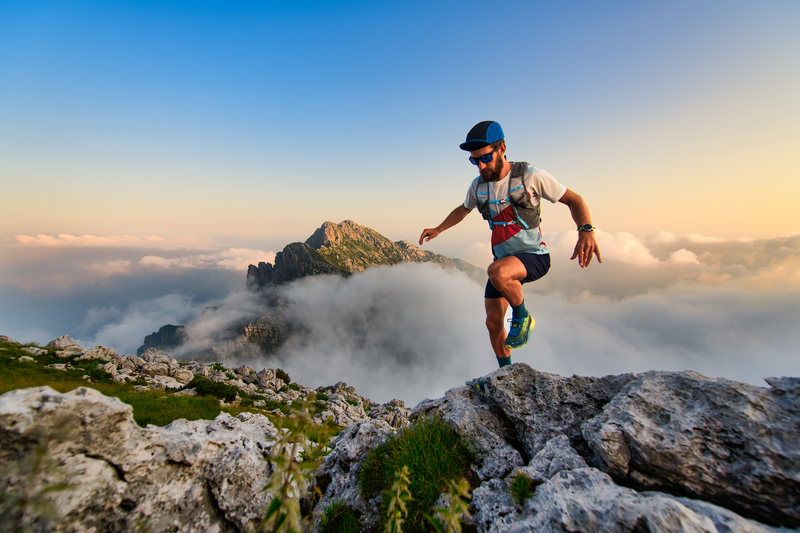
94% of researchers rate our articles as excellent or good
Learn more about the work of our research integrity team to safeguard the quality of each article we publish.
Find out more
ORIGINAL RESEARCH article
Front. Pharmacol. , 11 November 2020
Sec. Pharmacology of Anti-Cancer Drugs
Volume 11 - 2020 | https://doi.org/10.3389/fphar.2020.579768
This article is part of the Research Topic Target Discovery for Anticancer Therapy Facilitated by Artificial Intelligence View all 16 articles
IDH1 mutations occur in about 20–30% of gliomas and are a promising target for the treatment of cancer. In the present study, the performance of aIDH1R132H was verified via glide-docking-based virtual screening. On the basis of the two crystal structures (5TQH and 6B0Z) with the best discriminating ability to identify IDH1R132H inhibitors from a decoy set, a docking-based virtual screening strategy was employed for identifying new IDH1R132H inhibitors. In the end, 57 structurally diverse compounds were reserved and evaluated through experimental tests, and 10 of them showed substantial activity in targeting IDH1R132H (IC50 < 50 μM). Molecular docking technology showed that L806-0255, V015-1671, and AQ-714/41674992 could bind to the binding pocket composed of hydrophobic residues. These findings indicate that L806-0255, V015-1671, and AQ-714/41674992 have the potential as lead compounds for the treatment of IDH1-mutated gliomas through further optimization.
Isocitrate dehydrogenase 1 (IDH1) is a critical metabolic enzyme involved in the tricarboxylic acid cycle. This enzyme catalyzes the oxidative decarboxylation of isocitrate acid to aketoglutaric (a-KG) in an NADP+-dependent manner by using divalent magnesium ion (Jiao et al., 2016), which is related to the progression of various tumors, including acute myeloid leukemia, gliomas, and other solid tumors (Yan and Reitman, 2010; Yen et al., 2016).
