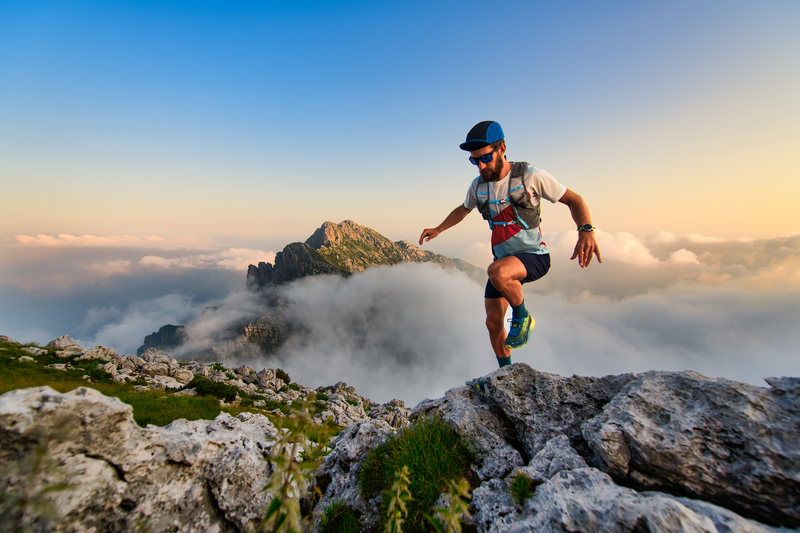
95% of researchers rate our articles as excellent or good
Learn more about the work of our research integrity team to safeguard the quality of each article we publish.
Find out more
REVIEW article
Front. Pharmacol. , 09 November 2020
Sec. Translational Pharmacology
Volume 11 - 2020 | https://doi.org/10.3389/fphar.2020.588480
This article is part of the Research Topic Novel Diagnostic and Therapeutic Innovations in Dental Care View all 5 articles
Periodontitis is a complex multifactorial disease that can lead to destruction of tooth supporting tissues and subsequent tooth loss. The most recent global burden of disease studies highlight that severe periodontitis is one of the most prevalent chronic inflammatory conditions affecting humans. Periodontitis risk is attributed to genetics, host-microbiome and environmental factors. Empirical diagnostic and prognostic systems have yet to be validated in the field of periodontics. Early diagnosis and intervention prevents periodontitis progression in most patients. Increased susceptibility and suboptimal control of modifiable risk factors can result in poor response to therapy, and relapse. The chronic immune-inflammatory response to microbial biofilms at the tooth or dental implant surface is associated with systemic conditions such as cardiovascular disease, diabetes or gastrointestinal diseases. Oral fluid-based biomarkers have demonstrated easy accessibility and potential as diagnostics for oral and systemic diseases, including the identification of SARS-CoV-2 in saliva. Advances in biotechnology have led to innovations in lab-on-a-chip and biosensors to interface with oral-based biomarker assessment. This review highlights new developments in oral biomarker discovery and their validation for clinical application to advance precision oral medicine through improved diagnosis, prognosis and patient stratification. Their potential to improve clinical outcomes of periodontitis and associated chronic conditions will benefit the dental and overall public health.
Periodontitis is one of the most prevalent chronic inflammatory diseases (Kassebaum et al., 2014). An estimated 42% of United States adults have been diagnosed with periodontitis with around 8% being severely affected (Eke et al., 2018). The clinical manifestation includes the loss of supporting tooth structures such as alveolar bone, soft tissue and the periodontal ligament (PDL). The pathogenesis is triggered by an immunoinflammatory response of the host to a bacterial challenge (Kornman et al., 2017). Gingivitis is a reversible periodontal disease that manifests clinically as gingival inflammation without loss of bone or PDL. In susceptible individuals, gingivitis can lead to periodontitis when untreated. Gingivitis always precedes but not always leads to periodontitis. The transition from a controlled inflammatory state to tissue destructive inflammation requires both a dysbiotic oral microbiome and a susceptible host (Hajishengallis et al., 2012; Hajishengallis, 2014). Besides the infectious nature of opportunistic pathogenic bacteria and their byproducts, host genetics governing the immune-inflammatory response as well as behavioral and environmental risk factors play a critical role in the initiation and progression of periodontal diseases (Page and Kornman, 2000). Therefore, the likelihood and rate of progression relies on multiple patient-related factors and cannot be generalized (Tonetti et al., 2018). Studies have shown that independent of external components, disease progression in different populations occurs in dynamic orders with similar rates (Loe et al., 1978; Teles et al., 2016). Periodontitis is an oscillating condition with periods of disease progression, inactivity, and/or regression (Goodson et al., 1982; Teles et al., 2018).
Notable with chronic diseases, early diagnosis is key to long-term treatment success. Classification criteria in the periodontal field have recently been updated (Papapanou et al., 2018) and include the categorization regarding the severity and extent of pathological destruction, as well as the determination of progression risk in the response to conventional therapy. The criteria that define periodontitis are mostly surrogate endpoints that measure localized tissue destruction. This only allows the quantification of past destruction and hence, limitations on prediction of future disease activity. Additionally, physical measurements are subject to error and variability between clinicians (Goodson et al., 1984). In accordance to the dynamic nature of periodontitis, the practical prognostic systems are fairly arbitrary (McGuire and Nunn, 1996; Kwok and Caton, 2007).
As the specialty of periodontics evolved over the past centuries, endosseous implants entered as reasonable alternatives in clinical reality. However, novel approaches come with de novo challenges. Periimplantitis is a pathological condition occurring in tissue around dental implants (Schwarz et al., 2018). Similar to periodontitis, periimplantitis results in inflammation of the surrounding structures and subsequent peri-implant tissue destruction.
Whether we are evaluating either teeth or implants, clinicians still find themselves challenged to determine which host factors are actually destructive, and which may be protective. The most desirable goal in health care delivery is to accurately monitor disease onset, progression and treatment outcomes.
The human microbiota includes thousands of diverse species with about 700 microbial genus members (MacGregor et al., 2005; Arweiler and Netuschil, 2016). The oral ecology with its boundless diversity (of oral microbiota) emphasizes the persistent symbiosis of microorganisms in the state of health. Within the oral cavity, hard and soft tissue surfaces are accessible to the cross-linking interaction of microorganisms and permeability of pathogenic etiologies. Disturbance of this equilibrium leads to the most prevalent oral diseases globally which are dental caries, periodontal disease and cancers of the lips and oral cavity (Frencken et al., 2017; Peres et al., 2019). Commonalities of these prevalent diseases are the patient profile and lifestyle habits (e.g., smoking, diet, stress, etc.). Poor oral health and infrequent dental visits have been shown to place patients at higher risk for developing head and neck cancers (Ahrens et al., 2014). Periodontal disease increases the risk of premalignant mucocutaneous diseases such as oral leukoplakia by up to five fold (Meisel et al., 2012). Contrastingly, loss of periodontal tissues may be of diagnostic relevance, as progression due to exacerbation of mucocutaneous diseases that can impair oral hygiene care by patients (Jimenez et al., 2002). Oro-mucosal diseases are associated with painful erosions and ulceration of the soft tissues lining the oral cavity. In order to avoid further discomfort, home care is hindered resulting in the accumulation of bacterial plaque biofilms and eventually in the loss of the mucosal tissues including those tooth-supporting periodontal tissues (Rai et al., 2016). Studies have confirmed the significance of periodontal pathogens and tooth loss as diagnostic markers for oral and head-and-neck cancers. (Mager et al., 2005; Zeng et al., 2013b).
In the chronic condition of periodontitis, the ramifications of long-standing inflammation can impact the oral microflora and leading to microbial dysbiosis. Like in periodontitis, the primary etiology of carious lesions is a microbiological infection combined with additional multifactorial environmental drivers (Kornman et al., 2000; Pitts et al., 2017). Yet, the clinical manifestations and patient symptoms of these two diseases differ significantly. Progression of carious lesions stimulates nerves within hard tooth structures, with a possible influence on the pulpal status–that can elicit pain. On the other hand, periodontitis tends to have a more silent onset with slower rate of tissue destruction and hence often more difficult to diagnose early and predict progression.
Today’s appreciation of periodontology is manifold, characterized by a relationship with systemic health. Evidence supporting the link between periodontal disease and systemic conditions is accumulating in the literature (Papageorgiou et al., 2017; Heikkila et al., 2018; Romandini et al., 2018; Sanz et al., 2018; Sen et al., 2018) (Table 1). This relationship is mainly attributed to the constant inflammatory state driven by the presence of virulent oral pathogens. Studies confirmed elevated levels of inflammatory markers in patients with periodontitis when compared to healthy controls (Buhlin et al., 2009). This chronic low-grade inflammation gradually and silently contributes to the aggregate systemic inflammatory burden. Chronic inflammation has been known for its close association with adverse cardiovascular events (Ridker and Silvertown, 2008) as well as the formation of thrombi (Mukhopadhyay et al., 2019).
TABLE 1. Key Publications on associations of periodontal disease and systemic conditions based on meta-analyses.
Additionally, periodontitis has been associated as a potential risk for increased morbidity for systemic conditions including diabetes mellitus, rheumatoid arthritis, obesity, osteoporosis and adverse pregnancy events (Bartold and Lopez-Oliva, 2000; Papapanou, 2015; Penoni et al., 2017; Sanz et al., 2018; Kang et al., 2019). The bidirectional relationship between diabetes mellitus and periodontitis has been extensively investigated and demonstrates that control of one disease positively impacts the other (Sima and Glogauer, 2013). A recent preclinical study reported the potential effect of periodontal inflammation on the pathogenesis of Irritable Bowel disease. The translocation of oral pathogens during an oral inflammatory state to the intestines may lead to the disruption of the colonization resistance of gut-resident microbiota (Kitamoto et al., 2020).
Although periodontitis primarily involves local destruction of tissues in the oral cavity, its management requires a whole-body, long-term strategy tailored to each individual’s profile. The comorbidity between periodontitis and systemic diseases raises the importance of reducing the cumulative inflammatory burden by pursuing early diagnosis and treatment from a dental point of view (Slots, 2000).
A biomarker is a defined characteristic that is measured as an indicator of normal biological processes, pathogenic processes or response to an exposure or intervention (Cagney et al., 2018). There is abundant evidence demonstrating that certain inflammatory biomarkers are elevated for years prior to resulting into clinically significant consequences (Ridker and Silvertown, 2008). These same biomarkers are highly predictive for disease onset when assessed timely and accurately. Oral fluids offer a non-invasive opportunity to weigh risks, predict disease initiation, refine diagnosis and stratify treatment modalities (Giannobile, 2012; Zhang et al., 2016).
Risk prediction models are generated using microbiological elements, salivary protein biomarkers as well as on a genetic/epigenetic information (Figure 1). The media to obtain these indicators can be derived from human saliva or gingival crevicular fluid (GCF). Numerous studies were carried to analyze the relationships between saliva composition and oral diseases including periodontitis (Brinkmann et al., 2011; Giannobile, 2012; Salminen et al., 2014; Kc et al., 2020), carious lesions (Teng et al., 2015), and oral cancer (Wang et al., 2015; Zhang et al., 2016).
Dating back to 2008, the term “Salivaomics” was introduced to facilitate the research focused on disease diagnosis and monitoring (Wong, 2012). Saliva essentially originates from blood serum and is filtrated by the salivary glands, effectively representing the body’s circulating health or disease markers (Spielmann and Wong, 2011). Saliva is a rich source of proteins related to oral conditions and systemic health. Saliva possesses multiple biological functions such as antibacterial, antiviral, antifungal, wound healing, buffering, tooth mineralization, food digestion, and coating (Vila et al., 2019). In addition to these functions, collection of salivary samples is easy and a non-invasive procedure.
GCF is essentially a serum exudate that accumulates in the gingival sulcus or pocket that is generally rich in biological markers. This site-specific fluid is easily obtainable and generally predictive of periodontal pathogenesis (Zhang et al., 2016). Over the past 2 decades studies have analyzed biomarkers in the GCF and their predictive ability in the periodontitis identification and progression.
Since periodontitis is a chronic inflammatory disease associated with microbial dysbiosis and host-driven tissue destruction (Kornman, 2008; Darveau, 2010), it is recognized that a single oral pathogen would not be sufficient to induce the most common forms of periodontitis. However, historically there were several studies focused on small subgroups of patients with rapidly progressing bone destruction occurring around certain teeth (molars and incisors), called localized aggressive periodontitis, which was found to associate with a specific bacterium, named Aggregatibacter actimomycetemcomitans (Aa). This specific pathogen was once used as a strong diagnostic to monitor treatment outcome (Socransky and Haffajee, 1992) as there were clinical studies showing that sites with residual infection of Aa resulted in further clinical attachment loss around teeth (Christersson et al., 1985; Fine et al., 2013). Systemic antibiotic therapy (tetracycline or metronidazole) was indicated to target this pathogen (Slots and Rosling, 1983; Kornman and Robertson, 1985). More recently, the focus has shifted to investigate a separate group of highly virulent bacteria that were identified under the deep periodontal pockets in patients with chronic periodontitis in the general population. They are the “red complex bacteria”(Socransky et al., 1998) or the keystone pathogens (Hajishengallis et al., 2012).
