- 1Heilongjiang Province Land Reclamation Headquarters General Hospital, Heilongjiang Agriculture and Reclamation Bureau, Harbin, China
- 2College of Traditional Chinese Medicine, Jilin Agricultural University, Changchun, China
- 3Department of Orthopedics, The Affiliated First Hospital of Heilongjiang University of Chinese Medicine, Harbin, China
High-throughput liquid chromatography–mass spectrometry (LC-MS)-based metabolomics can provide the holistic analysis of the low molecular weight endogenous metabolites in cells and reflect the changes of cellular regulation and metabolic pathways. Our study designed to reveal the potentially pharmacological effects of dioscin on SW480 rectal cancer cells using nontargeted metabolomics method to probe into small molecular metabolites and pathway changes. After the cell assay of proliferation, apoptosis, migration, and invasion, the dioscin-treated cell samples were prepared for nontargeted metabolomics analysis based on LC-MS tool to describe the metabolic profiles. Dioscin has prevented cell proliferation and accelerated cell apoptosis, and it also inhibited the SW480 rectal cancer cells’ migration and invasion. A total of 22 metabolites were selected as promising biomarkers of pharmacological reaction of dioscin to rectal cancer, and eight highly correlated pathways including D-glutamine and D-glutamate metabolism, pyruvate metabolism, arachidonic acid metabolism, phenylalanine metabolism, tryptophan metabolism, glycolysis or gluconeogenesis, citrate cycle (TCA cycle), and butanoate metabolism were identified. It showed that strategies based on cell metabolomics are helpful tools to discover the small molecular metabolites to elucidate the action mechanism of drug.
Introduction
Rectal carcinoma, as the most universal detrimental gastrointestinal lump between the dentate line and the rectosigmoid junction, brings enormous suffering to patients. It becomes the second dominant reason for mortality of cancer field in the western countries (Zhang et al., 2013a; Siegel et al., 2014). It is reported that rectal cancer is the third main cause of male and female cancer death in America, and the number of patients with rectal cancer grows by about 1.2 million every year all over the world (Schootman et al., 2017). The etiology of rectal cancer is still not very clear, and the incidence is closely linked to the social environment, eating habits, genetic factors, and others. It is generally accepted that superabundant animal fat and protein intake and inadequate dietary fiber intake are high risk factors for rectal cancer (Vermeer et al., 2014; Zhang et al., 2014a). Because of the low location of the disease, it is easy to be diagnosed by digital rectal examination and sigmoidoscopy (Maas et al., 2015). Currently, operation followed by radiation and chemotherapy is a major therapeutic strategy; however, nearly 30% of invalids with stage II rectal cancer suffer from recurrence and die (Deming et al., 2015). Single target drugs or functional products with less pharmacological activity can’t satisfy the multiple pathological changes. In addition, limited efficiency of therapy also leads to drug resistance and severe toxicity (Chan and Giovannucci, 2010; Panczyk, 2014). It is an imperative need to look for original therapeutic options for the handling of rectal cancer.
With the advancement of modern science and the increase in social needs, more and more experts and scholars pay more attention to multitarget drugs (Zhang et al., 2013b; Zhang et al., 2016a). Owing to the ingredients’ diversity and low toxicity, traditional Chinese medicines (TCMs) have been applied in treating a large number of diseases for a long time and achieved marked multiple-target effects (Zhang et al., 2018). Since the item on artemisinin resisting malaria by Youyou Tu, who has obtained the “2015 Nobel Prize in Hysiology or Medicine”, various kinds of active natural product studies that stem from TCMs have been performed to obtain huge advance (Sun et al., 2018) . Dioscin is a steroidal saponin compound in Dioscoreae rhizoma, which is characterized by antitumor, immunomodulatory, anti-inflammatory, hypolipidemic, anti-AIDS, and other biological activities involved in cell processes, metabolic processes, biological regulation, stress response, signaling pathways (Wang et al., 2014; Zhang et al., 2014b; Zhang et al., 2016c; Zhang et al., 2016d). It also has confirmed that diosgenin produced by dioscin metabolism can exert antitumor effects (Zhang et al., 2013c; Zhang et al., 2018). Hence, dioscin is a hopeful multitarget nominee to fight cancer; however, the underlying mechanisms of dioscin on rectal cancer in metabolic level were little known.
Metabolomics is a high-throughput “omics” technique, which offers holistic identification and quantification measurement of all small endogenous metabolites (MW < 1,500 Da), and is affirmed in resisting carcinoma study (Wang et al., 2013). As a final downstream product in vivo, metabolome reflects the changes of enzyme level, cellular regulation, signaling pathway control, genetic variations, and catabolic and anabolic reactions in health and physiopathologic state (Dong et al., 2012). Metabolomics has the ability to simultaneously measure thousands of metabolites to characterize the global and dynamic profiling of disease occurrence and development by distinct association of liquid (LC) and gas (GC) chromatography binded with mass spectrometry (MS) or nuclear magnetic resonance (NMR) (Sun et al., 2013; Zhang et al., 2015a). To date, more valuable information about biomarker findings for forecasting, early diagnosis, accurate curing, and prognosis has been successfully brought about (Wu et al., 2011; Zhang et al., 2014c; Wang et al., 2015; Liu et al., 2018). Metabolomics has its special advantages in the study of complex disease mechanisms and could correlate changes in metabolite content with biological phenotype. In the present study, we explore the potential metabolite biomarkers and pathway for predicting antitumor response of dioscin in SW480 rectal cancer cells and make an attempt to elucidate the protective mechanism of dioscin.
Experimental Method
Chemicals and Reagents
Acetonitrile, methanol, and formic acid in HPLC grade were obtained from Merck (Darmstadt, Germany). Pure water for mobile phase and aqueous solution preparation were obtained from a Milli-Q water ultrapure water system (Millipore, Bedford, MA, USA). Leucine enkephalin was bought from Sigma-Aldrich (St. Louis, MO, USA). Dioscin of over 99% purity was bought from Tianjin Chemical Reagent Co. (Tianjin, China). Human SW480 rectal cancer cell lines were from the England collection of cell cultures (Salisbury, UK). Cell culture reagents RPMI1640 and fetal bovine serum (FBS) were gained from China Langchem INC. (St. Caliun, Shanghai). Dimethyl sulfoxide (DMSO) and MTT (3-(4,5-dimethyl-2-thiazolyl)-2,5-diphenyl-2-H-tetrazolium bromide) were purchased from GIBCO (Piscataway, USA). Viacount cell counting reagent and Annexin V-FITC/PI kit were obtained from Sabinsa Corporation (Piscataway, USA). Transwell chamber was obtained from Roche Applied Science (Roche, Germany). Other reagents were of the highest grade commercially accessible.
Cell Culture
Human rectal cancer cell line SW480 was trained in RPMI-1640 medium (Invitrogen) with 10% fetal bovine serum (FBS). Under an incubator with an atmosphere of 5% CO2 at 37°C, SW480 cells were seeded into a 6-wells culture plate at a density of 1.05 × 106 cells per well and then incubated for 24 hours. Then, cold PBS was applied to rinse the cell medium for three times. The growing cells were treated with dioscin for 24, 48, and 72 h, which the stock solution of dioscin (80 c/μ mol·L−1) was intended in DMSO and deposited at −20°C. The morphological changes of the growth process of SW480 rectal cancer cell were recorded by inverted optical microscope.
Cytobiology Analysis
IMTT Assay for Cell Proliferation Analysis
SW480 rectal cancer cells were placed in 96-well flat-bottom trays for 24 h common promotion. After the medium was replaced, dioscin was dissolved in DMSO and diluted by the RPMI1640 culture solution at the final concentrations of 5 c/μ mol·L−1, 10 c/μ mol·L−1, 20 c/μ mol·L−1, 40 c/μ mol·L−1, 80 c/μ mol·L−1 and respectively incubated for 24h, 48h, and 72 h at 5% CO2, 37°C. Then, the suspension was discarded and the cells were hatched with DMEM containing 5 mg/ml MTT solution for 3 h. The obtained sediment in each well was dismissed with 150 μl DMSO, and rocked until the crystals dissolved. Control cells were performed with the same bulk of DMSO as the same means. Every group was set six parallel samples. The optical density value (OD) was gauged at 540 nm on a microplate reader abide by computational formula as the following: inhibition rate% = (1−OD treatment group/OD control group) × 100%.
Annexin V-FITC/PI Assay for Cell Apoptosis Analysis
According to the kit specification, cell apoptosis of SW480 rectal cancer cells induced by dioscin was determined by the Annexin V fluorescein isothiocyanate (Annexin V-FITC)/propidium iodide (PI) assay. 1.5 × 106/well cells were inoculated in 96-well dishes containing RPMI 1640 solution before dioscin administration for 24, 48, and 72 h at 80 c/μ mol·L−1. Then, the harvested cells were reascended in cold PBS solution including 137 mM NaCl, 2.7 mM KCl, 4.3 mM Na2HPO4-7H2O, 1.4 mM KH2PO4 at pH 7.4 and dyed by Annexin V and PI for 15 min at indoor and dark environment condition. The apoptosis changes and the percentage of apoptosis cells were resolved by FACStar Plus flow cytometer coupled with Flowjo software. The different evident regions of the flow cytometry disperse profile represent cell changing process: lower left region represents movable cells, upper left region represents dead cells, lower right region stands for apoptotic cells in early period, and upper right region stands for apoptotic cells in farther phase. The general apoptosis proportion is the summation of apoptotic cells in the whole process.
