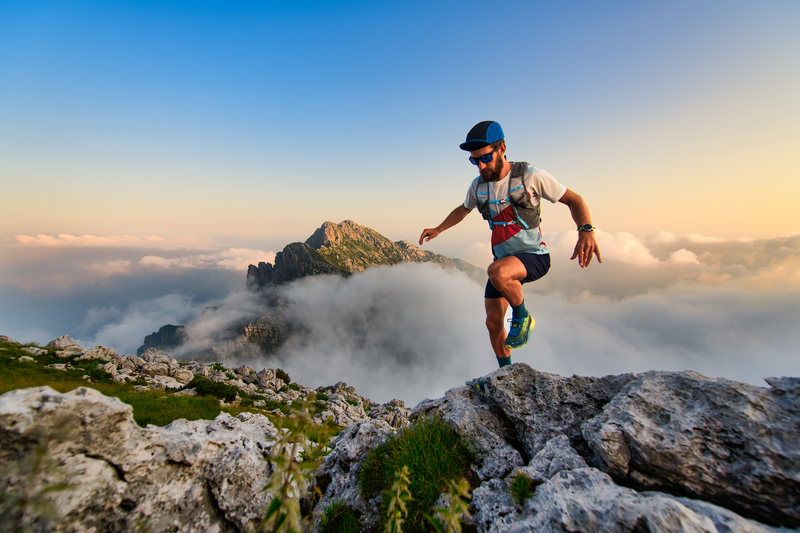
94% of researchers rate our articles as excellent or good
Learn more about the work of our research integrity team to safeguard the quality of each article we publish.
Find out more
ORIGINAL RESEARCH article
Front. Pharmacol. , 21 January 2021
Sec. Pharmacogenetics and Pharmacogenomics
Volume 11 - 2020 | https://doi.org/10.3389/fphar.2020.575765
This article is part of the Research Topic The Potential of Machine-learning in Pharmacogenetics, Pharmacogenomics and Pharmacoepidemiology: Volume II View all 5 articles
Acute type A aortic dissection (ATAAD) is a life-threatening disease. The understanding of its pathogenesis and treatment approaches remains unclear. In the present work, differentially expressed genes (DEGs) from two ATAAD datasets GSE52093 and GSE98770 were filtered. Transcription factor TEAD4 was predicted as a key modulator in protein-protein interaction (PPI) network. Weighted correlation network analysis (WGCNA) identified five modules in GSE52093 and four modules in GSE98770 were highly correlated with ATAAD. 71 consensus DEGs of highly correlated modules were defined and functionally annotated. L1000CDS2 was executed to predict drug for drug repositioning in ATAAD treatment. Eight compounds were filtered as potential drugs. Integrative analysis revealed the interaction network of five differentially expressed miRNA and 16 targeted DEGs. Finally, master DEGs were validated in human ATAAD samples and AD cell model in vitro. TIMP3 and SORBS1 were downregulated in ATAAD samples and AD cell model, while PRUNE2 only decreased in vitro. Calcium channel blocker and glucocorticoid receptor agonist might be potential drugs for ATAAD. The present study offers potential targets and underlying molecular mechanisms ATAAD pathogenesis, prevention and drug discovery.
Aortic dissection (AD) is a potentially critical break in the lining of the main arterial outflow from the heart. It comprises 85%–95% of all acute aortic syndromes (Bossone et al., 2018). The morbidity of AD is increasing in the last 16 years (Olsson et al., 2006), and current studies in different countries have found that the incidence of AD occurs in 3–6 cases per 100,000 people per year (Nienaber and Clough, 2015; Mussa et al., 2016). AD can be classified using Stanford or DeBakey systems. The Stanford system divides dissections according to whether the ascending aorta is involved (type A) or not (type B) (Golledge and Eagle, 2008). In the International Registry of Acute Aortic Dissection series, acute type A aortic dissection (ATAAD) is more common than type B (Evangelista et al., 2018). ATAAD is more prone to have the complication of acute heart failure on presentation than type B (Mussa et al., 2016), and the in-hospital mortality rate of patients with type A is 22%, while type B is only 13% (Evangelista et al., 2018).
Surgery is widely regarded as the most important treatment for ATAAD. Surgical management was increased from 79% to 90% to treat ATAAD for the past 17 years (p < 0.001) (Pape et al., 2015), but the mortality of patients with ATAAD managed surgically was high (Hagan et al., 2000). Moreover, the medical mortality rate of those with ATAAD also remained high and did not change overtime (Evangelista et al., 2018). Drug treatment before the onset and after surgery would be beneficial to decrease mortality and recurrence of ATAAD. Drugs used for AD mainly aimed at controlling heart rate, lowering blood pressure and stabilizing plaque (Erbel et al., 2014). However, there is no public evidence proved to prevent the occurrence of AD (Bossone et al., 2018; Evangelista et al., 2018). Therefore, finding potential targets or compounds would be useful and promising approaches to ATAAD prevention or treatment.