Those red complex and keystone pathogens have been implicated in many studies that showed their association and predictive value for periodontitis. In a 12-months longitudinal study using subgingival plaque with real-time PCR to predict periodontitis progression, red complex pathogens [including Porphyromonas gingivalis (Pg), Tannerella forsythensis (Tf) and Treponema denticola (Td) in conjunction with E. corrodens (Er), F. nucleatum (Fn) and P. intermedia (Pi)] equally contributed to strong sensitivity and specificity values (Kinney et al., 2014). Additionally, P. gingivalis and T. denticola in saliva, together with elevated levels of MMP-8 can also be a strong indicator for severe periodontitis (Gursoy et al., 2018; Nascimento et al., 2019; Sorsa et al., 2020). However, at the initial periodontitis lesion, the red complex cannot accurately predict a robust inflammatory response for gingivitis. In a human experimental gingivitis study utilizing checkboard DNA-DNA hybridization, it was found that the orange complex, especially Fusobacterium nucleatum (Fn), predisposes patients to a more pronounced inflammation (Lee et al., 2012). F. nucleatum is an early colonizer that bridges the pathogens and thus identified as an emerging opportunistic pathogen that plays a critical role in the dysbiosis of microbiome (Hajishengallis, 2015). However, metatranscriptomic analyses of the human oral microbiome found that F. nucleatum upregulates all genes involved inL-lysine metabolism to short chain fatty acid butyrate, which can establish a favorable growth environment for disease-associated communities but is also a beneficial regulator of host-microbiome balance in the gut, and potentially in the oral cavity (Chang et al., 2014; Jorth et al., 2014; Schulthess et al., 2019). The concept of dysbiosis and the recognition of host-microbe interaction mandates the field to not only consider specific oral pathogens but also several host biomarkers including receptors for microbiome metabolites, lipid mediators of inflammation, chemokines, cytokines, enzymes and other proteins. Combining clinical measures, pathogen levels, and cytokines like interleukin (IL)-1β, osteoprotegerin (OPG) and matrix metalloproteinases (MMP)-8 can provide up to 74% sensitivity for predicting periodontitis progression (Kinney et al., 2014).
With the growing use of dental implants, particularly in patients with tooth loss due to periodontitis, peri-implantitis and its increasing prevalence has gained tremendous attention to elucidate the pathogenesis and develop predictable therapeutic approaches. T. denticola was found associated with peri-implantitis, and combined with the levels of IL-1β, vascular endothelial growth (VEGF) and tissue inhibitors of metalloproteinases (TIMP)-2 in the peri-implant crevicular fluid (PICF), it enhances the diagnostic accuracy for disease activity (Wang et al., 2016). In a 6 months, prospective interventional clinical trial, lower post-treatment pathogen levels in a panel of oral bacteria, together with >30% reduction of IL-1β, VEGF, and IL-6 in PICF had a 77% sensitivity to predict a favorable treatment outcome (Renvert et al., 2017). More recently, using next generation sequencing technologies, previously unculturable potential pathogens were identified in periodontitis and peri-implantitis lesions, and the level of dysbiosis highly correlated with disease severity (Kroger et al., 2018; Shi et al., 2018).
The methodological advancements in high-throughput sequencing and bioinformatics have provided unprecedented resolution in profiling the oral microbiome and host-microbiome interfaces. Using molecular methods, we have significantly expanded the number of bacterial species (more than two hundred) identified in oral microbiome. However, the field is calling for functional investigation of the bacteria within the biofilm microenvironment and of the associated host oral immune functions to elucidate the ultimate impact of host-microbe interaction on dysbiosis and tissue destruction (Wade, 2011; Krishnan et al., 2017). For example, patients with localized aggressive periodontitis may have selective impaired phagocyte functions (especially for F. nucleatum) resulting in overgrowth of opportunistic pathogens relative to commensal bacteria, which represents a potential mechanism for “host-mediated dysbiosis” of the microbiome (Wang et al., 2015). Ultimately, understanding the host-microbe interactions and the influence of dysbiosis in each individual holds the key for future diagnostics and therapeutics.
Precision medicine demands a systems wide approach to integrate multi-level data in search for accurate and reliable biomarkers of disease activity, and novel therapeutic targets to improve the management of chronic conditions in all fields of medicine (Giannobile et al., 2013). Protein biomarkers pave the way to individualized prevention, diagnosis and treatment. When considering the periodontal pathogenic processes, periodontitis can be generally divided into three phases: inflammation, connective tissue degradation, and bone resorption. During each phase of the disease activity, specific biomarkers have been identified to provide a general sense of what stage of pathologic breakdown the patient is currently residing (Korte and Kinney, 2016) (Table 2). Numerous studies were carried out to analyze the relationships between oral diseases and saliva composition. The most recent systematic review reported macrophage inflammatory protein-1 alpha, IL-1β, IL-6, and MMP-8 as promising diagnostic biomarkers (Kc et al., 2020).
TABLE 2. Oral-based biomarkers with major effector functions associated with oral and systemic diseases.
The pro-inflammatory cytokines IL-1β and tumor necrosis factor alpha (TNF)-α were frontrunner targets in the quest for molecular biomarkers of periodontitis activity (Page and Kornman, 2000). Decreases in IL-1β and TNF-α levels were found to reduce periodontitis progression (Delima et al., 2001). Further, the relationship between inflammatory cell infiltrates and tissue degradation is centered on the production of MMPs: collagenases, gelatinases and the stromelysins. They are synthesized by resident gingival and innate immune cells including epithelial cells, fibroblasts, neutrophils and macrophages. TIMPS control the local extracellular MMP activity in tissue remodeling, allowing a constant balance of reparative and destructive phases in soft tissue matrices (Meikle et al., 1994; Reynolds and Meikle, 2000). In the same sense, inflammatory osteolysis and bone remodeling are regulated by several bone metabolites and immune mediators (Giannobile, 1999; McCauley and Nohutcu, 2002; Kinney et al., 2007). Receptor-activator of NF-κβ ligand (RANKL), a member the TNF-superfamily, is an essential pro-osteoclast factor, which, together with its decoy receptor OPG, is critical for bone resorption-formation coupling. RANKL binds to RANK, which leads to the activation and differentiation of osteoclasts with subsequent bone destruction. In active periodontitis, levels of RANKL are increased and levels of OPG are decreased, therefore RANKL/OPG ratio may serve as a biomarker for periodontitis.
Recent studies have shown that site specific GCF biomarkers can be effective measures of periodontal treatment efficacy [e.g., hypoxia induced factor-1 alpha (HIF-1α), VEGF and TNF-α levels] and hence their ability to surveil therapeutic modalities (Afacan et al., 2020). When evaluating the sensitivity of GCF-based tests, longitudinal studies found that addition of biofilm-associated pathogens may have higher predictive value for periodontitis progression (Kinney et al., 2014).
Page and Kornman suggested that the pathological mechanism of periodontitis, is centered around the host’s responses against microbial challenges, a paradigm that continues to this day (Hajishengallis and Korostoff, 2000; Page and Kornman, 2000). Environmental and genetic risk factors are mediators of the host immune-inflammatory response, and connective tissue and bone metabolism. The susceptibility to periodontitis is fundamentally polygenic in nature and regulated through genes induced by environment interactions (Schaefer, 2000). The heritability of periodontitis has been investigated in familial and twin studies, estimating to be around 30–50% after being adjusted for covariates (Michalowicz et al., 2000; Nibali et al., 2019).
The human genome sequencing and subsequent functional investigations advanced our understanding of genetic regulation, gene by environment interactions and their roles in complex chronic conditions. The current emerging paradigm proposes that specific disease phenotypes can be attributed to the effects of combinations of various genetic risk alleles and their interaction with internal and external factors (Schaefer, 2000). Severe periodontal genotypes may carry susceptibility alleles more frequently. IL-1 is a potent pro-inflammatory cytokine and is involved in the pathogenesis of many human chronic conditions including cardiovascular, metabolic, autoimmune and others (Sims and Smith, 2010). For periodontitis, the IL-1 genotype has a regulatory effect on the local inflammatory processes (Kornman et al., 1997; Offenbacher et al., 2018). IL-1A allele 2 and IL-1B+3953 allele 2 genotypes were recognized as strong indicators for severe periodontitis in an adult populations (Kornman et al., 1997). Carriers of these alleles produce more IL-1 by blood mono-and polymorphonuclear cells, and have a higher IL-1 prevalence in GCF (Rogus et al., 2008; Latella et al., 2009). IL-1 genotypes have been consistently associated with periodontitis within diverse ethnic groups. A high frequency of the IL-1b allele 1 was observed in localized juvenile periodontitis patients in an African-American population (Walker et al., 2000).
Longitudinal studies of IL-1 gene polymorphisms (Cullinan et al., 2001) and the genome-wide association studies (GWAS) shed light on the IL-1 role as a critical risk factor for periodontitis and determinant of the pro-inflammatory host response. These findings support patient stratification based on the IL-1 genotype for tailored measures to prevent periodontitis progression and associated tooth loss (Giannobile et al., 2013).
Epigenetics implies DNA modification that does not change the DNA sequence, but rather influences gene expression and the consequent disease activity (Schmidl et al., 2018). Epigenetic alterations are known to affect transcription and translation of genes through DNA methylation, post-translational modification of histones and/or non-coding RNA (Barros et al., 2000; Larsson et al., 2015). Several studies have found hypomethylation profiles within the interferon (IFN)-γ, IL-6, and TNF-α promoters, which was associated with increased IFN-γ, IL-6, and TNF-α transcription in gingival biopsies from patients with periodontitis (Zhang et al., 2010; Kobayashi et al., 2016). Furthermore, a hypermethylation pattern of the prostaglandin-endoperoxide synthase 2 (PTGS2) promoter was found associated with reduced PTGS2 transcription, consistent with a dampening of cyclooxygenase- expression that had been reported in gingival samples collected from sites with periodontitis (Zhang et al., 2010). One study found that microbial challenges induced changes in DNA methylation affecting the barrier function of oral epithelial cells (Barros et al., 2020).
The impact of risk variants and their interaction on modifying disease susceptibility is currently being investigated (Freitag-Wolf et al., 2019). Complex diseases like periodontitis are significantly influenced through genes by environment interactions. McGuire et al. reported that the IL-1 genotype-positive heavy smoker patients had significantly worse tooth survival rates (McGuire and Nunn, 1999). Parkhill reported that IL-1β, IL-1-Receptor Antagonist and IL-1β genotype combined with smoking in Caucasians are risk factors for early onset periodontitis (Parkhill et al., 2000). Smoking was associated with DNA hypomethylation in the SOCS1 promoter of epithelial cells isolated from saliva of periodontitis subjects (Martinez et al., 2019). Also, DNA hypomethylation of the IL-8 gene promoter in oral epithelial cells from periodontitis subjects was decreased (Oliveira et al., 2009).
Advances in analyzing equipment have recently been developed and the next generation sequencer (NGS) has emerged as a powerful tool. Due to NGS, GWAS have been widely accepted and performed worldwide to elucidate linkages between genetic background and potential susceptibility to diseases.
GWAS for periodontal diseases reported 41 consensus master regulator genes (Sawle et al., 2016) and signals for periodontal complex traits (Offenbacher et al., 2016). More specifically, in patients with chronic periodontal conditions four genes (NIN, ABHD12B, WHAMM, and AP32) have been identified (Rhodin et al., 2014). Sanger sequencing revealed the association of NOD2 mutations in a Japanese population with aggressive periodontal disease (Sudo et al., 2017).
Due to the diversity in the pathogens, environmental factors and genetics, further studies need to be performed in order to develop their association with periodontitis.
The expression proteome combines the terms proteins and genes, and implies the simultaneous study of all proteins in a cell and protein complements of the genome (Parker et al., 2010). Studies in periodontology using proteomic analysis of whole saliva from chronic periodontitis patients were able to show the suppression of several salivary proteins related to protective functions and oral homeostasis (Hartenbach et al., 2020).
The advancement of analyzing equipment such as NGSs and mass spectrometry (MS) allowed the emergence of the new field of “Omics.” Omic technologies analyze genetic or molecular profiles and obtain a complete assessment of the functional activity of biochemical pathways with their structural genetic sequence differences among individuals. (Aardema, 2002). The complementary advances in information technology and sequencing devices in this decade have enabled analysis of vast amounts of Omics information, contributing to better understanding of disease activity to improve our prediction for disease onset and progression (Eicher et al., 2020). In recent years, various investigations were carried out using preclinical animal and translational Omics analyses to elucidate pathological mechanisms (Rhodin et al., 2014; Offenbacher et al., 2016; Sawle et al., 2016; Sudo et al., 2017; Maekawa et al., 2019; Shin et al., 2019). Since Omics probe different cellular layers, they are categorized at DNA, RNA, protein, or metabolite levels (Yugi et al., 2016). Accordingly, current studies encompass genomics/epigenomics, transcriptomics, proteomics and metabolomics. The oral microbiome research has recently moved to a higher level of granularity using these approaches to both host and microbe investigations. For example, transcriptomics allows us to understand the conserved disease-associated gene expression profile in the microbiome for the onset of gingivitis and progression to periodontitis (Yost et al., 2015; Nowicki et al., 2018). Another more general approach is metagenome analysis, with the purpose to identify novel biocatalyst genes to increase our understanding of microbial ecology (Streit and Schmitz, 2004). It enables investigators to screen for activities and functions of interest.
Studies in periodontology using proteomic analysis of whole saliva from chronic periodontitis patients were able to show the suppression of several salivary proteins related to protective functions and oral homeostasis (Hartenbach et al., 2020). In 2019, deep sequencing combined with rapid shotgun analysis for ultra-deep and quantitative saliva proteome characterization identified 5,500 host proteins and more than 2,000 microbial proteins in samples of periodontitis patients, with a strong correlation between dynamics of the oral microbiome and salivary proteins (Grassl et al., 2016).
The “shotgun” proteomics analysis revealed that S100A8 and S100A9 in saliva are potential biomarkers for active periodontitis (Shin et al., 2019). In support of this, several studies have revealed their pathogenic roles in periodontitis progression (Maekawa et al., 2019; Liu et al., 2020). S100A8 and S100A9, two pro-inflammatory calcium-binding S100 proteins acting as damage-associated molecular patterns, were implicated in pathogenesis of rheumatic diseases having strong associations with the disease activity and potential as therapeutic targets (Austermann et al., 2018). S100A9 was also reported to increase IL-6 production and RANKL expression in osteocyte-like cells suggesting potential as osteoimmune biomarker for inflammatory osteolysis (Takagi et al., 2020).