Somatic mutations of IDH1 have been frequently identified in many types of cancer, including approximately 80% of grade II-III gliomas, nearly 45% of secondary glioblastoma multiforme (GBM), and 33%-50% of adult primitive neuroectodermal tumors (Dang et al., 2009; Wang et al., 2013). IDH1 mutations have also been discovered in other cancers, such as colorectal cancer (Xu et al., 2011), acute myeloid leukemia (Parsons et al., 2008), and prostate cancer (Hartmann et al., 2009). Key amino acid residue Arg132 is the most common mutation in IDH1, which is located in the catalytic pocket (Dang et al., 2009). Specific mutations belong to heterozygous missense mutations and lead to a new form of IDH1 catalytic activity, which convert α-KG into an oncometabolite D2-hydroxyglutarate (Dang et al., 2009). The oncometabolite (D2-HG) is associated with tumorigenesis, which impairs hematopoietic differentiation and promotes leukemia by inducing the hypermethylation of histone and chromatin and preventing cell differentiation (Figueroa et al., 2010; Xu et al., 2011). Due to the IDH1 mutation, high levels of D2-HG are created that promote the occurrence and development of cancers, such as gliomas (Parsons et al., 2008) and acute myeloid leukemia (Mardis et al., 2009). Therefore, although the contribution of IDH1 mutants to carcinogenic properties has yet to be elucidated, IDH1 mutants have become therapeutic targets for cancer, especially AML.
Mutant IDH1 has become a very attractive therapeutic target in the field of antitumor drug discovery, and several pharmaceutical companies have attempted to develop novel small molecule inhibitors against mutant IDH1. So far, several small molecule inhibitors targeting mutant IDH1 enzymes have been developed (see Figure 1) (Rohle et al., 2013; Davis et al., 2014; Deng et al., 2015; Kim et al., 2015; Okoye-Okafor et al., 2015; Law et al., 2016; Chaturvedi et al., 2017; Xie et al., 2017; Popovici-Muller et al., 2018; Nakagawa et al., 2019; Caravella et al., 2020; Konteatis et al., 2020). Some of these have been studied in various preclinical models, and some are currently being evaluated in phase I/II clinical studies for different tumor pathologies with IDH1 enzyme mutations. AG-120 as the only mutant IDH1 inhibitor in clinic approved by the FDA that has shown encouraging clinical benefits with a total overall response rate of 42% for advanced hematological malignancies (Foran et al., 2019). In light of these encouraging finding, we employed docking-based virtual screening to identify active hits with novel skeleton for targeting mutant IDH1.
Structure-based virtual screening is now widely used in early-stage drug discovery (Sheisi et al., 2019), and has been applied to the discovery of IDH1 inhibitors. To date, there have been several attempts to identify potential IDH1 inhibitors by using structure-based virtual screening in terms of the reported crystal structures of the IDH1 complex (Zou et al., 2016; Zheng et al., 2017; Zou et al., 2018). In 2016, by using a docking-based virtual screening strategy (PDB: 4UMX), Zou et al. identified a series of IDH1 inhibitor FX-03 with IC50 values of 55.50 μM and 68.38 μM in HEK-293T cells transfected with IDH1 R132H and IDH1 R132C, respectively (Zou et al., 2016). Importantly, FX-03 exhibited significant selectivity between the IDH1WT and IDH1R132H mutants. In 2017, Zheng et al. discovered a natural product, clomifene, as an effective inhibitor against the IDH1R132H mutant with a Kd value of 18.45 μM by using docking-based virtual screening (PDB: 4UMX) (Zheng et al., 2017). They also proved that clomifene selectively inhibits mutant IDH1 activities in vitro and in vivo models. It should be noted that, although these studies have identified several IDH1R132H inhibitors, they used the same IDH1R132H crystal structure in structure-based virtual screening. Considering the difference in binding mode after the binding of various ligands, comparing the virtual screening capabilities of different IDH1R132H crystal structures based on docking-based virtual screening appears to a more reasonable strategy to discover potential IDH1R132H inhibitors.
In the present study, the performance of docking-based virtual screening for nine crystal structures of IDH1R132H were compared through a combination of docking power and screening power. Two best performing IDH1R132H complexes were employed to identify potential IDH1R132H inhibitors with diverse structures from ChemDiv (http://www.chemdiv.com) and Specs (http://www.specs.net) databases. Followed by further examination and verification, a series of compounds with novel skeleton were addressed and could be used as IDH1R132H inhibitors. The overall workflow was shown in Figure 2.
