Corrigendum: Sensing Technology to Monitor Behavioral and Psychological Symptoms and to Assess Treatment Response in People With Dementia. A Systematic Review
- 1Department of Global Public Health and Primary Care, Centre for Elderly and Nursing Home Medicine, University of Bergen, Bergen, Norway
- 2Department of Nursing Home Medicine, Municipality of Bergen, Bergen, Norway
- 3Division of Geriatric Psychiatry, McLean Hospital, Belmont, MA, United States
- 4NKS Olaviken Gerontopsychiatric Hospital, Bergen, Norway
- 5Department of Psychiatry, Harvard Medical School, Boston, MA, United States
Background: The prevalence of dementia is expected to rapidly increase in the next decades, warranting innovative solutions improving diagnostics, monitoring and resource utilization to facilitate smart housing and living in the nursing home. This systematic review presents a synthesis of research on sensing technology to assess behavioral and psychological symptoms and to monitor treatment response in people with dementia.
Methods: The literature search included medical peer-reviewed English language publications indexed in Embase, Medline, Cochrane library and Web of Sciences, published up to the 5th of April 2019. Keywords included MESH terms and phrases synonymous with “dementia”, “sensor”, “patient”, “monitoring”, “behavior”, and “therapy”. Studies applying both cross sectional and prospective designs, either as randomized controlled trials, cohort studies, and case-control studies were included. The study was registered in PROSPERO 3rd of May 2019.
Results: A total of 1,337 potential publications were identified in the search, of which 34 were included in this review after the systematic exclusion process. Studies were classified according to the type of technology used, as (1) wearable sensors, (2) non-wearable motion sensor technologies, and (3) assistive technologies/smart home technologies. Half of the studies investigated how temporarily dense data on motion can be utilized as a proxy for behavior, indicating high validity of using motion data to monitor behavior such as sleep disturbances, agitation and wandering. Further, up to half of the studies represented proof of concept, acceptability and/or feasibility testing. Overall, the technology was regarded as non-intrusive and well accepted.
Conclusions: Targeted clinical application of specific technologies is poised to revolutionize precision care in dementia as these technologies may be used both by patients and caregivers, and at a systems level to provide safe and effective care. To highlight awareness of legal regulations, data risk assessment, and patient and public involvement, we propose a necessary framework for sustainable ethical innovation in healthcare technology. The success of this field will depend on interdisciplinary cooperation and the advance in sustainable ethic innovation.
Systematic Review Registration: PROSPERO, identifier CRD42019134313.
Introduction
The global health challenge of dementia is exceptional in size, cost and impact (Wortmann, 2012). The World Health Organization estimates that 47 million persons live with dementia worldwide, a number expected to reach 75 million by 2030 and more than triple by 2045 (World Health Organization, 2017). According to the Alzheimer’s Association, dementia-related costs range from $157 to $215 billion - higher than costs associated with cancer or cardiac disease — in the US alone, with roughly $42,000 to $56,000 spent per individual. These costs are driven to a significant extent by behavioral and psychological symptoms of dementia (BPSD) such as psychosis, apathy, hyperactivity, agitation, sleep disorders or depression (Ballard and Howard, 2006). This symptomatology may be caused or exaggerated by a range of conditions, such as hypoglycemia, pain and general discomfort, or they may arise secondary to the use of both psychotropic and non-psychotropic medications, which are known to precipitate a wide range of symptoms (Lyketsos et al., 2006). The prevalence of polypharmacy further adds to this clinical challenge (Gulla et al., 2016). Compounding this, no FDA approved pharmacologic treatments for BPSD exist and a wide range of psychotropic medications — including antipsychotics, mood stabilizers, antidepressants, and cholinesterase inhibitors — are regularly used to manage the symptoms, despite clear guidelines as to when and how to use them (Ballard and Corbett, 2010). This has led to vast variance in clinical practice around pharmacologic management of BPSD (Livingston et al., 2017). Polypharmacy and inappropriate prescribing can lead to significant adverse events, including increased fall risk, higher rates of inpatient care, loss of independence, and it increase the need for monitoring, which can significantly raise costs of dementia care, especially in nursing homes (Winblad et al., 2016).
Thus, there is an urgent need for tools that facilitate diagnoses that are more precise and a deeper understanding of patterns and triggers for BPSD (Kang et al., 2010). This includes tools that generate continuous data on behavior patterns, which may facilitate earlier detection of temporal events and guide more precise pharmacotherapy. Finally, there is a need for tools that can more closely monitor treatment response in dementia across care settings (Teipel et al., 2018).