Liquid biopsies are an emerging innovative strategy with reduced invasiveness, especially in the medical field of cancer (Heitzer et al., 2019). So far, biopsy samples are mainly blood and serve for the detection of tumor components including circulating tumor cells, circulating tumor DNA, cell-free RNA, extracellular vesicles, and miRNA. Unlike solid biopsies, liquid biopsy are less invasive and easier to obtain with the purpose of early disease detection (Sierra et al., 2020), prognosis and follow-up (Wan et al., 2017). Recently, attention has also been drawn toward oral fluid-based liquid biopsies for detecting RNA and miRNA in saliva and GCF.
miRNAs are gene regulatory molecules in multicellular organisms and likely influence the output of many protein-coding genes (Bartel, 2004). Over the past decade, research related to microRNAs (miRNA) in periodontitis has accumulated (Kebschull and Papapanou, 2000; Xie et al., 2011; Perri et al., 2012; Stoecklin-Wasmer et al., 2012). To date, 25 miRNAs were reported to be significantly up-regulated and six miRNAs down-regulated in inflamed gingival tissues in periodontal patients (Luan et al., 2017; Luan et al., 2018). Several studies on the expression of miRNAs using various dental tissue cells such as gingival fibroblasts (Sipert et al., 2014; Matsui and Ogata, 2016) and PDL cells (Chen et al., 2015) have been performed (Sehic et al., 2017).
Recently, Saito et al. investigated 600 miRNAs in GCF and found that they had a different profile compared to GCF in healthy subjects (Saito et al., 2017). The expression of miR-1226 in GCF in patients with severe chronic periodontitis was significantly down-regulated (Mico-Martinez et al., 2018) while other groups reported that miRNAs associated with inflammation (e.g., miR-146a and miR-155) were significantly up-regulated in chronic periodontitis with type 2 diabetes. Furthermore, pilot studies have reported increased expression of miR-143-3p (Nisha et al., 2019), miR-381-3p (Fujimori et al., 2019) in chronic periodontitis patients. Interestingly, these microRNAs expressions in GCF were reduced after non-surgical therapy when compared to the level of healthy controls (Radovic et al., 2018).
Due to the scarcity of studies on miRNAs in GCF and saliva (Saito et al., 2017; Atsawasuwan et al., 2018; Mico-Martinez et al., 2018; Radovic et al., 2018; Fujimori et al., 2019; Al-Rawi et al., 2020) further studies are still needed. Wearable sensors in the oral cavity may allow detection of miRNA changes, which may be useful for more detailed diagnosis and tracking of dynamics in periodontitis.
In search for functional and regulatory periodontitis biomarkers small extracellular vesicles (sEV) in saliva related to miRNAs were recently investigated. One study reported that three miRNAs, hsa-miR-140-5p, hsa-miR-146a-5p and hsa-miR-628-5p in sEV, may have potential as diagnostic biomarkers for periodontitis (Han et al., 2020).
Modern matrix-assisted laser desorption ionization time-of-flight (MALDI-TOF) MS can perform quick and accurate proteome analyses to characterize a wide spectrum of samples. A study using MALDI-TOF MS examined the differentially expressed peptide peaks in saliva, GCF, and dental plaque samples and revealed high sensitivity and specificity in diagnosing periodontitis (Antezack et al., 2020).
Taken together, these technological advances in Omics performance and the need for a systems approach to achieve the goal of precision medicine highlight the next Frontier in multi-level Omics studies on large populations to define periodontitis phenome and refine biomarker arrays with high predictive values for disease progression. Moreover, multi-level omics analyses (Trans-OWAS) emerge to elucidate more comprehensive mechanisms in diseases to ultimately define disease phenotypes based on pathogenesis rather than amount and pattern of tissue destruction (Yugi et al., 2016). Trans-OWAS analysis is reconstructing a global biochemical network by connecting multi-omic layers. Further technologies, combining multi-omics with deep learning will help develop diagnostic systems using various biomarkers, clinical parameters and demographic data to create databases that perpetually refine the phenome (Figure 2).
FIGURE 2. Patient stratification workflow for oral screening and monitoring. Multi-level omics analyses (Trans-OWAS) analyses are used to construct the relationships between periodontal phenotypes, which collectively define the phenome, and key biomarkers identified across omics layers. The combination of genetic (1) and environmental information (2) to develop deep learning algorithms from dense population-wide data helps us design periodontal phenotype-specific biomarker panels to more accurately and precisely predict disease progression and response to therapy. This further allows for validation of diagnostic systems based on pathogenesis rather than amount and pattern of tissue destruction. Population-wide trans-OWAS integration (3) and deep learning identify and perpetually redefine phenotypes and refine by-phenotype biomarker panel array systems (4). This facilitates patient stratification for high predictive values of tests to determine disease susceptibility. Salivary screening for disease activity biomarkers classifies individuals as being at low, moderate or high risk for disease onset or progression. This allows for timely disease assessment and precise management by combining clinical examinations with further targeted salivary tests and pharmacogenomic analyses.
The most reliable and common methods for detecting protein and gene expression are conventional techniques such as ELISA and quantitative PCR (qPCR), respectively. These analyses achieve both high sensitivity and high specificity. However, qPCR analysis requires physical manpower, prolonged reaction times, expensive equipment and significant training (Zhu et al., 2020).
In recent years, the accelerated progress in biotechnologies led to the development of biosensors and microelectro-mechanical systems (MEMS). Biosensors recognize the analytes that are sent to reader devices via electrical signals (Kim et al., 2019). MEMS essentially miniaturized the system of biosensors using microfluidics, microdevices and micro total analysis systems with a reduction of the reagents and reaction time (Seker et al., 2011). These technologies enable the rapid detection of analytes with greater sensitivity. Currently, toxins can also be detected using biosensors (Chauhan et al., 2016). Recent advancements that occurred in the field are significant. However, overall commercialization and clinical translation seems to be modest (Kaisti, 2017).
Lab-on-a-chip (LOC) is a subset of MEMS integrating laboratory function on an integrated chip. LOC can provide in vitro diagnostic results ad-hoc. After the recognition of the specific analyte, the information is transduced to an integrated chip (Nguyen et al., 2018). In 2020, a new polymeric LOC based on a microfluidic capillary flow assay with on-chip dried reagents was created to detect unbound cortisol in saliva. Increased cortisol levels were reported in mentally-challenged patients (Heinze et al., 2016), thus monitoring cortisol level in saliva can be a valuable predictor for early detection of psychological disorders.
Another technique known as integrated two-dimensional paper network implements an automated multi-step processing for viral detection, enabling us to identify the presence of viruses within minutes. Influenza virus can be identified by surface glycoproteins and hemagglutinin. This technology has already been applied to other viruses such as norovirus, rotavirus, adenovirus, influenza virus, and respiratory syncytial virus (Zhu et al., 2020).
Various field-effect transistor sensors can enable detection of ions, molecules, oligonucleotides and proteins in a fast response time (Kaisti, 2017). Furthermore, biosensors using wireless graphene nanosensors are able to detect oral microorganisms at a single cell level on hard tissue surfaces like tooth enamel (Mannoor et al., 2012). Mannoor et al., 2012 confirmed that biosensors placed on a tooth were capable of remote monitoring and detection of bacteria in saliva. Additionally, biosensors on mouthguards have been reported to analyze metabolites (Kim et al., 2014) and the uric acid (Kim et al., 2015) in saliva. This device could detect analytes with high sensitivity and stability, and monitor them in a real-time manner. Heikenfeld et al. (Heikenfeld et al., 2019) reported recent trends to wear the lab, and in the future the implantation of the lab could follow. In oral environments, there are many opportunities to place the LOC technology in association with restorations of teeth or dental implants, or simply wear the LOC embedded into a mouthguard (Figure 3).
FIGURE 3. The evolution of diagnostic devices and wearable lab-on-a-chip’s (LOC) for precision medicine applications. (A): The most common diagnostic approaches to measuring soluble biomarkers are “sample-to-lab” and “lab-to-sample,” i.e., samples are either collected from patients, transferred to the lab and analyzed, or tests are delivered directly at the point-of-care for rapid actionable results in the clinic. Technological advancements of the 21st century allow for the development of LOC analyzers to gather diagnostic information chairside in real time. (B) The emerging integration of wearable LOC’s in health care allows for continuous monitoring of physiological and pathological processes, and provide dense individual-level data for Artificial Intelligence (AI)-assisted personalized management. The next Frontier in LOC development may be the fabrication of biocompatible implantable sensors for continuous measurement of soluble biomarkers difficult to measure through the skin. Such advancements will expand diagnostic capabilities, at-home care and telemedicine; (C): Example of a wearable biosensor integrated into a mouthguard to capture a single analyte in saliva over time and transduce the signal via Wi-Fi for analysis; (D): Example of a graphene-based nanosensor adhered to the tooth surface and marginal gingiva to capture and quantify multiple analytes over time. Data is processed onboard and deep learning algorithms applied to establish personal physiological thresholds and out of personal norm trends. Wirelessly transferred output data supports clinical decisions during in-office or teledentistry appointments.
Focus should be directed to discover oral locations in order to monitor patient health conditions, systemic disease, and detect viral and bacterial infections.
Various technologies emerge constantly and develop rapidly. Machine learning using artificial intelligence (AI) accelerates the development of identification of biomarkers for application in dental medicine (Bianchi et al., 2020). As of this moment, technologies have developed to a point where we have achieved a reduction in size of sensors, providing multiple salivary analytes simultaneously and improvements in the sensitivity and specificity of testing. Ongoing developments and innovative approaches will help in the detection of the risk of oral disease, disease onset, and monitoring of oral and systemic conditions.
Oral health care (OHC) practices can contribute to broad-based COVID-19 prevention and management measures through screening, point-of-care (POC) diagnostic tests and appropriate referral of patients in these settings (Figure 4). The integration of POC testing platforms for nucleic acids and antibodies into OHC workflows will allow for continuity of care, rational use of advanced personal protective equipment, reduction in transmission at community level, serosurveillance and contact tracing support during pandemics with pathogens transmitted through respiratory droplets, including SARS-CoV-2.
FIGURE 4. Screening and SARS-CoV-2 point-of-care (POC) Testing for Oral health care (OHC) Decision Making and Serosurveillance. Screening and testing can provide actionable results at POC. COVID-19 screening and prevention protocols in OHC settings should be implemented within the clinical decision trees on delivery of elective or urgent care. Pre-appointment screenings via patient portals or mobile phone apps supplemented with in-house measurement of body temperature and POC testing can be the basis for safe practices and rational use of advanced personal protective equipment. This protocol first establishes who is at low-to-moderate risk (<65 years old, no known risk factors for severe disease outcome, no known exposure to individuals with active disease or recent travel to and from locations with outbreaks, no symptoms and temperature <100°F/37.8°C) vs. high-risk (>65 years old, existing risk factors for severe disease outcome, known exposure to individuals with active disease or travel to and from outbreak locations in the past 14 days, or present symptoms and body temperature >100°F/37.8°C). It then determines by testing if a patient was likely never infected, was previously infected and is immunized or is currently infected with SARS-CoV-2. Non-infected and immunized patients will benefit from both urgent and elective care. Infected asymptomatic should benefit from urgent care only with appropriate prevention measures. All treatments should be deferred for symptomatic infected individuals until recovery. Recovered COVID-19 patients with undetectable virus should benefit from urgent and elective care. Referral of positive cases to primary care physicians, and of immunized, but virus negative patients to blood donation, and contact tracing support will contribute to early management and control of disease spread.
SARS-CoV-2 is detected in saliva of infected individuals (Wyllie et al., 2020; Azzi et al., 2020; Iwasaki et al., 2020; Sabino-Silva et al., 2020; To et al., 2020a; To et al., 2020b). Both saliva and posterior oropharyngeal swab sampling are less invasive, more acceptable to patients, and reduce exposure risks for healthcare workers compared to nasopharyngeal swab sampling. Therefore, salivary diagnostics can provide a convenient and cost-effective POC platform for fast detection of SARS-CoV-2 RNA. Further, using reliable self-collection devices can facilitate direct-to-patient diagnostic testing. The FDA has authorized specific SARS-CoV-2 testing on saliva samples collected at home using a designated self-collection kit (FDA, 2020). The feasibility and reliability of POC testing in OHC settings depends on simplicity and accuracy of the assay, availability and training of personnel to carry out the tests, and efficient integration without significant interruption of care.
New serological tests are being developed as IgM, IgG or combined lateral flow or ELISA assays to measure antibodies against SARS-CoV-2. They are intended for the qualitative detection and differentiation of IgM (developed early during infection) and IgG (developed late during infection) antibodies to SARS-CoV-2 in serum, plasma or venipuncture whole blood specimens from patients suspected of COVID-19 by a healthcare provider. The validation of such assays on fingerpick blood samples supports the feasibility of testing for anti-SARS-CoV-2 antibodies at the POC, including in OHC settings. It is important to note that: 1) assays to certify an individual’s immunity need to be correlated with protection and have near-perfect specificity when seroprevalence is low e.g., 5% or less; 2) assays to ascertain population-level exposure need well defined sensitivity and specificity for the target population, allowing for adjustment of seroprevalence estimates (Bryant et al., 2020). Further, identification of viral RNA via PCR-free isothermal reaction allows for SARS-CoV-2 identification in saliva samples, but large studies are needed to establish assay accuracy and precision. To achieve precise estimates of disease burden, optimal thresholds for sensitivity/specificity should be set depending on local prevalence and intended use of the assay, prioritizing specificity at the expense of sensitivity in low-prevalence settings, and the opposite in high-prevalence settings.
