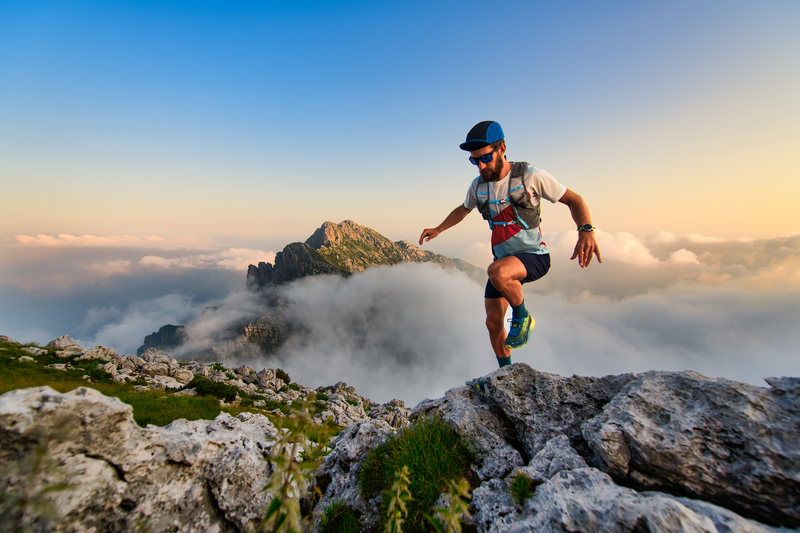
94% of researchers rate our articles as excellent or good
Learn more about the work of our research integrity team to safeguard the quality of each article we publish.
Find out more
SYSTEMATIC REVIEW article
Front. Pharmacol. , 29 April 2020
Sec. Drugs Outcomes Research and Policies
Volume 11 - 2020 | https://doi.org/10.3389/fphar.2020.00570
This article is part of the Research Topic Deprescribing and Minimizing Use of Anticholinergic Medications View all 6 articles
Background: Greater anticholinergic burden (ACB) increases the risk of mortality in older individuals, yet the strength of this association varies between studies. One possible explanation for this variance is the use of different approaches to quantify ACB. This systematic review (PROSPERO number CRD42019115918) assessed the prognostic utility of ACB-specific measures on mortality in older individuals.
Methods: Multiple cross-disciplinary databases were searched from 2006–2018. Observational studies assessing the association between ACB and mortality utilizing ≥1 ACB measure, involving persons aged ≥65 years were included. Screening and data extraction were performed by two independent reviewers, with disagreements resolved by a third independent reviewer. Risk of bias and quality of evidence were assessed using Quality in Prognosis Studies (QUIPS) and Grading of Recommendations, Assessment, Development, and Evaluations (GRADE) criteria. Meta-analysis was conducted where appropriate.
Results: Of 19,224 titles, 20 articles describing 18 cohort studies involving 498,056 older individuals were eligible. Eight anticholinergic-specific measures were identified; the Anticholinergic Cognitive Burden Scale (ACBS, n=9) and Anticholinergic Risk scale (ARS, n=8) were most frequently reported. The evidence base was of poor quality, with moderate to high risk of bias. Meta-analysis showed increased mortality risk.
Conclusions: There was a modest association between some ACB measures and mortality, with most evidence derived from the ACBS. Studies comparing different measures within the same population were lacking. Analysis was limited by poor generalizability between studies, specifically regarding heterogeneity in methodology and reporting, as well as high risk of bias for most studies in the evidence base.
The cumulative use of anticholinergics, termed anticholinergic burden (ACB), has been highlighted as one problematic area of polypharmacy in older populations (Hilmer et al., 2007; Salahudeen et al., 2015). It is estimated that 20–50% of older persons have been prescribed at least one medication with anticholinergic activity (Campbell et al., 2009), with increases in prescribing rates and ACB as individuals age (Lu et al., 2015). While anticholinergics are useful in conditions such as depression, incontinence, Parkinson’s disease, and gastrointestinal disorders, their broad range of action on the central and peripheral nervous systems can result in significant side-effects. Central side-effects include sedation, confusion and delirium (Rudolph et al., 2008; Fox et al., 2014). Peripheral side-effects include dry mouth and eyes, tachycardia, and constipation (Rudolph et al., 2008; Fox et al., 2014). Additionally, concerns have been raised as to the potential of anticholinergics to exacerbate physical and mental decline, especially regarding dementia development (Fox et al., 2014; Myint et al., 2014; Salahudeen et al., 2015). Consequently, various anticholinergics have been listed as potentially inappropriate medications for use in older individuals, as indicated by the Beers Criteria® (The American Geriatrics Society Beers Criteria® Update Expert Panel et al., 2019) and STOPP criteria (O’Mahony et al., 2010).
One risk suggested to be associated with greater ACB amongst older individuals is mortality, although the significance and strength of this association varies between studies (Fox et al., 2014). One explanation may lie in how ACB was quantified. To date, multiple measures exist to assess ACB but differences are apparent between measures in terms of which medications are included and the level of anticholinergic potency attributed to individual medications (Bostock et al., 2013; Salahudeen et al., 2015). For example, clomipramine is assessed as having “high” potency according to Boustani et al. (2008) and Carnahan et al. (2006), yet this drug is not included in the analysis by Rudolph et al. (2008). Drug availability and formularies between countries can also impact the ability of measures, typically developed with the drug formularies of one country, to be applied internationally (Salahudeen et al., 2015). Currently, there is no evidence to support the decision to use or not use one ACB measure above another. Improving this understanding will allow informed ACB measure choices to be made and enhance the quality of future research. Therefore, this systematic review aims to describe the association of individual ACB measures with mortality in older adults.