Transwell Assay for Cell Migration and Invasion Analysis
50 μl Matrigel prediluted with DMEM medium at the ratio of 1 to 8 is placed on the bottom of the chamber surface of the Transwell at 37°C for 6 h. Human SW480 rectal cancer cells treated with DMEM in logarithmic growth phase for 24 h were inoculated in the upper chamber of the Transwell and then treated with different concentrations of dioscin including 10 c/μmol•L−1, 20 c/μmol•L−1, 40 c/μmol•L−1. After 24 h cultivation, the filter was removed, and the uninjured cells on the surface only were removed by a cotton swab. The chamber was set in a 4% volume paraformaldehyde solution for 15 min, and the volume fraction 0.5% crystal violet solution was stained at room temperature for 20 min. Through three times PBS washing and air drying, the number of cells (N) was counted using a light microscope, which the average number expresses the invasive ability of the tumor cells (inhibition inhibition rate = (1 − N experimental group/N control group) × 100%. Compared with the invasive ability experiment, the activity evaluation of migration in human SW480 rectal cancer cells is performed with matrigel at the bottom of the upper chamber of the Transwell culture dish. The remaining procedure is carried out according to cell invasion experiment (Migration inhibition rate = (1 − N experimental group/N control group) × 100%).
Cell Metabolomics
Sample Preparation
Cells from the control group and different dioscin-treated group were prepared for metabolomics study. 1 × 106 cells in each group were disposed by 20 c/μ mol•L−1 of dioscin for 24, 48, and 72 h, respectively. In order to obtain parallelism and avoid cell cytotoxicity, cells of the control group were managed by the same dose of DMSO with a proper concentration of less than 0.1%. The culture medium was removed after dioscin treatment, and then cells in the control and therapeutic groups were fleetly washed by 3,000 μl of 37°C deionized water and gathered in 5 ml neat pipe. After being off-center at 1,500 rpm for 15 min at 4°C and eliminating the liquid supernatant, the quenched cells collected in 3 ml centrifuge tube were obtained by 1 ml of cold methanol/water (5:1), and then were performed to ultrasonication with 90 kHz ultrasound frequency and 30 W power on ice bath for 5min. The supernatants of the cell fragment were centrifuged at 12,000 rpm for 15 min at 4°C and then were leached using a 0.22 µm filter membrane for instrumental analysis. Quality control (QC) cell specimen was intended by blending 2 µl each sample.
LC–MS Condition
The LC–MS data was fulfilled by an ultrahigh performance liquid chromatography (UPLC) system (1290 series, Agilent Technologies, California, Santa Clara, USA) coupled to high throughput G2Si High-definition mass spectrometry (Agilent 6550, Agilent Technologies, USA) in positive and negative ion modes. Chromatographic separation is achieved in BEH C18 column (2.1 mm × 100 mm, particle size of 1.7 μm) held at 35°C. The optimized gradient mobile phase is composed by A (water: formic acid = 100:0.1) and B (acetonitrile: formic acid = 100:0.1) operated under the linear elution gradient program at 0.8 ml/min flow rate and 2 μl injection volume as follows: 0–1 min, 95% A; 1–2 min, 95–85% A; 2–3 min, 85–70% A; 3–5 min, 70–55% A; 5–8 min, 55–20% A; 8–10 min, 20-0% A, 10–11 min 0–99%, 12–13min 99%. The vintage MS parameters are set as listed below: in positive ion mode ([M + H] + = 556.2771), the desolvation gas flow of 500 L/h, the capillary voltage of 4,000 V, and the cone voltage of 40 V were set; in negative ion mode ([M − H] − = 554.2615), the desolvation gas flow of 400 L/h, the capillary voltage of 2,500 kV, and the cone voltage of 30 V were set. The ion source temperature was set at 150°C and the cone gas flow was fixed at 100 L/h in both modes. During data acquisition, all cell samples were randomly injected, and blank samples were prepared with 80% methanol in water, and QC samples were injected every 8 samples. Leucine-enkephalin as an internal standard was applied to supervise the accurate mass measurement and monitor the reproducibility of the system.
Data Mining and Statistical Analysis
The fresh data of LC/MS from different series were handled though MarkerLynx software (version 4.1, Waters Corporation, MA, USA), which includes retention time (RT), mass range, mass tolerance, intensity threshold, maximum mass, and retention time tolerance. EZinfo 2.0 software is applied for further multivariate data analyses, such as principal component analysis (PCA), orthogonal projection to latent structure-discriminant analysis (OPLS-DA), and variable importance in projection (VIP) score plot. In an unsupervised PCA score plot, metabolic ingredients with higher similarity present the trend of gathering together and the opposite present the trend of separation. OPLS-DA resolution was utilized to comprehend the whole metabolic disorder between untreated cells and dioscin-treated cells, then the S-plot and homologous variable importance in the projection (VIP values) were calculated in the PLS model.
Metabolite peaks with VIP more than 1 and P-value was less than 0.05 in student’s t-test were considered latent biomarkers for more research. A substantiation test was applied to evaluate the legality of the PLS model by contrasting the goodness of fit (R2 and Q2) of the PLS models with the goodness of fit of 200 Y-permutated models. Metabolite identifications were employed by RT, precise MS, MS/MS fragments data, and databases online such as Chempider (http://www.chemspider.com/), HMDB (http://www.hmdb.ca/) as well as KEGG (http://www.genome.jp/kegg/). The pathways and network establishment which are closely associated with dioscin treatment were performed to resolve through MetaboAnalyst 4.0 and ingenuity pathway analysis (IPA) platform for revealing the action mechanism of dioscin on SW480 rectal cancer cells.
Results
The Effect of Dioscin on Cell Proliferation and Apoptosis
In Figure S1A, the morphology of SW480 rectal cancer cells in the control group was in a good growth state under inverted optical microscope. With the increasing treating time, SW480 rectal cancer cells stimulated by dioscin for 24, 48, and 72 h were evidently changed, which brings out the phenomena of cell growth suppression increase including cell volume shrinkage, lower volume, and bigger cell gap. As shown in Figure S1B, when the concentration was less than or equal to 10 c/μmol·L−1, the inhibition rate of the SW480 rectal cancer cells was less than 42%. However, 80 c/μmol·L−1 dioscin in 72 h possessed the highest inhibition rate of 84.5% as the treating time and concentration increased. In Figures S1C, S1D, the total apoptosis rate was 68.52 ± 4.03% at a level of 80 c/μmol·L−1 after dioscin treatment, which displayed considerable statistical differences in comparison with the control group (p < 0.01). Similarly, the scale of apoptosis cells in a time-dependent way tended to the right area after 24, 48, and 72 h at 80 c/μmol·L−1 of dioscin in flow cytometry scatter plots. Cells in the control group were centered on the lower left zone suggesting that there was no cell apoptosis. It is implicit that dioscin has latent aptness to hinder SW480 rectal cancer cell proliferation and accelerate cell apoptosis.
The Effect of Dioscin on Cell Migration and Invasion
In Figure S2A, the numbers of migrated cells were decreased with the increase of concentration, which suggested that dioscin inhibited the migration of SW480 rectal cancer cells in vitro in a concentration-dependent way. Compared with dioscin in 10 μmol•L−1, the number of migrated cells after treatment was significantly reduced in 40 μmol•L−1 (P < 0.01). In Figure S2B, the invasive ability from 10 μmol•L−1 to 40 μmol•L−1 was much lower than that of the control group, suggesting that dioscin inhibited the invasive ability of SW480 rectal cancer cells in vitro. Compared with dioscin in 10 μmol•L−1, the inhibitory ability was increased with increasing concentration, the number of invading cells after treatment was significantly reduced in 40 μmol•L−1 (P < 0.01). It showed that dioscin possessed a latent capability to hinder SW480 rectal cancer cells’ migration and invasion.
Multivariate Statistical Analysis
All the data were resolved in both ion modes using high-throughput metabolomics based on LC-MS conditions described above. Raw data of cell samples were imported into Progenesis QI software for multiple statistical analyses. In Figures 1A and 2A, the PCA score plot of the metabolic route in dioscin-treated sample was clearly discreted, which was distant from the control group orientation, and the bias was the highest at 72 h. Then, a wide multidimensional array including RT, m/z, and peak height intensity between the control group and 72 h dioscin group was taken into EZinfo plug-ins for pattern recognition analysis. In both ion modes (Figure 1B and Figure 2B), OPLS-DA score plots between the control and 72 h treated groups were well separated suggesting that obvious alteration of fluid in the cell had appeared on the metabolic lay after dioscin treatment. The ions farther away from the origin have the greater the contribution for the change of the metabolic profile trajectory in the loading plot (Figures 1C and 2C) and S-plot (Figures 1D and 2D). With the intention of recognizing and seeking potential biomarker closely related with dioscin activity in positive and negative ion modes (Figures 1E and 2E), the VIP score plot presents the donation of the factors to bunch and prejudice.
