the central compartment to the blood and lung, respectively, while Acb50 (mg) and Acl50 (mg) denote the amount of plasma compartments where the rate to blood is half-maximal of Kcbmax and Kclmax, respectively. Kpc (1/h) and Kcp (1/h) denote the rate of transfer between the peripheral compartment and central compartment.
Model Evaluation
Model evaluation was performed using goodness-of-fit (GOF) plots with several diagnostic scatter plots and the comparison of observation and individual concentration time profiles, including (1) observed vs. population-predicted concentration (DV vs. PRED), (2) observed vs. individual-predicted concentration (DV vs. IPRED), (3) conditional standardized residuals vs. time (CSRES vs. TIME), and (4) conditional standardized residuals vs. population-predicted concentration (CSRES vs. PRED).
Pharmacokinetic Profile Simulation
The simulation of the pharmacokinetic profile following multiple HCQ doses was conducted using the typical parameters of the final model. The simulated HCQ concentration in the plasma, blood, and lung tissue was plotted over time.
Software and Platform Used
Population analysis was used to develop an overall pharmacokinetic model for describing the HCQ concentration sampled from each of the four components. Maximum likelihood estimates for model parameters were obtained through the application of the expectation maximization algorithm to the parametric, nonlinear mixed-effects maximum likelihood model, as proposed an developed by Schumitzky (Schumitzky, 1995) and Walker (Walker, 1996)and implemented in ADAPT (version 5, MLEM module) (D'Argenio et al., 2009). Model parameters were assumed to follow a multivariate log-normal distribution, with stage 1 random error taken to be normally distributed with a proportional plus additive error variance. Model simulation was performed by “SIM” module in ADAPT.
Results
Validation of HPLC-MS/MS
HCQ and DHCQ were spiked into blank plasma and various tissue samples, respectively, to determine the calibration curve. The linear responses for HCQ were observed over the corresponding concentration ranges of 5.00-1,000.00 ng/mL, 2.00-200.00 ng/mL, and 0.40-200.00 ng/mL in the blood, plasma, tissue, respectively. While the linear responses for DHCQ were observed over the corresponding concentration ranges of 2.50-500.00 ng/mL, 0.20-200.00 ng/mL, 0.40-200.00 ng/mL in the blood, plasma, tissue, respectively.
All the correlation coefficients were greater than 0.99, indicating a good linearity of the calibration curves. The lowest limit of quantification (LLOQ) of HCQ in blood, plasma, and tissue samples were 5.00 ng/mL, 2.00 ng/mL and 0.40 ng/mL, respectively. While the lowest limit of quantification (LLOQ) of DHCQ in blood, plasma, and tissue samples were 2.50 ng/mL, 0.20 ng/mL, and 0.40 ng/mL, respectively.
HCQ Pharmacokinetics
The developed and validated LC–MS/MS method was successfully applied to the determination of HCQ pharmacokinetics and tissue distribution after intragastric administration of HCQ in monkeys following the dose regimen described in “Section 2.5.” The blood and plasma concentrations vs. time profiles for the HCQ are shown in Figure 1. The maximum concentration (Cmax) of HCQ was 292.33 ± 114.66 ng/mL in blood and 36.90 ± 22.52 ng/mL in plasma. The value of area under curve (AUC0–∞) were determined as 5,978.94 ± 1981.30 and 363.31 ± 195.38 h*ng/mL for blood and plasma, respectively. HCQ were detected in the blood and plasma up to 72 h, which was the last time point monitored. The percentage extrapolation of the AUC from the last measured time point to infinity was less than 10% and 20% in the blood and plasma, respectively.
Biodistribution
Concentrations of HCQ and DHCQ were detected in all studied tissues (Tables 1–3). In addition, our data showed that all the tissues to blood concentration ratio (Kp_tissue/blood) were ≥1, indicating accumulation of HCQ in tissues. The HCQ Kp_tissue/blood ratio for the various tissues was observed in the descending order of thymus > kidney > spleen > liver > heart > subcutaneous fat > brain > lung in group E, whereas the Kp_tissue/blood ratio was different in group F with the descending order of liver > thymus > spleen > kidney > lung > heart > subcutaneous fat > brain. But the last sampling time of group E was 264 h, and the corresponding plasma concentration was below the limit of quantification, resulting the Kp_tissue/plasma was only available in group F. Similar to the Kp_tissue/blood ratio, the descending order of the Kp_tissue/plasma ratio in group F was liver > spleen > kidney > lung > heart > subcutaneous fat > brain. The Kp_tissue/plasma ratio and Kp_tissue/blood ratio for the lung was 267.38 ± 42.93 and 5.55 ± 0.46, respectively.