This systematic review, followed Preferred Reporting Items for Systematic Reviews and Meta-Analyses (PRISMA) guidance (www.prisma-statement.org; see Supplementary Table 2 for PRISMA checklist) and was registered with PROSPERO (www.crd.york.ac.uk/prospero), registration number CRD42019115918).
MEDLINE (Ovid), EMBASE (Ovid), PsycINFO (Ovid), and CINAHL (EBSCO) were searched using a comprehensive search strategy, adapted to suit each specific database. Study searches were undertaken on 16th November 2018. The validated search filter utilized to identify prognostic studies was in line with the recommendations of Geersing et al. (2012). Both MeSH and text/key words were included. Studies published between 1st January 2006 and 16th November 2018 were included. The initial timepoint of 2006 was used as cumulative ACB was first described during this period. Reference lists and citation checks of identified eligible studies were reviewed for further eligible studies. The search strategy is reported in Supplementary Table 1.
Studies were deemed eligible if they were in accordance with the following criteria:
● Prospective and retrospective observational studies, for example longitudinal cohorts and case-control studies from any setting (e.g., community/primary care/general practice, secondary care/hospital/acute care, nursing homes/homes).
● Mean study participant age was ≥65 years.
● Human studies investigating the total exposure of anticholinergic burden by use of ACB-specific measure. The comparator required was non-users of anticholinergic medications and outcome of interest was mortality.
Studies were excluded if:
● They were systematic reviews, randomized control trials, cross-sectional studies, opinion/editorial articles, qualitative studies, or animal studies.
● Study mean age was <65 years.
● Non-anticholinergic specific measures (e.g., BEERS criteria, Drug Burden Index(total)) were used
● Specific anticholinergic medications or groups of medications (e.g., bladder antispasmodics) were assessed and not total anticholinergic exposure.
Studies identified through database searches were entered RefWorks (ProQuest LLC, Ann Arbor, MI, USA) for bibliographic management to remove duplicates. Studies were transferred to Covidence systematic review software ©2019 (Veritas Health Innovation Ltd., www.covidence.org) for screening, whereafter 13,197 studies remained after duplicate removal. Titles and abstracts of records were screened by two of three independent reviewers (shared between CS, KY, MK) to determine whether inclusion criteria were met. For a title to be excluded, two independent reviewers had to agree on exclusion; where disagreement occurred, a third independent reviewer (TQ) would aid resolution. Thereafter, full texts of potentially eligible studies (n=119) were obtained and further independently examined by two of three reviewers (CS, KY, MK) against the inclusion criteria to determine eligibility. Study authors were contacted where full texts could not be sourced. Again, a third independent reviewer (TQ) resolved any disagreements regarding exclusion. Included studies had their citations reviewed through PubMed and reference lists hand searched to check for further eligible studies. Reference lists and citations of recent seminal articles (Salahudeen et al., 2015) were also searched.
A data extraction template was developed in accordance with guidance by the Cochrane Prognostic Review Group framework (https://methods.cochrane.org/prognosis/our-publications) requirements for data. Key data extracted included study characteristics (e.g., publication date, country, study setting), population descriptives, ACB measure(s) used, types of data (e.g., continuous, ordinal), the timing of predictor, and outcome variables, covariates adjusted for, statistical plan and results. Data was extracted by two of three independent reviewers (KG, CS, MT); any disagreements were resolved by a third independent reviewer (RS). Thereafter, data were transferred to a Microsoft Excel 2016 (https://products.office.com/en-gb/excel) sheet and imported to Comprehensive Meta-Analysis (CMA) (v3.3.070 Biostat, Englewood, USA; https://www.meta-analysis.com/) for analysis, with forest plots produced where appropriate. All meta-analysis data presented compared ACB = 0 with ACB >0.
The quality and risk of bias of included studies was assessed by the Quality in Prognosis Studies tool, developed by the Cochrane Prognosis Methods Group (QUIPS, available: https://methods.cochrane.org/sites/methods.cochrane.org.prognosis/files/public/uploads/QUIPS%20tool.pdf). The QUIPS tool comprises of six areas: study participation, attrition, prognostic measurement, outcome measurement, study confounding, and statistical analysis. The wording of the QUIPS anchoring statement was modified to be suitable for the current review question as recommended. Any published baseline measure of ACB was acceptable and adjusting for a minimum set of confounders (age, sex, and any measure of comorbidity burden) was considered adequate for assessing quality of statistical analysis. Risk of bias assessments were carried out by two of three reviewers (KG, CS, MT). Any discrepancies were resolved by a third independent reviewer (RS). The summary of the QUIPS risk of bias assessment can be found in Figure 4.
Data were analyzed and split into two primary groups: hazard ratio and odds ratio data. Data were further stratified according to the ACB measurement tool utilized and whether these data were continuous or ordinal. Where possible, analyses were further stratified by strength of ACB exposure into “high” or “low,” though precise definitions varied by study and were reported individually for each meta-analysis (see Table 3 for definitions of “high” and “low” categories within each study). Meta-analyses were undertaken on comparable datasets. 95% confidence intervals (CIs) were utilized. CMA was used to facilitate the production of meta-analyses and forest plots. Random effects models were used.
If multiple results were provided in the same study, the most appropriate result was chosen to be included in the meta-analyses by two researchers (CS and KG) so as not to duplicate the same study population within one meta-analysis (see results). For Lattanzio 2018 A (Lattanzio et al., 2018a) and Lattanzio 2018 B (Lattanzio et al., 2018b), one cohort was used for both studies. Again, to avoid cohort duplication, data assessing ACB on the cohort as a whole (Lattanzio 2018 A (Lattanzio et al., 2018a)) were chosen as more generalizable than data produced after stratification of individuals by Basic Activities of Daily Living (BADL) scores.
Where available, data adjusted for covariates (e.g., age, sex, comorbidities) and/or temporal changes in ACB were given priority over unadjusted, baseline ACB-only data. For studies which had multiple follow-up periods, the follow up period most comparable to the other studies within the review was chosen. Where data were considered too heterogenous to be included in any meta-analysis, they were analyzed narratively.