A wide array of new technologies may provide solutions, especially those explicitly designed to support people with dementia and their formal and informal caregivers (Yang and Kels, 2017). The evidence around this has also been growing with research highlighting aspects of active and passive technology used in dementia (Pillai and Bonner-Jackson, 2015; Martinez-Alcala et al., 2016; Giggins et al., 2017; Brims and Oliver, 2018), the impact of safety equipment on wandering in dementia (Lin et al., 2014; Mangini and Wick, 2017), ethical considerations of surveillance technology in dementia (Sorell and Draper, 2012), or the need for real-world evidence-based solutions to conduct clinical trials (Teipel et al., 2018).
In this review paper, we present a synopsis of existing research studies in this space, including work on both commercially available as well as prototype technologies. This includes diagnostic technologies that utilize active and passive sensing in connection with smart housing, voice recognition and motion mapping (Teipel et al., 2018), and prognostic approaches that may inform clinicians about a range of potential responses, including alterations in circadian rhythm, changes in gait speed, falls, and variations in spatial location and reduction in resistance to care.
Finally, we discuss the potential pitfalls of this technology, specifically related to issues around ethics, privacy and security of data (Bantry-White, 2018; Chalghoumi et al., 2019) and the scalability of these technologies in terms to social living and activities.
Methods
This systematic review presents a synthesis of previous research on sensing technology to assess behavioral and psychological symptoms and to monitor treatment response in people with dementia.
Literature Search
We initially searched for peer-reviewed English language publications indexed in the following databases: Embase, Medline, Cochrane library and Web of Sciences, published up to the 5thof April 2019. Keywords included MESH terms and phrases synonymous with “dementia”, “sensor”, “patient”, “monitoring”, “behavior”, “therapy”. See full search history in the supplementary material. We assessed papers for eligibility using the PICO criteria (P: population, I: intervention, C: comparison and O: outcome), (see Table 1). We included studies applying both cross-sectional and prospective designs, including randomized controlled trials, cohort studies, and case-control studies. Reviews, opinion papers, protocols, and conference abstracts were excluded from the main search results.
After removal of duplicates, one researcher (BH) screened all the manuscripts on title and abstract level to select relevant studies based on the inclusion and exclusion criteria. Potentially relevant studies were assessed for eligibility by all coauthors by evaluating the inclusion and exclusion criteria on the full-text manuscripts. Reference lists of manuscripts and reviews were screened to identify additional relevant publications. The final selection of included publications was by consensus among all authors. The study was registered in PROSPERO 3rd of May 2019.
Results
The systematic search generated 1,337 potential publications from Embase (601), Cochrane (77) Medline (275), and Web of Science (384), of which 161 papers were identified as relevant for full-text evaluation (Figure 1). Of these, 127 papers were removed from the following results because they were either editorial review pieces or opinion articles, or because the studies involved use of technology but not for the primary goal of managing BPSD. Eighteen (53%) articles were published in Europe, 10 (29%) in the United States and 6 (18%) in Oceania.
Of the 34 studies selected, 23 focused on management of BPSD itself, while 11 studies that utilized sophisticated technological approaches for studying other factors. For example, one study (Whelan et al., 2018) assessed communication between caregivers and nursing home staff, while another utilized technology to assess ability to perform activities of daily living (ADLs) (Stucki et al., 2014). While these studies did not meet the original inclusion criteria, by consensus among the co-authors, we elected to include the studies since they reflect potentially meaningful applications of technology and may have implications for pharmacologic and non-pharmacologic management of BPSD.
For our final review, we assessed the full text from the 34 papers that were identified as relevant and divided studies into four broad categories, based on the type of technology used: (1) wearable technology, (2) non-wearable motion sensor technology, (3) assistive technology/smart home technology, and (4) other technologies not meeting criteria for the above three. We identified six papers that utilized more than one type of technology, and incorporated them into one of the above sections, based on the primary technology for each study.
Wearable Technologies
We identified seven studies that used wearable technologies — these included multiple sensor systems (two papers), ankle or wristbands (three papers), or a combination of both (two papers). We identified four prospective or retrospective cross-sectional studies along with two cohort studies and one case-control study. Study length ranged from 100 sec to 18 months. We noted that five studies utilized wearable technologies to primarily detect motion and two papers utilized wearable technologies that detected variables other than motion (body posture, stress). The details of the identified studies are summarized in Table 2.