This review presented a general overview of innovation in point-of care in the periodontal field, however emerging research regarding this topic is ongoing and will allow for future systemic analyses as more data becomes available. There have been many advancements in the development of rapid, chairside POC diagnostics in the biomedical diagnostics arena for the timely assessments of disease diagnosis. With the strong needs within the infectious diseases field for non-invasive and rapid assessment, the use of LOC microfluidics and biosensors offers new avenues in oral disease identification and risk assessment. While the detection of biomarkers of disease in periodontology remains overall limited, innovations in biomedical engineering have provided strong potential in dental medicine. The coupling of computational approaches (AI, machine learning and deep learning) will exploit clinical, biological and other omics data elements based on large data sets for the better stratification of patients. The multi-analyte technologies and use of these approaches will undoubtedly lead to more accurate and comprehensive determinants of other polygenic diseases beyond periodontitis.
All of the authors were involved in the preparation of the manuscript and give consent to the integrity of the contents for consideration of publication.
The work was supported by the Osteology Foundation.
The authors declare that the research was conducted in the absence of any commercial or financial relationships that could be construed as a potential conflict of interest.
This authors thank James Sugai for critically reading the manuscript. All figures were made in BioRender (biorender.com). SM was supported by funding from an Osteology Foundation Research Scholarship.
Aardema, M. J., and MacGregor, J. T. (2002). Toxicology and genetic toxicology in the new era of “toxicogenomics”: impact of “-omics” technologies. Mutat. Res. Fund Mol. Mech. Mutagen 499 (1), 13–25. doi:10.1016/s0027-5107(01)00292-5PubMed |
Afacan, B., Keleş Yücel, Z. P., Paşali, Ç., Atmaca İlhan, H., Köse, T., and Emingil, G. (2020). Effect of non‐surgical periodontal treatment on gingival crevicular fluid hypoxia inducible factor‐1 alpha, vascular endothelial growth factor and tumor necrosis factor‐alpha levels in generalized aggressive periodontitis patients. J. Periodontol. 1–8. doi:10.1002/JPER.19-0521PubMed |
Agard, M., Asakrah, S., and Morici, L. A. (2013). PGE2 suppression of innate immunity during mucosal bacterial infection. Front. Cell Infect. Microbiol. 3, 4. doi:10.3389/fcimb.2013.00045PubMed |
Ahrens, W., Pohlabeln, H., Foraita, R., Nelis, M., Lagiou, P., Lagiou, A., et al. (2014). Oral health, dental care and mouthwash associated with upper aerodigestive tract cancer risk in Europe: the ARCAGE study. Oral Oncol. 50 (6), 616–625. doi:10.1016/j.oraloncology.2014.03.001PubMed |
Akdis, M., Burgler, S., Crameri, R., Eiwegger, T., Fujita, H., Gomez, E., et al. (2011). Interleukins, from 1 to 37, and interferon-γ: receptors, functions, and roles in diseases. J. Allergy Clin. Immunol. 127 (3), 701–721. el 70. doi:10.1016/j.jaci.2010.11.050PubMed |
Akram, Z., Abduljabbar, T., Abu Hassan, M. I., Javed, F., and Vohra, F. (2016). Cytokine profile in chronic periodontitis patients with and without obesity: a systematic review and meta-analysis. Dis. Markers 2016, 1. doi:10.1155/2016/4801418PubMed |
Almeida, A. P. C. P. S. C., Fagundes, N. C. F., Maia, L. C., and Lima, R. R. (2018). Is there an association between periodontitis and atherosclerosis in adults? A systematic review. Curr. Vasc. Pharmacol. 16 (6), 569–582. doi:10.2174/1570161115666170830141852PubMed |
Al-Rawi, N. H., Al-Marzooq, F., Al-Nuaimi, A. S., Hachim, M. Y., and Hamoudi, R. (2020). Salivary microRNA 155, 146a/b and 203: a pilot study for potentially non-invasive diagnostic biomarkers of periodontitis and diabetes mellitus. PLoS One 15 (8), e0237004. doi:10.1371/journal.pone.0237004PubMed |
Al-Shammari, K. F., Giannobile, W. V., Aldredge, W. A., Iacono, V. J., Eber, R. M., Wang, H.-L., et al. (2001). Effect of non-surgical periodontal therapy on C-telopeptide pyridinoline cross-links (ICTP) and interleukin-1 levels. J. Periodontol. 72 (8), 1045–1051. doi:10.1902/jop.2001.72.8.1045PubMed |
Antezack, A., Chaudet, H., Tissot-Dupont, H., Brouqui, P., and Monnet-Corti, V. (2020). Rapid diagnosis of periodontitis, a feasibility study using MALDI-TOF mass spectrometry. PLoS One 15 (3), e0230334. doi:10.1371/journal.pone.0230334PubMed |
Artese, H. P. C., Foz, A. M., Rabelo, M. d. S., Gomes, G. H., Orlandi, M., Suvan, J., et al. (2015). Periodontal therapy and systemic inflammation in type 2 diabetes mellitus: a meta-analysis. PLoS One 10 (5), e0128344. doi:10.1371/journal.pone.0128344PubMed |
Arweiler, N. B., and Netuschil, L. (2016). The oral microbiota. Adv. Exp. Med. Biol. 902, 45–60. doi:10.1007/978-3-319-31248-4_4PubMed |
Atieh, M. A., M. Faggion, C., and Seymour, G. J. (2014). Cytokines in patients with type 2 diabetes and chronic periodontitis: a systematic review and meta-analysis. Diabetes Res. Clin. Pract. 104 (2), e38–e45. doi:10.1016/j.diabres.2014.02.002PubMed |
Atsawasuwan, P., Lazari, P., Chen, Y., Zhou, X., Viana, G., and Evans, C. A. (2018). Secretory microRNA-29 expression in gingival crevicular fluid during orthodontic tooth movement. PLoS One 13 (3), e0194238. doi:10.1371/journal.pone.0194238PubMed |
Austermann, J., Spiekermann, C., and Roth, J. (2018). S100 proteins in rheumatic diseases. Nat. Rev. Rheumatol. 14 (9), 528–541. doi:10.1038/s41584-018-0058-9PubMed |
Azzi, L., Carcano, G., Gianfagna, F., Grossi, P., Gasperina, D. D., Genoni, A., et al. (2020). Saliva is a reliable tool to detect SARS-CoV-2. J. Infect. 81 (1), e45–e50. doi:10.1016/j.jinf.2020.04.005PubMed |
Baeza, M., Morales, A., Cisterna, C., Cavalla, F., Jara, G., Isamitt, Y., et al. (2020). Effect of periodontal treatment in patients with periodontitis and diabetes: systematic review and meta-analysis. J. Appl. Oral Sci. 28, e20190248. doi:10.1590/1678-7757-2019-0248PubMed |
Bahekar, A. A., Singh, S., Saha, S., Molnar, J., and Arora, R. (2007). The prevalence and incidence of coronary heart disease is significantly increased in periodontitis: a meta-analysis. Am. Heart J. 154 (5), 830–837. doi:10.1016/j.ahj.2007.06.037PubMed |
Barros, S. P., Fahimipour, F., Tarran, R., Kim, S., Scarel‐Caminaga, R. M., Justice, A., et al. (2020). Epigenetic reprogramming in periodontal disease: dynamic crosstalk with potential impact in oncogenesis. Periodontol. 2000 82 (1), 157–172. doi:10.1111/prd.12322PubMed |
Barros, S. P., Hefni, E., Fahimipour, F., Kim, S., and Arora, P. (2020). Maintaining barrier function of infected gingival epithelial cells by inhibition of DNA methylation. J. Periodontol. 91 (Suppl. 1), S68–S78. doi:10.1002/JPER.20-0262PubMed |
Bartold, P. M., and Lopez‐Oliva, I. (2020). Periodontitis and rheumatoid arthritis: an update 2012–2017. Periodontol. 2000 83 (1), 189–212. doi:10.1111/prd.12300PubMed |
Bender, P., Bürgin, W. B., Sculean, A., and Eick, S. (2017) Serum antibody levels against Porphyromonas gingivalis in patients with and without rheumatoid arthritis - a systematic review and meta-analysis. Clin. Oral Invest. 21 (1), 33–42. doi:10.1007/s00784-016-1938-5PubMed |
Bianchi, J., de Oliveira Ruellas, A. C., Gonçalves, J. R., Paniagua, B., Prieto, J. C., Styner, M., et al. (2020). Osteoarthritis of the temporomandibular joint can be diagnosed earlier using biomarkers and machine learning. Sci. Rep. 10 (1), 8012. doi:10.1038/s41598-020-64942-0PubMed |
Blaizot, A., Vergnes, J. N., Nuwwareh, S., Amar, J., and Sixou, M. (2009). Periodontal diseases and cardiovascular events: meta-analysis of observational studies. Int. Dent. J. 59 (4), 197–209. doi:10.1922/IDJ_2114Sixou13PubMed |
Bostanci, N., İlgenli, T., Emingil, G., Afacan, B., Han, B., Töz, H., et al. (2007). Gingival crevicular fluid levels of RANKL and OPG in periodontal diseases: implications of their relative ratio. J. Clin. Periodontol. 34 (5), 370–376. doi:10.1111/j.1600-051X.2007.01061.xPubMed |
Brinkmann, O., Zhang, L., Giannobile, W. V., and Wong, D. T. (2011). Salivary biomarkers for periodontal disease diagnostics. Expert Opin. Med. Diagn. 5 (1), 25–35. doi:10.1517/17530059.2011.542144PubMed |
Bryant, J. E., Azman, A. S., Ferrari, M. J., Arnold, B. F., Boni, M. F., Boum, Y., et al. (2020). Serology for SARS-CoV-2: apprehensions, opportunities, and the path forward. Sci. Immunol. 5 (47), eabc6347. doi:10.1126/sciimmunol.abc6347PubMed |
Buck, M. R., Karustis, D. G., Day, N. A., Honn, K. V., and Sloane, B. F. (1992). Degradation of extracellular-matrix proteins by human cathepsin B from normal and tumour tissues. Biochem. J. 282 (Pt 1), 273–278. doi:10.1042/bj2820273PubMed | | PMID: 1540143; Central PMCID: PMCPMC1130919.
Buhlin, K., Hultin, M., Norderyd, O., Persson, L., Pockley, A. G., Rabe, P., et al. (2009). Risk factors for atherosclerosis in cases with severe periodontitis. J. Clin. Periodontol. 36 (7), 541–549. doi:10.1111/j.1600-051X.2009.01430.xPubMed |
Cagney, D. N., Sul, J., Huang, R. Y., Ligon, K. L., en, P. Y., and Alexander, B. M. (2018). The FDA NIH biomarkers, endpointS, and other tools (BEST) resource in neuro-oncology. Neuro Oncol. 20 (9), 1162–1172. doi:10.1093/neuonc/nox242PubMed |
Calderaro, D. C., Corrêa, J. D., Ferreira, G. A., Barbosa, I. G., Martins, C. C., Silva, T. A., et al. (2017). Influence of periodontal treatment on rheumatoid arthritis: a systematic review and meta-analysis. Rev. Bras. Reumatol. 57 (3), 238–244. doi:10.1016/j.rbre.2016.11.011PubMed |
Chang, P. V., Hao, L., Offermanns, S., and Medzhitov, R. (2014). The microbial metabolite butyrate regulates intestinal macrophage function via histone deacetylase inhibition. Proc. Natl. Acad. Sci. U.S.A. 111 (6), 2247–2252. doi:10.1073/pnas.1322269111PubMed |
Chauhan, R., Singh, J., Sachdev, T., Basu, T., and Malhotra, B. D. (2016). Recent advances in mycotoxins detection. Biosens. Bioelectron. 81, 532–545. doi:10.1016/j.bios.2016.03.004PubMed |
Chen, Y., Mohammed, A., Oubaidin, M., Evans, C. A., Zhou, X., Luan, X., et al. (2015). Cyclic stretch and compression forces alter microRNA-29 expression of human periodontal ligament cells. Gene 566 (1), 13–17. doi:10.1016/j.gene.2015.03.055PubMed |
Christersson, L. A., Slots, J., Rosling, B. G., and Genco, R. J. (1985). Microbiological and clinical effects of surgical treatment of localized juvenile periodontitis. J. Clin. Periodontol. 12 (6), 465–476. doi:10.1111/j.1600-051x.1985.tb01382.xPubMed |
Cui, N., Hu, M., and Khalil, R. A. (2017). Biochemical and biological attributes of matrix metalloproteinases. Prog. Mol. Biol. Transl. Sci. 147, 1–73. doi:10.1016/bs.pmbts.2017.02.005PubMed |
Cullinan, M. P., Westerman, B., Hamlet, S. M., Palmer, J. E., Faddy, M. J., Lang, N. P., et al. (2001). A longitudinal study of interleukin-1 gene polymorphisms and periodontal disease in a general adult population. J. Clin. Periodontol. 28 (12), 1137–1144. doi:10.1034/j.1600-051x.2001.281208.xPubMed |
Darré, L., Vergnes, J.-N., Gourdy, P., and Sixou, M. (2008). Efficacy of periodontal treatment on glycaemic control in diabetic patients: a meta-analysis of interventional studies. Diabetes Metabol. 34 (5), 497–506. doi:10.1016/j.diabet.2008.03.006PubMed |
Darveau, R. P. (2010). Periodontitis: a polymicrobial disruption of host homeostasis. Nat. Rev. Microbiol. 8 (7), 481–490. doi:10.1038/nrmicro2337PubMed |
10.1038/nrmicro2337 CrossRef Full Text | 10.1038/nrmicro2337&btnG= Google Scholar
Delima, A. J., Oates, T., Assuma, R., Schwartz, Z., Cochran, D., Amar, S., et al. (2001). Soluble antagonists to interleukin-1 (IL-1) and tumor necrosis factor (TNF) inhibits loss of tissue attachment in experimental periodontitis. J. Clin. Periodontol. 28 (3), 233–240. doi:10.1034/j.1600-051x.2001.028003233PubMed |
Eicher, T., Kinnebrew, G., Patt, A., Spencer, K., Ying, K., Ma, Q., et al. (2020). Metabolomics and multi-omics integration: a survey of computational methods and resources. Metabolites 10 (5), 202. doi:10.3390/metabo10050202PubMed |
Eke, P. I., Thornton-Evans, G. O., Wei, L., Borgnakke, W. S., Dye, B. A., and Genco, R. J. (2018). Periodontitis in US adults. J. Am. Dent. Assoc. 149 (7), 576–588. doi:10.1016/j.adaj.2018.04.023PubMed |
Engebretson, S., and Kocher, T. (2013). Evidence that periodontal treatment improves diabetes outcomes: a systematic review and meta-analysis. J. Clin. Periodontol. 40 (Suppl. 14), S153–S163. doi:10.1111/jcpe.12084PubMed |
Erdemir, E. O., Duran, I., and Haliloglu, S. (2004). Effects of smoking on clinical parameters and the gingival crevicular fluid levels of IL-6 and TNF-alpha in patients with chronic periodontitis. J. Clin. Periodontol. 31 (2), 99–104. doi:10.1111/j.0303-6979.2004.00454.xPubMed |
Eskandari-Nasab, E., Moghadampour, M., and Tahmasebi, A. (2017). Meta-analysis of risk association between interleukin-17a and F gene polymorphisms and inflammatory diseases. J. Interferon Cytokine Res. 37 (4), 165–174. doi:10.1089/jir.2016.0088PubMed |
FAD (2020). Administration. Available at: https://www.fda.gov/news-events/press-announcements/coronavirus-covid-19-update-fda-authorizes-first-diagnostic-test-using-home-collection-saliva/ (Accessed July 15, 2020).