The quality of the body of evidence collected throughout this systematic review was assessed with the GRADE tool. The GRADE tool assesses the quality of evidence for given outcomes of studies, as opposed to assessing the evidence from individual studies (Guyatt et al., 2008). Evidence quality was assessed by two researchers (CS, KG) across seven criteria; study limitations, inconsistency, indirectness, imprecision, publication bias, effect size and dose-effect. Subsequently, studies were upgraded or downgraded in relation to the seven criteria and a final decision was made as to the quality of evidence (ranging from high quality to very low quality). This process was adapted for prognostic review studies in accordance with the recommendations laid out by Huguet et al. (2013).
Twenty papers, including 18 cohorts, were included. Reasons for exclusion are presented in the Preferred Reporting Items for Systematic Reviews and Meta-Analyses (PRISMA) flowchart (Figure 1). The PRISMA checklist is outlined in Supplementary Table 2. An electronic database search identified 19,224 studies; these reduced to 13,197 after removing duplicates. Titles were screened manually for eligibility whereafter 119 articles remained. Full texts were assessed leaving 20 articles. Most common reasons for exclusion were studies assessing the wrong outcome and using non ACB-specific measures. Descriptive information on the included papers can be found in Table 1.
Figure 1 Preferred Reporting Items for Systematic Reviews and Meta-Analyses (PRISMA) flowchart depicting the study screening and selection process.
Table 1 Descriptive data of papers included in review (n = 20), stratified by anticholinergic burden (ACB) measure.
A total of 498,056 older individuals participated across the 18 studies, with a sample size range of 71 (Mangoni et al., 2013) to 245,410 (McIsaac et al., 2018) people. Two identical study cohorts were each used twice in separate papers (Lattanzio 2018 A (Lattanzio et al., 2018a) and B (Lattanzio et al., 2018b); Lowry 2011 (Lowry et al., 2011) and 2012 (Lowry et al., 2012)); as a result, participants were only counted once. Ages ranged from mean 71 years (SD 12) (Agar et al., 2010) to median 93 years (IQR 91-95) (Kidd et al., 2014). Fifteen studies were based in Europe, three in North America and two in Australia (see Table 1).
Eight ACB measures were used (Han et al., 2001; Carnahan et al., 2006; Hilmer et al., 2007; Boustani et al., 2008; Chew et al., 2008; Rudolph et al., 2008; Durán et al., 2013; Klamer et al., 2017). The most frequently used were the Anticholinergic Cognitive Burden Scale (ACBS; n=9), Anticholinergic Risk Scale (ARS; n=8), Anticholinergic Drug Scale (ADS; n=4) and the Drug Burden Index(anticholinergic) (DBI(antichol); n=2). Measures reported once each were the list of Chew (hereon referred to as “Chew”), Clinician Rated Anticholinergic Scale (CrAS), Duran’s list and the Muscarinic Acetylcholinergic Receptor ANTagonist Exposure (MARANTE) scale. Summaries of each anticholinergic measure can be found in Supplementary Table 3. Four papers used more than one ACB measure in their analysis. Sevilla-Sánchez et al. (2018) used two measures, however one of these (DBI(total)) was not anticholinergic-specific (see exclusion criteria). The data for this measure were therefore excluded from analysis.
Forest plot of all hazard ratios and odds ratios of all-cause mortality within the evidence base can be found in Figures 2 and 3, respectively. Meaningful meta-analysis of all studies was not possible due to high heterogeneity in study design and reporting.
Figure 2 Forest plot of all hazard ratios of all-cause mortality within the evidence base. A summary statistic is not included as the same study population is used more than once. ACBS, Anticholinergic Cognitive Burden Scale; ADS, Anticholinergic Drug Scale; ARS, Anticholinergic Risk Scale; DBI, Anticholinergic Component of the Drug Burden Index. Variation was apparent in how individual studies classed “high” and “low” exposure through ACB measures. Lattanzio 2018 A and Vetrano 2016 classed “low” exposure as a score of ACBS one and “high” exposure as ACBS two or more. Kumpula 2011 and McIsaac 2018 classed “low” exposure as ARS one to two and “high” exposure as ARS three or more.
Figure 3 Forest plot of all odds ratios of all-cause mortality within the evidence base. A summary statistic is not included as the same study population is used more than once. ACBS, Anticholinergic Cognitive Burden Scale; ADS, Anticholinergic Drug Scale; ARS, Anticholinergic Risk Scale. Variation was apparent in how individual studies classed “high” and “low” exposure through ACB measures. “Low” anticholinergic exposure data for Chatterjee 2017 was not given. “High” exposure for Chatterjee 2017 ACBS/ADS was classed as a score of 2 or 3. Egberts 2017 classed “low” exposure as ACBS/ARS one to two and “high” exposure as ACBS/ARS three or more. Kidd 2014 classed “low” exposure as ACBS one and “high” exposure as ACBS two or more. Fox 2011 classed “low” exposure as ACBS one or more and “high” as ACBS two or more. Gutierrez-Valencia 2017 classed “low” exposure as ARS one and “high” as ARS two or more.
Results of the QUIPS risk of bias assessment are available in Figure 4. Of the 20 papers in the final analysis, 15 were considered high risk of bias in at least one or more QUIPS category. Of these, three papers (Vetrano et al., 2016; Wauters et al., 2017; Aalto et al., 2018) had high risk of bias scores in four or more of the six QUIPS categories. A common area of bias included a lack of reporting of prognostic factor measurement—no study had assessed anticholinergic intake by two independent means. Additionally, studies presented with a lack of justification for the adjustment of certain confounders, as well as overall lack of adjustment or possible over-adjustment for confounders. Only two studies adjusted for temporal change in a patient’s ACB exposure. Furthermore, statistical reporting was frequently not detailed and access to study protocols was limited. Frequently, unadjusted results were not reported as opposed to adjusted results, negating the possibility of unadjusted meta-analysis synthesis.