Non-Wearable Motion Sensor Technologies
We identified 12 studies that utilized sensor-based motion detection approaches other than wearables. Only one of these was a randomized controlled trial, with one cohort study, four cross-sectional studies, two proof of concept studies, two case studies, and one longitudinal study. The sample sizes of the studies ranged vastly — from 1 to 265. This broad range reflects the heterogeneity of applications of motion sensor technologies for dementia. Study length ranged from two weeks to three years. Likewise, in the case of wearable technologies, we identified vast heterogeneity in study indications and purpose. Identified studies are summarized in Table 3.
Assistive/Smart Home Technologies
We identified 12 studies that utilized sensor rays placed in the living environment of study subjects. These were variously referred to as assistive or smart home technologies, since they required minimal active engagement by the patient or subject. The studies identified in this category included only one partial RCT — this study had 3 sites, but investigators were able to implement the RCT design at only one site. In addition, we identified three cross sectional/case-control studies, one cohort study, two case series and two open feasibility studies. We also identified three qualitative studies in this category. Study length ranged from 30 min interviews to 15 months and sample sizes ranged from four individuals to 65. Details of studies using these technologies are summarized in Table 4.
Other Technologies;
In addition, we identified three studies, each of which deployed a unique technological approach that could not be classified into one of the three categories above. One feasibility study (Khosla et al., 2017) used a human-like robot to assess social and emotional responses to nonhuman caregivers. Another study utilized a suite of apps administered via a tablet device as a nonpharmacologic intervention for agitation in dementia (Vahia et al., 2017). We identified one study that utilized a text analysis tool to detect variance and patterns of communication between patients, staff, and caregivers (Whelan et al., 2018). The details of the studies are summarized in Table 5.
Finally, during the entire review process, we became increasingly aware of the discrepancies and lack of consensus of the terminology used in this field. An overview of terminology and content are presented in Table 6.
Discussion
The goal for this review was to identify and summarize the extent to which literature on technologies (specifically sensors) have been used in the assessment and management of behavioral and psychological symptoms of dementia. As these technologies become widely available, this role is likely to expand (Collier et al., 2018). We identified several ways in which these technologies are being studied. This body of literature will play a crucial role in helping researchers, clinicians and municipalities, and industry partners to develop precision approaches to dementia care. We did note, however, that even though we in our original search aimed at clinical intervention studies with control groups, the majority of the studies found are preliminary with relatively small sample sizes and small durations. Some studies with much larger sample sizes were not intervention studies; rather they represented large surveys of participants around technology use. This dearth in intervention studies suggests that the grounds for innovation, validation, and clinical transference of technology in the management of behavioral symptoms are fertile.
Though we classified technologies into three broad categories, we identified several common underlying themes. Firstly, almost half of studies across the three categories represent ways in which temporarily dense data on motion can be processed and aggregated as proxy for behavior. Findings from these studies indicate high validity of using motion data to detect and track behavioral symptoms such as sleep disturbances, agitation, and wandering (Rowe et al., 2009; Bankole et al., 2012; Sacco et al., 2012; Yamakawa et al., 2012; Aloulou et al., 2013; Galambos et al., 2013; Stucki et al., 2014; Fleiner et al., 2016; Hattink et al., 2016; Jekel et al., 2016; Lazarou et al., 2016; Merilahti et al., 2016; Alvarez et al., 2018; Enshaeifar et al., 2018). Continuous motion monitoring of people with dementia using sensor technology provides informal caregivers and health care providers with the ability to more immediately and accurately diagnose and manage behavioral disturbances and can help to delay admission to long-term care or inpatient facilities. In a prodromal population, data from 8 of the studies suggest that motion data can also be useful in early detection of mild cognitive impairment and/or mild Alzheimer`s disease (Sacco et al., 2012; Hsu et al., 2014; Akl et al., 2015; Dodge et al., 2015; Gochoo et al., 2018; Zhou et al., 2018). While the majority of identified studies focused on the assessment of behaviors, we also identified 8 studies that developed intervention approaches based on sensor data or other feedback (Rowe et al., 2009; Aloulou et al., 2013; Martin et al., 2013; Hattink et al., 2016; Vahia et al., 2017; Khosla et al., 2017; Melander et al., 2018).