Model Development and Evaluation
A five-compartment model with first-order oral absorption and elimination was selected as the base model for HCQ concentration in the plasma, blood, and lung tissue (Figure 2). The absorption rate Ka of plasma HCQ was estimated to be 0.592 h−1, and Ke was estimated to be 0.236 h−1. The volume of distribution in the central (Vc/F) and other two peripheral compartments (Vb/F and Vl/F) were 114, 2.68 and 5.55 L, respectively. Estimates of Acb50, Acl50, Kbc, Klc, Kcbmax, Kclmax, Kcp, and Kpc between the central compartment and different peripheral compartments were 7.05 mg, 0.498 mg, 0.718 h−1, 0.159 h−1, 2.48 h−1, 1.92 h−1, 0.600 h−1, and 0.514 h−1, respectively. The interindividual variability (IIV) was estimated for Ka, Ke, Vc/F, Vb/F, Vl/F, Acb50, Acl50, Kbc, Klc, Kcbmax, Kclmax, Kcp, and Kpc. The IIV for PK parameters were expressed as CV% and ranged from 29 to 88.7%, except for IIV of Kcl (160%). The final model parameter estimates are summarized in Table 4.
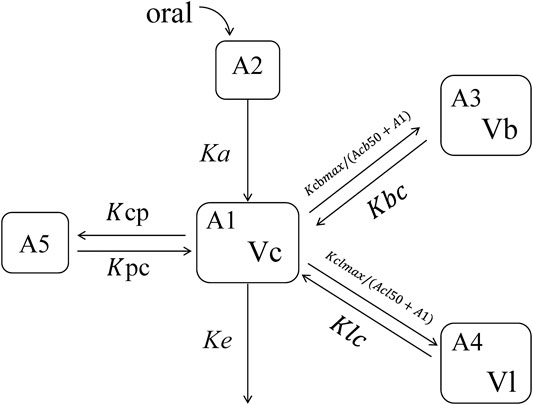
FIGURE 2. Schematic representation of the pharmacokinetic model used to describe HCQ concentration–time profiles after oral administration. A, amounts; Ka, the rate of constant of absorption; Ke, the rate of elimination; Vc, central volume of distribution; Vb, red blood cell volume of distribution; VL, lung volume of distribution; Kcbmax, the maximum drug transport rate from the central compartment to red blood cells; Kclmax, the maximum drug transport rate from the central compartment to the lung tissue; Acb50, the HCQ amount resulting in 50% of maximum transport rate from the central compartment to red blood cells; Kbc, the rate of transport from red blood cells to the central compartment; Acb50, the HCQ amount resulting in 50% of maximum transport rate from the central compartment to the lung tissue; Klc, the rate of transport from the lung tissue to the central compartment; Kpc and Kcp, the rate constant between the peripheral compartment and central compartment.
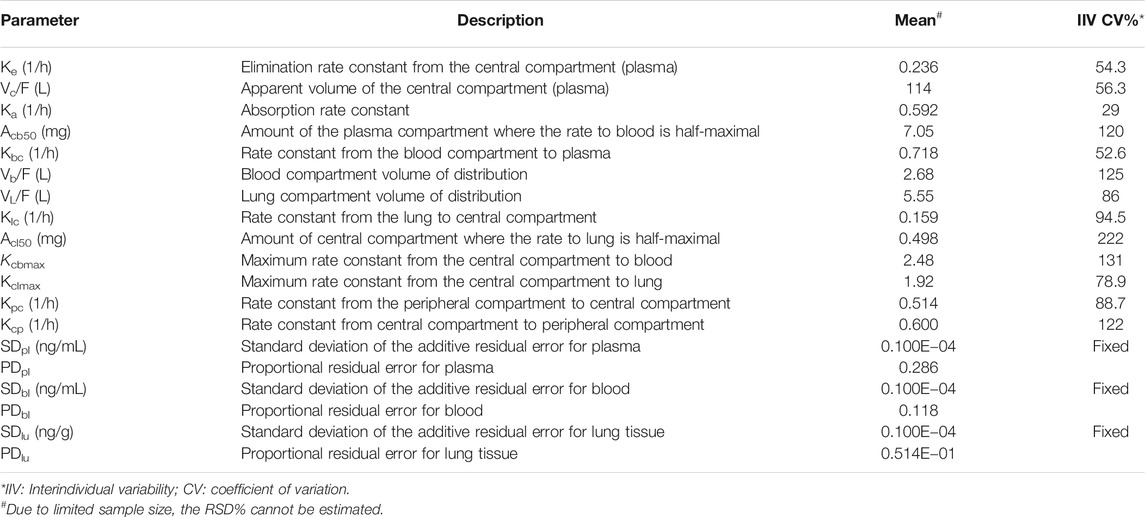
TABLE 4. Model parameter estimation for the final pharmacokinetic model of HCQ in cynomolgus macaques.
GOF plots for the final model were generated, as depicted in Figures 3–5. The population- and individual-predicted plasma, blood, and lung HCQ concentrations vs. observed concentrations showed no major bias. The conditional standardized residuals (CSRES) vs. time or vs. individual-predicted concentrations showed that most of CSRES were within the range of (−2, 2). These model diagnostics suggested that the final population PK model of the monkey to adequately describes the HCQ plasma, blood, and lung data. Figures 6–8 display the individual prediction GOF of HCQ concentration in the plasma, blood, and lung tissue for the final model.