Microarray technology has been widely used to diagnose disease, reveal the pathogenesis and discover new drug targets in recent years. Several studies reported new targets and underlying mechanisms in ATAAD using microarray technology (Weis-Muller et al., 2006; Cheuk and Cheng, 2011; Pan et al., 2014; Kimura et al., 2017; Pan et al., 2017; Wang L et al., 2017). However, the small amounts of samples and sample heterogeneity limited the understanding of ATAAD in these studies. Therefore, integrating microarray data can provide reliable and valuable signals for ATAAD. Drug repositioning refers to the development of existing drugs or compounds for new indications (Ashburn and Thor, 2004). The gene-phenotype connection has been used to predict novel disease signatures and potential targets for drugs, and large-scale data derived from systems biology were integrated by bioinformatics and cheminformatics tools for drug repositioning strategies (Karaman and Sippl, 2018). The library of integrated network-based cellular signatures (LINCS) L1000 dataset consists of over a million gene expression profiles of chemically perturbed human cell lines. Characteristic direction signature search engine L1000CDS2 based on L1000 dataset provides prioritization of thousands of small-molecule signatures, and could be used in drug repositioning for therapeutics (Duan et al., 2016). MiRNAs inhibit target mRNAs at post transcriptional level, and take important roles in the AD pathological process (Sbarouni and Georgiadou, 2018a). Analysis of miRNA-mRNA network of ATAAD could provide more reliable potential genes in ATAAD pathogenesis, prevention and drug discovery.
In the present work, two datasets GSE52093 (Pan et al., 2014) and GSE98770 (Kimura et al., 2017) were downloaded from the public database National Center for Biotechnology Information (NCBI) Gene Expression Omnibus (GEO) (https://www.ncbi.nlm.nih.gov/geo/) for integrative analysis. Differentially expressed genes (DEGs) from ascending aorta samples of both datasets were filtered. Protein-protein interaction (PPI) network and transcription factor network identification were applied in these DEGs. Weighted correlation network analysis (WGCNA) constructed the coexpression network and identified the highly correlated modules with ATAAD in two datasets respectively. DEGs in highly correlated modules were defined as consensus DEGs, and selected for Gene Ontology (GO) and Kyoto Encyclopedia of Genes and Genomes (KEGG) pathway enrichment analyses. Furthermore, L1000CDS2 was applied to predict drugs for ATAAD treatment using consensus DEGs. Finally, differentially expressed miRNA as well as their predicted target genes were analyzed. Targeted DEGs were identified with predicted targets and consensus DEGs. As a result, our study aims to highlight the insight into pathological mechanism, prevention and treatment of ATAAD.
The gene expression datasets GSE52093 and GSE98770 were obtained from NCBI GEO. All samples of both datasets were obtained from ascending aorta tissues. GSE52093 performing with mRNA microarrays contained five normal organ donors and seven ATAAD patients (Pan et al., 2014). GSE98770 contained 11 dissected ascending aorta samples of five normal organ donors and six ATAAD patients (Kimura et al., 2017). Gene expression profiling of GSE98770 was performed with mRNA and miRNA microarrays.
Raw data of GSE52093 and GSE98770 were obtained from GEO. These two datasets are based on different platforms, therefore the methods to identify the DEGs were different. The dataset of GSE52093 was based on the platform GPL10558 (Illumina HumanHT-12 V4.0 expression beadchip), and the DEGs were analyzed based on the limma package in R. Limma package identified the DEGs using Empirical Bayes test. The dataset of GSE98770 was based on the platform GPL14550 (Agilent-028004 SurePrint G3 Human GE 8 × 60K Microarray) for mRNA microarray, and were analyzed for DEGs identification using unpaired t test with Benjamini-Hochberg false discovery rate correction by Agilent GeneSpring GX (version 11.5, Genomax Technologies Pte Ltd., Singapore). Statistically significant DEGs of both datasets were defined with an FDR <0.05 and |fold change (FC)|>1.5 as the cut-off criteria. The common DEGs in two datasets were identified by Venn diagram.
A PPI network of the DEGs was analyzed by STRING database (http://string-db.org), and a confidence score > 0.9 (highest confidence) was set as significant. The FCs of common DEGs were presented as averages of GSE52093 and GSE98770. PPI network was then visualized by Cytoscape software (version 3.7.2, The Cytoscape Consortium, New York, NY, United States).
Cytoscape plugin iRegulon was used to identify TFs of the PPI network. The iRegulon was set as default. TFs with NES ≥3 and targeted more than 50% of the network nodes were identified as master TFs and then selected to construct the regulatory network. The expression levels of master TFs and all nodes in the PPI network were calculated and applied to Pearson correlation analysis.
The weighted correlations between ATAAD and all genes in GSE52093 or GSE98770 were analyzed by WGCNA package respectively as previously described (Li et al., 2018). The soft threshold power was calculated automatically. Modules are distinguished by colors. The correlation relationships between modules and ATAAD were then calculated to elucidate the highly correlated modules with ATAAD. The correlation coefficients and p-values were represented in the module-trait relationships. Modules with |correlation coefficient| > 0.5 were set as highly correlated modules with ATAAD.
Consensus DEGs were filtered by overlapping the DEGs and genes in highly correlated modules with ATAAD in two datasets using Venn diagram. KEGG pathway enrichment and GO biological process enrichment were analyzed by Cytoscape using ClueGO and CluePedia. ClueGO was set as follows: kappa score threshold to 0.4, the p-value to 0.05, two-sided hypergeometric test, Bonferroni step down.
L1000CDS2 (http://amp.pharm.mssm.edu/l1000cds2/#/index) is an advanced version of Connectivity Map with significantly increased drug treatments, cell types and gene signatures based on LINCS L1000 high-throughput technology. Consensus DEGs were inputted for drug repositioning. Compounds with overlap >0.1 were screened as potential drugs. MOA for potential drugs was provided by L1000 Fireworks Display (http://amp.pharm.mssm.edu/l1000fwd).
The dataset of GSE98770 was based on platform GPL17660 (Agilent-031181 Unrestricted Human miRNA V16.0 Microarray 030840) for miRNA microarray. The raw data were analyzed by NCBI web tool GEO2R (https://www.ncbi.nlm.nih.gov/geo/geo2r/) to identify differentially expressed miRNA. GEO2R performs comparisons using the limma R packages from the Bioconductor project. Significance was defined with FDR < 0.05 and |log2 FC| > 1 as the cut-off criteria.