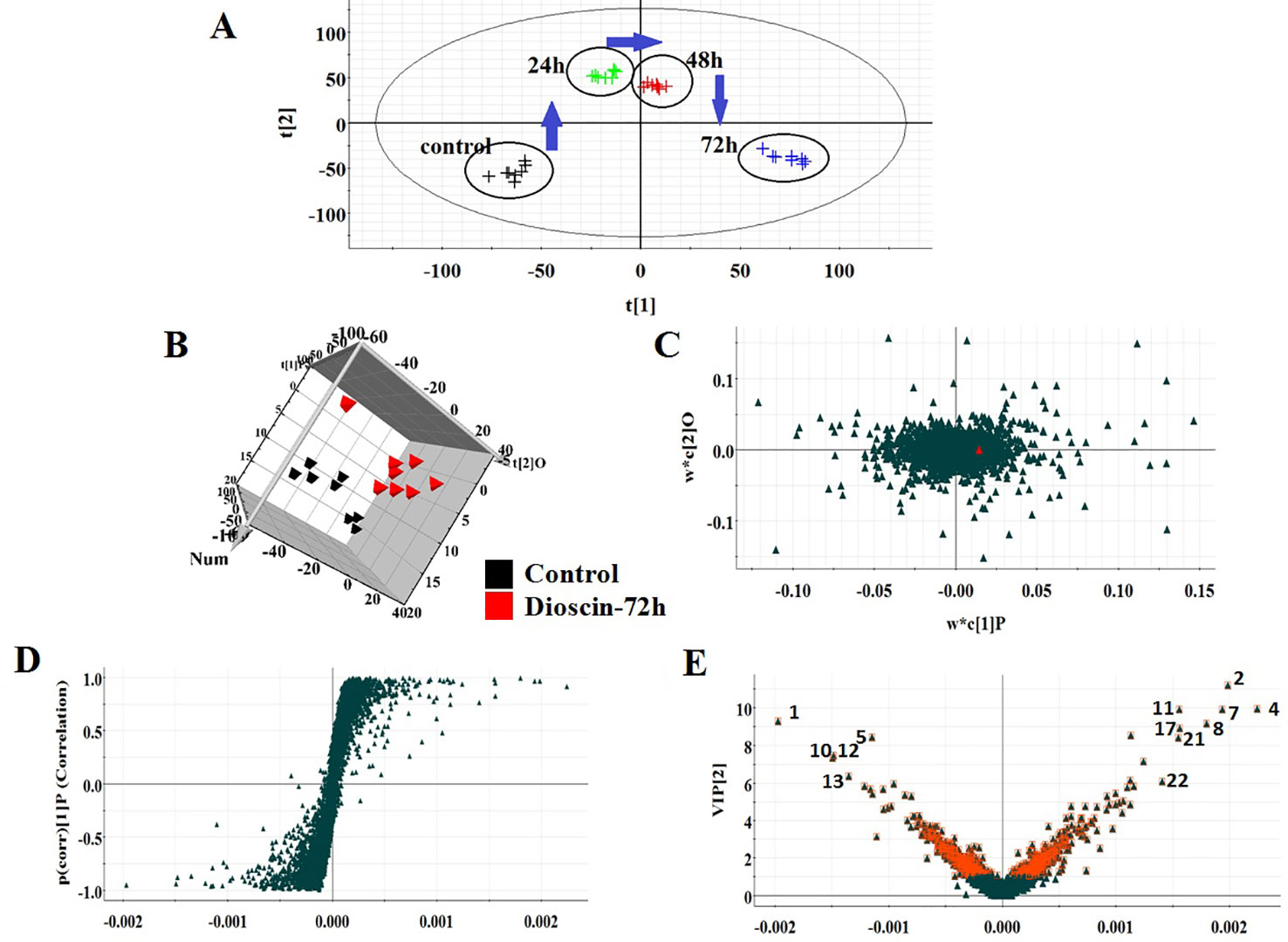
Figure 1 PCA scores’ plot of cell metabolism trajectory in the dioscin-treated group at different time points in positive mode (A). 3D OPLS-DA score plot of cell UPLC-MS spectra data between control and 72 h dioscin-treated group in positive mode (B). Loading-plot of OPLS-DA model of UPLC-MS spectra data between control and 72 h dioscin-treated group in positive mode (C). S-plot of OPLS-DA model of UPLC-MS spectra data between control and 72 h dioscin-treated group in positive mode (D). VIP-plot of OPLS-DA model of UPLC-MS spectra data between control and 72 h dioscin-treated group in positive mode (E).
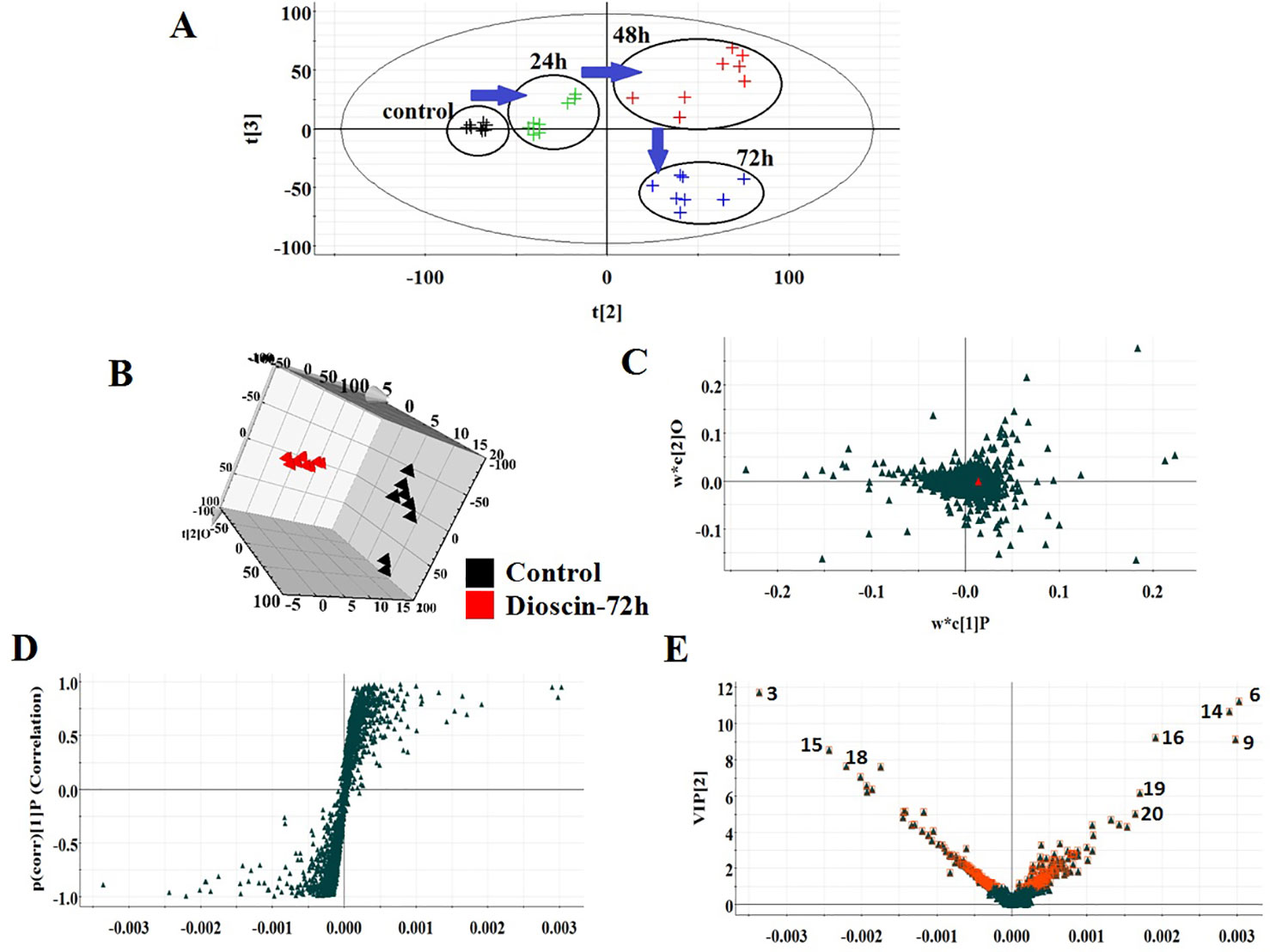
Figure 2 PCA scores’ plot of cell metabolism trajectory in the dioscin-treated group at different time points in negative mode (A). 3D OPLS-DA score plot of cell UPLC-MS spectra data between control and 72 h dioscin-treated group in negative mode (B). Loading-plot of OPLS-DA model of UPLC-MS spectra data between control and 72 h dioscin-treated group in negative mode (C). S-plot of OPLS-DA model of UPLC-MS spectra data between control and 72 h dioscin-treated group in negative mode (D). VIP-plot of OPLS-DA model of UPLC-MS spectra data between control and 72 h dioscin-treated group in negative mode (E).