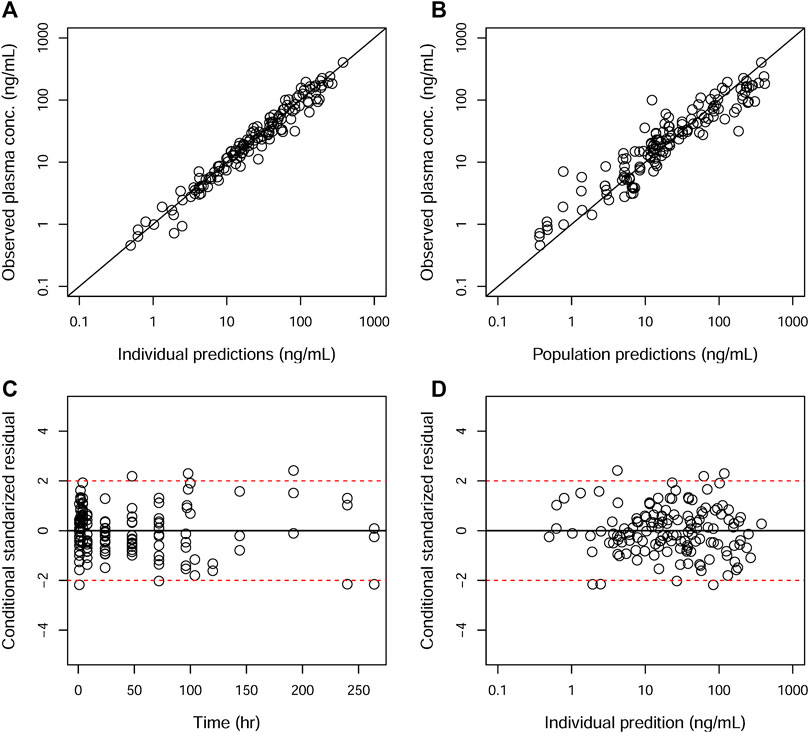
FIGURE 3. Goodness-of-fit plots of the plasma for the final model. The hollow circles represent the observed data from individual monkeys. The black diagonal (A and B) and horizontal lines (C and D) are the lines of identity and zero lines, respectively. The dashed lines in the bottom panel are the conditional standardized residual equal ±2.
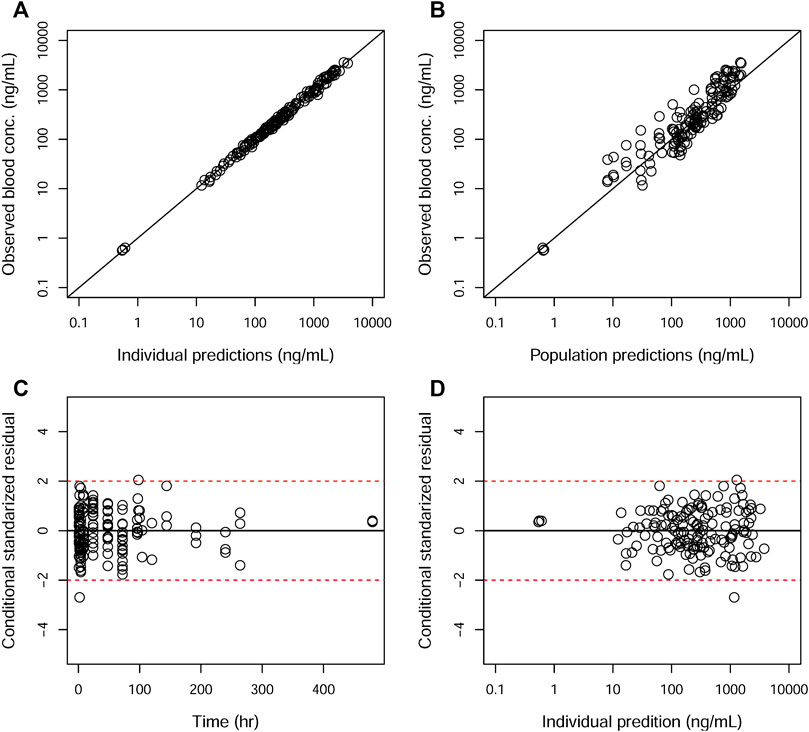
FIGURE 4. Goodness-of-fit plots of the blood for the final model. The hollow circles represent the observed data from individual monkeys. The black diagonal (A and B) and horizontal lines (C and D) are the lines of identity and zero lines, respectively. The dashed lines in the bottom panel are the conditional standardized residual equal ±2.