Full GRADE results can be viewed in Table 2. Overall, the quality of the evidence base was considered very low for each ACB measure. Issues arose across the GRADE criteria and included: studies having a high risk of bias, limited generalizability of study setting, small effect sizes, risk of publication bias, and heterogenous reporting of potential dose-effect. For publication bias assessment, it was not possible to build funnel plots due to insufficient studies and variation in statistical effect sizes.
Table 2 Results of the Grading of Recommendations, Assessment, Development, and Evaluations (GRADE) analysis.
Full information regarding study results can be found in Table 3. Nine studies Chatterjee et al. (2017), Cross et al. (2017), Egberts et al. (2017), Fox et al. (2011), Kidd et al. (2014), Lattanzio et al., (2018a), Lattanzio et al. (2018b), Mangoni et al. (2013) and Vetrano et al. (2016) involving 244,090 individuals were included, with cohort sizes ranging from 71 Mangoni et al. (2013) to 224,740 Chatterjee et al. (2017). Two studies Lattanzio et al. (2018a); Lattanzio et al. (2018b) utilized the same study population; subsequently, their population was only counted once within the total. Five took place in hospital settings, two in nursing homes, one incorporated community and nursing home residents and one in an outpatient clinic setting.
Table 3 Summary of results of papers included in review (n=20), stratified by anticholinergic burden (ACB) measure.
Summary statistics for “low” anticholinergic exposure measured with the ACBS were HR 1.39 (95%CI 1.1–1.75) and OR 1.53 (95%CI 1.34–1.75); for “high” ACBS these were HR 1.47 (95%CI 1.19–1.81) and OR 1.37 (95% 1.12–1.68) Figure 5. Continuous data was analyzed separately, yielding a summary HR of 1.87 (95%CI 1.07–1.32), however it should be noted that within this, results from Mangoni et al. (2013) were unadjusted.
Figure 5 Forest plot of odds ratios of all-cause mortality grouped by anticholinergic exposure level using the ACBS (Anticholinergic Cognitive Burden Scale). “Low” anticholinergic exposure data for Chatterjee 2017 was not given. “High” exposure for Chatterjee 2017 ACBS was classed as a score of 2 or 3. Egberts 2017 classed “low” exposure as ACBS one to two and “high” exposure as ACBS three or more. Kidd 2014 classed “low” exposure as ACBS one and “high” exposure as ACBS two or more. Fox 2011 classed “low” exposure as ACBS one or more and “high” as ACBS two or more.
Data for Lattanzio et al. (2018b) was not included in meta-analysis so as not to include the same cohort twice. However, the results from this study were stratified into individuals with a dependency in one or more Basic Activities of Daily Living (BADL) and those without. These results indicated that those who were dependent in at least one BADL were more likely to have a significant association between greater ACB and mortality (HR 1.28 (95%CI 1.11–1.49)) than those who did not (HR 0.98 (95%CI 0.75–1.28). Results from Mangoni et al. (2013) were unadjusted.
The ADS was reported in four papers Chatterjee et al. (2017), Mangoni et al. (2013), Sarbacker et al. (2017), and Sevilla-Sánchez et al. (2018). The total population size was 226,543 individuals, with sample sizes ranging from 71 Mangoni et al. (2013) to 224,740 Chatterjee et al. (2017). Two studies took place in hospital settings, one in nursing homes and one was community-based.
Meta-analysis of three studies Mangoni et al. (2013), Sarbacker 2017 (Sarbacker et al., 2017), Sevilla-Sánchez et al. (2018) produced a summary HR of 1.1 (95%CI 1.04–1.15). Data not included in the meta-analysis Chatterjee et al. (2017), comparing “high” ADS (ADS 2/3) to no ADS 90 days before death indicated a stronger association [OR 1.37 (95%CI 1.34–1.4) (Figure 2)]. Data for low or continuous ACB within the Chatterjee et al. (2017) cohort were not reported. Results from Mangoni et al. (2013) were unadjusted.
Eight papers reported use of the ARS Aalto et al. (2018), Egberts et al. (2017), Gutiérrez-Valencia et al. (2017), Kumpula et al. (2011), Landi et al. (2014), Lowry et al. (2011), Mangoni et al. (2013), McIsaac et al. (2018). Cohort sizes ranged from 71 Mangoni et al. (2013) to 245,410 McIsaac et al. (2018) and included 252,595 participants. Six cohorts were based in hospital settings and two were in nursing home settings.
Meta-analysis of categorical data for “low” ARS produced summary HR 1.15 (95%CI 1.08–1.22) and OR 1.07 (95%CI 0.68–1.68) and “high” ARS produced HR 1.14 (95%CI 1.06–1.22) and OR 0.81 (95%CI 0.3–2.16). A further two studies Lowry et al. (2011), Mangoni et al. (2013) comprised a meta-analysis of continuous ARS data and resulted in a summary HR of 1.48 (95%CI 0.71–3.08).
Two papers were not included in the meta-analysis Aalto et al. (2018), Landi et al. (2014). It should be noted that the cumulative nature of the ARS measure was not applied in the analysis of Aalto et al. (2018); rather, the medications listed within the ARS were used to sum the number of anticholinergic medications a patient was taking. Landi et al. (2014) reported OR 1.02 (95%CI 1.02–0.92–1.12).
Two prospective cohort studies reported use of the DBI(antichol) [Lowry 2012 (Agar et al., 2010), Mangoni et al. (2013) and included a total population of 433 older patients in acute care settings. Lowry 2012 (Agar et al., 2010) reported HR 1.10 (95%CI 0.44–2.74) and Mangoni et al. (2013) reported HR 3.2 (1.1–9.4). Results from Mangoni et al. (2013) [HR 3.2 (95%CI 1.1–9.35)] were unadjusted.