The potential gene targets of differentially expressed miRNA were predicted by miRWalk Target Mining page (http://mirwalk.umm.uni-heidelberg.de/search_mirnas/). The Target Mining page provides an advanced search option for several miRNAs. The score was set as ≥0.95. Potential targets which also act as consensus DEGs were filtered and identified as targeted DEGs. miRNA-targeted DEG interaction network was visualized by Cytoscape software.
Human ascending aorta tissue samples obtained from six ATAAD patients and four non-ATAAD patients who underwent surgical resection. Non-ATAAD samples were normal tissues surrounding the cut samples from surgical donors. All specimens from ATAAD patients had a pathological diagnosis at the time of assessment. Studies were conducted under the prior informed consent procedure and with written consent from the human ethics committee of the first affiliated hospital (No. 2019-1493), college of medicine, Zhejiang University, China.
Human VSMCs were treated with 0.1 μm AngII for 12 h to mimic AD models in vitro (Li et al., 2017). Cells then were collected for DEGs validation.
The overlapped genes of consensus DEGs regulated by predicted drugs and targeted DEGs of differentially expressed miRNAs were identified as master DEGs. Master DEGs were validated by quantitative reverse transcription polymerase chain reaction (qRT-PCR) as previously described (Li et al., 2018). Total RNA was extracted by RNAsimple Total RNA Kit (Tian Gen, Beijing, China). cDNAs were conducted by PrimeScript TM RT reagent Kit (Takara Bio, Shiga, Japan). PCR amplifications were applied by TB Green TM Premix Ex Taq TM II (TaKaRa Bio). Measurement were normalized to GAPDH. All primer pairs are listed in Supplementary Table S1.
At least three independent cell experiments were carried out as repeats. Statistical analysis was performed by GraphPad Prism 6 (GraphPad Software Inc., San Diego, CA, United States). For human samples, statistical analysis was performed by unpaired t test. For cell samples, statistical analysis was performed by paired t test. A value of p < 0.05 was considered to be significant.
GSE52093 and GSE98770 were obtained from NCBI GEO. All of the samples from these datasets were obtained from ascending aorta. DEGs were defined as false discovery rate (FDR) < 0.05, and |fold change (FC)| > 1.5. A total of 1600 DEGs were screened in GSE52093, while 625 DEGs were filtered from GSE98770. As a result, 22 upregulated DEGs (Figure 1A) and 66 downregulated DEGs (Figure 1B) were found in both datasets. The expressions of these 88 common DEGs in both two datasets were displayed in a heatmap (Figure 1C). As shown in Figure 1C, these DEGs could clearly distinguish ATAAD from normal samples.
Figure 1. Identification of commonly changed DEGs in GSE52093 and GSE98770. (A), (B) Venn diagram displayed the upregulated DEGs (A) and downregulated DEGs (B) in both two datasets. (C) Heatmap of the 88 common DEGs in two datasets. Red: up-regulation; blue: down-regulation. Gene probe values were normalized by row Z-score.
All 88 DEGs were analyzed by the STRING database to verify the functional connectivity. As a consequence, there were 16 nodes and 12 edges in the PPI network with the highest confidence score, which represented proteins and functional interactions (Figure 2A). ACTG2 (degree = 3) and TPM2 (degree = 3) had the highest degree scores in the PPI network. TFs in the network were then predicted by iRegulon plugin of cytoscape. Five TFs SRF, TBP, TEAD4, CAT and E2F6 which had a NES ≥ 3 and targeted more than 50% of the network nodes were identified as master TFs of PPI network and displayed in Figure 2B. Interaction network of the master TFs and potential targeted DEGs was constructed (Figure 2C). The correlations between master TFs and PPI network were shown in Figure 2D (GSE98770) and 2E (GSE52093). We found that in the expression profiles of GSE98770, TEAD4 (Pearson r = −0.63, p = 0.036) was negatively correlated with PPI network, and CAT (Pearson r = 0.86, p = 0.0008) was positively correlated with the PPI network at significant levels (Figure 2D). In the expression profiles of GSE52093, TEAD4 (Pearson r = −0.51, p = 0.087) was also negatively correlated with PPI network, even though the difference did not reach statistical significance. Therefore, we concluded that TEAD4 might be a key TF in regulating PPI network of ATAAD.
Figure 2. PPI and transcription factor network construction in DEGs of ATAAD. (A). PPI network of commonly changed DEGs was constructed. Red indicated the upregulated DEGs and blue indicated the downregulated DEGs. (B) Master regulators of the PPI network. TFs with NES ≥3 and covered more than 50% of the network nodes were identified as master regulators. (C) Master regulator targeted network of the PPI network. Blue octagon nodes represent the predicted transcription factors. Red oval nodes represent transcription factor regulated genes. (D), (E) Correlations between master regulators and PPI network. Pearson correlation coefficient was shown in the circles.
Weighted coexpression networks were analyzed by WGCNA in two datasets respectively. The sample dendrogram and ATAAD heatmap of GSE52093 and GSE98770 were shown in Figures 3A and 3D. β = 12 was selected for GSE52093, and β = 8 was selected for GSE98770. Altogether nine modules were identified by the hierarchical clustering dendrogram in GSE52093 (Figure 3B). Among these gene modules, turquoize module and green module were negatively correlated with ATAAD, while pink module, blue module and yellow module were positively correlated with ATAAD (Figure 3C). In GSE98770, a total of 19 distinct modules were identified (Figure 3E). Magenta module was found to be negatively related to ATAAD, while red module, brown module and yellow module were positively related to ATAAD (Figure 3F).