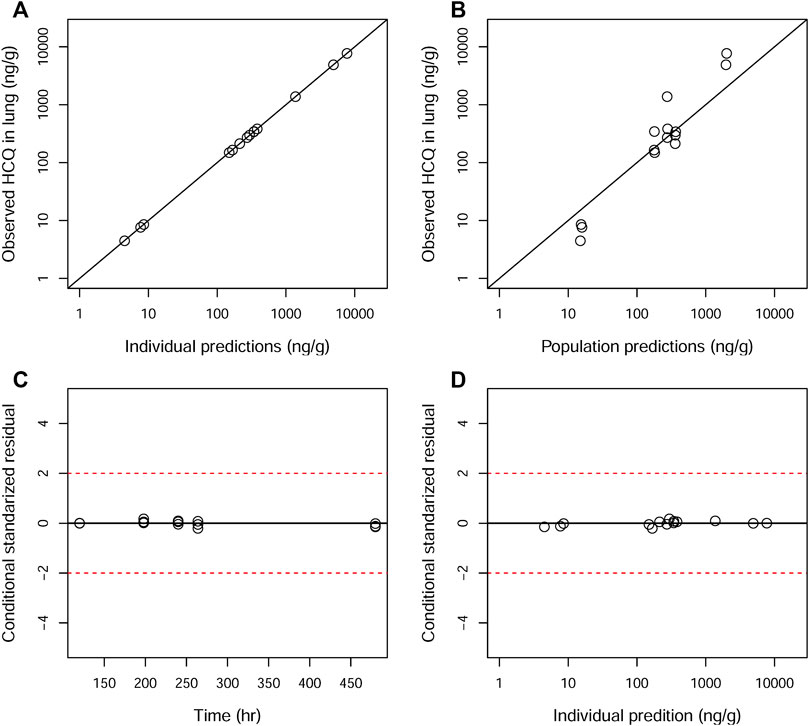
FIGURE 5. Goodness-of-fit plots of the lung for the final model. The hollow circles represent the observed data from individual monkeys. The black diagonal (A and B) and horizontal lines (C and D) are the lines of identity and zero lines, respectively. The dashed lines in the bottom panel are the conditional standardized residual equal ±2.
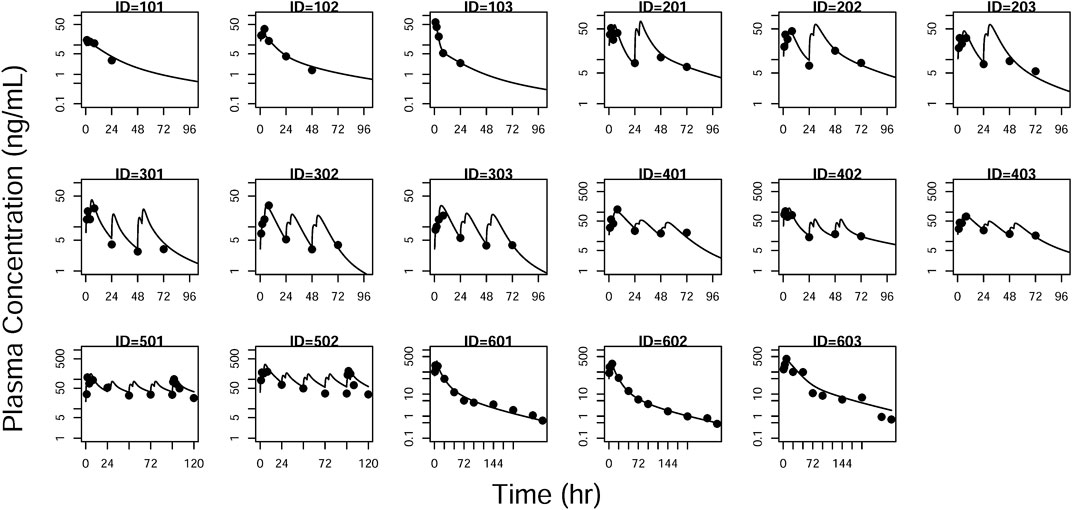
FIGURE 6. Individual prediction plots for plasma concentration. The symbols are the observed data. The lines are the individual fitting lines from final PK model.
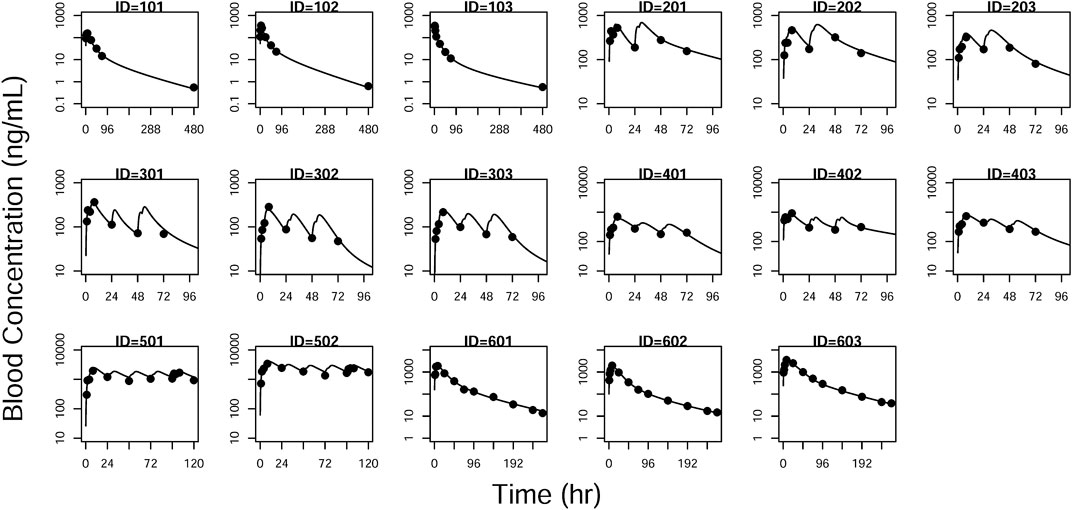
FIGURE 7. Individual prediction plots for blood concentration. The symbols are the observed data. The lines are the individual fitting lines from final PK model.