Faggion, C. M., Cullinan, M. P., and Atieh, M. (2016). An overview of systematic reviews on the effectiveness of periodontal treatment to improve glycaemic control. J. Periodontal. Res. 51 (6), 716–725. doi:10.1111/jre.12358PubMed |
Fine, D. H., Markowitz, K., Fairlie, K., Tischio-Bereski, D., Ferrendiz, J., Furgang, D., et al. (2013). A consortium of aggregatibacter actinomycetemcomitans, Streptococcus parasanguinis, and Filifactor alocis is present in sites prior to bone loss in a longitudinal study of localized aggressive periodontitis. J. Clin. Microbiol. 51 (9), 2850–2861. doi:10.1128/JCM.00729-13PubMed |
Freitas, C. O. T. d., Gomes-Filho, I. S., Naves, R. C., Nogueira Filho, G. d. R., Cruz, S. S. d., Santos, C. A. d. S. T., et al. (2012). Influence of periodontal therapy on C-reactive protein level: a systematic review and meta-analysis. J. Appl. Oral Sci. 20 (1), 1–8. doi:10.1590/s1678-77572012000100002PubMed |
Freitag-Wolf, S., Munz, M., Wiehe, R., Junge, O., Graetz, C., Jockel-Schneider, Y., et al. (2019). Smoking modifies the genetic risk for early-onset periodontitis. J. Dent. Res. 98 (12), 1332–1339. doi:10.1177/0022034519875443PubMed |
Frencken, J. E., Sharma, P., Stenhouse, L., Green, D., Laverty, D., and Dietrich, T. (2017). Global epidemiology of dental caries and severe periodontitis - a comprehensive review. J. Clin. Periodontol. 44 (Suppl. 18), S94–S105. doi:10.1111/jcpe.12677PubMed |
Fujimori, K., Yoneda, T., Tomofuji, T., Ekuni, D., Azuma, T., Maruyama, T., et al. (2019). Detection of salivary miRNAs reflecting chronic periodontitis: a pilot study. Molecules 24 (6), 103, 4. doi:10.3390/molecules24061034PubMed |
Garde, S., Akhter, R., Nguyen, M. A., Chow, C. K., and Eberhard, J. (2019). Periodontal therapy for improving lipid profiles in patients with type 2 diabetes mellitus: a systematic review and meta-analysis. Int. J. Mol. Sci. 20 (15), 3826. doi:10.3390/ijms20153826PubMed |
Giannobile, W. V. (1999). C-telopeptide pyridinoline cross-links: sensitive indicators of periodontal tissue destruction. Ann. N. Y. Acad. Sci. 878, 404–412. doi:10.1111/j.1749-6632.1999.tb07698.xPubMed |
Giannobile, W. V. (2012). Salivary diagnostics for periodontal diseases. J. Am. Dent. Assoc. 143 (10 Suppl. l), 6S–11S. doi:10.14219/jada.archive.2012.0341PubMed |
Giannobile, W. V., Braun, T. M., Caplis, A. K., Doucette-Stamm, L., Duff, G. W., and Kornman, K. S. (2013). Patient stratification for preventive care in dentistry. J. Dent. Res. 92 (8), 694–701. doi:10.1177/0022034513492336PubMed |
Giannobile, W. V., Kornman, K. S., and Williams, R. C. (2013). Personalized medicine enters dentistry J. Am. Dent. Assoc. 144 (8), 874–876. doi:10.14219/jada.archive.2013.0200PubMed |
Giannobile, W. V., Lynch, S. E., Denmark, R. G., Paquette, D. W., Fiorellini, J. P., and Williams, R. C. (1995). Crevicular fluid osteocalcin and pyridinoline cross-linked carboxyterminal telopeptide of type I collagen (ICTP) as markers of rapid bone turnover in periodontitis. A pilot study in beagle dogs. J. Clin. Periodontol. 22 (12), 903–910. doi:10.1111/j.1600-051x.1995.tb01793.xPubMed |
Goodson, J. M., Haffajee, A. D., and Socransky, S. S. (1984). The relationship between attachment level loss and alveolar bone loss. J. Clin. Periodontol. 11 (5), 348–359. doi:10.1111/j.1600-051x.1984.tb01331.xPubMed |
Goodson, J. M., Tanner, A. C. R., Haffajee, A. D., Sornberger, G. C., and Socransky, S. S. (1982). Patterns of progression and regression of advanced destructive periodontal disease. J. Clin. Periodontol. 9 (6), 472–481. doi:10.1111/j.1600-051x.1982.tb02108.xPubMed |
Grassl, N., Kulak, N. A., Pichler, G., Geyer, P. E., Jung, J., Schubert, S., et al. (2016). Ultra-deep and quantitative saliva proteome reveals dynamics of the oral microbiome. Genome Med. 8 (1), 44. doi:10.1186/s13073-016-0293-0PubMed |
Gürsoy, U. K., Könönen, E., Tervahartiala, T., Gürsoy, M., Pitkänen, J., Torvi, P., et al. (2018). Molecular forms and fragments of salivary MMP‐8 in relation to periodontitis. J. Clin. Periodontol. 45 (12), 1421–1428. doi:10.1111/jcpe.13024PubMed |
Hajishengallis, G. (2014). Immunomicrobial pathogenesis of periodontitis: keystones, pathobionts, and host response. Trends Immunol. 35 (1), 3–11. doi:10.1016/j.it.2013.09.001PubMed |
Hajishengallis, G. (2015). Periodontitis: from microbial immune subversion to systemic inflammation. Nat. Rev. Immunol. 15 (1), 30–44. doi:10.1038/nri3785PubMed |
Hajishengallis, G., Darveau, R. P., and Curtis, M. A. (2012). The keystone-pathogen hypothesis. Nat. Rev. Microbiol. 10 (10), 717–725. doi:10.1038/nrmicro2873PubMed |
Hajishengallis, G., and Korostoff, J. M. (2017). Revisiting the Page & Schroeder model: the good, the bad and the unknowns in the periodontal host response 40 years later. Periodontol. 2000 75 (1), 116–151. doi:10.1111/prd.12181PubMed |
Han, J. Y., and Reynolds, M. A. (2012). Effect of anti-rheumatic agents on periodontal parameters and biomarkers of inflammation: a systematic review and meta-analysis. J. Periodontal Implant Sci. 42 (1), 3–12. doi:10.5051/jpis.2012.42.1.3PubMed |
Han, P., Bartold, P. M., Salomon, C., and Ivanovski, S. (2020). Salivry small extracellular vesicles associated miRNAs in periodontal status-A pilot study. Int. J. Mol. Sci. 21 (8), 2809. doi:10.3390/ijms21082809PubMed |
Hartenbach, F. A. R. R., Velasquez, É., Nogueira, F. C. S., Domont, G. B., Ferreira, E., and Colombo, A. P. V. (2020). Proteomic analysis of whole saliva in chronic periodontitis. J. Proteom. 213, 103602. doi:10.1016/j.jprot.2019.103602PubMed |
Heikenfeld, J., Jajack, A., Feldman, B., Granger, S. W., Gaitonde, S., Begtrup, G., et al. (2019). Accessing analytes in biofluids for peripheral biochemical monitoring. Nat. Biotechnol. 37 (4), 407–419. doi:10.1038/s41587-019-0040-3PubMed |
Heikkilä, P., But, A., Sorsa, T., and Haukka, J. (2018). Periodontitis and cancer mortality: register-based cohort study of 68,273 adults in 10-year follow-up. Int. J. Canc. 142 (11), 2244–2253. doi:10.1002/ijc.31254PubMed |
Heinze, K., Lin, A., Reniers, R. L. E. P., and Wood, S. J. (2016). Longer-term increased cortisol levels in young people with mental health problems. Psychiatr. Res. 236, 98–104. doi:10.1016/j.psychres.2015.12PubMed |
Heitzer, E., Haque, I. S., Roberts, C. E. S., and Speicher, M. R. (2019). Current and future perspectives of liquid biopsies in genomics-driven oncology. Nat. Rev. Genet. 20 (2), 71–88. doi:10.1038/s41576-018-0071-5PubMed |
Hussain, S. B., Botelho, J., Machado, V., Zehra, S. A., Mendes, J. J., Ciurtin, C., et al. (2020). Is there a bidirectional association between rheumatoid arthritis and periodontitis? A systematic review and meta-analysis. Semin. Arthritis Rheum. 50 (3), 414–422. doi:10.1016/j.semarthrit.2020.01.009PubMed |
Iwasaki, S., Fujisawa, S., Nakakubo, S., Kamada, K., Yamashita, Y., Fukumoto, T., et al. (2020). Comparison of SARS-CoV-2 detection in nasopharyngeal swab and saliva. J. Infect. 81, e145. doi:10.1016/j.jinf.2020.05.071PubMed |
Jain, A., Jain, A., Gupta, J., Bansal, D., Sood, S., and Gupta, S. (2019). Effect of scaling and root planing as monotherapy on glycemic control in patients of type 2 diabetes with chronic periodontitis: a systematic review and meta-analysis. J. Indian Soc. Periodontol. 23 (4), 303–310. doi:10.4103/jisp.jisp_417_18PubMed |
Jiménez, Y., Bagán, J., Milián, M., Gavaldá, C., and Scully, C. (2002). Lichen sclerosus et atrophicus manifesting with localized loss of periodontal attachment. Oral Dis. 8 (6), 310–313. doi:10.1034/j.1601-0825.2002.02858.xPubMed |
Jorth, P., Turner, K. H., Gumus, P., Nizam, N., Buduneli, N., and Whiteley, M. (2014). Metatranscriptomics of the human oral microbiome during health and disease. mBio 5 (2), e01012-14. doi:10.1128/mBio.01012-14PubMed |
Joshi, C., Bapat, R., Anderson, W., Dawson, D., Hijazi, K., and Cherukara, G. (2019). Detection of periodontal microorganisms in coronary atheromatous plaque specimens of myocardial infarction patients: a systematic review and meta-analysis. Trends Cardiovasc. Med. S1050–S1738, 30175–30176. doi:10.1016/j.tcm.2019.12.005PubMed |
Kaisti, M. (2017). Detection principles of biological and chemical FET sensors. Biosens. Bioelectron. 98, 437–448. doi:10.1016/j.bios.2017.07.010PubMed |
Kang, J., Smith, S., Pavitt, S., and Wu, J. (2019). Association between central obesity and tooth loss in the non‐obese people: results from the continuous National Health and Nutrition Examination Survey ( NHANES ) 1999–2012. J. Clin. Periodontol. 46 (4), 430–437. doi:10.1111/jcpe.13091PubMed |
Kassebaum, N. J., Bernabé, E., Dahiya, M., Bhandari, B., Murray, C. J. L., and Marcenes, W. (2014). Global burden of severe periodontitis in 1990-2010. J. Dent. Res. 93 (11), 1045–1053. doi:10.1177/0022034514552491PubMed |
Kaur, S., Bright, R., Proudman, S. M., and Bartold, P. M. (2014). Does periodontal treatment influence clinical and biochemical measures for rheumatoid arthritis? A systematic review and meta-analysis. Semin. Arthritis Rheum. 44 (2), 113–122. doi:10.1016/j.semarthrit.2014.04.009PubMed |
Kc, S., Wang, X. Z., and Gallagher, J. E. (2020). Diagnostic sensitivity and specificity of host‐derived salivary biomarkers in periodontal disease amongst adults: systematic review. J. Clin. Periodontol. 47 (3), 289–308. doi:10.1111/jcpe.13218PubMed |
Kebschull, M., and Papapanou, P. N. (2015). Mini but mighty: microRNAs in the pathobiology of periodontal disease. Periodontol. 2000 69 (1), 201–220. doi:10.1111/prd.12095PubMed |
Kim, J., Campbell, A. S., de Ávila, B. E.-F., and Wang, J. (2019). Wearable biosensors for healthcare monitoring. Nat. Biotechnol. 37 (4), 389–406. doi:10.1038/s41587-019-0045-yPubMed |
Kim, J., Imani, S., de Araujo, W. R., Warchall, J., Valdés-Ramírez, G., Paixão, T. R. L. C., et al. (2015). Wearable salivary uric acid mouthguard biosensor with integrated wireless electronics. Biosens. Bioelectron. 74, 1061–1068. doi:10.1016/j.bios.2015.07.039PubMed |
Kim, J., Valdés-Ramírez, G., Bandodkar, A. J., Jia, W., Martinez, A. G., Ramírez, J., et al. (2014). Non-invasive mouthguard biosensor for continuous salivary monitoring of metabolites. Analyst 139 (7), 1632–1636. doi:10.1039/c3an02359aPubMed |
Kinney, J. S., Morelli, T., Oh, M., Braun, T. M., Ramseier, C. A., Sugai, J. V., et al. (2014). Crevicular fluid biomarkers and periodontal disease progression. J. Clin. Periodontol. 41 (2), 113–120. doi:10.1111/jcpe.12194PubMed |
Kinney, J. S., Ramseier, C. A., and Giannobile, W. V. (2007). Oral fluid-based biomarkers of alveolar bone loss in periodontitis. Ann. N. Y. Acad. Sci. 1098, 230–251. doi:10.1196/annals.1384.028PubMed |
Kitamoto, S., Nagao-Kitamoto, H., Jiao, Y., Gillilland, M. G., Hayashi, A., Imali, J., et al. (2020). The intermucosal connection between the mouth and gut in commensal pathobiont-driven colitis. Cell 182, 447–462.e14. doi:10.1016/j.cell.2020.05.048PubMed |
Kobayashi, T., Ishida, K., and Yoshie, H. (2016). Increased expression of interleukin-6 (IL-6) gene transcript in relation to IL-6 promoter hypomethylation in gingival tissue from patients with chronic periodontitis. Arch. Oral Biol. 69, 89–94. doi:10.1016/j.archoralbio.2016.05.018PubMed |
Kornman, K. S. (2008). Mapping the pathogenesis of periodontitis: a new look. J. Periodontol. 79 (8 Suppl. l), 1560–1568. doi:10.1902/jop.2008.080213PubMed |
Kornman, K. S., Crane, A., Wang, H.-Y., Giovlne, F. S. d., Newman, M. G., Pirk, F. W., et al. (1997). The interleukin-1 genotype as a severity factor in adult periodontal disease. J. Clin. Periodontol. 24 (1), 72–77. doi:10.1111/j.1600-051x.1997.tb01187.xPubMed |
Kornman, K. S., Giannobile, W. V., and Duff, G. W. (2017). Quo vadis: what is the future of periodontics? How will we get there? Periodontol. 2000 75 (1), 353–371. doi:10.1111/prd.12217PubMed |