Figure 3. WGCNA detected the correlated modules with ATAAD in GSE52093 and GSE98770. (A), (D) Sample dendrogram and trait heatmap of GSE52093 (A) and GSE98770 (D). The red color represents ATAAD sample, while the white represents normal samples. (B), (E) Construction of coexpression modules differentiated by colors. Each color indicated a correlated module of highly connected genes in GSE52093 (B) and GSE98770 (E). The gray module contained unassigned genes. (C), (F) Module and ATAAD relationships of GSE52093 (C) and GSE98770 (F) were displayed. Each row corresponds to a module eigengene. Each cell contains the corresponding correlation and the p-value in the parenthesis. Red represents a positive correlation and blue represents a negative correlation. Modules with |correlation coefficient| > 0.5 were set as highly correlated modules with ATAAD.
In order to elucidate the deep relationships between genes and ATAAD based on coexpression networks, we overlapped the DEGs and genes in highly correlated modules, and identified these genes as consensus DEGs. WGCNA indicated that turquoize, green, pink, blue and yellow modules of GSE52093 are highly correlated with ATAAD, while magenta, red, brown and yellow modules of GSE98770 are highly correlated with ATAAD (Figures 3E and 3F). 5,299 genes were included in highly correlated modules of GSE52093, and 3,381 genes were included in highly correlated modules of GSE98770. As a result, 71 consensus DEGs were filtered by Venn diagram (Figure 4A). The KEGG pathway analysis demonstrated that arginine and proline metabolism, dilated cardiomyopathy (DCM), and adrenergic signaling in cardiomyocytes were enriched in consensus DEGs (Figure 4B). GO biological process analysis showed that positive regulation of calcium ion-dependent exocytosis, regulation of cardiac muscle contraction by regulation of the release of sequestered calcium ion, calcium-dependent cell-cell adhesion via plasma membrane cell adhesion molecules, regulation of vascular smooth muscle proliferation, AV node cell action potential, and regulation of sodium ion transmembrane transporter activity were enriched in consensus DEGs (Figure 4C).
Figure 4. Identification and functional pathways annotation of consensus DEGs in ATAAD based on WGCNA. (A) Venn diagram showed the consensus DEGs included in highly correlated modules in GSE52093 and GSE98770. (B) KEGG pathway analysis of consensus DEGs. (C) Gene annotation enrichment analysis of GO biological process of consensus DEGs.
To explore potential drugs for ATAAD prevention or treatment after surgery, the characteristic direction signature search engine L1000CDS2 was applied. Consensus DEGs were inputted for drug repositioning. Depending on the identified 71 consensus DEGs, compounds or drugs that potentially reversed the gene signatures were acquired. Altogether 10 compounds with overlap > 0.1 were filtered as potential drugs. The heatmap of potential drugs and their modulated signatures were shown in Figure 5A. Upregulated consensus DEGs were downregulated by these compounds, while downregulated consensus DEGs were upregulated after compounds stimulation. Tivozanib regulated seven signatures among inputted consensus DEGs while others regulated six signatures. Among these compounds, tivozanib is a VEGFR inhibitor; S1030, BRD-K13810148, trichostatin A are HDAC inhibitors; geldanamycin is a HSP inhibitor; TL_HRAS26 is a calcium channel blocker; hydrocortisone hemisuccinate is a glucocorticoid receptor agonist (Figure 5B).
Figure 5. Predicted potential drugs for ATAAD through consensus DEGs by L1000CDS2. (A) Heatmap of predicted compounds and their modulated DEGs. Purple triangle indicated upregulated consensus DEGs, green triangle indicated downregulated consensus DEGs, red square indicated up expression after compounds stimulation, blue square indicated down expression after compounds stimulation. (B) MOA of the predicated compounds was provided by L1000FWD.
Gene expression profiling of GSE98770 was also performed with miRNA microarrays. Here we identified the differentially expressed miRNAs by GEO2R. FDR < 0.05 was set as the cut-off criteria, and seven differentially expressed miRNAs were filtered (Figure 6A). The miRNA expression profiles were shown in the heatmap, and all of the miRNAs were upregulated in ATAAD (Figure 6B). We next predicted the potential target genes of these miRNA by miRWalk database. As a result, 4,384 potential targets were found. miRNA always regulated genes negatively. Since the miRNAs were all upregulated, therefore we predicted the targeted DEGs between target genes and 52 downregulated consensus DEGs. As a result, 16 target genes were also found in consensus DEGs (Figure 6C). Furthermore, interaction network of the miRNA and potential targeted DEGs was constructed (Figure 6D). These targeted DEGs were regulated by five of all differentially expressed miRNAs. JAK2 was regulated by miR-374c-5p. DMD and SORBS1 were regulated by miR-7-1-3p. KRT222, SCN4B, PGR, TIMP3, and HOGA1 were only regulated by miR-193a-3p. PRUNE2, CACNB2 and IL16 were only regulated by miR-214-5p. SLC1A7, ALDH2 and PPP1R12B were only regulated by miR-181c-3p. KCNB1 was regulated by miR-193a-3p and miR-181c-3p. YPEL1 was regulated by miR181c-3P and miR-214-5p.
Figure 6. miRNA and mRNA interaction network. (A) Differentially expressed miRNAs of GSE98770 identified by GEO2R. (B) Heatmap of differentially expressed miRNA expression profiles. (C) Targeted DEGs were the overlapped genes obtained between target genes and consensus DEGs. (D) Interaction network of miRNA and targeted DEGs analyzed by miRWalk. Blue hexagons nodes indicated the potential targets which also act as consensus DEGs. Red ovals indicated the miRNA regulating these targeted DEGs.