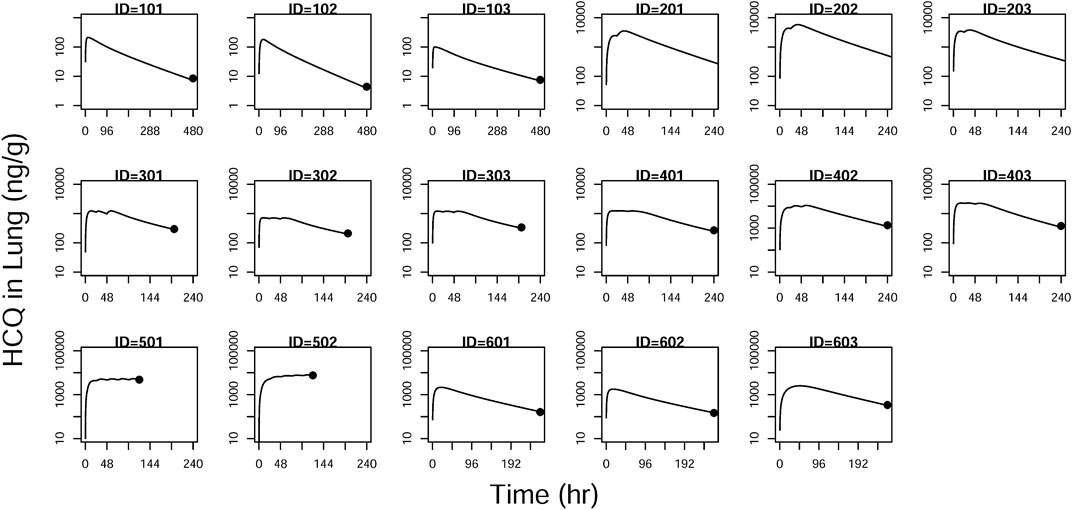
FIGURE 8. Individual prediction plots for concentration in the lung. The symbols are the observed data. The lines are the individual fitting lines from final PK model.
Model Simulation
One dosing regimen of HCQ was simulated using the final population PK model. The simulated HCQ mean concentration in plasma, blood, and lung tissue is shown in Figure 9. And there were also simultaneously simulated the ratios of the lung tissue to plasma in different time points after dosing, as shown in Table 5.
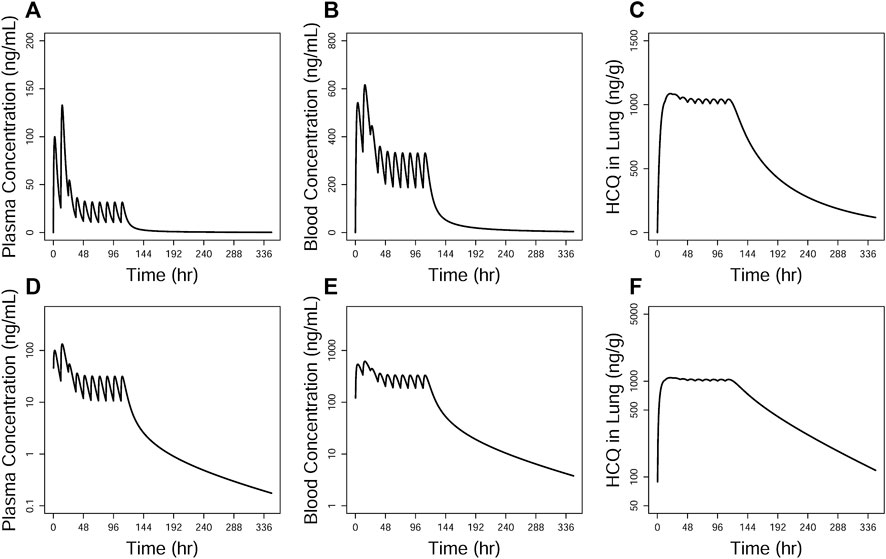
FIGURE 9. Simulations of HCQ pharmacokinetic profiles in the plasma (A and D), blood (B and E), and lung tissue (C and F), respectively. The upper row is constant coordinate plots and the lower row semi-log ones.

TABLE 5. Simulated HCQ concentration data in the plasma, blood and lung, and the ratio of lung/plasma based on the final population PK model.