Four papers (three prospective cohorts, one retrospective cohort) reported measures used only once each Agar et al. (2010), Egberts et al. (2017), Gutiérrez-Valencia et al. (2017), Wauters et al. (2017). These measures were: Clinician Rated Anticholinergic Scale (CrAS); Chew; Duran’s list; MARANTE.
Agar et al. (2010) stated “Log-rank data showed no evidence that survival differed significantly between the three groups,” however, no quantitative data were provided. Significant associations between ACB and mortality were produced by Gutiérrez-Valencia et al. (2017) [Duran’s=1, OR 1.69 (95%CI 1.02–2.82) and Wauters et al. (2017) (MARANTE “high” ≥2: HR 2.20 (95%CI 1.03–4.67)].
Four papers utilized more than one measure to assess ACB within the same cohort of individuals Chatterjee et al. (2017), Egberts et al. (2017), Gutiérrez-Valencia et al. (2017), Mangoni et al. (2013). Chatterjee et al. (2017) compared ADS with ACBS, whereby ADS resulted in significantly higher odds ratios than ACBS [ADS level 2/3: OR 1.37 (95%CI 1.34, 1.40); ACBS level 2/3: OR 1.24 (95%CI 1.21, 1.27)]. No data were given for low anticholinergic exposure for either scale.
Data provided by Egberts et al. (2017) compared ARS, ACBS, and Chew. Most results showed no significant association, with wide CIs (Table 3). ACBS exhibited higher odds ratios compared to ARS and Chew, but the differences were not statistically significant. Gutiérrez-Valencia et al. (2017) compared ARS and Duran’s list. There was a positive association between ACB measured by Duran’s list and mortality (OR 1.69 (95%CI 1.02–2.82), while the association between ARS and mortality was not significant. Mangoni et al. (2013) compared ACBS, ADS, ARS, and DBI(antichol), however, interpretation of these results were limited as analyses for all measures (apart from ARS three-month mortality) were not adjusted for confounders. Hazard ratios were small and not statistically significant for ACBS and ADS and increased with ARS (HR 2.2 (95%CI 1.2–3.7) and DBI(antichol) (HR 3.2 (95%CI 1.1–9.4)).
This is the first systematic review and meta-analysis assessing the prognostic utility of individual ACB measures to predict mortality specifically in individuals ≥65 years. Of 20 included studies, 11 reported a significant association between ACB and mortality and meta-analysis showed a positive association between greater ACB and mortality. However, a dose-effect response was not consistently apparent. The most frequently used ACB measures were ACBS, ARS and ADS. Inter-study variation and methodological heterogeneity between studies precluded combined meta-analyses across all scales.
Our finding that greater ACB appears associated with increased risk of mortality conflicts with the findings reported in the Fox et al. (2014) systematic review, where no significant association between ACB and mortality was identified. This may be because our review focused on older people, where risk of mortality is much higher. Also, the current review focused on use of any published ACB scale whilst their review relied on defining ACB where medications were included in the ACBS, a scale developed to predict cognitive decline rather than mortality. Another explanation may be that the majority (n=14) of our included studies were published post-2014, in line with increasing interest in this topic area, providing a larger pool of data to analyze. Specifically, this allowed the production of summary statistics, which were not possible in the Fox et al. (2014) review.
It was not possible for us to declare one scale as superior to another. Larger odds and hazard ratios were seen with the ACBS, supporting the findings of Ruxton et al. (2015) where the ACBS showed the largest effect sizes. The discrepancies in effect sizes between scales may be a result of the variations in how differing ACB measures assess the potency of an anticholinergic agent, as highlighted by Salahudeen et al. (2015). For example, the ACBS was developed through systematic review and a multi-disciplinary panel to identify anticholinergic medications and assess their potency (Boustani et al., 2008). On the other hand, the ARS was developed by geriatricians and pharmacists within the Veterans Affairs Boston Healthcare System via a literature review of the most commonly prescribed medications among predominantly male, veteran patients (Rudolph et al., 2008). The varying number of drugs with anticholinergic properties included in each measure, along with their graded potencies, may contribute towards variations in risk seen in the current review. The fact the three most frequently used measures (ACBS, ARS, ADS) were all developed in the USA may limit applications internationally due to differing drug formularies between countries.
While our meta-analysis adds helpful new information to the existing literature base, there are limitations that should be noted. Firstly, the reporting of results between studies varied greatly; this led to multiple levels of stratification before data could be grouped for meta-analysis. Consequently, a large combined meta-analysis, including all 20 studies, could not be created and meaningfully interpreted. Once stratified by ACB measure, data were limited, with some single meta-analyses containing only two studies. Additionally, many studies did not have long enough follow-up times. Also, the DBI(antichol) is the only ACB measure which takes into account the dose of drug being administered to a patient, yet it was only used twice in the current review, limiting the ability to interpret whether having a scale which takes dose into account is of benefit in assessing morality risk. Finally, the indication of anticholinergic medications in each study is not known. It is possible this may have an impact on mortality risk; while those being treated for multiple conditions are more likely to be users of anticholinergic medications, multi-morbidity alone is associated with mortality risk (Wauters et al., 2017).
A clear advantage of the current study was the use of contemporary best practice guidance for prognostic reviews; the framework by the Cochrane Prognostic Review Group was used in order to ensure the methodology and reporting within this review adhered to high quality standards.