Kornman, K. S., Page, R. C., and Tonetti, M. S. (1997). The host response to the microbial challenge in periodontitis: assembling the players. Periodontol. 2000 14, 33–53. doi:10.1111/j.1600-0757.1997.tb00191.xPubMed |
Kornman, K. S., and Robertson, P. B. (1985). Clinical and microbiological evaluation of therapy for juvenile periodontitis. J. Periodontol. 56 (8), 443–446. doi:10.1902/jop.1985.56.8.443PubMed |
Korte, D. L., and Kinney, J. (2016). Personalized medicine: an update of salivary biomarkers for periodontal diseases. Periodontol. 2000 70 (1), 26–37. doi:10.1111/prd.12103PubMed |
Krishnan, K., Chen, T., and Paster, B. (2017). A practical guide to the oral microbiome and its relation to health and disease. Oral Dis. 23 (3), 276–286. doi:10.1111/odi.12509PubMed |
Kröger, A., Hülsmann, C., Fickl, S., Spinell, T., Hüttig, F., Kaufmann, F., et al. (2018). The severity of human peri‐implantitis lesions correlates with the level of submucosal microbial dysbiosis. J. Clin. Periodontol. 45 (12), 1498–1509. doi:10.1111/jcpe.13023PubMed |
Kwok, V., and Caton, J. G. (2007). Commentary: prognosis revisited: a system for assigning periodontal prognosis. J. Periodontol. 78 (11), 2063–2071. doi:10.1902/jop.2007.070210PubMed |
Larsson, L., Castilho, R. M., and Giannobile, W. V. (2015). Epigenetics and its role in periodontal diseases: a state-of-the-art review. J. Periodontol. 86 (4), 556–568. doi:10.1902/jop.2014.140559PubMed |
Latella, M. C., de Gaetano, M., Di Castelnuovo, A., Napoleone, E., Lorenzet, R., Gattone, M., et al. (2009). Interleukin 1 gene cluster, myocardial infarction at young age and inflammatory response of human mononuclear cells. Immunol. Invest. 38 (3-4), 203–219. doi:10.1080/08820130902766142PubMed |
Lauritano, D., Boccalari, E., Stasio, D., Vella, F., Carinci, F., Lucchese, A., et al. (2019). Prevalence of oral lesions and correlation with intestinal symptoms of inflammatory bowel disease: a systematic review. Diagnostics 9 (3), 77. doi:10.3390/diagnostics9030077PubMed |
Lee, A., Ghaname, C. B., Braun, T. M., Sugai, J. V., Teles, R. P., Loesche, W. J., et al. (2012). Bacterial and salivary biomarkers predict the gingival inflammatory profile. J. Periodontol. 83 (1), 79–89. doi:10.1902/jop.2011.110060PubMed |
Liu, M., Won Lee, J., Jung, S., Ji, S., and Choi, Y. (2020). Ability of S100 proteins and matrix metalloproteinase‐9 to identify periodontitis in a ligature‐induced periodontitis dog model. J. Clin. Periodontol. 47 (2), 182–192. doi:10.1111/jcpe.13215PubMed |
Loe, H., Anerud, A., Boysen, H., and Smith, M. (1978). The natural history of periodontal disease in man. J. Periodontal. Res. 13 (6), 563–572. doi:10.1111/j.1600-0765.1978.tb00210.xPubMed |
Luan, X., Zhou, X., Naqvi, A., Francis, M., Foyle, D., Nares, S., et al. (2018). MicroRNAs and immunity in periodontal health and disease. Int. J. Oral Sci. 10 (3), 24. doi:10.1038/s41368-018-0025-yPubMed |
Luan, X., Zhou, X., Trombetta-eSilva, J., Francis, M., Gaharwar, A. K., Atsawasuwan, P., et al. (2017). MicroRNAs and periodontal homeostasis. J. Dent. Res. 96 (5), 491–500. doi:10.1177/0022034516685711PubMed |
MacGregor, J. A., Paster, B. J., Stokes, L. N., Olsen, I., and Dewhirst, F. E. (2005). Defining the normal bacterial flora of the oral cavity. J. Clin. Microbiol. 43 (11), 5721–5732. doi:10.1128/jcm.43.11.5721-5732.2005PubMed |
Maekawa, S., Onizuka, S., Katagiri, S., Hatasa, M., Ohsugi, Y., Sasaki, N., et al. (2019). RNA sequencing for ligature induced periodontitis in mice revealed important role of S100A8 and S100A9 for periodontal destruction. Sci. Rep. 9 (1), 14663. doi:10.1038/s41598-019-50959-7PubMed |
Mager, D., Haffajee, A., Devlin, P., Norris, C., Posner, M., and Goodson, J. (2005). The salivary microbiota as a diagnostic indicator of oral cancer: a descriptive, non-randomized study of cancer-free and oral squamous cell carcinoma subjects. J. Transl. Med. 3, 27. doi:10.1186/1479-5876-3-27PubMed |
Mannoor, M. S., Tao, H., Clayton, J. D., Sengupta, A., Kaplan, D. L., Naik, R. R., et al. (2012). Graphene-based wireless bacteria detection on tooth enamel. Nat. Commun. 3, 763. doi:10.1038/ncomms1767PubMed |
Martinez, C. d. J. H., Villafuerte, K. R. V., Luchiari, H. R., Cruz, J. d. O., Sales, M., Palioto, D. B., et al. (2019). Effect of smoking on the DNA methylation pattern of the SOCS1 promoter in epithelial cells from the saliva of patients with chronic periodontitis. J. Periodontol. 90 (11), 1279–1286. doi:10.1002/JPER.18-0692PubMed |
Matsui, S., and Ogata, Y. (2016). Effects of miR-223 on expression of IL-1β and IL-6 in human gingival fibroblasts. J. Oral Sci. 58 (1), 101–108. doi:10.2334/josnusd.58.101PubMed |
McCauley, L. K., and Nohutcu, R. M. (2002). Mediators of periodontal osseous destruction and remodeling: principles and implications for diagnosis and therapy. J. Periodontol. 73 (11), 1377–1391. doi:10.1902/jop.2002.73.11.1377PubMed |
McGuire, M. K., and Nunn, M. E. (1996). Prognosis versus actual outcome. II. The effectiveness of clinical parameters in developing an accurate prognosis. J. Periodontol. 67 (7), 658–665. doi:10.1902/jop.1996.67.7.658PubMed |
McGuire, M. K., and Nunn, M. E. (1999). Prognosis versus actual outcome. IV. The effectiveness of clinical parameters and IL-1 genotype in accurately predicting prognoses and tooth survival. J. Periodontol. 70 (1), 49–56. doi:10.1902/jop.1999.70.1.49PubMed |
Meikle, M. C., Hembry, R. M., Holley, J., Horton, C., McFarlane, C. G., and Reynolds, J. J. (1994). Immunolocalization of matrix metalloproteinases and TIMP-1 (tissue inhibitor of metalloproteinases) in human gingival tissues from periodontitis patients. J. Periodontal. Res. 29 (2), 118–126. doi:10.1111/j.1600-0765.1994.tb01100.xPubMed |
Meisel, P., Holtfreter, B., Biffar, R., Suemnig, W., and Kocher, T. (2012). Association of periodontitis with the risk of oral leukoplakia. Oral Oncol. 48 (9), 859–863. doi:10.1016/j.oraloncology.2012.02.022PubMed |
Michalowicz, B. S., Diehl, S. R., Gunsolley, J. C., Sparks, B. S., Brooks, C. N., Koertge, T. E., et al. (2000). Evidence of a substantial genetic basis for risk of adult periodontitis. J. Periodontol. 71 (11), 1699–1707. doi:10.1902/jop.2000.71.11.1699PubMed |
Mico-Martinez, P., Garcia-Gimenez, J., Seco-Cervera, M., Lopez-Roldan, A., Alminana-Pastor, P., Alpiste-Illueca, F., et al. (2018). miR-1226 detection in GCF as potential biomarker of chronic periodontitis: a pilot study. Med. Oral 23 (3), e308–e14. doi:10.4317/medoral.22329PubMed |
Mukhopadhyay, S., Johnson, T. A., Duru, N., Buzza, M. S., Pawar, N. R., Sarkar, R., et al. (2019). Fibrinolysis and inflammation in venous thrombus resolution. Front. Immunol. 10, 1348. doi:10.3389/fimmu.2019.01348PubMed |
Muñoz Aguilera, E., Suvan, J., Buti, J., Czesnikiewicz-Guzik, M., Barbosa Ribeiro, A., Orlandi, M., et al. (2020). Periodontitis is associated with hypertension: a systematic review and meta-analysis. Cardiovasc. Res. 116 (1), 28–39. doi:10.1093/cvr/cvz201PubMed |
Munz, M., Richter, G. M., Loos, B. G., Jepsen, S., Divaris, K., Offenbacher, S., et al. (2018). Genome-wide association meta-analysis of coronary artery disease and periodontitis reveals a novel shared risk locus. Sci. Rep. 8 (1), 13678. doi:10.1038/s41598-018-31980-8PubMed |
Mustapha, I. Z., Debrey, S., Oladubu, M., and Ugarte, R. (2007). Markers of systemic bacterial exposure in periodontal disease and cardiovascular disease risk: a systematic review and meta-analysis. J. Periodontol. 78 (12), 2289–2302. doi:10.1902/jop.2007.070140PubMed |
Nascimento, G. G., Baelum, V., Sorsa, T., Tervahartiala, T., Skottrup, P. D., and López, R. (2019). Salivary levels of MPO, MMP-8 and TIMP-1 are associated with gingival inflammation response patterns during experimental gingivitis. Cytokine 115, 135–141. doi:10.1016/j.cyto.2018.12.002PubMed |
Nguyen, T., Zoëga Andreasen, S., Wolff, A., and Duong Bang, D. (2018). From lab on a chip to point of care devices: the role of open source microcontrollers. Micromachines 9 (8), 403. doi:10.3390/mi9080403PubMed |
Nibali, L., Bayliss-Chapman, J., Almofareh, S. A., Zhou, Y., Divaris, K., and Vieira, A. R. (2019). What is the heritability of periodontitis? A systematic review. J. Dent. Res. 98 (6), 632–641. doi:10.1177/0022034519842510PubMed |
Nisha, K. J., Janam, P., and Harshakumar, K. (2019). Identification of a novel salivary biomarker miR‐143‐3p for periodontal diagnosis: a proof of concept study. J. Periodontol. 90 (10), 1149–1159. doi:10.1002/JPER.18-0729PubMed |
Nowicki, E. M., Shroff, R., Singleton, J. A., Renaud, D. E., Wallace, D., Drury, J., et al. (2018). Microbiota and metatranscriptome changes accompanying the onset of gingivitis. mBio 9 (2), e00575. doi:10.1128/mBio.00575-18PubMed |
Offenbacher, S., Barros, S., Mendoza, L., Mauriello, S., Preisser, J., Moss, K., et al. (2010). Changes in gingival crevicular fluid inflammatory mediator levels during the induction and resolution of experimental gingivitis in humans. J. Clin. Periodontol. 37 (4), 324–333. doi:10.1111/j.1600-051X.2010.01543.xPubMed |
Offenbacher, S., Divaris, K., Barros, S. P., Moss, K. L., Marchesan, J. T., Morelli, T., et al. (2016). Genome-wide association study of biologically informed periodontal complex traits offers novel insights into the genetic basis of periodontal disease. Hum. Mol. Genet. 25 (10), 2113–2129. doi:10.1093/hmg/ddw069PubMed |
Offenbacher, S., Jiao, Y., Kim, S. J., Marchesan, J., Moss, K. L., Jing, L., et al. (2018). GWAS for Interleukin-1β levels in gingival crevicular fluid identifies IL37 variants in periodontal inflammation. Nat. Commun. 9 (1), 3686. doi:10.1038/s41467-018-05940-9PubMed |
Oliveira, N. F. P., Damm, G. R., Andia, D. C., Salmon, C., Nociti, F. H., Line, S. R. P., et al. (2009). DNA methylation status of theIL8gene promoter in oral cells of smokers and non-smokers with chronic periodontitis. J. Clin. Periodontol. 36 (9), 719–725. doi:10.1111/j.1600-051X.2009.01446.xPubMed |
Ouyang, W., and O’Garra, A. (2019). IL-10 family cytokines IL-10 and IL-22: from basic science to clinical translation. Immunity 50 (4), 871–891. doi:10.1016/j.immuni.2019.03.020PubMed |
Page, R. C., and Kornman, K. S. (1997). The pathogenesis of human periodontitis: an introduction. Periodontol. 2000 14, 9–11. doi:10.1111/j.1600-0757.1997.tb00189.xPubMed |
Papageorgiou, S. N., Hagner, M., Nogueira, A. V. B., Franke, A., Jäger, A., and Deschner, J. (2017). Inflammatory bowel disease and oral health: systematic review and a meta-analysis. J. Clin. Periodontol. 44 (4), 382–393. doi:10.1111/jcpe.12698PubMed |
Papageorgiou, S. N., Reichert, C., Jäger, A., and Deschner, J. (2015). Effect of overweight/obesity on response to periodontal treatment: systematic review and a meta-analysis. J. Clin. Periodontol. 42 (3), 247–261. doi:10.1111/jcpe.12365PubMed |
Papapanou, P. N. (2015). Systemic effects of periodontitis: lessons learned from research on atherosclerotic vascular disease and adverse pregnancy outcomes. Int. Dent. J. 65 (6), 283–291. doi:10.1111/idj.12185PubMed |
Papapanou, P. N., Sanz, M., Buduneli, N., Dietrich, T., Feres, M., Fine, D. H., et al. (2018). Periodontitis: consensus report of workgroup 2 of the 2017 world workshop on the classification of periodontal and peri-implant diseases and conditions. J. Clin. Periodontol. 45 (Suppl. 20), S162–S170. doi:10.1111/jcpe.12946PubMed |
Paraskevas, S., Huizinga, J. D., and Loos, B. G. (2008). A systematic review and meta-analyses on C-reactive protein in relation to periodontitis. J. Clin. Periodontol. 35 (4), 277–290. doi:10.1111/j.1600-051X.2007.01173.xPubMed |
Parker, C. E., Warren, M. R., and Mocanu, V. (2010). “Mass spectrometry for proteomics,” in Neuroproteomics. Frontiers in neuroscience. Editor O. Alzate (Boca Raton, FL: CRC Press/Taylor & Francis).