Altogether 5 master DEGs, TIMP3, PRUNE2, ALDH2, SORBS1 and DMD were included in consensus DEGs regulated by predicted drugs and targeted DEGs of differentially expressed miRNAs (Figures 5A and 6D). As a result, we found that TIMP3 and SORBS1 were decreased in ATAAD ascending aorta tissue samples (Figure 7A). Moreover, we established an AD cell model using human Vascular smooth muscle cells (VSMCs) under the stimulation of Angiotensin II (AngII). We demonstrated that TIMP3, PRUNE2 and SORBS1 were all downregulated in AD cell model (Figure 7B).
Figure 7. Validation of master DEGs in human ATAAD samples in vivo and AD cell model in vitro. (A) The mRNA levels in ascending aorta tissues in normal samples (n = 4) and ATAAD samples (n = 6). The line represented median. Statistical significance was set as *p < 0.05, **p < 0.01 vs. normal. (B) Human VSMCs were treated with AngII. The line represented median. n = 3. Statistical significance was set as *p < 0.05, **p < 0.01 vs. control.
ATAAD is a common type of AD with high mortality (Evangelista et al., 2018). The limited understanding in pathogenesis and treatment approaches impaired ATAAD patients’ long term outcomes. Surgery is the most important treatment for ATAAD. Due to the high mortality, drug treatment before the onset and after surgery should be significant for reducing mortality and recurrence of ATAAD. Limited drugs used for AD mainly included beta-blockers, hypotensive drugs and statins (Erbel et al., 2014). Therefore, elucidating potential drug targets as well as the underlying mechanisms in ATAAD would contribute to highlight new strategies for ATAAD treatment. In the present study, we obtained two datasets GSE52093 and GSE98770 from public NCBI GEO. GSE52093 contained five normal organ donors and seven ATAAD patients (Pan et al., 2014), and GSE98770 contained five normal organ donors and six ATAAD patients (Kimura et al., 2017). All samples of the two datasets were acquired from dissected ascending aorta samples. Here we combined the two datasets in order to achieve more reliable and effective evidences for the pathogenesis and treatment of ATAAD by enlarging sample numbers.
In the present work, 88 DEGs were obtained totally. The PPI network identified ACTG2 and TPM2 had the highest degree scores in the PPI network. ACTG2 encodes actin gamma two; a smooth muscle actin. TPM2 is a member of the actin filament binding protein family, and mainly expressed in slow, type 1 muscle fibers. A recent study found that the mRNA and protein expression levels of TPM2 were significantly upregulated in aorta of ATAAD patients (Zhong et al., 2020). However, our analysis indicated a decrease of TPM2 in ATAAD samples. It remains further exploration to elucidate the effects of TPM2 in ATAAD. Until now, little is known about the roles of ACTG2 in AD. Generally, Actins are highly conserved proteins, our result might indicate the cell motility alteration in cytoskeleton of ATAAD aorta smooth muscle cells.
Five TFs SRF, TBP, TEAD4, CAT and E2F6 were predicted to regulate the PPI network of DEGs (Figure 2B). SRF, the serum response factor which had the highest NES among 5 TFs contributed to maintain the contractile phenotype of VSMCs (Xu et al., 2019) and smooth muscle differentiation (Horita et al., 2016). VSMC-specific EP4 deletion exacerbated angiotensin II-induced AD accompanied with decreased SRF transcriptional activity (Xu et al., 2019). Myocardin activates SRF, a conditional mutant of myocardin resulted in AD generation (Huang et al., 2015). Therefore, SRF might be a key TF in ATAAD pathogenesis. The relationships between other TFs and ATAAD have not been elucidated. CAT was positively correlated with the PPI network of GSE98770. CAT encodes catalase, a key antioxidant enzyme in the body’s defense against oxidative stress. Our result was consistent with the previous result that aortic ischemia-reperfusion increased the level of CAT (Kiris et al., 2008). TEAD4 was negatively correlated with PPI network of both datasets (Figures 2D and 2E). TEAD4 is a member of the transcriptional enhancer factor family of transcription factors. It participated in the 9p21.3 locus conferred risk of coronary artery disease in human aortic smooth muscle cells (Almontashiri et al., 2015). We conjectured that TEAD4 might be a novel target of ATAAD.
The coexpression network analysis identified five highly correlated modules in GSE52093 and four highly correlated modules in GSE98770 with ATAAD respectively (Figure 3). 71 consensus DEGs were filtered from genes in highly correlated modules and DEGs (Figure 4A). Functional pathway enriched in adrenergic signaling in cardiomyocytes for KEGG pathway analysis (Figure 4B), and positive regulation of calcium ion-dependent exocytosis, regulation of cardiac muscle contraction by regulation of the release of sequestered calcium ion, calcium-dependent cell-cell adhesion via plasma membrane cell adhesion molecules for GO biological process (Figure 4C). Beta-adrenergic blockers and calcium channel blockers act as two kinds of the drugs for ATAAD. Beta-adrenergic blockers, which controlling both heart rate and blood pressure, improved survival in ATAAD patients overall and who received surgery (Suzuki et al., 2012) However, there is still a lack of high-quality, random trials to evaluate the long-term efficacy of beta adrenergic-blocker in AD treatment (Koo et al., 2017). Calcium channel blockers have been shown to selectively improve survival only in type B AAD patients (Suzuki et al., 2012). We conjectured that calcium related signaling might be closely related to the causes of ATAAD. Our results suggested potential roles of beta-blocker and calcium channel blockers in the prevention and treatment of ATAAD.