In addition, out of 34 studies, we found that 16 studies represented proof-of-concept, acceptability, and/or feasibility testing for technologies that are new and have not been used in the dementia population previously. These studies demonstrated some usability issues for smart home and assistive systems, e.g., technological malfunctions and general user-unfriendliness; however, the technology used was predominantly non-intrusive and well-accepted (Hattink et al., 2016; Olsson et al., 2018).
In terms of data privacy and security, we noted that the majority of our identified articles conclude their discussion by encouraging stakeholders to respect users’ privacy and autonomy. Several ask for legal frameworks and regulations to monitor the rapid development of this promising area (Yokokawa, 2012; Yang and Kels, 2017; Khan et al., 2018; Teipel et al., 2018). While we did not specifically identify clinical studies related to ethics, data privacy and security in our review, we present a synopsis of this topic, since the eventual acceptability of new technologies in dementia will be contingent on the development of transparency and trust around digital tools. This is highlighted in several opinion papers and review articles, which discuss ethical considerations in sensing technology for people with dementia or intellectual and developmental disabilities (Table 7).
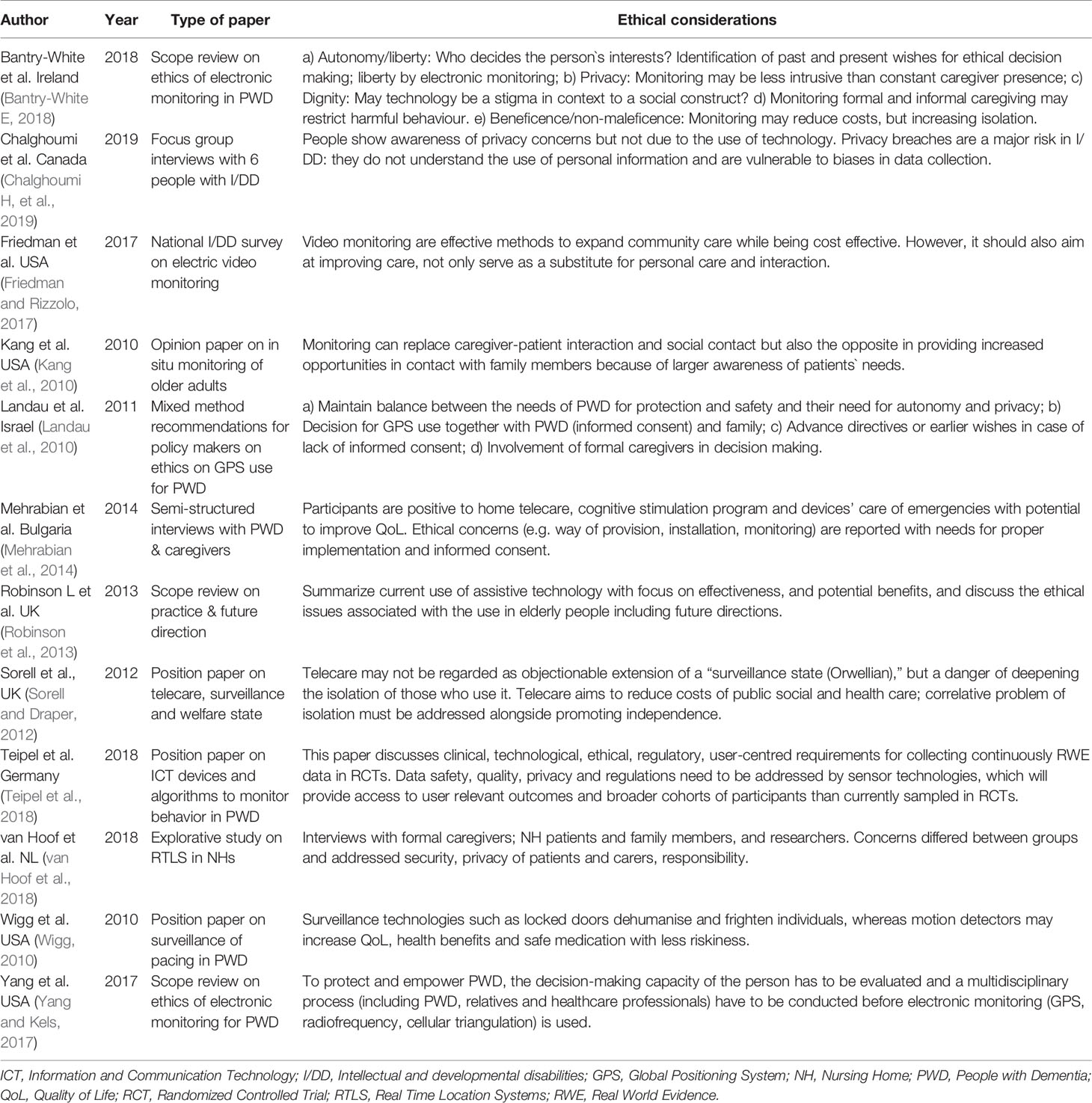
Table 7 Review and opinion articles on ethical considerations in sensing technology for people with dementia or intellectual and developmental disabilities.