The crystal structures of the IDH1R132H in complex with an inhibitor were downloaded from the PDB database (http://www.rcsb.org), including 4UMX, 5L57, 5L58, 5LGE, 5SUN, 5SVF, 5TQH, 6ADG, and 6B0Z. For each complex, the Protein Preparation Wizard module in Schrödinger 2015 (Schrödinger, LLC, New York, NY, 2015) was applied to add hydrogen and missing side chains, remove all water molecules, assign protonation states and partial charges through OPLS2005 force field (Jorgensen et al., 1996), and minimize all heavy atoms until the root-mean-square deviation (RMSD) was reached ≤0.3 Å.
To evaluate the virtual screening capability of different crystal structures, 423 actives were directly extracted from the PubChem database and served as a validation data set (https://pubchem.ncbi.nlm.nih.gov/bioassay/1344832#section=Top), and their decoys, generated by DUD•E (Mysinger et al., 2012), were considered as a decoy data set. In total, 23,900 decoys were generated.
In order to discover the favorable crystal structure for virtual screening, the docking performance of each IDH1R132H structure was systematically evaluated. All actives and decoys were preprepared using the LigPrep (LigPrep, Schrödinger, LLC, New York, NY, 2015) module in the Schrödinger package. The possible ionized states of each compound were calculated by using Epik (Shelley et al., 2007) at pH= 7.0 ± 2.0. The chirality of the IDH1R132H inhibitors with 3D structures were preserved, while the chirality of the decoys was determined from 3D structures based on the different combinations. The stereoisomers for each ligand generated, at most, 32, and the other parameters were set to default values. Subsequently, a grid box of each complex was generated by using the Receptor Grid Generation module of Schrödinger software, which was centered at the native ligand of the complex and defined as a similar size to the native ligand space. Finally, all chemicals in the validation set and decoy set were docked into the binding site of each IDH1R132H complex in turn and evaluated by using the standard precision (SP) and extra precision (XP) scoring function of Glide. In order to choose the best crystal structure of IDH1R132H for virtual screening, the enrichment factor (EF) (Halgren et al., 2004) was used to evaluate the virtual screening capability of each model, which was defined as the following Equation:
where Hitsset is the number of actives in the selected subset n of the ranked database and Hitsall is the total number of actives in the database. The model with the highest EF value was reserved and used to screen potential IDH1R132H inhibitors.
All compounds in the ChemDiv and Specs database were first preprocessed according to the method of the above step, and then screened by docking-based virtual screening against two IDH1R132H crystal structures (PDB ID: 5TQH and 6B0Z). After the possible ionized states and tautomer were calculated at pH=7.0 ± 2.0 by using Epik module, the chirality of each compound was determined from 3D structures; the stereoisomer for each ligand generated, at most, 32. The final virtual screening library was generated to include approximately 2 million compounds, and then initially filtered by Lipinski’s Rule, removing ligands with reactive functional groups. Finally, docking-based virtual screening was employed by use of the high throughput virtual screening (HTVS) scoring function, SP scoring function, and XP scoring function of Glide in sequence. In the screening process at each step, 10% of the best compounds were reserved for further analysis.
The reserved compounds after docking-based virtual screening were structurally clustered into 30 clusters by using K-means clustering on the MACCS structural keys in Canvas (Canvas, Schrödinger, LLC, New York, NY, 2015), and the compound in each cluster with the lowest docking score was selected. In the end, 60 chemicals were eventually submitted to purchase from Topscience Co., Ltd (https://www.tsbiochem.com).
The primary assay was carried out in 10 μL of base buffer (10 mM MgCl2, 20 mM Tris pH7.5, 150 mM NaCl, 0.05% (w/v) bovine serum albumin) containing 2.5 μL of the test compound, 5 μL of an enzyme solution (0.3 ng/μL mutant IDH1R132H), and 2.5 μL of a substrate solution (4 mM α-KG, 16 μM NADPH). This assay added into a 384-well blank plate and then incubated at room temperature for 60 min. The secondary assay, with 5 μL of base buffer containing 15 μM resazurin and 0.01 unit diaphorase, was added to the entire plate and incubated at room temperature for 10 min. Florescence was read on a SYNERGYH1 microplate reader (BioTek) at Ex 540 Em 590. Curve fitting for dose response IC50 was done using GraphPad Prism.