Surgery is the most important treatment for ATAAD. Due to the high mortality, drug treatment before the onset and after surgery should be significant for reducing mortality and recurrence of ATAAD. Here we predicted the potential drugs based on integrated analysis of consensus DEGs. The predicted compounds mainly included VEGFR inhibitor, HDAC inhibitors, HSP inhibitor, calcium channel blocker and glucocorticoid receptor agonist (Figure 5B). As mentioned above, calcium channel blocker TL_HRAS26 which regulates calcium signals might act as a potential drug for ATAAD. A recent study showed that glucocorticoids regulate the vascular remodeling of aortic dissection, (Zhang et al., 2018) our results also indicated that hydrocortisone hemisuccinate might be a useful drug by activating glucocorticoid receptor. Previous research demonstrated that aorta samples are mainly made up of aortic smooth muscle cells, fibroblasts and endothelial cells. We speculated that VEGFR inhibitor might regulate the endothelial cells in the aorta in ATAAD treatment(Pan et al., 2017). Although there is no evidence for using the remaining compound categories in ATAAD, our results provided new insights and possibilities for drug treatment.
miRNAs alternation in serum and aortic tissues have been reported to contribute to the diagnosis and development of acute aortic dissection (Liao et al., 2011; Wang et al., 2015; Dong et al., 2017; Wang T et al., 2017; Xu et al., 2017; Sbarouni and Georgiadou, 2018b). Here, we identified seven upregulated differentially expressed miRNAs including miR-215, miR-181c-3p, miR-7-1-3p, miR-374c-5p, miR-214-5p, miR-193a-3p, miR-365a-3p from GSE98770 (Figure 6A). The roles of these miRNAs are still unclear in ATAAD. Integrative analysis revealed the interaction of miRNA and targeted DEG. Altogether 16 targeted DEGs were filtered. Consistent with previous studies, (Kimura et al., 2017; Corbitt et al., 2018) our data implied that TIMP3 might take an important role in ATAAD pathogenesis. The downregulated DEG JAK2 in our study has been demonstrated to have complex effects on aortic disease. Previous study of GSE52093 hypothesized that chronic inflammation in the aortic wall leading by decreased JAK2 expression contributed to ATAAD (Pan et al., 2014). However, JAK2 activation by increasing JAK2 phosphorylation was found in angiotensin II treated murine vascular smooth muscle cells (VSMCs), namely the in vitro model of dissecting aneurysm recently (Tanaka et al., 2018). Therefore, the functional role of JAK2 in ATAAD still needs further exploration. The roles of remaining targeted DEGs in ATAAD are still unclear, and these genes might be novel and potential targets of ATAAD.
At last, we validated 5 master DEGs in the human ATAAD sample and AD cell model. SORBS1 and TIMP3 were downregulated in ATAAD samples and AD models in vitro, while PRUNE2 only decreased in AD cell model (Figure 7). Among the predicted compounds, TL_HRAS26 modulated all three master DEGs, hydrocortisone hemisuccinate regulated SORBS1 and TIMP3 at the same times. These results implied that calcium channel blocker TL_HRAS26 and hydrocortisone hemisuccinate might be potential drugs for prevention of ATAAD and recurrence after surgery. On the other hand, we did not find a difference in PRUNE2, ALDH2 and DMD in vivo ATAAD samples and in vitro AD model, while SORBS1 downregulated only in AD cell model. We hypothesized that this might be due to the individual differences or a small sample size of in vivo ATAAD samples.
In conclusion, the present study offers potential targets and underlying molecular mechanisms ATAAD pathogenesis, prevention and drug discovery.
The original contributions presented in the study are included in the article/Supplementary Material, further inquiries can be directed to the corresponding author.
The study was conducted under prior informed consent procedure and with written consent from the human ethics committee of the first affiliated hospital, college of medicine, Zhejiang University, China. (No.2019-1493)
All authors have made a substantial, direct, and intellectual contribution to the work and approved it for publication.
The authors declare that the research was conducted in the absence of any commercial or financial relationships that could be construed as a potential conflict of interest.
The Supplementary Material for this article can be found online at: https://www.frontiersin.org/articles/10.3389/fphar.2020.575765/full#supplementary-material.