Launched in May 2018, the General Data Protection Regulation (GDPR) is the novel European Union-wide law on data protection — a significant step towards more responsible protection of individuals (Crutzen et al., 2019). While it is recognized that participation in research is based on affirmative, unambiguous, voluntary, informed, and specific consent (Mendelson, 2018), people with advanced dementia or intellectual and developmental disabilities are not able to give informed consent or understand the consequences of data acquisition (Friedman and Rizzolo, 2017; Chalghoumi et al., 2019; Timmers et al., 2019). Article 6 of the GDPR addresses this issue by including provisions that protecting persons with dementia and their relatives from being coerced into providing consent without awareness of how their data will be used (Cool, 2019; Crutzen et al., 2019). Despite this regulation, local legislation differs between European countries (de Lange et al., 2019). In Norway, for, e.g., a family member or legal advocate may provide or refuse consent based on their determination around whether the person with dementia would agree or decline to participate in a given study (Husebo et al., 2019). In Germany, the inclusion of people with dementia is limited for only those who may directly benefit from research results. To further strengthen privacy protections, Article 35 of the GDPR requires the Data Protection Impact Assessment (DPIA) (Figure 2) (Donnelly and McDonagh, 2019), which mandates that only the most relevant personal data is collected (data minimization), and limits data access to those who are authorized or given permission by the individual (Yang and Kels, 2017). Overall, in this review, we did not specifically include search keywords relating to ethics in sensor technology but we recognized an engaged discussion in a considerable number of position papers and reviews around ethical considerations and especially, the need for data protection, proper transfer and storage (Holm and Ploug, 2013; Ploug and Holm, 2013).
Agencies that provide funding for research increasingly require patient and public involvement (PPI) in design, implementation, and dissemination of health research (Figure 2) (Melander et al., 2017; Melander et al., 2018). The goal of PPI is to ensure user-centered design so that persons who may benefit from it have an opportunity, especially in the early stages of their disease (Landau and Werner, 2012; Bantry-White, 2018) to understand the purpose of the technology (e.g. GPS) and to express values, wishes, and concerns to formal and informal caregivers, We noted that this principle was incorporated into at least three studies that we reviewed (Landau and Werner, 2012; Lariviere, 2017; Mangini and Wick, 2017; Bantry-White, 2018). This approach is also likely to optimize technology engagement in dementia (Nijhof et al., 2013; Mehrabian et al., 2014). A related principle, Responsible Research and Innovation (RRI) (Figure 2), is defined as a transparent, interactive process by which societal actors and innovators become mutually responsive to each other (von Schomberg, 2013). They are encouraged to assume a critical perspective when evaluating the innovation and marketability of products (Holthe et al., 2018; Lehoux and Grimard, 2018). This approach may serve to promote awareness of technologies and related issues across both groups of stakeholders (van Haeften-van Dijk et al., 2015; Wu et al., 2017; Rostill et al., 2018).
Limitations
Our findings and recommendations must be interpreted in the context of some limitations. During the process, we recognized that the MESH terms and phrases synonymous with “dementia”, “sensor”, “patient”, “monitoring”, and “behavior”, and “ therapy” probably did not cover the whole range of interesting topics. For instance, items such as ethics, activities of daily living (ADL), and communication, may increase the understanding for and connection to the clinical aspects of this quickly developing area. Because of the vast heterogeneity of the literature, including terminology and definitions of sensing technology, a meta-analysis that may facilitate aggregated recommendations was not feasible. We also noted that the majority of the studies were open-label early-stage studies. Replication of these findings in larger trials will be required before these findings can become the standard of care. Our search algorithm also has potential limitations. We restricted our search to the past decade, since we anticipate that future sensor-based care models will be built on contemporary technology. We determined that tools that are more than a decade old are unlikely to have relevance in the future.