As a significant indicator of the docking reliability, docking power was used to reveal the binding pose of the experiment between small molecules and proteins, which was mainly evaluated after redocking with the RMSD value of the docking pose and native pose of the small molecule in the IDH1R132H complex. For each IDH1R132H complex, after the native inhibitor was separated from the corresponding complex and preprepared, it was redocked into the original binding site. The RMSD value between the native conformation of the inhibitor and the docked pose for each crystal structure was respectively computed, and RMSD ≤ 2.0 Å served as the evaluation standard to verify the docking reliability. It can be seen from Table 1 that Glide docking could identify the near-native pose of most inhibitors in IDH1R132H crystal structures by using the XP or SP scoring function in Glide.
Table 1 The summary of the docking power of molecular docking in glide for nine IDH1R132H crystal structures.
Next, screening power of glide docking was used to identify the reported inhibitors from the decoys in nine IDH1R132H complexes, and these were compared and calculated. In contrast with the docking power of glide docking, the screening power of each crystal structure is a more important index for the docking-based virtual screening process. Herein, we performed student’s t test to evaluate the significant difference between the means of the two distributions of the Glide XP or SP scores for the known actives and decoys. It can be seen from Table 2 that molecular docking of Glide can efficiently discriminate the IDH1R132H inhibitors from the decoys in nine complexes of IDH1R132H based on the relatively low p value. The area under the receiver operating characteristic curve (AUC-ROC), EF, and Robust Initial Enhancement (RIE) were also employed to comprehensively evaluate the screening capabilities of each crystal structure. As shown in Figure 3, the best screening power (p value = 2.42x10-135, AUC-ROC=0.96, and RIE= 12.88) was acquired by using SP scoring function and 6B0Z was reserved as the screening template. However, 5TQH exhibited the best screening power (p value = 6.09x10-102, AUC-ROC=0.96, and RIE= 15.76) in XP scoring function, which was also retained as a screening complex. Our results suggest that that it is necessary to compare the performance of different complexes in the process of virtual screening.
Table 2 The summary of the screening power of molecular docking in glide for nine IDH1R132H crystal structures for validation set.
Figure 3 The distributions of Glide docking scores of validation sets for two IDH1R132H crystal structures with the best screening power.
The overall workflow of structure-based virtual screening was shown in Figure 2. The Specs and ChemDiv database, which consisted of more than 2,100,000 compounds, have been used for virtual screening of small molecule databases. Firstly, Lipinski’s rules of five was employed to filter compounds that did not meet the criteria, and then these compounds containing PAINS substructures were also removed. A total of 1.46 million compounds were retained. According to the MACCS structural fingerprint, residual chemicals were structurally clustered in 30 clusters via K-means clustering in Canvas, and the chemical with the lowest docking score in each cluster was retained was retained (see Table 3). Finally, a total of 57 chemicals were purchased and tested based on the docking-based virtual screening on two IDH1 complex (5TQH and 6B0Z).
To verify the inhibitory activity of screening compounds targeting IDH1R132H, enzyme activity assay was performed. As shown in Figure 4, we found that 12 compounds (7, 8, 14, 23, 25, 33, 44, 46, 47, 52, 53, and 57) exhibited over 50% inhibition at 50 μM. These 12 ligands were submitted to determine the IC50. It can be seen from Table 4 that 10 of them show IC50 ≤ 50 μM. Molecular structures of the 10 selected compounds of IDH1R132H are exhibited in Figure 5. The enzymatic curves and docking score for these 10 compounds against IDH1R132H are depicted in Figure 6. Tanimoto coefficient (Tc) (Willett and Winterman, 1986; Willett et al., 1986), in terms of the ECFP4 fingerprint, was calculated to compare the structural similarity between 10 compounds and reported inhibitors. As shown in Figure S1, we can find that putative hits have low similarity with reported inhibitors (Tc < 0.2). Therefore, these compounds are structurally new and have the potential to be promising leads for further optimizations.