Parkhill, J. M., Hennig, B. J. W., Chapple, I. L. C., Heasman, P. A., and Taylor, J. J. (2000). Association of interleukin-1 gene polymorphisms with early-onset periodontitis. J. Clin. Periodontol. 27 (9), 682–689. doi:10.1034/j.1600-051x.2000.027009682.xPubMed |
Penoni, D. C., Fidalgo, T. K. S., Torres, S. R., Varela, V. M., Masterson, D., Leão, A. T. T., et al. (2017). Bone density and clinical periodontal attachment in postmenopausal women: a systematic review and meta-analysis. J. Dent. Res. 96 (3), 261–269. doi:10.1177/0022034516682017PubMed |
Peres, M. A., Macpherson, L. M. D., Weyant, R. J., Daly, B., Venturelli, R., Mathur, M. R., et al. (2019). Oral diseases: a global public health challenge. Lancet 394 (10194), 249–260. doi:10.1016/S0140-6736(19)31146-8PubMed |
Perri, R., Nares, S., Zhang, S., Barros, S. P., and Offenbacher, S. (2012). MicroRNA modulation in obesity and periodontitis. J. Dent. Res. 91 (1), 33–38. doi:10.1177/0022034511425045PubMed |
Pietruska, M., Pietruski, J., Skurska, A., Bernaczyk, A., Żak, J., Żelazowska, B., et al. (2009). Assessment of aprotinin influence on periodontal clinical status and matrix metalloproteinases 1, 2 and their tissue inhibitors saliva concentrations in patients with chronic periodontitis. Adv. Med. Sci. 54 (2), 239–246. doi:10.2478/v10039-009-0027-2PubMed |
Pitts, N. B., Zero, D. T., Marsh, P. D., Ekstrand, K., Weintraub, J. A., Ramos-Gomez, F., et al. (2017). Dental caries. Nat. Rev. Dis. Primers 3, 17030. doi:10.1038/nrdp.2017.30PubMed |
Qiao, Y., Wang, Z., Li, Y., Han, Y., Zhou, Y., and Cao, X. (2020). Rheumatoid arthritis risk in periodontitis patients: a systematic review and meta-analysis. Joint Bone Spine, S1297-319X(20)30117-2 doi:10.1016/j.jbspin.2020.04.024PubMed |
Radovic, N., Nikolic Jakoba, N., Petrovic, N., Milosavljevic, A., Brkovic, B., and Roganovic, J. (2018). MicroRNA-146a and microRNA-155 as novel crevicular fluid biomarkers for periodontitis in non-diabetic and type 2 diabetic patients. J. Clin. Periodontol. 45 (6), 663–671. doi:10.1111/jcpe.12888PubMed |
Rai, N. P., Kumar, P., Mustafa, S. M., Divakar, D. D., Kheraif, A. A., Ramakrishnaiah, R., et al. (2016). Relation between periodontal status and pre-cancerous condition (oral lichen planus): a pilot study. Adv. Clin. Exp. Med. 25 (4), 763–766. doi:10.17219/acem/59014PubMed |
Renvert, S., Widén, C., and Persson, R. G. (2017). Cytokine and microbial profiles in relation to the clinical outcome following treatment of peri-implantitis. Clin. Oral Implants Res. 28 (9), 1127–1132. doi:10.1111/clr.12927PubMed |
Reynolds, J. J., and Meikle, M. C. (1997). Mechanisms of connective tissue matrix destruction in periodontitis. Periodontol. 2000 14, 144–157. doi:10.1111/j.1600-0757.1997.tb00195.xPubMed |
Rhodin, K., Divaris, K., North, K. E., Barros, S. P., Moss, K., Beck, J. D., et al. (2014). Chronic periodontitis genome-wide association studies. J. Dent. Res. 93 (9), 882–890. doi:10.1177/0022034514544506PubMed |
Ridker, P. M., and Silvertown, J. D. (2008). Inflammation, C-reactive protein, and atherothrombosis. J. Periodontol. 79 (8 Suppl. l), 1544–1551. doi:10.1902/jop.2008.080249PubMed |
Roca-Millan, E., Gonzalez-Navarro, B., Sabater-Recolons, M., Mari-Roig, A., Jane-Salas, E., and Lopez-Lopez, J. (2018). Periodontal treatment on patients with cardiovascular disease: systematic review and meta-analysis. Med. Oral 23 (6), e681–e90. doi:10.4317/medoral.22725PubMed |
Rogus, J., Beck, J. D., Offenbacher, S., Huttner, K., Iacoviello, L., Latella, M. C., et al. (2008). IL1B gene promoter haplotype pairs predict clinical levels of interleukin-1β and C-reactive protein. Hum. Genet. 123 (4), 387–398. doi:10.1007/s00439-008-0488-6PubMed |
Romandini, M., Laforí, A., Romandini, P., Baima, G., and Cordaro, M. (2018). Periodontitis and platelet count: a new potential link with cardiovascular and other systemic inflammatory diseases. J. Clin. Periodontol. 45 (11), 1299–1310. doi:10.1111/jcpe.13004PubMed |
Sabino-Silva, R., Jardim, A. C. G., and Siqueira, W. L. (2020). Coronavirus COVID-19 impacts to dentistry and potential salivary diagnosis. Clin. Oral Invest. 24 (4), 1619–1621. doi:10.1007/s00784-020-03248-xPubMed |
Saito, A., Horie, M., Ejiri, K., Aoki, A., Katagiri, S., Maekawa, S., et al. (2017). MicroRNA profiling in gingival crevicular fluid of periodontitis-a pilot study. FEBS Open Bio. 7 (7), 981–994. doi:10.1002/2211-5463.12238PubMed |
Salminen, A., Gursoy, U. K., Paju, S., Hyvärinen, K., Mäntylä, P., Buhlin, K., et al. (2014). Salivary biomarkers of bacterial burden, inflammatory response, and tissue destruction in periodontitis. J. Clin. Periodontol. 41 (5), 442–450. doi:10.1111/jcpe.12234PubMed |
Sanz, M., Ceriello, A., Buysschaert, M., Chapple, I., Demmer, R. T., Graziani, F., et al. (2018). Scientific evidence on the links between periodontal diseases and diabetes: consensus report and guidelines of the joint workshop on periodontal diseases and diabetes by the International Diabetes Federation and the European Federation of Periodontology. J. Clin. Periodontol. 45 (2), 138–149. doi:10.1111/jcpe.12808PubMed |
Sawle, A. D., Kebschull, M., Demmer, R. T., and Papapanou, P. N. (2016). Identification of master regulator genes in human periodontitis. J. Dent. Res. 95 (9), 1010–1017. doi:10.1177/0022034516653588PubMed |
Schaefer, A. S. (2018). Genetics of periodontitis: discovery, biology, and clinical impact. Periodontol. 2000 78 (1), 162–173. doi:10.1111/prd.12232PubMed |
Schmidl, C., Delacher, M., Huehn, J., and Feuerer, M. (2018). Epigenetic mechanisms regulating T-cell responses. J. Allergy Clin. Immunol. 142 (3), 728–743. doi:10.1016/j.jaci.2018.07.014PubMed |
Schulthess, J., Pandey, S., Capitani, M., Rue-Albrecht, K. C., Arnold, I., Franchini, F., et al. (2019). The short chain fatty acid butyrate imprints an antimicrobial program in macrophages. Immunity 50 (2), 432–445 e7. doi:10.1016/j.immuni.2018.12.018PubMed | | .