Discussion
SARS-CoV-2 can attack cells expressing angiotensin I-converting enzyme 2 (ACE2), leading to serious infections of the lungs, the gastrointestinal tract, and other tissues (Hamming et al., 2004). Moreover, antiviral drugs are needed to penetrate the multiple sites where SARS-CoV-2 infection occurs with sufficient concentrations to inhibit viral replication. Global research efforts are focused on screening the activity of existing compounds in vitro to identify candidates to repurpose for SARS-CoV-2. The antimalarial HCQ has demonstrated antiviral activity against SARS–CoV-2 in vitro and in several clinical studies with small sample size. However, the efficacy of HCQ in the treatment of COVID-19 was quite controversial in clinical studies. It appeared that the free plasma concentration of HCQ was lower than extracellular EC50in vitro (submitted data). However, it is quite complicated to extrapolate efficacy from in vitro to humans just based on this simple comparison, as pointed out by Jansson-Löfmark et al. (2020). Moreover, HCQ has specific affinity with pigmented tissues, lysosomes, and golgi (Hoekenga, 1954; Fox, 1993; Schroeder and Gerber, 2014). We later measured intracellular EC50, which could be compared with lung tissue concentration, and there are still many challenges, such as possible differences in mechanism, active metabolites (unpublished data). Although we knew more about its cellular kinetics and its distribution in monkeys, the recommendation of appropriate dosage to treat COVID-19 still needs to consider safety and efficacy margin to allow possible difference between in vivo and in vitro.
Considering that it is impossible to carry out biodistribution research in clinical studies, the additional pharmacokinetics experiments in animals are necessary to understand its exposure in tissues. It is especially helpful when the PBPK modeling strategy was recognized to be supportive but need more validated data, such as lung tissue or lung lining fluid concentrations (Rowland Yeo et al., 2020b; Maharaj et al., 2020). It has been reported the elimination rate of the drug was rapid in mice and its tissue distribution kinetics data are very different from those of human tissue distribution (Chhonker et al., 2018). Thus, it is necessary to perform the distribution kinetic study in monkeys in order to understand the distribution characteristics of HCQ to predict human pharmacokinetics with more confidence. These are essential information for further studies of the pharmacokinetics/pharmacodynamics relationship of HCQ. Higher concentrations in different tissues indicated that further studies are required to investigate the relationship between drug concentration and efficacy, both therapeutic and toxicity, after therapeutic regimens. There is little information regarding tissue distribution of HCQ in monkeys, even less information about metabolite concentrations accumulating in tissues. To evaluate the therapeutic effects on COVID-19 and drug toxicity, it is critical that assays be developed that are sensitive enough to quantitate drug concentrations in tissues, especially the lungs. Moreover, the relationship between metabolite concentration and efficacy or toxicity is unclear. Developing an enhanced understanding of the PKs of HCQ and its metabolites in animal models (including their concentrations in tissue and corresponding kinetics), and their interaction with other therapies is crucial in supporting the clinical efficacy and safety, characterizing and further optimizing combination therapies involving HCQ.
The pharmacokinetics data obtained from different dosing regimens could be used simultaneously to perform population pharmacokinetics model and evaluate the pharmacokinetics characteristic of HCQ. Since the HCQ concentration in the lung tissue is higher than that in the plasma or blood, we could confirm that it might be effective in the lung. In addition, there has been simulated that lung pH reduction from 6.7 to 6 in COVID-19 patients could cause 4.0-fold increases in lung exposure of HCQ, indicating that higher concentrations of HCQ in patients’ lung tissue with COVID-19 were sustained for a longer period after administration (Rowland Yeo et al., 2020a). Many other weakly basic drugs have also been demonstrated to accumulate in lysosomes (Ohkuma and Poole, 1981; Duvvuri and Krise, 2005). However, there are few data about the population pharmacokinetics model to describe the characteristic of these drugs. Furthermore, with the simulation results of multidose regimen, the accumulated concentration in the lung tissue was more than that in the blood or plasma, and the rate of HCQ concentration decrease was faster in the circulation system than that in the lung tissue, indicating that there was a dynamic change course as shown by the ratio of the blood or plasma HCQ concentration to the lung tissue HCQ concentration, which could be used to provide information for HCQ clinical treatment.
Finally, as stereoisomeric drug, HCQ is administered as racemates, whereas enantiomeric differences may be existing in pharmacokinetics (Iredale et al., 1993; Tett, 1993). Therefore, in order to better understand the pharmacokinetics of enantiomers of HCQ on efficacy and safety, it is necessary to design the studies with two isomeric forms of HCQ.
Conclusion
In summary, a population pharmacokinetics model for HCQ in cynomolgus macaques was developed and constructed using data from monkeys. The derived population PK model should be able to evaluate the dynamic process of HCQ in the plasma, blood, and lung tissue concentration to support the clinical applications.
Data Availability Statement
The raw data supporting the conclusions of this article will be made available by the authors, without undue reservation.