Differentiated Cell Biomarker Recognition
Using an independent t-test, the metabolites with VIP values were picked from the OPLS-DA which means that P values less than 0.05 were singled out conducing to the clustering differentiation between different groups as potential biomarkers. MS spectrum and relevant MS/MS fragments were used for preliminary analysis, then further, retrieving from online database and searching reference substance were essential to affirm their structure and information. A total of 22 different metabolites were identified in Table S1. As shown in Figure 3, color heatmap indicated that 10 of the 22 biomarkers were upregulated and 12 of these were downregulated after treatment. Comparative indication strength of 22 cell metabolites from UPLC-MS were calculated and listed in Figure 4. Compared with the control group, 10 potential biomarkers, including LysoPC(20:1(11Z)), CPA(16:0/0:0), L-tryptophan, N-a-acetyl-L-arginine, arachidonic acid, prostaglandin A2, L-lactic acid, pyruvic acid, D-glutamic acid, L-phenylalanine, have significant differences for emphasizing the protective effect of dioscin.
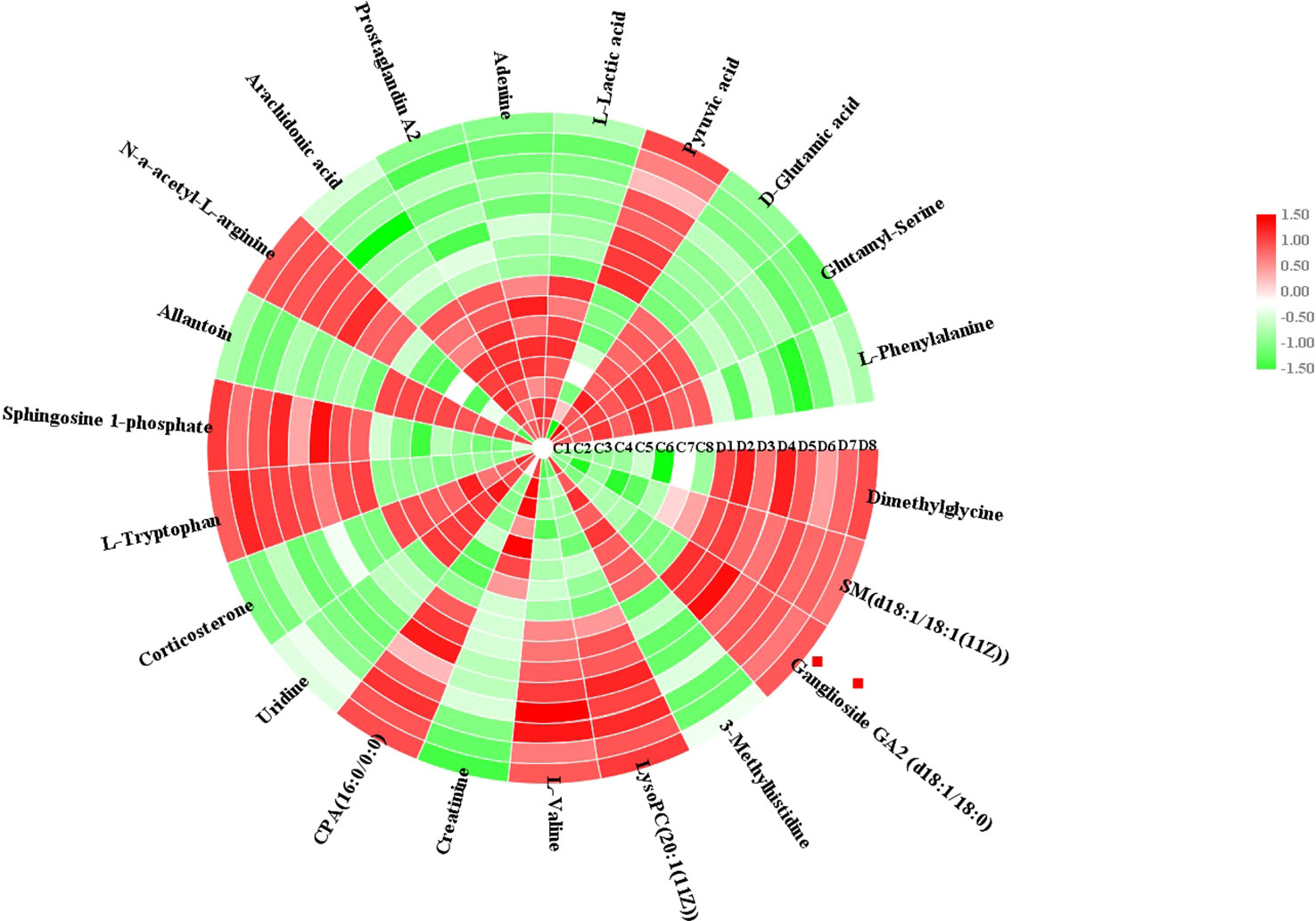
Figure 3 Heatmap visualization of cell biomarker candidates between control and 72 h dioscin administration groups to show significant changes.
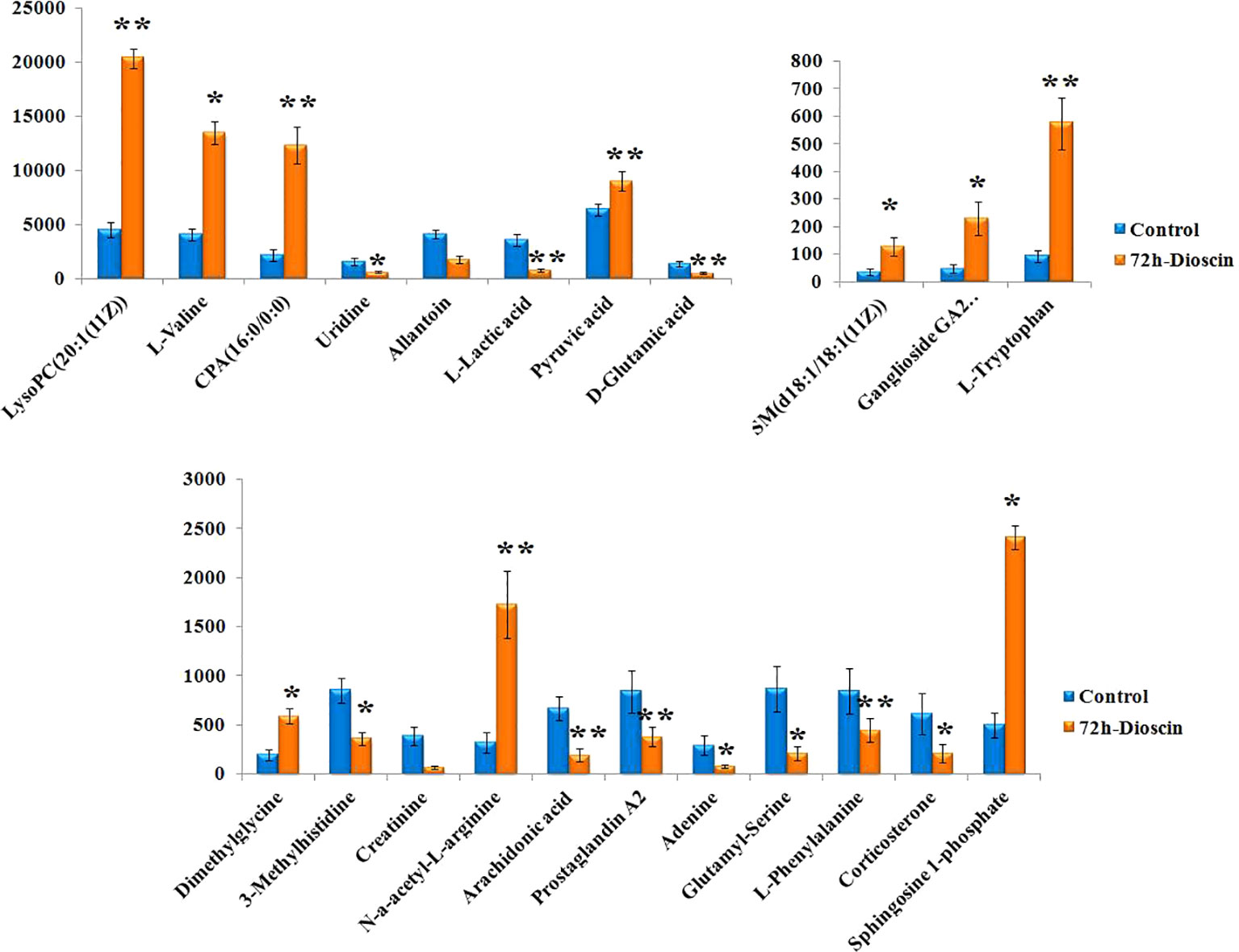
Figure 4 Relative signal intensities for metabolic biomarkers in the cell identified in control and 72 h dioscin administration groups. Compared with control group: *P < 0.05, **P < 0.01.