Schwarz, F., Derks, J., Monje, A., and Wang, H.-L. (2018). Peri-implantitis. J. Clin. Periodontol. 45 (Suppl. 20), S246–S266. doi:10.1111/jcpe.12954PubMed |
Sehic, A., Tulek, A., Khuu, C., Nirvani, M., Sand, L. P., and Utheim, T. P. (2017). Regulatory roles of microRNAs in human dental tissues. Gene 596, 9–18. doi:10.1016/j.gene.2016.10.009PubMed |
Seker, E., Jong Hwan Sung, J. H., Shuler, M. L., and YarmushYarmush, M. M. L. (2011). Solving medical problems with BioMEMS. IEEE Pulse 2 (6), 51–59. doi:10.1109/MPUL.2011.942928PubMed |
Sen, S., Giamberardino, L. D., Moss, K., Morelli, T., Rosamond, W. D., Gottesman, R. F., et al. (2018). Periodontal disease, regular dental care use, and incident ischemic stroke. Stroke 49 (2), 355–362. doi:10.1161/STROKEAHA.117.018990PubMed |
She, Y.-Y., Kong, X.-B., Ge, Y.-P., Liu, Z.-Y., Chen, J.-Y., Jiang, J.-W., et al. (2020). Periodontitis and inflammatory bowel disease: a meta-analysis. BMC Oral Health 20 (1), 67. doi:10.1186/s12903-020-1053-5PubMed |
Shi, M., Wei, Y., Hu, W., Nie, Y., Wu, X., and Lu, R. (2018). The subgingival microbiome of periodontal pockets with different probing depths in chronic and aggressive periodontitis: a pilot study. Front. Cell. Infect. Microbiol. 8, 124. doi:10.3389/fcimb.2018.00124PubMed |
Shin, M.-S., Kim, Y.-G., Shin, Y. J., Ko, B. J., Kim, S., and Kim, H.-D. (2019). Deep sequencing salivary proteins for periodontitis using proteomics. Clin. Oral Invest. 23 (9), 3571–3580. doi:10.1007/s00784-018-2779-1PubMed |
Sierra, J., Marrugo-Ramírez, J., Rodriguez-Trujillo, R., Mir, M., and Samitier, J. (2020). Sensor-integrated microfluidic approaches for liquid biopsies applications in early detection of cancer. Sensors 20 (5), 1317. doi:10.3390/s20051317PubMed |
Sima, C., and Glogauer, M. (2013). Diabetes mellitus and periodontal diseases. Curr. Diabetes Rep. 13 (3), 445–452. doi:10.1007/s11892-013-0367-yPubMed |
Sims, J. E., and Smith, D. E. (2010). The IL-1 family: regulators of immunity. Nat. Rev. Immunol. 10 (2), 89–102. doi:10.1038/nri2691PubMed |
Sipert, C. R., Morandini, A. C., Dionísio, T. J., Trachtenberg, A. J., Kuo, W. P., and Santos, C. F. (2014). MicroRNA-146a and microRNA-155 show tissue-dependent expression in dental pulp, gingival and periodontal ligament fibroblasts in vitro. J. Oral Sci. 56 (2), 157–164. doi:10.2334/josnusd.56.157PubMed |
Slots, J. (2020). Primer on etiology and treatment of progressive/severe periodontitis: a systemic health perspective. Periodontol. 2000 83 (1), 272–276. doi:10.1111/prd.12325PubMed |
Slots, J., and Rosling, B. G. (1983). Suppression of the periodontopathic microflora in localized juvenile periodontitis by systemic tetracycline. J. Clin. Periodontol. 10 (5), 465–486. doi:10.1111/j.1600-051x.1983.tb02179.xPubMed |
Socransky, S. S., and Haffajee, A. D. (1992). The bacterial etiology of destructive periodontal disease: current concepts. J. Periodontol. 63 (Suppl. 4S), 322–331. doi:10.1902/jop.1992.63.4s.322PubMed |
Socransky, S. S., Haffajee, A. D., Cugini, M. A., Smith, C., and Kent, R. L. (1998). Microbial complexes in subgingival plaque. J. Clin. Periodontol. 25 (2), 134–144. doi:10.1111/j.1600-051x.1998.tb02419.xPubMed |
Sorsa, T., Alassiri, S., Grigoriadis, A., Räisänen, I. T., Pärnänen, P., Nwhator, S. O., et al. (2020). Active MMP-8 (aMMP-8) as a grading and staging biomarker in the periodontitis classification. Diagnostics 10 (2), 61. doi:10.3390/diagnostics10020061PubMed |
Spielmann, N., and Wong, D. (2011). Saliva: diagnostics and therapeutic perspectives. Oral Dis. 17 (4), 345–354. doi:10.1111/j.1601-0825.2010.01773.xPubMed |
Stoecklin-Wasmer, C., Guarnieri, P., Celenti, R., Demmer, R. T., Kebschull, M., and Papapanou, P. N. (2012). MicroRNAs and their target genes in gingival tissues. J. Dent. Res. 91 (10), 934–940. doi:10.1177/0022034512456551PubMed |
Streit, W. R., and Schmitz, R. A. (2004). Metagenomics - the key to the uncultured microbes. Curr. Opin. Microbiol. 7 (5), 492–498. doi:10.1016/j.mib.2004.08.002PubMed |
Sudo, T., Okada, Y., Ozaki, K., Urayama, K., Kanai, M., Kobayashi, H., et al. (2017). Association of NOD2 mutations with aggressive periodontitis. J. Dent. Res. 96 (10), 1100–1105. doi:10.1177/0022034517715432PubMed |
Takagi, R., Sakamoto, E., Kido, J.-i., Inagaki, Y., Hiroshima, Y., Naruishi, K., et al. (2020). S100A9 increases IL-6 and RANKL expressions through MAPKs and STAT3 signaling pathways in osteocyte-like cells. BioMed Res. Int. 2020, 1. doi:10.1155/2020/7149408PubMed |
Tang, Q., Fu, H., Qin, B., Hu, Z., Liu, Y., Liang, Y., et al. (2017). A possible link between rheumatoid arthritis and periodontitis: a systematic review and meta-analysis. Int. J. Periodont. Restor. Dent. 37 (1), 79–86. doi:10.11607/prd.2656PubMed |
Teeuw, W. J., Slot, D. E., Susanto, H., Gerdes, V. E. A., Abbas, F., D’Aiuto, F., et al. (2014). Treatment of periodontitis improves the atherosclerotic profile: a systematic review and meta-analysis. J. Clin. Periodontol. 41 (1), 70–79. doi:10.1111/jcpe.12171PubMed |
Teles, R., Benecha, H. K., Preisser, J. S., Moss, K., Starr, J. R., Corby, P., et al. (2016). Modelling changes in clinical attachment loss to classify periodontal disease progression. J. Clin. Periodontol. 43 (5), 426–434. doi:10.1111/jcpe.12539PubMed |
Teles, R., Moss, K., Preisser, J. S., Genco, R., Giannobile, W. V., Corby, P., et al. (2018). Patterns of periodontal disease progression based on linear mixed models of clinical attachment loss. J. Clin. Periodontol. 45 (1), 15–25. doi:10.1111/jcpe.12827PubMed |
Teng, F., Yang, F., Huang, S., Bo, C., Xu, Z. Z., Amir, A., et al. (2015). Prediction of early childhood caries via spatial-temporal variations of oral microbiota. Cell Host Microbe 18 (3), 296–306. doi:10.1016/j.chom.2015.08.005PubMed |
To, K. K.-W., Tsang, O. T.-Y., Leung, W.-S., Tam, A. R., Wu, T.-C., Lung, D. C., et al. (2020a). Temporal profiles of viral load in posterior oropharyngeal saliva samples and serum antibody responses during infection by SARS-CoV-2: an observational cohort study. Lancet Infect. Dis. 20 (5), 565–574. doi:10.1016/S1473-3099(20)30196-1PubMed |
To, K. K.-W., Tsang, O. T.-Y., Yip, C. C.-Y., Chan, K.-H., Wu, T.-C., Chan, J. M.-C., et al. (2020b). Consistent detection of 2019 novel coronavirus in saliva. Clin. Infect. Dis., 71, 841. doi:10.1093/cid/ciaa149PubMed |
Tonetti, M. S., Greenwell, H., and Kornman, K. S. (2018). Staging and grading of periodontitis: framework and proposal of a new classification and case definition. J. Periodontol. 89 (Suppl. 1), S159–S172. doi:10.1002/JPER.18-0006PubMed |
Vila, T., Rizk, A. M., Sultan, A. S., and Jabra-Rizk, M. A. (2019). The power of saliva: antimicrobial and beyond. PLoS Pathog. 15 (11), e1008058. doi:10.1371/journal.ppat.1008058PubMed |
Wade, W. G. (2011). Has the use of molecular methods for the characterization of the human oral microbiome changed our understanding of the role of bacteria in the pathogenesis of periodontal disease? J. Clin. Periodontol. 38 (Suppl. 11), 7–16. doi:10.1111/j.1600-051X.2010.01679.xPubMed |
Walker, S. J., Van Dyke, T. E., Rich, S., Kornman, K. S., di Giovine, F. S., and Hart, T. C. (2000). Genetic polymorphisms of the IL-1α and IL-1β genes in african-American LJP patients and an african-American control population. J. Periodontol. 71 (5), 723–728. doi:10.1902/jop.2000.71.5.723PubMed |
Wan, J. C. M., Massie, C., Garcia-Corbacho, J., Mouliere, F., Brenton, J. D., Caldas, C., et al. (2017). Liquid biopsies come of age: towards implementation of circulating tumour DNA. Nat. Rev. Canc. 17 (4), 223–238. doi:10.1038/nrc.2017.7PubMed |
Wang, C.-W., Colas, R. A., Dalli, J., Arnardottir, H. H., Nguyen, D., Hasturk, H., et al. (2015). Maresin 1 biosynthesis and proresolving anti-infective functions with human-localized aggressive periodontitis leukocytes. Infect. Immun. 84 (3), 658–665. doi:10.1128/IAI.01131-15PubMed |
Wang, H.-L., Garaicoa-Pazmino, C., Collins, A., Ong, H.-S., Chudri, R., and Giannobile, W. V. (2016). Protein biomarkers and microbial profiles in peri-implantitis. Clin. Oral Implants Res. 27 (9), 1129–1136. doi:10.1111/clr.12708PubMed |
Wang, Y., Springer, S., Mulvey, C. L., Silliman, N., Schaefer, J., Sausen, M., et al. (2015). Detection of somatic mutations and HPV in the saliva and plasma of patients with head and neck squamous cell carcinomas. Sci. Transl. Med. 7 (293), 293ra104, doi:10.1126/scitranslmed.aaa8507PubMed |
Wang, Y., Wang, P., Andrukhov, O., Wang, T., Song, S., Yan, C., et al. (2018). Meta-analysis of the prognostic value of the neutrophil-to-lymphocyte ratio in oral squamous cell carcinoma. J. Oral Pathol. Med. 47 (4), 353–358. doi:10.1111/jop.12688PubMed |
Wong, D. T. W. (2012). Salivaomics. J. Am. Dent. Assoc. 143 (10 Suppl. l), 19S–24S. doi:10.14219/jada.archive.2012.0339PubMed |
Wyllie, A. L., Fournier, J., Casanovas-Massana, A., Campbell, M., Tokuyama, M., Vijayakumar, P., et al. (2020). Saliva is more sensitive for SARS-CoV-2 detection in COVID-19 patients than nasopharyngeal swabs. N. Engl. J. Med. 383, 1283–1286. doi:10.1056/NEJMc2016359PubMed | | .
Xie, Y. f., Shu, R., Jiang, S. y., Liu, D. l., and Zhang, X. l. (2011). Comparison of microRNA profiles of human periodontal diseased and healthy gingival tissues. Int. J. Oral Sci. 3 (3), 125–134. doi:10.4248/IJOS11046PubMed |
Xu, S., Zhang, G., Guo, J. f., and Tan, Y. h. (2020). Associations between osteoporosis and risk of periodontitis: a pooled analysis of observational studies. Oral Dis. doi:10.1111/odi.13531PubMed |
Yao, Q.-W., Zhou, D.-S., Peng, H.-J., Ji, P., and Liu, D.-S. (2014). Association of periodontal disease with oral cancer: a meta-analysis. Tumor Biol. 35 (7), 7073–7077. doi:10.1007/s13277-014-1951-8PubMed |
Ye, L., Jiang, Y., Liu, W., and Tao, H. (2016). Correlation between periodontal disease and oral cancer risk: a meta-analysis. J. Canc. Res. Therapeut. 12, 237–C40. doi:10.4103/0973-1482.200746PubMed |
Yost, S., Duran-Pinedo, A. E., Teles, R., Krishnan, K., and Frias-Lopez, J. (2015). Functional signatures of oral dysbiosis during periodontitis progression revealed by microbial metatranscriptome analysis. Genome Med. 7 (1), 27. doi:10.1186/s13073-015-0153-3PubMed |
Yugi, K., Kubota, H., Hatano, A., and Kuroda, S. (2016). Trans-omics: how to reconstruct biochemical networks across multiple ‘omic’ layers. Trends Biotechnol. 34 (4), 276–290. doi:10.1016/j.tibtech.2015.12.013PubMed |
Zeng, X.-T., Deng, A.-P., Li, C., Xia, L.-Y., Niu, Y.-M., and Leng, W.-D. (2013a). Periodontal disease and risk of head and neck cancer: a meta-analysis of observational studies. PLoS One 8 (10), e79017. doi:10.1371/journal.pone.0079017PubMed |
Zeng, X.-T., Luo, W., Huang, W., Wang, Q., Guo, Y., and Leng, W.-D. (2013b). Tooth loss and head and neck cancer: a meta-analysis of observational studies. PLoS One 8 (11), e79074. doi:10.1371/journal.pone.0079074PubMed |
Zhang, C.-Z., Cheng, X.-Q., Li, J.-Y., Zhang, P., Yi, P., Xu, X., et al. (2016). Saliva in the diagnosis of diseases. Int. J. Oral Sci. 8 (3), 133–137. doi:10.1038/ijos.2016.38PubMed |
Zhang, D., Hou, J., Wu, Y., Liu, Y., Li, R., Xu, T., et al. (2019). Distinct gene expression characteristics in epithelial cell-porphyromonas gingivalis interactions by integrating transcriptome analyses. Int. J. Med. Sci. 16 (10), 1320–1327. doi:10.7150/ijms.33728PubMed |
Zhang, Q., Chen, B., Zhu, D., and Yan, F. (2016). Biomarker levels in gingival crevicular fluid of subjects with different periodontal conditions: a cross-sectional study. Arch. Oral Biol. 72, 92–98. doi:10.1016/j.archoralbio.2016.08.020PubMed |
Zhang, S., Barros, S. P., Niculescu, M. D., Moretti, A. J., Preisser, J. S., and Offenbacher, S. (2010). Alteration of PTGS2 promoter methylation in chronic periodontitis. J. Dent. Res. 89 (2), 133–137. doi:10.1177/0022034509356512PubMed |
Zhang, S., Crivello, A., Offenbacher, S., Moretti, A., Paquette, D. W., and Barros, S. P. (2010). Interferon-gamma promoter hypomethylation and increased expression in chronic periodontitis. J. Clin. Periodontol. 37 (11), 953–961. doi:10.1111/j.1600-051X.2010.01616.xPubMed |
Zhu, H., Fohlerová, Z., Pekárek, J., Basova, E., and Neužil, P. (2020). Recent advances in lab-on-a-chip technologies for viral diagnosis. Biosens. Bioelectron. 153, 112041. doi:10.1016/j.bios.2020.112041PubMed |
Ziukaite, L., Slot, D. E., and Van der Weijden, F. A. (2018). Prevalence of diabetes mellitus in people clinically diagnosed with periodontitis: a systematic review and meta-analysis of epidemiologic studies. J. Clin. Periodontol. 45 (6), 650–662. doi:10.1111/jcpe.12839PubMed |
Keywords: periodontal diseases/periodontitis, patient stratification, precision medicine, biotechnology, saliva, biomarkers
Citation: Steigmann L, Maekawa S, Sima C, Travan S, Wang C-W and Giannobile WV (2020) Biosensor and Lab-on-a-chip Biomarker-identifying Technologies for Oral and Periodontal Diseases. Front. Pharmacol. 11:588480. doi: 10.3389/fphar.2020.588480
Received: 28 July 2020; Accepted: 23 September 2020;
Published: 09 November 2020.
Edited by:
Dorina Lauritano, University of Milano-Bicocca, ItalyReviewed by:
Antonio Romano, University of Campania Luigi Vanvitelli, ItalyCopyright © 2020 Steigmann, Maekawa, Sima, Travan, Wang and Giannobile. This is an open-access article distributed under the terms of the Creative Commons Attribution License (CC BY). The use, distribution or reproduction in other forums is permitted, provided the original author(s) and the copyright owner(s) are credited and that the original publication in this journal is cited, in accordance with accepted academic practice. No use, distribution or reproduction is permitted which does not comply with these terms.
*Correspondence: William V. Giannobile, V2lsbGlhbV9HaWFubm9iaWxlQGhzZG0uaGFydmFyZC5lZHU=
Disclaimer: All claims expressed in this article are solely those of the authors and do not necessarily represent those of their affiliated organizations, or those of the publisher, the editors and the reviewers. Any product that may be evaluated in this article or claim that may be made by its manufacturer is not guaranteed or endorsed by the publisher.
Research integrity at Frontiers
Learn more about the work of our research integrity team to safeguard the quality of each article we publish.