Ethics Statement
The animal study was reviewed and approved by Institutional Animal Care and Use Committee(IACUC) Pharmaron Beijing Co., Ltd, Beijing, China.
Author Contributions
DL conceived and designed the study. HL and CS directed this whole study. KW developed and optimized the hydroxychloroquine population PK model. QL and GB designed the dose regimen of hydroxychloroquine and analyzed the experiment data. XG and ST performed population PK model analysis. XT, MX, and JW carried out the animal experiments. GC, ML, BW, and HJ conducted the bioanalysis of hydroxychloroquine. QL, GB, and DL prepared this manuscript.
Funding
This work was supported by the Bill & Melinda Gates Foundation (INV-015694), the Ministry of Science and Technology of the People’s Republic of China (Grant No. 2020YFC0844500), and the National Natural Science Foundation of China (Project Nos. 81672133 and 81874010).
Conflict of Interest
Authors GC, ML, BW, and HJ were employed by the company HR-Biopharm Technology Co., Ltd. Authors XT, MX, and JW were employed by the company Pharmaron Beijing Co., Ltd. Author KW was employed by the company Shanghai Qiangshi Information Technology Co., Ltd.
The remaining authors declare that the research was conducted in the absence of any commercial or financial relationships that could be construed as a potential conflict of interest.
Acknowledgments
We want to thank Ping Zhao from the Bill & Melinda Gates Foundation for the proofreading work.
References
Chhonker, Y. S., Sleightholm, R. L., Li, J., Oupický, D., and Murry, D. J. (2018). Simultaneous quantitation of hydroxychloroquine and its metabolites in mouse blood and tissues using LC-ESI-MS/MS: an application for pharmacokinetic studies. J. Chromatogr. B Analyt. Technol. Biomed. Life Sci 1072, 320–327. doi:10.1016/j.jchromb.2017.11.026
D'Argenio, D., Schumitzky, A., and Wang, X. (2009). ADAPT 5 user's guide: pharmacokinetic/pharmacodynamic systems analysis software. Los Angeles: Biomedical Simulations Resource.
Duvvuri, M., and Krise, J. P. (2005). A novel assay reveals that weakly basic model compounds concentrate in lysosomes to an extent greater than pH-partitioning theory would predict. Mol. Pharm 2(6), 440–448. doi:10.1021/mp050043s
Fox, R. I. (1993). Mechanism of action of hydroxychloroquine as an antirheumatic drug. Semin. Arthritis Rheum 23(2 Suppl. 1), 82–91. doi:10.1016/s0049-0172(10)80012-5
Gao, J., Tian, Z., and Yang, X. (2020). Breakthrough: chloroquine phosphate has shown apparent efficacy in treatment of COVID-19 associated pneumonia in clinical studies. Biosci. Trends 14(1), 72–73. doi:10.5582/bst.2020.01047
Gautret, P., Lagier, J. C., Parola, P., Hoang, V. T., Meddeb, L., Mailhe, M., et al. (2020). Hydroxychloroquine and azithromycin as a treatment of COVID-19: results of an open-label non-randomized clinical trial. Int. J. Antimicrob. Agents 8, 105949. doi:10.1016/j.ijantimicag.2020.105949
Hamming, I., Timens, W., Bulthuis, M. L., Lely, A. T., Navis, G., and van Goor, H. (2004). Tissue distribution of ACE2 protein, the functional receptor for SARS coronavirus. A first step in understanding SARS pathogenesis. J. Pathol 203(2), 631–637. doi:10.1002/path.1570
Hoekenga, M. T. (1954). The treatment of acute malaria with single oral doses of amodiaquin, chloroquine, hydroxychloroquine and pyrimethamine. Am. J. Trop. Med. Hyg 3(5), 833–838. doi:10.4269/ajtmh.1954.3.833
Iredale, J., Fieger, H., and Wainer, I. W. (1993). Determination of the stereoisomers of hydroxychloroquine and its major metabolites in plasma and urine following a single oral administration of racemic hydroxychloroquine. Semin. Arthritis Rheum 23(2 Suppl. 1), 74–81. doi:10.1016/s0049-0172(10)80011-3
Jansson-Löfmark, R., Hjorth, S., and Gabrielsson, J. (2020). Does in vitro potency predict clinically efficacious concentrations? Clin. Pharmacol. Ther 108(2), 298–305. doi:10.1002/cpt.1846
Liu, J., Cao, R., Xu, M., Wang, X., Zhang, H., Hu, H., et al. (2020). Hydroxychloroquine, a less toxic derivative of chloroquine, is effective in inhibiting SARS-CoV-2 infection in vitro. Cell Discov 6, 16. doi:10.1038/s41421-020-0156-0