Figure 4 Inhibitory activity of the 57 candidates at 50μM. The bars indicate the inhibitory activity of chemicals targeting IDH1R132H. AG-120 at 100 nM was used as the positive control.
Figure 5 Molecular structures of the 10 selected IDH1R132H inhibitors by using based-docking virtual screening.
The binding pocket of IDH1 lies on the dimer interface and most of the reported compounds have been shown to bind to this allosteric site. In order to gain insight into the structural basis of the identified IDH1R132H inhibitor, the binding mode of the three compounds was compared with AG-120. As shown in Figure 7, three molecules that could be docked into the binding pocket consisted of hydrophobic residues in a similar manner to AG-120, and formed intermolecular hydrogen bonds with key residues, which stabilized the complex. L806-0255 and V015-1671 form a key hydrogen bond with ILE128, which is consistent with AG-120. In addition, V015-1671 and AQ-714/41674992 also form a key hydrogen bond with ALA111. Moreover, the hydrophobic contacts formed between surrounded residues, such as VAL276, SER278, SER287, ILE128, PRO118, and compounds also contribute to enhanced binding of the small molecule inhibitor to IDH1 R132H. Therefore, the above results suggested that L806-0255, V015-1671, and AQ-714/41674992 could bind to IDH1R132H.
Figure 7 Binding mode of identified IDH1R132H inhibitor and AG-120. (A) L806-0255. (B) V015-1671. (C) AQ-714/41674992. (D)AG-120.
In the present work, we first verified the performance of IDH1R132H by using glide-docking-based virtual screening and discovered two crystal structures with the most credible screening ability. Based on the best performing crystal structure, docking-based virtual screening was performed to identify new IDH1R132H inhibitors. A total of 57 potential hits were purchased and their activity against IDH1R132H was addressed, and 10 of them exhibited anti-IDH1R132H activity.
All datasets presented in this study are included in the article/Supplementary Material.
HG, XY, and EL conceived this study and revised the manuscript. YW, ST, HL, RJ, and XL carried out the experiments and analyzed the experimental data. YW and XY wrote the manuscript. All authors reviewed the manuscript. All authors contributed to the article and approved the submitted version.
This work was supported by the National Natural Science Foundation of China (82003653), the Shaanxi University of Chinese Medicine (Project No. 2020XG01), the Subject Innovation Team of Shaanxi University of Chinese Medicine (Project No. 2019-PY02), and Macau Science and Technology Development Fund (Project Nos. 005/2014/AMJ, 082/2015/A3, 046/2016/A2 and 086/2015/A3).
The authors declare that the research was conducted in the absence of any commercial or financial relationships that could be construed as a potential conflict of interest.
The Supplementary Material for this article can be found online at: https://www.frontiersin.org/articles/10.3389/fphar.2020.579768/full#supplementary-material
Supplementary Figure 1 | The Tanimoto coefficient matrix based on ECFP4 fingerprint of identified IDH1R132H inhibitor vs reported IDH1R132H inhibitor.