Another advantage of the current study was the analysis of dose-effect within ACB measures (where possible), something not apparent within other systematic reviews. In theory, a clear increase in odds should have been visible in “high” exposure groups compared to “low” throughout scales. Not only was this not visible in odds data for ACBS and ARS when grouped by exposure level, but the inverse was apparent on these two occasions. A contributing factor may have been the tendency for participant numbers to be far lower in “high” exposure categories, yet other clear mechanisms for this have not yet been established. Further advantages were that this study assessed the risk of bias using an appropriate prognostic review risk of bias tool, QUIPS, and the body of evidence was assessed using the GRADE tool (adapted specifically for prognostic studies). This provided a clear understanding of the quality of the current evidence and where improvements in future research can be made. Specifically, there is a need for large-scale cohort studies supported by adequate sample size calculations based on clinically meaningful effect sizes. The use of multiple ACB measures on the same population reduces risk from heterogeneity and would increase confidence in making comparisons across the various ACB measures. Next, if a follow-up period of over one year is used, then repeated ACB measurements would prove useful to control for temporal changes in patients’ anticholinergic exposure. Most studies within the review did not adjust for temporal changes in ACB, yet it is evident that patients’ prescriptions, and therefore ACB, will change over time (Lu et al., 2015), which may have influenced risk.
Our findings provide further evidence of the risks associated with the use of anticholinergic medications. This will help with clinical decision making. Being aware of, and able to discuss available evidence with patients will allow clinicians to make more informed treatment decisions. From a provider perspective, the economic implications of the use of anticholinergic medications are not known and warrant further investigation. At present we do not know how reducing or stopping anticholinergic medications, and/or switching to non-anticholinergic alternatives, may impact upon the costs of patient care. We recommend those designing future trials aiming to de-prescribe anticholinergic medications build economic evaluation into their design.
This systematic review identified twenty studies assessing ACB and mortality using anticholinergic-specific measures in older adults. Meta-analysis indicated an association between anticholinergic exposure and higher risk of mortality using the ACBS, ARS, and ADS. The evidence base was of poor quality overall, with moderate to high risk of bias such that it was not possible to ascertain if any ACB measure was superior to another, though the ACBS generally exhibited larger effect sizes than other ACB measures. Future large-scale high-quality research investigating validated measures is recommended. Meanwhile, this review’s findings further add to the evidence base that clinicians should be cautious around prescribing anticholinergic medications to older persons.
All datasets generated for this study are included in the article/Supplementary Material.
Wrote manuscript: KG-M, CS, RS, MT-R, TQ, YL, and PM. Designed research: CS, RS, TQ, YL, and PM. Performed research: KG-M, CS, RS, MT-R, TQ, and PM. Analyzed data: KG-M, CS, RS, MT-R, TQ, and YL.
This work was supported by The Dunhill Medical Trust (grant number RPGF1806/66) and KG-M received an Aberdeen Summer Research Scholarship funded by the Institute of Applied Health Sciences as part of Aberdeen Clinical Academic Training (ACAT) Programme.
The authors declare that the research was conducted in the absence of any commercial or financial relationships that could be construed as a potential conflict of interest.
We thank Ms. Kaisa Yrjana and Ms. Mitrysha Kishor greatly for their help during the study search and screening phases of this review.
The Supplementary Material for this article can be found online at: https://www.frontiersin.org/articles/10.3389/fphar.2020.00570/full#supplementary-material
Aalto, U. L., Roitto, H. M., Finne-Soveri, H., Kautiainen, H., Pitkälä, K. (2018). Use of anticholinergic drugs and its relationship with psychological well-being and mortality in long-term care facilities in Helsinki. J. Am. Med. Dir Assoc. 19, 511–515. doi: 10.1016/j.jamda.2017.11.013
Agar, M., To, T., Plummer, J., Abernethy, A., Currow, D. C. (2010). Anti-cholinergic load, health care utilization, and survival in people with advanced cancer: a pilot study. J. Palliat Med. 13, 745–752. doi: 10.1089/jpm.2009.0365
Bostock, C. V., Soiza, R. L., Mangoni, A. A. (2013). Associations between different measures of anticholinergic drug exposure and Barthel Index in older hospitalized patients. Ther. Adv. Drug Saf. 4, 235–245. doi: 10.1177/2042098613500689
Boustani, M. A., Campbell, N. L., Munger, S., Maidment, I., Fox, G. C. (2008). Impact of anticholinergics on the aging brain: a review and practical application. Aging Health 4, 311–320. doi: 10.2217/1745509X.4.3.311
Campbell, N., Boustani, M., Limbil, T., Ott, C., Fox, C., Maidment, I., et al. (2009). The cognitive impact of anticholinergics: a clinical review. Clin. Interv. Aging 4, 225. doi: 10.2147/cia.s5358