Maharaj, A. R., Wu, H., Hornik, C. P., Balevic, S. J., Hornik, C. D., Smith, P. B., et al. (2020). Simulated assessment of pharmacokinetically guided dosing for investigational treatments of pediatric patients with coronavirus disease 2019. JAMA Pediatr, e202422. doi:10.1001/jamapediatrics.2020.2422
Mehra, M. R., Desai, S. S., Ruschitzka, F., and Patel, A. N. (2020). Hydroxychloroquine or chloroquine with or without a macrolide for treatment of COVID-19: a multinational registry analysis. Lancet 12, 49. doi:10.1016/s0140-6736(20)31180-6
Molina, J. M., Delaugerre, C., Le Goff, J., Mela-Lima, B., Ponscarme, D., Goldwirt, L., et al. (2020). No evidence of rapid antiviral clearance or clinical benefit with the combination of hydroxychloroquine and azithromycin in patients with severe COVID-19 infection. Med. Maladies Infect 50(4), 384. doi:10.1016/j.medmal.2020.03.006
Ohkuma, S., and Poole, B. (1981). Cytoplasmic vacuolation of mouse peritoneal macrophages and the uptake into lysosomes of weakly basic substances. J. Cell Biol 90(3), 656–664. doi:10.1083/jcb.90.3.656
Rowland Yeo, K., Zhang, M., Pan, X., Ban Ke, A., Jones, H. M., Wesche, D., et al. (2020a). Impact of disease on plasma and lung exposure of chloroquine, hydroxy-chloroquine and azithromycin: application of PBPK modelling. Clin. Pharmacol. Ther 14, 23–29. doi:10.1002/cpt.1955
Rowland Yeo, K., Zhang, M., Pan, X., Ban Ke, A., Jones, H. M., Wesche, D., et al. (2020b). Impact of disease on plasma and lung exposure of chloroquine, hydroxychloroquine and azithromycin: application of PBPK modeling. Clin. Pharmacol. Ther 44, 149–153. doi:10.1002/cpt.1955
Schroeder, R. L., and Gerber, J. P. (2014). Chloroquine and hydroxychloroquine binding to melanin: some possible consequences for pathologies. Toxicol. Rep 1, 963–968. doi:10.1016/j.toxrep.2014.10.019
Schumitzky, A. (1995). “EM Algorithms and two stage methods in pharmacokinetic population analysis,” in Advanced methods of pharmacokinetic and pharmacodynamic systems analysis, 2 Editor: D. Z. D'Argenio (New York: Plenum Press) 60.
Tett, S. E. (1993). Clinical pharmacokinetics of slow-acting antirheumatic drugs. Clin. Pharmacokinet 25(5), 392–407. doi:10.2165/00003088-199325050-00005
Walker, S. (1996). An EM algorithm for nonlinear random effects models. Biometrics 52(3), 934–944. doi:10.2307/2533054
Wang, M., Cao, R., Zhang, L., Yang, X., Liu, J., Xu, M., et al. (2020). Remdesivir and chloroquine effectively inhibit the recently emerged novel coronavirus (2019-nCoV) in vitro. Cell Res 30(3), 269–271. doi:10.1038/s41422-020-0282-0
WHO Situation Report. (2019). WHO Situation Report—209. Available at: https://www.who.int/emergencies/diseases/novel-coronavirus-2019/situation-reports.
Xu, C., Zhu, L., Chan, T., Lu, X., Shen, W., Madigan, M. C., et al. (2016). Chloroquine and hydroxychloroquine are novel inhibitors of human organic anion transporting polypeptide 1A2. J. Pharmacol. Sci 105(2), 884–890. doi:10.1002/jps.24663
Keywords: hydroxychloroquine, biodistribution, population pharmacokinetics, cynomolgus macaques, time-dependent
Citation: Liu Q, Bi G, Chen G, Guo X, Tu S, Tong X, Xu M, Liu M, Wang B, Jiang H, Wang J, Li H, Wang K, Liu D and Song C (2021) Time-Dependent Distribution of Hydroxychloroquine in Cynomolgus Macaques Using Population Pharmacokinetic Modeling Method. Front. Pharmacol. 11:602880. doi: 10.3389/fphar.2020.602880
Received: 04 September 2020; Accepted: 16 November 2020;
Published: 14 January 2021.
Edited by:
Sabina Passamonti, University of Trieste, ItalyReviewed by:
Snehal Samant, University of Florida, United StatesConstantin Mircioiu, Carol Davila University of Medicine and Pharmacy, Romania
Copyright © 2021 Liu, Bi, Chen, Guo, Tu, Tong, Xu, Liu, Wang, Jiang, Wang, Li, Wang, Liu and Song. This is an open-access article distributed under the terms of the Creative Commons Attribution License (CC BY). The use, distribution or reproduction in other forums is permitted, provided the original author(s) and the copyright owner(s) are credited and that the original publication in this journal is cited, in accordance with accepted academic practice. No use, distribution or reproduction is permitted which does not comply with these terms.
*Correspondence: Kun Wang, S3VuLndhbmdAc2hjc2NjLmNvbQ==; Dongyang Liu, bGl1ZG9uZ3lhbmdAdmlwLnNpbmEuY29t; Chunli Song, c2NobEBiam11LmVkdS5jbg==
†These authors share first authorship