Conclusion
Overall, our systematic review demonstrates that sensor technologies have a broad range of potential applications in dementia care, ranging from early detection of cognitive impairment to aid in the management of behavioral and psychological symptoms in late stage dementia. Targeted clinical application of specific technologies is poised to revolutionize precision care in dementia as these technologies may be used by patients themselves, caregivers, or even applied at a systems level (e.g., nursing homes) to provide more safe and effective care. As sensor technology matures in its ability to guide care in BPSD, it may generate novel ways to capture early symptomatology (e.g., social isolation), improve specificity for cognitive testing in-situ and facilitate cost effective research approaches. A small but rapidly growing body of evidence around sensors in dementia care is paving the early way for the field, bringing into focus both the potential and pitfalls of this approach. Next step in this field may be to investigate the validity of use not only for care purposes, but also for prognostics as well as acceptability, feasibility, and responsiveness in clinical trials. The eventual success of this field will depend on inter-disciplinary models of research, development by industry partners, and sustainable ethic innovation in healthcare technology and smart housing.
Data Availability Statement
All datasets generated for this study are included in the article/supplementary material.
Author Contributions
BH and IV developed the study idea, designed the study protocol for the systematic search and BH applied for funding. BH screened all the manuscripts on title and abstract level to select relevant studies. All the co-authors assessed potentially relevant studies on full-text manuscripts for eligibility using inclusion and exclusion criteria. HH, LB, PO, and AR drafted the first version of the result evaluation with supervision from IV and BH. Contribution to the subsequent drafts were provided by BH, HH, LB, PO, AR, and IV. All authors approved the final version of the manuscript.
Funding
This work is supported by the University of Bergen, The Norwegian Research Council and, in part, by the Technology and Aging Lab at McLean Hospital.
Conflict of Interest
The authors declare that the research was conducted in the absence of any commercial or financial relationships that could be construed as a potential conflict of interest.
Acknowledgments
The authors would like to thank medical librarian Regina Küfner Lein at the University of Bergen, Norway. BH would like to thank the Norwegian Government and the GC Rieber Foundation for supporting her time for this work at the Centre for Elderly and Nursing Home Medicine, University of Bergen. LB would like to thank the Research Council of Norway for the support during her postdoctoral period.
References
Akl, A., Taati, B., Mihailidis, A. (2015). Autonomous unobtrusive detection of mild cognitive impairment in older adults. IEEE Trans. Biomed. Eng 62 (5), 1383–1394. doi: 10.1109/TBME.2015.2389149
Aloulou, H., Mokhtari, M., Tiberghien, T., Biswas, J., Phua, C., Kenneth Lin, J. H., et al. (2013). Deployment of assistive living technology in a nursing home environment: methods and lessons learned. BMC Med. Info. Decis. Making 13, 42. doi: 10.1186/1472-6947-13-42
Alvarez, F., Popa, M., Solachidis, V., Hernandez-Penaloza, G., Belmonte-Hernandez, A., Asteriadis, S., et al. (2018). Behavior analysis through Multimodal Sensing for Care of Parkinson’s and Alzheimer’s Patients. IEEE Multimedia 25 (1), 14–25. doi: 10.1109/MMUL.2018.011921232
Asghar, I., Cang, S., Yu, H. (2018). Impact evaluation of assistive technology support for the people with dementia. Assist. Technol. 31 (4), 180–192. doi: 10.1080/10400435.2017.1411405
Ballard, C., Corbett, A. (2010). Management of Neuropsychiatric Symptoms in People with Dementia. CNS Drugs 24 (9), 729–739. doi: 10.2165/11319240-000000000-00000
Ballard, C., Howard, R. (2006). Neuroleptic drugs in dementia: benefits and harm. Nat. Rev. Neurosci. 7 (6), 492–500. doi: 10.1038/nrn1926