Caravella, J. A., Lin, J., Diebold, R. B., Campbell, A.-M., Ericsson, A., Gustafson, G., et al. (2020). Structure-Based Design and Identification of FT-2102 (Olutasidenib), a Potent Mutant-Selective IDH1 Inhibitor. J. Med. Chem. 63 (4), 1612–1623. doi: 10.1021/acs.jmedchem.9b01423
Chaturvedi, A., Herbst, L., Pusch, S., Klett, L., Goparaju, R., Stichel, D., et al. (2017). Pan-mutant-IDH1 inhibitor BAY1436032 is highly effective against human IDH1 mutant acute myeloid leukemia in vivo. Leukemia 31 (10), 2020–2028. doi: 10.1038/leu.2017.46
Dang, L., White, D. W., Gross, S., Bennett, B. D., Bittinger, M. A., Driggers, E. M., et al. (2009). Cancer-associated IDH1 mutations produce 2-hydroxyglutarate. Nature 462, 739. doi: 10.1038/nature08617
Davis, M. I., Gross, S., Shen, M., Straley, K. S., Pragani, R., Lea, W. A., et al. (2014). Biochemical, cellular, and biophysical characterization of a potent inhibitor of mutant isocitrate dehydrogenase IDH1. J. Biol. Chem. 289 (20), 13717–13725. doi: 10.1074/jbc.M113.511030
Deng, G., Shen, J., Yin, M., McManus, J., Mathieu, M., Gee, P., et al. (2015). Selective inhibition of mutant isocitrate dehydrogenase 1 (IDH1) via disruption of a metal binding network by an allosteric small molecule. J. Biol. Chem. 290 (2), 762–774. doi: 10.1074/jbc.M114.608497
Figueroa, M. E., Abdel-Wahab, O., Lu, C., Ward, P. S., Patel, J., Shih, A., et al. (2010). Leukemic IDH1 and IDH2 mutations result in a hypermethylation phenotype, disrupt TET2 function, and impair hematopoietic differentiation. Cancer Cell 18 (6), 553–567. doi: 10.1016/j.ccr.2010.11.015
Foran, J. M., DiNardo, C. D., Watts, J. M., Stein, E. M., De Botton, S., Fathi, A. T., et al. (2019). Ivosidenib (AG-120) in Patients with IDH1-Mutant Relapsed/Refractory Myelodysplastic Syndrome: Updated Enrollment of a Phase 1 Dose Escalation and Expansion Study. Blood 134 (Supplement_1), 4254–4254. doi: 10.1182/blood-2019-123946
Halgren, T. A., Murphy, R. B., Friesner, R. A., Beard, H. S., Frye, L. L., Pollard, W. T., et al. (2004). Glide: A New Approach for Rapid, Accurate Docking and Scoring. 2. Enrichment Factors in Database Screening. J. Med. Chem. 47 (7), 1750–1759. doi: 10.1021/jm030644s
Hartmann, C., Meyer, J., Balss, J., Capper, D., Mueller, W., Christians, A., et al. (2009). Type and frequency of IDH1 and IDH2 mutations are related to astrocytic and oligodendroglial differentiation and age: a study of 1,010 diffuse gliomas. Acta Neuropathol. 118 (4), 469–474. doi: 10.1007/s00401-009-0561-9
Jiao, C., Jie, Y., Peng, C. (2016). The Evolving Landscape in the Development of Isocitrate Dehydrogenase Mutant Inhibitors. Mini Rev. Med. Chem. 16 (16), 1344–1358. doi: 10.2174/1389557516666160609085520
Jorgensen, W. L., Maxwell, D. S., Tirado-Rives, J. (1996). Development and Testing of the OPLS All-Atom Force Field on Conformational Energetics and Properties of Organic Liquids. J. Am. Chem. Soc. 118 (45), 11225–11236. doi: 10.1021/ja9621760
Kim, H. J., Fei, X., Cho, S. C., Choi, B. Y., Ahn, H. C., Lee, K., et al. (2015). Discovery of alpha-mangostin as a novel competitive inhibitor against mutant isocitrate dehydrogenase-1. Bioorg. Med. Chem. Lett. 25 (23), 5625–5631. doi: 10.1016/j.bmcl.2015.10.034