Metabolic Pathways and Network Establishment
MetaboAnalyst 4.0 (http://www.metaboanalyst.ca/) was utilized to perform pathway topology analysis and network establishment related to the conditions being studied. It was shown that metabolites regulated by dioscin on rectal cancer were involved in eight key metabolic pathways as follows: D-glutamine and D-glutamate metabolism, pyruvate metabolism, arachidonic acid metabolism, phenylalanine metabolism, tryptophan metabolism, glycolysis or gluconeogenesis, citrate cycle (TCA cycle), and butanoate metabolism (Figure 5). As shown in the KEGG global metabolic network, the distributional range of metabolites and enzymes/KEGG Orthologs referred to amino acid metabolism, sphingolipid metabolism, aminoacyl-tRNA biosynthesis, pantothenate, and CoA biosynthesis, glycolysis or Gluconeogenesis, pyruvate metabolism, vitamin B6 metabolism, propanoate metabolism, nitrogen metabolism is depicted (Figure S3A). Metabolite–metabolite interaction network highlights the potential functional relationships such as arachidonic acid, pyruvic acid, D-glutamic acid, L-phenylalanine, L-tryptophan, and adenine, which possess similar chemical structures leading to similar molecular activities (Figure S3B). In addition, the HMDB information of these 22 metabolites was imported into IPA software for establishing merging metabolic networks to illuminate molecular mechanism of dioscin on SW480 rectal cancer cell, which was concerned with molecule transport, nucleic acid metabolism, molecule biochemistry, cell signaling and interplay, cellular growth and proliferation, cellular compromise (Figure 6).
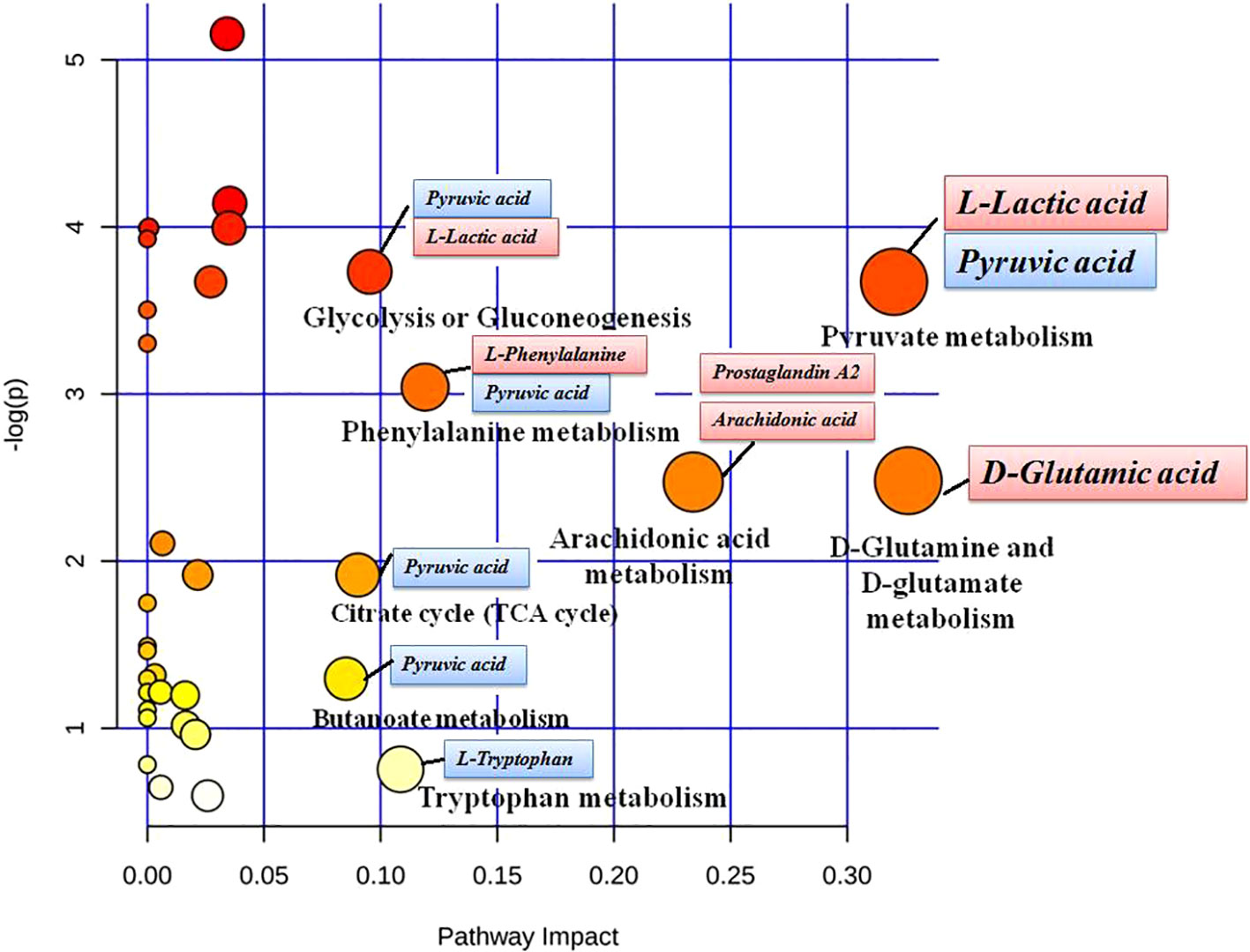
Figure 5 Pathway analysis of metabolic variations after dioscin treatment in cell. The ordinate is the original P values obtained from the pathway analysis, and the abscissa is the influence value of pathways obtained from the topological analysis. They are represented by the depth and size of the color of the circle.
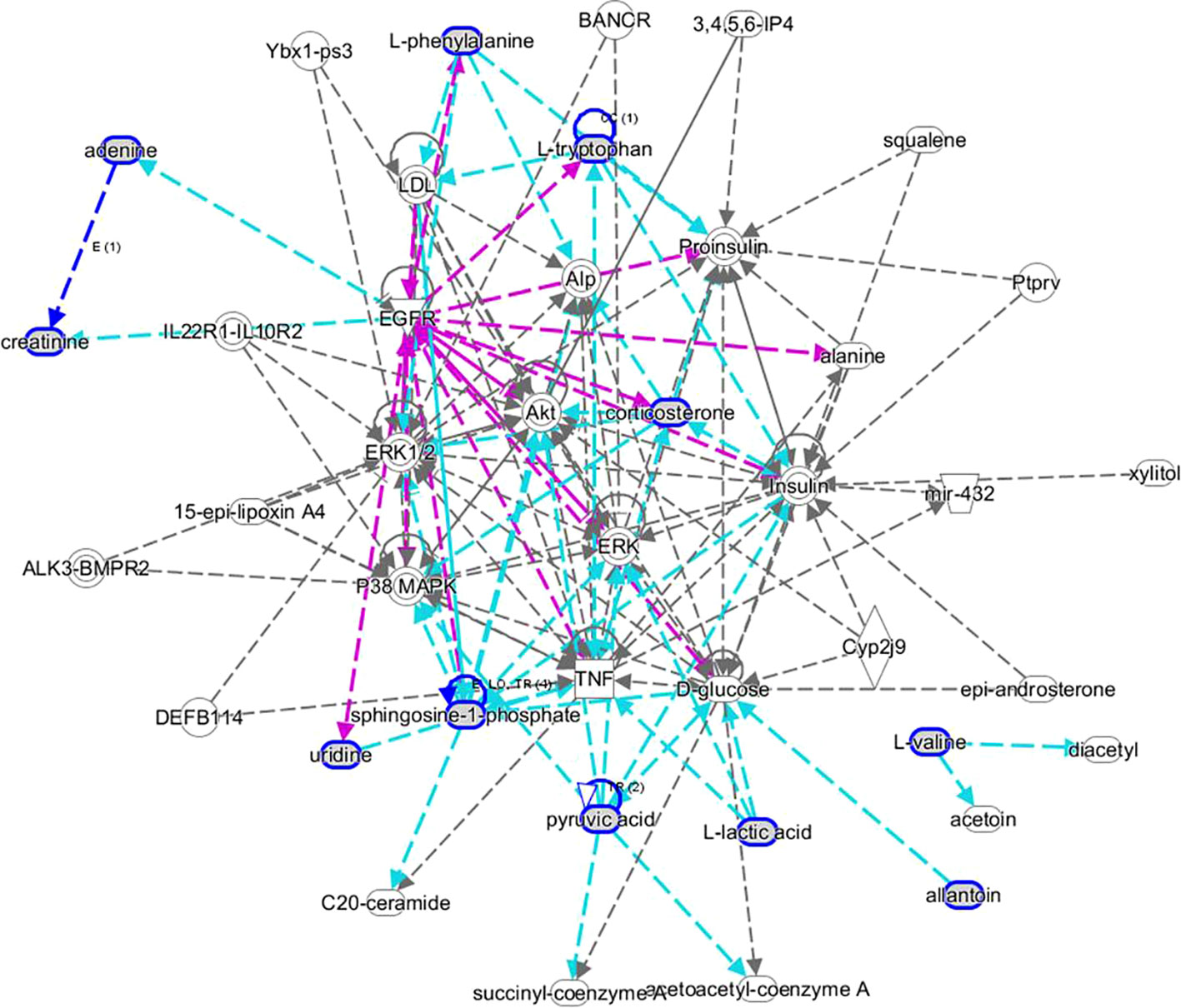
Figure 6 IPA prediction networks associated with dioscin protective activity on SW480 rectal cancer cells.