Almontashiri, N. A., Antoine, D., Zhou, X., Vilmundarson, R. O., Zhang, S. X., Hao, K. N., et al. (2015). 9p21.3 coronary artery disease risk variants disrupt tead transcription factor-dependent transforming growth factor beta regulation of p16 expression in human aortic smooth muscle cells. Circulation. 132, 1969–1978. doi:10.1161/CIRCULATIONAHA.114.015023
Ashburn, T. T., and Thor, K. B. (2004). Drug repositioning: identifying and developing new uses for existing drugs. Nat. Rev. Drug Discov. 3, 673–683. doi:10.1038/nrd1468
Bossone, E., LaBounty, T. M., and Eagle, K. A. (2018). Acute aortic syndromes: diagnosis and management, an update. Eur. Heart J. 39, 739–749. doi:10.1093/eurheartj/ehx319
Cheuk, B. L., and Cheng, S. W. (2011). Differential expression of elastin assembly genes in patients with stanford type a aortic dissection using microarray analysis. J. Vasc. Surg. 53, 1071–1078. doi:10.1016/j.jvs.2010.11.035
Corbitt, H., Morris, S. A., Gravholt, C. H., Mortensen, K. H., Tippner-Hedges, R., Silberbach, M., et al. (2018). Timp3 and timp1 are risk genes for bicuspid aortic valve and aortopathy in turner syndrome. PLoS Genet. 14, e1007692. doi:10.1371/journal.pgen.1007692
Dong, J., Bao, J., Feng, R., Zhao, Z., Lu, Q., Wang, G., et al. (2017). Circulating micrornas: a novel potential biomarker for diagnosing acute aortic dissection. Sci. Rep. 7, 12784. doi:10.1038/s41598-017-13104-w
Duan, Q., Reid, S. P., Clark, N. R., Wang, Z., Fernandez, N. F., Rouillard, A. D., et al. (2016). L1000cds2: lincs l1000 characteristic direction signatures search engine. Npj Syst. Biol. Appl. 2. doi:10.1038/npjsba.2016.15
Erbel, R., Aboyans, V., Boileau, C., Bossone, E., Bartolomeo, R. D., Eggebrecht, H., et al. (2014). 2014 esc guidelines on the diagnosis and treatment of aortic diseases: document covering acute and chronic aortic diseases of the thoracic and abdominal aorta of the adult. The task force for the diagnosis and treatment of aortic diseases of the european society of cardiology (esc). Eur. Heart J. 35, 2873–2926. doi:10.1093/eurheartj/ehu281
Evangelista, A., Isselbacher, E. M., Bossone, E., Gleason, T. G., Eusanio, M. D., Sechtem, U., et al. (2018). Insights from the international registry of acute aortic dissection: a 20-year experience of collaborative clinical research. Circulation. 137, 1846–1860. doi:10.1161/CIRCULATIONAHA.117.031264
Golledge, J., and Eagle, K. A. (2008). Acute aortic dissection. Lancet. 372, 55–66. doi:10.1016/S0140-6736(08)60994-0
Hagan, P. G., Nienaber, C. A., Isselbacher, E. M., Bruckman, D., Karavite, D. J., Russman, P. L., et al. (2000). The international registry of acute aortic dissection (irad): new insights into an old disease. Jama. 283, 897–903. doi:10.1001/jama.283.7.897
Horita, H., Wysoczynski, C. L., Walker, L. A., Moulton, K. S., Li, M., Ostriker, A., et al. (2016). Nuclear pten functions as an essential regulator of srf-dependent transcription to control smooth muscle differentiation. Nat. Commun. 7, 10830. doi:10.1038/ncomms10830
Huang, J., Wang, T., Wright, A. C., Yang, J., Zhou, S., Li, L., et al. (2015). Myocardin is required for maintenance of vascular and visceral smooth muscle homeostasis during postnatal development. Proc. Natl. Acad. Sci. U. S. A. 112, 4447–4452. doi:10.1073/pnas.1420363112
Karaman, B., and Sippl, W. (2018). Computational drug repurposing: current trends. Curr. Med. Chem. 26, 5389–5409. doi:10.2174/0929867325666180530100332
Kimura, N., Futamura, K., Arakawa, M., Okada, N., Emrich, F., Okamura, H., et al. (2017). Gene expression profiling of acute type a aortic dissection combined with in vitro assessment. Eur. J. Cardio. Thorac. Surg. 52, 810–817. doi:10.1093/ejcts/ezx095
Kiris, I., Kapan, S., Kilbas, A., Yilmaz, N., Altuntaş, I., Karahan, N., et al. (2008). The protective effect of erythropoietin on renal injury induced by abdominal aortic-ischemia-reperfusion in rats. J. Surg. Res. 149, 206–213. doi:10.1016/j.jss.2007.12.752
Koo, H. K., Lawrence, K. A., and Musini, V. M. (2017). Beta-blockers for preventing aortic dissection in marfan syndrome. Cochrane Database Syst. Rev. 11, D11103. doi:10.1002/14651858.CD011103.pub2
Li, B., Wang, Z., Hu, Z., Zhang, M., Ren, Z., Zhou, Z., et al. (2017). P38 mapk signaling pathway mediates angiotensin ii-induced mir143/145 gene cluster downregulation during aortic dissection formation. Ann. Vasc. Surg. 40, 262–273. doi:10.1016/j.avsg.2016.09.016
Li, L., Pan, Z., and Yang, X. (2018). Key genes and co-expression network analysis in liver of type 2 diabetes. J. Diabetes Investig. 10, 951–962. doi:10.1111/jdi.12998
Liao, M., Zou, S., Weng, J., Hou, L., Yang, L., Zhao, Z., et al. (2011). A microrna profile comparison between thoracic aortic dissection and normal thoracic aorta indicates the potential role of micrornas in contributing to thoracic aortic dissection pathogenesis. J. Vasc. Surg. 53, 1341–1349. doi:10.1016/j.jvs.2010.11.113
Mussa, F. F., Horton, J. D., Moridzadeh, R., Nicholson, J., Trimarchi, S., and Eagle, K. A. (2016). Acute aortic dissection and intramural hematoma: a systematic review. Jama. 316, 754–763. doi:10.1001/jama.2016.10026
Nienaber, C. A., and Clough, R. E. (2015). Management of acute aortic dissection. Lancet. 385, 800–811. doi:10.1016/S0140-6736(14)61005-9