Konteatis, Z., Artin, E., Nicolay, B., Straley, K., Padyana, A. K., Jin, L., et al. (2020). Vorasidenib (AG-881): A First-in-Class, Brain-Penetrant Dual Inhibitor of Mutant IDH1 and 2 for Treatment of Glioma. ACS Med. Chem. Lett. 11 (2), 101–107. doi: 10.1021/acsmedchemlett.9b00509
Law, J. M., Stark, S. C., Liu, K., Liang, N. E., Hussain, M. M., Leiendecker, M., et al. (2016). Discovery of 8-Membered Ring Sulfonamides as Inhibitors of Oncogenic Mutant Isocitrate Dehydrogenase 1. ACS Med. Chem. Lett. 7 (10), 944–949. doi: 10.1021/acsmedchemlett.6b00264
Mardis, E. R., Ding, L., Dooling, D. J., Larson, D. E., McLellan, M. D., Chen, K., et al. (2009). Recurring mutations found by sequencing an acute myeloid leukemia genome. N Engl. J. Med. 361 (11), 1058–1066. doi: 10.1056/NEJMoa0903840
Mysinger, M. M., Carchia, M., Irwin, J. J., Shoichet, B. K. (2012). Directory of Useful Decoys, Enhanced (DUD-E): Better Ligands and Decoys for Better Benchmarking. J. Med. Chem. 55 (14), 6582–6594. doi: 10.1021/jm300687e
Nakagawa, M., Nakatani, F., Matsunaga, H., Seki, T., Endo, M., Ogawara, Y., et al. (2019). Selective inhibition of mutant IDH1 by DS-1001b ameliorates aberrant histone modifications and impairs tumor activity in chondrosarcoma. Oncogene 38 (42), 6835–6849. doi: 10.1038/s41388-019-0929-9
Okoye-Okafor, U. C., Bartholdy, B., Cartier, J., Gao, E. N., Pietrak, B., Rendina, A. R., et al. (2015). New IDH1 mutant inhibitors for treatment of acute myeloid leukemia. Nat. Chem. Biol. 11 (11), 878–886. doi: 10.1038/nchembio.1930
Parsons, D. W., Jones, S., Zhang, X., Lin, J. C.-H., Leary, R. J., Angenendt, P., et al. (2008). An integrated genomic analysis of human glioblastoma multiforme. Science (New York N.Y.) 321 (5897), 1807–1812. doi: 10.1126/science.1164382
Popovici-Muller, J., Lemieux, R. M., Artin, E., Saunders, J. O., Salituro, F. G., Travins, J., et al. (2018). Discovery of AG-120 (Ivosidenib): A First-in-Class Mutant IDH1 Inhibitor for the Treatment of IDH1 Mutant Cancers. ACS Med. Chem. Lett. 9 (4), 300–305. doi: 10.1021/acsmedchemlett.7b00421
Rohle, D., Popovici-Muller, J., Palaskas, N., Turcan, S., Grommes, C., Campos, C., et al. (2013). An inhibitor of mutant IDH1 delays growth and promotes differentiation of glioma cells. Science 340 (6132), 626–630. doi: 10.1126/science.1236062
12Sheisi, F., Carolina, G. O., Harold, H. F., Carlos, M. R. S. A. (2019). Virtual Screening Techniques in Drug Discovery: Review and Recent Applications. Curr. Top. Med. Chem. 19 (19), 1751–1767. doi: 10.2174/1568026619666190816101948
Shelley, J. C., Cholleti, A., Frye, L. L., Greenwood, J. R., Timlin, M. R., Uchimaya, M. (2007). Epik: a software program for pKaprediction and protonation state generation for drug-like molecules. J. Comput. Aided Mol. Des. 21 (12), 681–691. doi: 10.1007/s10822-007-9133-z
Wang, F., Travins, J., DeLaBarre, B., Penard-Lacronique, V., Schalm, S., Hansen, E., et al. (2013). Targeted Inhibition of Mutant IDH2 in Leukemia Cells Induces Cellular Differentiation. Science 340 (6132), 622. doi: 10.1126/science.1234769
Willett, P., Winterman, V. (1986). A Comparison of Some Measures for the Determination of Inter-Molecular Structural Similarity Measures of Inter-Molecular Structural Similarity. Quant. Structure-Activity Relat. 5 (1), 18–25. doi: 10.1002/qsar.19860050105