Discussion
Cancer caused by a combination of various factors is characterized by uncontrolled growth and spread of abnormal cells or tissues, which is next only to cardiovascular disease. It is reported that more than 16 million new cancer cases by 2020 and 10 million people will face the threat of death (Liu et al., 2009). To date, about 60% of anticancer agents and 75% of anti-infectious agents are derived from natural products, and more than 30 natural-derived compounds are used in clinical treatment of various stages of cancer (Das and Satyalakshmi, 2012). The most studied of the natural products include taxol, camptothecin, curcumin, betulinic acid, podophyllotoxin, and resveratrol.
Dioscin is an anticancer natural product in recent years (Brahmbhatt et al., 2015; Vassilakopoulou et al., 2015; Si et al., 2016). Glucose is firstly decomposed into pyruvic acid without aerobic action, and then a tricarboxylic acid (TCA) cycle is performed in the mitochondria to produce a large amount of ATP. However, in the 1950s, Warburg (Sun et al., 2018b) reported that pyruvate decomposed by glucose in tumor cells is converted to lactic acid in the presence of oxygen rather than in the mitochondria. Tumor cells use the metabolic pathways of glucose and its derivatives to produce the green medium needed for their biosynthesis, maintain a good redox circumstance, and meet their required vitality. Pyruvate dehydrogenase (PDH) is a key regulator of pyruvate, which varies pyruvate to acetyl-CoA in the mitochondria. As a vital conditioner of PDH activity is PDH kinase (PDK), it promotes the inactivation of PDH by promoting phosphorylation of specific sites in serine (Zhang et al., 2018), resulting in a decrease in the amount of pyruvate entering the mitochondria and an increase in the amount of lactic acid. Compared with the control group, the level of L-lactic acid is downregulated and the content of pyruvic acid is upregulated after dioscin treatment, which indicated that dioscin monitors pyruvate metabolism for inhibiting abnormal conversion among sugars, proteins, and lipids. In addition to altering glucose metabolism, tumor cells also increase the application and dependence of glutamine to facilitate cell growth and survival. The dramatic increase in glutamine is closely associated with the metabolism of oncogene c-Myc signaling in tumors (Gao et al., 2009) and other oncogene variants, such as K-ras (Son et al., 2013). In the cytosol, glutamine can be employed as a substrate for de novo synthesis of proteins, purines, and pyrimidines and can be varied to glutamic acid by glutaminases (GLS). Tumor cells utilize glutamine-derived glutamic acid to perform various activities (Hensley et al., 2013).
As shown in the above result, the level of D-glutamic acid is increased in the control group suggesting that abnormal activity in the pathogenic period of tumor cells has been employed to maintain growth and survival, and then dioscin validly reverses D-glutamine and D-glutamate metabolism. Phenylalanine is one of the eight essential amino acids in the human body, which referred to the formation of various protein components but cannot be synthesized in the human body. Under normal circumstances, about 50% of the ingested phenylalanine is used to synthesize various components of the protein, and the rest is changed to tyrosine by the action of phenylalanine hydroxylase and then converted to dopa, dopamine, adrenaline, norepinephrine and melanin by other enzymes. Phenylalanine hydroxylase is a complex enzyme system including dihydropterin reductase and coenzyme tetrahydrobiopterin. An enzyme deficiency can induce an increase in blood phenylalanine. In the control group, the content of phenylalanine was increased, and dioscin regulated them close to the abnormal level. Activated arachidonic acid (AA) metabolic enzymes and their products arrange the inflammatory reply and adjust multiple cellular procedures such as cell proliferation, survival, penetration, and pervasion, which accelerates carcinogenesis and plays a significant role in inflammation and tumorigenesis. Some studies have suggested that natural as well as synthetical molecules lead to pharmacological prophylaxis of the AA pathway, which stimulates researchers to probe into therapeutic intervention of the AA pathway (Wang and Dubois, 2010; Li et al., 2010). As a cyclopentenone compound and an endogenous metabolite of arachidonic acid, prostaglandin A has ability to resist cell proliferation in vivo and in vitro (Zhang et al., 2014d). The upregulated level of AA and prostaglandin A is attributed to self-protection of SW480 rectal cancer cells in the inflammatory response. Dioscin exhibit reverses the content of AA and prostaglandin a mediated in AA metabolism.
Metabolomics approach plays a key role in researching the metabolic disorder-related diseases (Zhang et al., 2013d; Zhang et al., 2014e; Zhang et al., 2015b; Sun et al., 2015; Wang et al., 2016; Zhao et al., 2016; Chu et al., 2016; Song et al., 2017; Ren et al., 2018; Qiu et al., 2020), discovery and identification of biomarkers (Zhang et al., 2014f) and treatment (Wang et al., 2012; Liang et al., 2015; Zhang et al., 2016b; Zhao et al., 2017; Liu et al., 2018; Sun et al., 2019; Wu et al., 2019; Qiu et al., 2020). Many biomarkers are being discovered for better understanding of metabolic mechanisms (Ma et al., 2016; Wang et al., 2016; Li et al., 2018) and evaluating the efficacy diseases (Chu et al., 2015a; Chu et al., 2015b; Wang et al., 2016; Zhang et al., 2017). These emerging candidate biomarkers own a promising future, and numerous studies are indispensable to validation of diseases. Our study showed that strategies based on cell metabolomics are helpful tools to discover the small molecular metabolites to elucidate the action mechanism of drug.
Conclusion
In this study, we used cell metabolomics strategy to explore the effect of dioscin on rectal cancer cells and to discover the small molecular metabolites for elucidating the action mechanism of dioscin. A total of twenty-two differentiated biomarkers were identified with distinct changes in rectal cancer cells. The metabolic mechanism of dioscin on SW480 rectal cancer cells was related to D-glutamine and D-glutamate metabolism, pyruvate metabolism, and arachidonic acid metabolism, respectively. It was implied that cell metabolomics is a beneficial tool to further promote the development of dioscin as antitumor drugs.
Data Availability Statement
All datasets generated for this study are included in the article/Supplementary Material.
Author Contributions
The list of authors who contributed to this work is as follows: X-XW conceived and designed the experiments. X-XW, P-CY, and JL performed the experiment. X-XW analyzed the data. JL guided the experiment. X-XW wrote the paper. P-CY revised the paper. All authors read and approved the final manuscript.
Conflict of Interest
The authors declare that the research was conducted in the absence of any commercial or financial relationships that could be construed as a potential conflict of interest.
The reviewer XW declared a shared affiliation, with no collaboration, with one of the authors JL to the handling editor at time of review.
Supplementary Material
The Supplementary Material for this article can be found online at: https://www.frontiersin.org/articles/10.3389/fphar.2020.00068/full#supplementary-material
References
Brahmbhatt, H., Oppermann, S., Osterlund, E. J., Leber, B., Andrews, D. W. (2015). Molecular pathways: leveraging the BCL-2 interactome to kill cancer cells-mitochondrial outer membrane permeabilization and beyond. Clin. Cancer Res. Off. J. Am. Assoc. Cancer Res. 21 (12), 2671–2676. doi: 10.1158/1078-0432.CCR-14-0959
Chan, A. T., Giovannucci, E. L. (2010). Primary prevention of colorectal cancer. Gastroenterology 138 (6), 2029–2043. doi: 10.1053/j.gastro.2010.01.057
Chu, H., Zhang, A. H., Han, Y., Wang, X. (2015a). Metabolomics and its potential in drug discovery anddevelopment from TCM. World J.Tradit. Chin. Med. 4, 26–32. doi: 10.15806/j.issn.2311-8571.2015.0022
Chu, H., Sun, H., Yan, G. L., Zhang, A. H., Liu, C., Dong, H., et al. (2015b). Metabolomics analysis of health functions of Physalis Pubescens L. using by ultra-performance liquid chromatography/electrospray ionization quadruple time-of-flight mass spectrometry. World J. Tradit. Chin. Med. 3, 09–20. doi: 10.15806/j.issn.2311-8571.2015.0015
Chu, H., Zhang, A., Han, Y., Lu, S., Kong, L., Han, J., et al, et al. (2016). Metabolomics approach to explore the effects of Kai-Xin-San on Alzheimer’s disease using UPLC/ESI-Q-TOF mass spectrometry. J. Chromatogr. B. 1015, 50–61. doi: 10.1016/j.jchromb.2016.02.007
Das, B., Satyalakshmi, G. (2012). Natural products based anticancer agents. Mini-Rev. Org. Chem. 9 (2), 169–177. doi: 10.2174/157019312800604706
Deming, D., Uboha, N., Zafar, S. Y., Rosenberg, S., Bassetti, M., Glasgow, S., et al. (2015). Adjuvant chemotherapy for stage II rectal cancer. Semin. Oncol. 42 (6), e99–107. doi: 10.1053/j.seminoncol.2015.09.033
Dong, W., Wang, P., Meng, X., Sun, H., Zhang, A., Wang, W., et al. (2012). Ultra-performance liquid chromatography–high-definition mass spectrometry analysis of constituents in the root of radix stemonae and those absorbed in blood after oral administration of the extract of the crude drug. Phytochem. Anal. 23 (6), 657–667. doi: 10.1002/pca.2370
Gao, P., Tchernyshyov, I., Chang, T. C., Lee, Y. S., Kita, K., Ochi, T., et al. (2009). c-Myc Suppression of miR-23a/b enhances mitochondrial glutaminase expression and glutamine metabolism. Nature 458 (7239), 762–765. doi: 10.1038/nature07823
Hensley, C. T., Wasti, A. T., DeBerardinis, R. J. (2013). Glutamine and cancer: cell biology, physiology, and clinical opportunities. J. Clin. Invest. 123 (9), 3678–3684. doi: 10.1172/JCI69600
Li, Y. F., Qiu, S., Gao, L. J., Zhang, A. H. (2018). Metabolomic estimation of the diagnosis of hepatocellular carcinoma based on ultrahigh performance liquid chromatography coupled with time-of-flight mass spectrometry. RSC Adv. 8 (17), 9375–9382. doi: 10.1039/C7RA13616A
Li, W. X., Zhang, A. H., Zhou, X. H., Nan, Y., Liu, Q., Sun, H., et al. (2020). High-throughput liquid chromatography mass-spectrometry-driven lipidomics discover metabolic biomarkers and pathways as promising targets to reveal the therapeutic effects of the Shenqi pill. RSC Adv. 10 (4), 2347-2358.