Olsson, C., Thelin, S., Stahle, E., Ekbom, A., and Granath, F. (2006). Thoracic aortic aneurysm and dissection: increasing prevalence and improved outcomes reported in a nationwide population-based study of more than 14,000 cases from 1987 to 2002. Circulation. 114, 2611–2618. doi:10.1161/CIRCULATIONAHA.106.630400
Pan, S., Lai, H., Shen, Y., Breeze, C., Beck, S., Hong, T., et al. (2017). Dna methylome analysis reveals distinct epigenetic patterns of ascending aortic dissection and bicuspid aortic valve. Cardiovasc. Res. 113, 692–704. doi:10.1093/cvr/cvx050
Pan, S., Wu, D., Teschendorff, A. E., Hong, T., Wang, L., Qian, M., et al. (2014). Jak2-centered interactome hotspot identified by an integrative network algorithm in acute stanford type a aortic dissection. PloS One. 9, e89406. doi:10.1371/journal.pone.0089406
Pape, L. A., Awais, M., Woznicki, E. M., Suzuki, T., Trimarchi, S., Evangelista, A., et al. (2015). Presentation, diagnosis, and outcomes of acute aortic dissection: 17-year trends from the international registry of acute aortic dissection. J. Am. Coll. Cardiol. 66, 350–358. doi:10.1016/j.jacc.2015.05.029
Sbarouni, E., and Georgiadou, P. (2018a). Micrornas in acute aortic dissection. J. Thorac. Dis. 10, 1256–1257. doi:10.21037/jtd.2018.03.27
Sbarouni, E., and Georgiadou, P. (2018b). Micrornas in acute aortic dissection. J. Thorac. Dis. 10, 1256–1257. doi:10.21037/jtd.2018.03.27
Suzuki, T., Isselbacher, E. M., Nienaber, C. A., Pyeritz, R. E., Eagle, K. A., Tsai, T. T., et al. (2012). Type-selective benefits of medications in treatment of acute aortic dissection (from the international registry of acute aortic dissection [irad]). Am. J. Cardiol. 109, 122–127. doi:10.1016/j.amjcard.2011.08.012
Tanaka, T., Kelly, M., Takei, Y., and Yamanouchi, D. (2018). Rankl-mediated osteoclastogenic differentiation of macrophages in the abdominal aorta of angiotensin ii-infused apolipoprotein e knockout mice. J. Vasc. Surg. 68, 48–59. doi:10.1016/j.jvs.2017.11.091
Wang, L., Zhang, S., Xu, Z., Zhang, J., Li, L., and Zhao, G. (2017). The diagnostic value of microrna-4787-5p and microrna-4306 in patients with acute aortic dissection. Am. J. Transl. Res. 9, 5138–5149.
Wang, T., He, X., Liu, X., Liu, Y., Zhang, W., Huang, Q., et al. (2017). Weighted gene co-expression network analysis identifies fkbp11 as a key regulator in acute aortic dissection through a nf-kb dependent pathway. Front. Physiol. 8, 1010. doi:10.3389/fphys.2017.01010
Wang, X. J., Huang, B., Yang, Y. M., Zhang, L., Su, W. J., Tian, L., et al. (2015). Differential expression of micrornas in aortic tissue and plasma in patients with acute aortic dissection. J Geriatr Cardiol. 12, 655–661. doi:10.11909/j.issn.1671-5411.2015.06.013
Weis-Muller, B. T., Modlich, O., Drobinskaya, I., Unay, D., Huber, R., Bojar, H., et al. (2006). Gene expression in acute stanford type a dissection: a comparative microarray study. J. Transl. Med. 4, 29. doi:10.1186/1479-5876-4-29
Xu, H., Du, S., Fang, B., Li, C., Jia, X., Zheng, S., et al. (2019). Vsmc-specific ep4 deletion exacerbates angiotensin ii-induced aortic dissection by increasing vascular inflammation and blood pressure. Proc. Natl. Acad. Sci. U. S. A. 116, 8457–8462. doi:10.1073/pnas.1902119116
Xu, Z., Wang, Q., Pan, J., Sheng, X., Hou, D., Chong, H., et al. (2017). Characterization of serum mirnas as molecular biomarkers for acute stanford type a aortic dissection diagnosis. Sci. Rep. 7, 13659. doi:10.1038/s41598-017-13696-3
Zhang, L., Zhou, J., Jing, Z., Xiao, Y., Sun, Y., Wu, Y., et al. (2018). Glucocorticoids regulate the vascular remodeling of aortic dissection via the p38 mapk-hsp27 pathway mediated by soluble tnf-rii. Ebiomedicine. 27, 247–257. doi:10.1016/j.ebiom.2017.12.002
Keywords: acute type a aortic dissection, differentially expressed genes, weighted correlation network analysis, drug repositioning, differentially expressed miRNA
Citation: Fang J, Pan Z, Yu H, Yang S, Hu X, Lu X and Li L (2021) Regulatory Master Genes Identification and Drug Repositioning by Integrative mRNA-miRNA Network Analysis for Acute Type A Aortic Dissection. Front. Pharmacol. 11:575765. doi: 10.3389/fphar.2020.575765
Received: 21 July 2020; Accepted: 21 December 2020;
Published: 21 January 2021.
Edited by:
Marcelo Rizzatti Luizon, Federal University of Minas Gerais, BrazilReviewed by:
Claudia Pisanu, University of Cagliari, ItalyCopyright © 2021 Fang, Pan, Yu, Yang, Hu, Lu and Li. This is an open-access article distributed under the terms of the Creative Commons Attribution License (CC BY). The use, distribution or reproduction in other forums is permitted, provided the original author(s) and the copyright owner(s) are credited and that the original publication in this journal is cited, in accordance with accepted academic practice. No use, distribution or reproduction is permitted which does not comply with these terms.
*Correspondence: Li Lu, bHVjaWxsZUB6anUuZWR1LmNu
Disclaimer: All claims expressed in this article are solely those of the authors and do not necessarily represent those of their affiliated organizations, or those of the publisher, the editors and the reviewers. Any product that may be evaluated in this article or claim that may be made by its manufacturer is not guaranteed or endorsed by the publisher.
Research integrity at Frontiers
Learn more about the work of our research integrity team to safeguard the quality of each article we publish.