Carnahan, R. M., Lund, B. C., Perry, P. J., Pollock BG and Culp, K. R. (2006). The anticholinergic drug scale as a measure of drug-related anticholinergic burden: associations with serum anticholinergic activity. J. Clin. Pharmacol. 46, 1481–1486. doi: 10.1177/0091270006292126
Chatterjee, S., Bali, V., Carnahan, R. M., Chen, H., Johnson, M. L., Aparasu, R. R. (2017). Risk of mortality associated with anticholinergic use in elderly nursing home residents with depression. Drug Aging 34, 691–700. doi: 10.1007/s40266-017-0475-5
Chew, M. L., Mulsant, B. H., Pollock, B. G., Lehman, M. E., Greenspan, A., Mahmoud, R. A., et al. (2008). Anticholinergic activity of 107 medications commonly used by older adults. J. Am. Geriatr. Soc. 56 (7), 1333–1341. doi: 10.1111/j.1532-5415.2008.01737.x
Cross, A. J., George, J., Woodward, M. C., Ames, D., Brodaty, H., Wolfe, R., et al. (2017). Potentially inappropriate medication, anticholinergic burden, and mortality in people attending memory clinics. J. Alzheimer’s Dis. 60, 349–358. doi: 10.3233/JAD-170265
Durán, C. E., Azermai, M., Vander Stichele, R. H. (2013). Systematic review of anticholinergic risk scales in older adults. Eur. J. Clin. Pharmacol. 69, 1485–1496. doi: 10.1007/s00228-013-1499-3
Egberts, A., van der Craats, S. T., van Wijk, M. D., Alkilabe, S., van den Bemt, P. M., Mattace-Raso, F. U. (2017). Anticholinergic drug exposure is associated with delirium and postdischarge institutionalization in acutely ill hospitalized older patients. Pharmacol. Res. Perspect. 5, e00310. doi: 10.1002/prp2.310
Fox, C., Richardson, K., Maidment, I. D., Savva, G. M., Matthews, F. E., Smithard, D., et al. (2011). Anticholinergic medication use and cognitive impairment in the older population: the medical research council cognitive function and ageing study. J. Am. Geriatr. Soc. 59, 1477–1483. doi: 10.1111/j.1532-5415.2011.03491.x
Fox, C., Smith, T., Maidment, I., Chan, W. Y., Bua, N., Myint, P. K., et al. (2014). Effect of medications with anti-cholinergic properties on cognitive function, delirium, physical function and mortality: a systematic review. Age Ageing 43, 604–615. doi: 10.1093/ageing/afu096
Geersing, G. J., Bouwmeester, W., Zuithoff, P., Spijker, R., Leeflang, M., Moons, K. (2012). Search filters for finding prognostic and diagnostic prediction studies in Medline to enhance systematic reviews. PloS One 7, e32844. doi: 10.1371/journal.pone.0032844
Gutiérrez-Valencia, M., Martínez-Velilla, N., Vetrano, D. L., Corsonello, A., Lattanzio, F., Ladrón-Arana, S., et al. (2017). Anticholinergic burden and health outcomes among older adults discharged from hospital: results from the CRIME study. Eur. J. Clin. Pharmacol. 73, 1467–1474. doi: 10.1007/s00228-017-2312-5
Guyatt, G. H., Oxman, A. D., Vist, G. E., Kunz, R., Falck-Ytter, Y., Alonso-Coello, P., et al. (2008). GRADE: An emerging consensus on rating quality of evidence and strength of recommendations. BMJ (Clin. Res. Ed.) 336, 924–926. doi: 10.1136/bmj.39489.470347.AD
Han, L., McCusker, J., Cole, M., Abrahamowicz, M., Primeau, F., Elie, M. (2001). Use of medications with anticholinergic effect predicts clinical severity of delirium symptoms in older medical inpatients. Arch. Intern. Med. 161, 1099–1105. doi: 10.1001/archinte.161.8.1099
Hilmer, S. N., Mager, D. E., Simonsick, E. M., Cao, Y., Ling, S. M., Windham, B. G., et al. (2007). A drug burden index to define the functional burden of medications in older people. Arch. Intern. Med. 167 (8), 781–787. doi: 10.1001/archinte.167.8.781
Huguet, A., Hayden, J. A., Stinson, J., McGrath, P. J., Chambers, C. T., Tougas, M. E., et al. (2013). Judging the quality of evidence in reviews of prognostic factor research: adapting the GADE framework. Sys Rev. 2, 71. doi: 10.1186/2046-4053-2-71
Kidd, A. C., Musonda, P., Soiza, R. L., Butchart, C., Lunt, C. J., Pai, Y., et al. (2014). The relationship between total anticholinergic burden (ACB) and early in-patient hospital mortality and length of stay in the oldest old aged 90 years and over admitted with an acute illness. Arch. Gerontol. Geriat 59, 155–161. doi: 10.1016/j.archger.2014.01.006
Klamer, T. T., Wauters, M., Azermai, M., Durán, C., Christiaens, T., Elseviers, M., et al. (2017). A novel scale linking potency and dosage to estimate anticholinergic exposure in older adults: the muscarinic acetylcholinergic receptor ANTagonist exposure scale. Basic Clin. Pharmacol. 120, 582–590. doi: 10.1111/bcpt.12699
Kumpula, E. K., Bell, J. S., Soini, H., Pitkälä, K. H. (2011). Anticholinergic drug use and mortality among residents of long-term care facilities: a prospective cohort study. J. Clin. Pharmacol. 51, 256–263. doi: 10.1177/0091270010368410
Landi, F., Dell’Aquila, G., Collamati, A., Martone, A. M., Zuliani, G., Gasperini, B., et al. (2014). Anticholinergic drug use and negative outcomes among the frail elderly population living in a nursing home. J. Am. Med. Dir Assoc. 15, 825–829. doi: 10.1016/j.jamda.2014.08.002
Lattanzio, F., Corica, F., Schepisi, R., Amantea, D., Bruno, F., Cozza, A., et al. (2018a). Anticholinergic burden and 1-year mortality among older patients discharged from acute care hospital. Geriatr. Gerontol. Int. 18, 705–713. doi: 10.1111/ggi.13234
Lattanzio, F., Onder, G., La Fauci, M. M., Volpato, S., Cherubini, A., Fabbietti, P., et al. (2018b). Anticholinergic burden is associated with increased mortality in older patients with dependency discharged from hospital. J. Am. Med. Dir Assoc. 19, 942–947. doi: 10.1016/j.jamda.2018.06.012