Liang, Q., Wang, C., Li, B., Zhang, A. H. (2015). Metabolic footprinting to understand therapeutic effects and mechanisms of silybin on acute liver damage in rat. Pharmacogn. Mag. ,11 (43), 586–593. doi: 10.4103/0973-1296.160469
Liu, E. H., Qi, L. W., Wu, Q., Peng, Y. B., Li, P. (2009). Anticancer agents derived from natural products. Mini Rev. Med. Chem. 9 (13), 1547–1555. doi: 10.2174/138955709790361520
Liu, X. Y., Zhang, A. H., Fang, H., Li, M. X., Song, Q., Su, J., et al. (2018). Serum metabolomics strategy for understanding the therapeutic effects of Yin-Chen-Hao-Tang against Yanghuang syndrome. RSC Adv. 8 (14), 7403–7413. doi: 10.1039/C7RA11048K
Ma, W. W., Zhang, A. H., Sun, H., Meng, X. C., Wang, X. (2016). Metabolomics deciphering therapeutic effects and mechanism of traditional chinese medicines. World J. Tradit. Chin. Med. 2(3), 17–24. doi: 10.15806/j.issn.2311-8571.2016.0027
Maas, M., Lambregts, D. M., Nelemans, P. J., Heijnen, L. A., Martens, M. H., Leijtens, J. W., et al. (2015). Assessment of clinical complete response after chemoradiation for rectal cancer with digital rectal examination, endoscopy, and MRI: selection for organ-saving treatment. Ann. Surg. Oncol. 22 (12), 3873–3880. doi: 10.1245/s10434-015-4687-9
Panczyk, M. (2014). Pharmacogenetics research on chemotherapy resistance in colorectal cancer over the last 20 years. World J. Gastroenterol. 20 (29), 9775–9827. doi: 10.3748/wjg.v20.i29.9775
Qiu, S., Zhang, A. H., Guan, Y., Sun, H., Zhang, T. L., Han, Y., et al. (2020). Functional metabolomics using UPLC-Q/TOF-MS combined with ingenuity pathway analysis as a promising strategy for evaluating the efficacy and discovering amino acid metabolism as a potential therapeutic mechanism-related target for geniposide against alcoholic liver disease. RSC Advances. 10 (5), 2677-2690.
Ren, J. L., Zhang, A. H., Kong, L., Wang, X. J. (2018). Advances in mass spectrometry-based metabolomics for investigation of metabolites. RSC Adv. 8 (40), 22335–22350. doi: 10.1039/C8RA01574K
Schootman, M., Gomez, S. L., Henry, K. A., Paskett, E. D., Ellison, G. L., Oh, A., et al. (2017). Geospatial approaches to cancer control and population sciences. Cancer Epidemiol. Biomarkers Prev. 26 (4), 472–475. doi: 10.1158/1055-9965.EPI-17-0104
Si, L., Zheng, L., Xu, L., Yin, L., Han, X., Qi, Y., et al. (2016). Dioscin suppresses human laryngeal cancer cells growth via induction of cell-cycle arrest and MAPK-mediated mitochondrial-derived apoptosis and inhibition of tumor invasion. Eur. J. Pharmacol. 13 (4), 105–117. doi: 10.1016/j.ejphar.2016.02.009
Siegel, R., DeSantis, C., Jemal, A. (2014). Colorectal Cancer Statistics. CA Cancer J. Clin. 64 (2), 104–117. doi: 10.3322/caac.21220
Son, J., Lyssiotis, C. A., Ying, H., Wang, X., Hua, S., Ligorio, M., et al. (2013). Glutamine supports pancreatic cancer growth through a KRAS-regulated metabolic pathway. Nature 496 (7443), 101–105. doi: 10.1038/nature12040
Song, Q., Zhang, A. H., Yan, G. L., Liu, L., Wang, X. J. (2017). Technological advances in current metabolomics and its application in tradition Chinese medicine. RSC Adv. 7 (84), 53516–53524. doi: 10.1039/C7RA02056B
Sun, H., Wang, M., Zhang, A., Ni, B., Dong, H., Wang, X. (2013). UPLC–Q-TOF–HDMS analysis of constituents in the root of two kinds of aconitum using a metabolomics approach. Phytochem. Anal. 24 (3), 263–276. doi: 10.1002/pca.2407
Sun, H., Wang, H., Zhang, A., Yan, G., Zhang, Y., An, N., Wang, X. (2015). Berberine ameliorates nonbacterial prostatitis via multi-target metabolic network regulation. Omics A J. Integr. Biol. 19 (3), 186–195. doi: 10.1089/omi.2014.0112
Sun, H., Zhang, A. H., Song, Q., Fang, H., Liu, X. Y., Su, J., et al. (2018a). Functional metabolomics discover pentose and glucuronate interconversion pathways as promising targets for Yang Huang syndrome treatment with Yinchenhao Tang. RSC Adv. 8 (64), 36831–36839. doi: 10.1039/C8RA06553E
Sun, H., Zhang, A. H., Liu, S. B., Qiu, S., Li, X. N., Zhang, T. L., et al. (2018b). Cell metabolomics identify regulatory pathways and targets of magnoline against prostate cancer. J. Chromatogr. B. Anal. Technol. BioMed. Life Sci. 1102-1103, 143–151. doi: 10.1016/j.jchromb.2018.10.017
Sun, H., Li, X. N., Zhang, A. H., Zhang, K. M., Yan, G. L., Han, Y., et al. (2019). Exploring potential biomarkers of coronary heart disease treated by Jing Zhi Guan Xin Pian using high-throughput metabolomics. RSC Adv. 9 (20), 11420–11432. doi: 10.1039/C8RA10557J
Vassilakopoulou, M., Psyrri, A., Argiris, A. (2015). Targeting angiogenesis in head and neck cancer. Oncol. 51 (5), 409–415. doi: 10.1016/j.oraloncology.2015.01.006
Vermeer, T. A., Orsini, R. G., Daams, F., Nieuwenhuijzen, G. A., Rutten, H. J. (2014). Anastomotic leakage and presacral abscess formation after locally advanced rectal cancer surgery: incidence, risk factors and treatment. Eur. J. Surg. Oncol. 40 (11), 1502–1509. doi: 10.1016/j.ejso.2014.03.019
Wang, D., DuBois, R. N. (2010). Eicosanoids and cancer. Nat. Rev. Cancer 10, 181–193. doi: 10.1038/nrc2809
Wang, X., Zhang, A., Sun, H. (2012). Future perspectives of Chinese medical formulae: chinmedomics as an effector. OMICS 16 (7-8), 414–421. doi: 10.1089/omi.2011.0138
Wang, X., Wang, Q., Zhang, A., Zhang, F., Zhang, H., Sun, H., et al. (2013). Metabolomics study of intervention effects of Wen-Xin-Formula using ultra high-performance liquid chromatography/mass spectrometry coupled with pattern recognition approach. J. Pharm. Biomed. Anal. 74, 22–30. doi: 10.1016/j.jpba.2012.10.009
Wang, X., Lv, H., Zhang, A., Sun, W., Liu, L., Wang, P., et al. (2014). Metabolite profiling and pathway analysis of acute hepatitis rats by UPLC-ESI MS combined with pattern recognition methods. Liver Int. 34 (5), 759–770. doi: 10.1111/liv.12301
Wang, X., Han, Y., Zhang, A., Sun, H. (2015). Metabolic profiling provides a system for the understanding of Alzheimer"s disease in rats post-treatment with Kaixin San. Chinmedomics (London: Elsevier Academic Press), 347–362.