Willett, P., Winterman, V., Bawden, D. (1986). Implementation of nearest-neighbor searching in an online chemical structure search system. J. Chem. Inf. Comput. Sci. 26 (1), 36–41. doi: 10.1021/ci00049a008
Xie, X., Baird, D., Bowen, K., Capka, V., Chen, J., Chenail, G., et al. (2017). Allosteric Mutant IDH1 Inhibitors Reveal Mechanisms for IDH1 Mutant and Isoform Selectivity. Structure 25 (3), 506–513. doi: 10.1016/j.str.2016.12.017
Xu, W., Yang, H., Liu, Y., Yang, Y., Wang, P., Kim, S.-H., et al. (2011). Oncometabolite 2-hydroxyglutarate is a competitive inhibitor of α-ketoglutarate-dependent dioxygenases. Cancer Cell 19 (1), 17–30. doi: 10.1016/j.ccr.2010.12.014
Yan, H., Reitman, Z. J. (2010). Isocitrate Dehydrogenase 1 and 2 Mutations in Cancer: Alterations at a Crossroads of Cellular Metabolism. J. Natl. Cancer Inst. 102 (13), 932–941. doi: 10.1093/jnci/djq187
Yen, K., Dang, L., Attar, E. C. (2016). IDH mutations in cancer and progress toward development of targeted therapeutics. Ann. Oncol. 27 (4), 599–608. doi: 10.1093/annonc/mdw013
Zheng, M., Sun, W., Gao, S., Luan, S., Li, D., Chen, R., et al. (2017). Structure based discovery of clomifene as a potent inhibitor of cancer-associated mutant IDH1. Oncotarget 8 (27), 44255–44265. doi: 10.18632/oncotarget.17464
Zou, F., Pusch, S., Eisel, J., Ma, T., Zhu, Q., Deng, D., et al. (2016). Identification of a novel selective inhibitor of mutant isocitrate dehydrogenase 1 at allosteric site by docking-based virtual screening. RSC Adv. 6 (99), 96735–96742. doi: 10.1039/c6ra21617j
Keywords: IDH1, gliomas, molecular docking, virtual screening, docking-based virtual screening
Citation: Wang Y, Tang S, Lai H, Jin R, Long X, Li N, Tang Y, Guo H, Yao X and Leung EL-H (2020) Discovery of Novel IDH1 Inhibitor Through Comparative Structure-Based Virtual Screening. Front. Pharmacol. 11:579768. doi: 10.3389/fphar.2020.579768
Received: 03 July 2020; Accepted: 14 September 2020;
Published: 11 November 2020.
Edited by:
Feng Zhu, Zhejiang University, ChinaReviewed by:
Wenjin Li, Shenzhen University, ChinaCopyright © 2020 Wang, Tang, Lai, Jin, Long, Li, Tang, Guo, Yao and Leung. This is an open-access article distributed under the terms of the Creative Commons Attribution License (CC BY). The use, distribution or reproduction in other forums is permitted, provided the original author(s) and the copyright owner(s) are credited and that the original publication in this journal is cited, in accordance with accepted academic practice. No use, distribution or reproduction is permitted which does not comply with these terms.
*Correspondence: Hui Guo, Z3VvaHVpQHNudGNtLmVkdS5jbg==; Xiaojun Yao, eGp5YW9AbXVzdC5lZHUubW8=; Elaine Lai-Han Leung, bGhsZXVuZ0BtdXN0LmVkdS5tbw==
Disclaimer: All claims expressed in this article are solely those of the authors and do not necessarily represent those of their affiliated organizations, or those of the publisher, the editors and the reviewers. Any product that may be evaluated in this article or claim that may be made by its manufacturer is not guaranteed or endorsed by the publisher.
Research integrity at Frontiers
Learn more about the work of our research integrity team to safeguard the quality of each article we publish.