Lowry, E., Woodman, R. J., Soiza, R. L., Mangoni, A. A. (2011). Associations between the anticholinergic risk scale score and physical function: potential implications for adverse outcomes in older hospitalized patients. J. Am. Med. Dir Assoc. 12, 565–572. doi: 10.1016/j.jamda.2011.03.006
Lowry, E., Woodman, R. J., Soiza, R. L., Hilmer, S. N., Mangoni, A. A. (2012). Drug burden index, physical function, and adverse outcomes in older hospitalized patients. J. Clin. Pharmacol. 52, 1584–1591. doi: 10.1177/0091270011421489
Lu, W. H., Wen, Y. W., Chen, L. K., Hsiao, F. Y. (2015). Effect of polypharmacy, potentially inappropriate medications and anticholinergic burden on clinical outcomes: a retrospective cohort study. Can. Med. Assoc. J. 187, E130–E137. doi: 10.1503/cmaj.141219
Mangoni, A. A., van Munster, B. C., Woodman, R. J., de Rooij, S. E. (2013). Measures of anticholinergic drug exposure, serum anticholinergic activity, and all-cause postdischarge mortality in older hospitalized patients with hip fractures. Am. J. Geriat Psychiat. 21, 785–793. doi: 10.1016/j.jagp.2013.01.012
McIsaac, D. I., Wong, C. A., Diep, ,. D., van Walraven, ,. C. (2018). Association of Preoperative Anticholinergic Medication Exposure With Postoperative Healthcare Resource Use and Outcomes: A Population-based Cohort Study. Ann. Surg. 270, 6. doi: 10.1097/SLA.0000000000002765
Myint, P. K., Fox, C., Kwok, C. S., Luben, R. N., Wareham, N. J., Khaw, K. T. (2014). Total anticholinergic burden and risk of mortality and cardiovascular disease over 10 years in 21,636 middle-aged and older men and women of EPIC-Norfolk prospective population study. Age Ageing 44, 219–225. doi: 10.1093/ageing/afu185
O’Mahony, D., Gallagher, P., Ryan, C., Byrne, S., Hamilton, H., Barry, P., et al. (2010). STOPP & START criteria: a new approach to detecting potentially inappropriate prescribing in old age. Eur. Geriatr. Med. 1, 45–51. doi: 10.1016/j.eurger.2010.01.007
Rudolph, J. L., Salow, M. J., Angelini, M. C., McGlinchey, R. E. (2008). The anticholinergic risk scale and anticholinergic adverse effects in older persons. Arch. Intern. Med. 168, 508–513. doi: 10.1001/archinternmed.2007.106
Ruxton, K., Woodman, R. J., Mangoni, A. A. (2015). Drugs with anticholinergic effects and cognitive impairment, falls and all-cause mortality in older adults: A systematic review and meta-analysis. Brit. J. Clin. Pharmacol. 80, 209–220. doi: 10.1111/bcp.12617
Salahudeen, M. S., Duffull, S. B., Nishtala, P. S. (2015). Anticholinergic burden quantified by anticholinergic risk scales and adverse outcomes in older people: a systematic review. BMC Geriatr. 15, 31. doi: 10.1186/s12877-015-0029-9
Sarbacker, G. B., Espino, D. V., Wood, R. C., Oakes, S. L., Anand, D., Markides, K. A. (2017). Total anticholinergic burden and survival within a cohort of elderly Mexican Americans. Geriatr. Gerontol. Int. 17, 1515–1521. doi: 10.1111/ggi.12907
Sevilla-Sánchez, D., Molist-Brunet, N., González-Bueno, J., Solà-Bonada, N., Espaulella-Panicot, J., Codina-Jané, C. (2018). Prevalence, risk factors and adverse outcomes of anticholinergic burden in patients with advanced chronic conditions at hospital admission. Geriatr. Gerontol. Int. 18, 1159–1165. doi: 10.1111/ggi.13330
The American Geriatrics Society Beers Criteria® Update Expert Panel, Fick, D. M., Semla, T. P., Steinman, M., Beizer, J., Brandt, N., et al. (2019). American Geriatrics Society 2019 updated AGS Beers Criteria® for potentially inappropriate medication use in older adults. J. Am. Geriatr. Soc. 67 (4), 674–694. doi: 10.1111/jgs.15767
Vetrano, D. L., La Carpia, D., Grande, G., Casucci, P., Bacelli, T., Bernabei, R., et al. (2016). Anticholinergic medication burden and 5-year risk of hospitalization and death in nursing home elderly residents with coronary artery disease. J. Am. Med. Dir Assoc. 17, 1056–1059. doi: 10.1016/jj.amda.2016.07.012
Keywords: anticholinergics, adverse outcomes, prognostic study, older adults, measurement scales
Citation: Graves-Morris K, Stewart C, Soiza RL, Taylor-Rowan M, Quinn TJ, Loke YK and Myint PK (2020) The Prognostic Value of Anticholinergic Burden Measures in Relation to Mortality in Older Individuals: A Systematic Review and Meta-Analysis. Front. Pharmacol. 11:570. doi: 10.3389/fphar.2020.00570
Received: 19 December 2019; Accepted: 15 April 2020;
Published: 29 April 2020.
Edited by:
Deslypere Jean Paul, CEO Aesculape CRO Singapore and Belsele, SingaporeReviewed by:
Frasia Oosthuizen, University of KwaZulu-Natal, South AfricaCopyright © 2020 Graves-Morris, Stewart, Soiza, Taylor-Rowan, Quinn, Loke and Myint. This is an open-access article distributed under the terms of the Creative Commons Attribution License (CC BY). The use, distribution or reproduction in other forums is permitted, provided the original author(s) and the copyright owner(s) are credited and that the original publication in this journal is cited, in accordance with accepted academic practice. No use, distribution or reproduction is permitted which does not comply with these terms.
*Correspondence: Roy L. Soiza, cm95LnNvaXphQG5ocy5uZXQ=
Disclaimer: All claims expressed in this article are solely those of the authors and do not necessarily represent those of their affiliated organizations, or those of the publisher, the editors and the reviewers. Any product that may be evaluated in this article or claim that may be made by its manufacturer is not guaranteed or endorsed by the publisher.
Research integrity at Frontiers
Learn more about the work of our research integrity team to safeguard the quality of each article we publish.