Wang, X., Zhang, A., Sun, H., Han, Y., Yan, G. (2016). Discovery and development of innovative drug from traditional medicine by integrated chinmedomics strategies in the post-genomic era. TrAC Trends in Anal. Chem. 76, 86–94. doi: 10.1016/j.trac.2015.11.010
Wang, X., Li, J., Zhang, A. H. (2016). Urine metabolic phenotypes analysis of extrahepatic cholangiocarcinoma disease using ultra-high performance liquid chromatography-mass spectrometry. RSC Adv. 6 (67), 63049–63057. doi: 10.1039/C6RA09430A
Wu, F., Sun, H., Wei, W., Han, Y., Wang, P., Dong, T., et al. (2011). Rapid and global detection and characterization of the constituents in ShengMai San by ultra-performance liquid chromatography–high-definition mass spectrometry. J. Sep. Sci. 34 (22), 3194–3199. doi: 10.1002/jssc.201100253
Wu, X. H., Sun, X. L., Zhao, C., Zhang, J. Q., Wang, X., Zhang, A. H., et al. (2019). Exploring the pharmacological effects and potential targets of paeoniflorin on the endometriosis of cold coagulation and blood stasis model rats by ultra-performance liquid chromatography tandem mass spectrometry with a pattern recognition a pproach. RSC Adv. 9 (36), 20796–20805. doi: 10.1039/C9RA03525G
Zhang, A. H., Sun, H., Qiu, S., Wang, X. J. (2013a). Metabolomics in noninvasive breast cancer. Clin. Chim. Acta 424, 3–7. doi: 10.1016/j.cca.2013.05.003
Zhang, A. H., Sun, H., Qiu, S., Wang, X. J. (2013b). Recent highlights of metabolomics in chinese medicine syndrome research. Evid. Based Complement Alter. Med. 2013, 402159. doi: 10.1155/2013/402159
Zhang, A., Sun, H., Qiu, S., Wang, X. (2013c). Advancing drug discovery and development from active constituents of yinchenhao tang, a famous traditional chinese medicine formula. Evidence-Based Complementary Altern. Med. 2013, 257909. doi: 10.1155/2013/257909
Zhang, A., Sun, H., Yan, G., Han, Y., Ye, Y., Wang, X. (2013d). Urinary metabolic profiling identifies a key role for glycocholic acid in human liver cancer by ultra-performance liquid-chromatography coupled with high-definition mass spectrometry. Clin. Chim. Acta 418, 86–90. doi: 10.1016/j.cca.2012.12.024
Zhang, A., Sun, H., Yan, G., Wang, P., Han, Y., Wang, X. (2014a). Metabolomics in diagnosis and biomarker discovery of colorectal cancer. Cancer Lett. 345 (1), 17–20. doi: 10.1016/j.canlet.2013.11.011
Zhang, A., Sun, H., Yan, G., Han, Y., Wang, X. J. (2014b). Metabolomics analysis of marker metabolites for patients with pancreatic cancer. Med. Chem. 4, 506–510. doi: 10.4172/2161-0444.1000186
Zhang, A., Sun, H., Wang, X. (2014c). Urinary metabolic profiling of rat models revealed protective function of scoparone against alcohol induced hepatotoxicity. Sci. Rep. 4, 6768. doi: 10.1038/srep06768
Zhang, A. H., Sun, H., Yan, G. L., Yuan, Y., Han, Y., Wang, X. J. (2014d). Metabolomics study of type 2 diabetes using ultra-performance LC-ESI/quadrupole-TOF high-definition MS coupled with pattern recognition methods. J. Physiol. Biochem. 70 (1), 117–128. doi: 10.1007/s13105-013-0286-z
Zhang, A., Yan, G., Han, Y., Wang, X. (2014e). Metabolomics approaches and applications in prostate cancer research. Appl. Biochem. Biotechnol. 174 (1), 6–12. doi: 10.1007/s12010-014-0955-6
Zhang, A. H., Qiu, S., Xu, H. Y., Sun, H., Wang, X. J. (2014f). Metabolomics in diabetes. Clin. Chim. Acta 429, 106–110. doi: 10.1016/j.cca.2013.11.037
Zhang, A., Sun, H., Sun, W., Wang, X. (2015a). Metabolomics and Proteomics Annotate Therapeutic Mechanisms of Geniposide. Chinmedomics (London: Elsevier Academic Press), 157–173.
Zhang, A., Wang, H., Sun, H., Zhang, Y., An, N., Yan, G., et al. (2015b). Metabolomics strategy reveals therapeutical assessment of limonin on nonbacterial prostatitis. Food Funct. 6 (11), 3540–3549. doi: 10.1039/C5FO00489F
Zhang, A., Sun, H., Yan, G., Wang, P., Wang, X. (2016a). Mass spectrometry-based metabolomics: applications to biomarker and metabolic pathway research. BioMed. Chromatogr. 30 (1), 7–12. doi: 10.1002/bmc.3453
Zhang, T., Zhang, A., Qiu, S., Sun, H., Han, Y., Guan, Y., et al. (2016c). High-throughput metabolomics approach reveals new mechanistic insights for drug response of phenotypes of geniposide towards alcohol-induced liver injury by using liquid chromatography coupled to high resolution mass spectrometry. Mol. Biosyst. ,13 (1), 73–82. doi: 10.1039/c6mb00742b
Zhang, T., Zhang, A., Qiu, S., Yang, S., Wang, X. (2016d). Current trends and innovations in bioanalytical techniques of metabolomics. Crit. Rev. Anal. Chem. 46 (4), 342–351. doi: 10.1080/10408347.2015.1079475
Zhang, A., Qiu, S., Sun, H., Zhang, T., Guan, Y., Han, Y., et al. (2016b). Scoparone affects lipid metabolism in primary hepatocytes using lipidomics. Sci. Rep. 6(1), 1–8. 28031. doi: 10.1038/srep28031
Zhang, Y., Liu, P., Li, Y., Zhang, A. H. (2017). Exploration of metabolite signatures using high-throughput mass spectrometry coupled with multivariate data analysis. RSC Adv. 7 (11), 6780–6787. doi: 10.1039/C6RA27461G
Zhang, A. H., Yu, J. B., Sun, H., Kong, L., Wang, X. Q., Zhang, Q. Y., et al. (2018). Identifying quality-markers from Shengmai San protects against transgenic mouse model of Alzheimer’s disease using chinmedomics approach. Phytomedicine 45, 84–92. doi: 10.1016/j.phymed.2018.04.004
Zhang, A. H., Sun, H., Yan, G. L., Han, Y., Zhao, Q. Q., Wang, X. J. (2019). Chinmedomics: a powerful approach integrating metabolomics with serum pharmacochemistry to evaluate the efficacy of traditional chinese medicine. Engineering. 5 (1), 60–68. doi: 10.1016/j.eng.2018.11.008
Zhang, A., Sun, H., Wang, X. (2018b). Mass spectrometry-driven drug discovery for development of herbal medicine. Mass. Spectrom. Rev. 37 (3), 307–320. doi: 10.1002/mas.21529
Zhao, Q., Zhang, A., Zong, W., An, N., Zhang, H., Luan, Y., et al. (2016). Chemometrics strategy coupled with high resolution mass spectrometry for analyzing and interpreting comprehensive metabolomic characterization of hyperlipemia. RSC Adv. 6 (113), 112534–112543. doi: 10.1039/C6RA24267G
Keywords: metabolomics, biomarker, natural product, metabolic pathways, mass spectrometry
Citation: Wang X-X, Yu P-c and Li J (2020) High-Throughput Metabolomics for Identification of Metabolic Pathways and Deciphering the Effect Mechanism of Dioscin on Rectal Cancer From Cell Metabolic Profiles Coupled With Chemometrics Analysis. Front. Pharmacol. 11:68. doi: 10.3389/fphar.2020.00068
Received: 26 July 2019; Accepted: 24 January 2020;
Published: 28 February 2020.
Edited by:
Lyndy Joy McGaw, University of Pretoria, South AfricaReviewed by:
Jianping Chen, Shenzhen Traditional Chinese Medicine Hospital, ChinaXijun Wang, Heilongjiang University of Chinese Medicine, China
Copyright © 2020 Wang, Yu and Li. This is an open-access article distributed under the terms of the Creative Commons Attribution License (CC BY). The use, distribution or reproduction in other forums is permitted, provided the original author(s) and the copyright owner(s) are credited and that the original publication in this journal is cited, in accordance with accepted academic practice. No use, distribution or reproduction is permitted which does not comply with these terms.
*Correspondence: Xin-Xin Wang, eGlueGlud2FuZzc5QHNpbmEuY24=
†These authors have contributed equally to this work