Bankole, A., Anderson, M., Smith-Jackson, T., Knight, A., Oh, K., Brantley, J., et al. (2012). Validation of noninvasive body sensor network technology in the detection of agitation in dementia. Am. J. Alzheimers Dis. Other Demen 27 (5), 346–354. doi: 10.1177/1533317512452036
Bantry-White, E. (2018). Supporting ethical use of electronic monitoring for people living with dementia: social work’s role in assessment, decision-making, and review. J. Gerontol. Soc Work 61 (3), 261–279. doi: 10.1080/01634372.2018.1433738
Brims, L., Oliver, K. (2018). Effectiveness of assistive technology in improving the safety of people with dementia: a systematic review and meta-analysis. Aging Ment. Health 23 (8), 942–951. doi: 10.1080/13607863.2018.1455805
Chalghoumi, H., Cobigo, V., Dignard, C., Gauthier-Beaupré, A., Jutai, J. W., Lachapelle, Y., et al. (2019). Information Privacy for Technology Users With Intellectual and Developmental Disabilities: Why Does It Matter? Ethics Behav. 29 (3), 201–217. doi: 10.1080/10508422.2017.1393340
Collier, S., Monette, P., Hobbs, K., Tabasky, E., Forester, B. P., Vahia, I. V. (2018). Mapping movement: applying motion measurement technologies to the psychiatric care of older adults. Curr. Psychiatry Rep. 20 (8), 64. doi: 10.1007/s11920-018-0921-z
Collins, M. E. (2018). Occupational Therapists’ Experience with assistive technology in provision of service to clients with Alzheimer’s Disease and Related Dementias. Phys. Occup. Ther. Geriatr. 36 (2-3), 179–188. doi: 10.1080/02703181.2018.1458770
Cool, A. (2019). Impossible, unknowable, accountable: dramas and dilemmas of data law. Soc Stud. Sci. 49 (4), 503–530. doi: 10.1177/0306312719846557
Crutzen, R., Ygram Peters, G. J., Mondschein, C. (2019). Why and how we should care about the General Data Protection Regulation. Psychol. Health 34 (11), 1347–1357. doi: 10.1080/08870446.2019.1606222
de Lange, D. W., Guidet, B., Andersen, F. H., Artigas, A., Bertolini, G., Moreno, R., et al. (2019). Huge variation in obtaining ethical permission for a non-interventional observational study in Europe. BMC Med. Ethics 20 (1), 39–39. doi: 10.1186/s12910-019-0373-y
Dodge, H. H., Zhu, J., Mattek, N. C., Austin, D., Kornfeld, J., Kaye, J. A. (2015). Use of high-frequency in-home monitoring data may reduce sample sizes needed in clinical trials. PloS One 10 (9), e0138095. doi: 10.1371/journal.pone.0138095
Donnelly, M., McDonagh, M. (2019). Health research, consent and the GDPR exemption. Eur. J. Health Law 26 (2), 97–119. doi: 10.1163/15718093-12262427
Enshaeifar, S., Zoha, A., Markides, A., Skillman, S., Acton, S. T., Elsaleh, T., et al. (2018). Health management and pattern analysis of daily living activities of people with dementia using in-home sensors and machine learning techniques. PloS One 13 (5), e0195605. doi: 10.1371/journal.pone.0195605
Fleiner, T., Haussermann, P., Mellone, S., Zijlstra, W. (2016). Sensor-based assessment of mobility-related behavior in dementia: feasibility and relevance in a hospital context. Int. Psychogeriatr 28 (10), 1687–1694. doi: 10.1017/S1041610216001034
Friedman, C., Rizzolo, M. C. (2017). Electronic video monitoring in medicaid home and community-based services waivers for people with intellectual and developmental disabilities. J. Policy Pract. Intellect. Dis. 14 (4), 279–284. doi: 10.1111/jppi.12222
Galambos, C., Skubic, M., Wang, S., Rantz, M. (2013). Management of dementia and depression utilizing in- home passive sensor data. Gerontechnol 11 (3), 457–468. doi: 10.4017/gt.2013.11.3.004.00
Giggins, O. M., Clay, I., Walsh, L. (2017). Physical activity monitoring in patients with neurological disorders: A review of novel body-worn devices. Digit Biomarkers 1 (1), 14–42. doi: 10.1159/000477384
Gochoo, M., Tan, T. H., Velusamy, V., Liu, S. H., Bayanduuren, D., Huang, S. C. (2018). Device-free non-privacy invasive classification of elderly travel patterns in a smart house using PIR Sensors and DCNN. IEEE Sensors J. 18 (1), 390–400. doi: 10.1109/JSEN.2017.2771287
Gulla, C., Selbaek, G., Flo, E., Kjome, R., Kirkevold, O., Husebo, B. S. (2016). Multi-psychotropic drug prescription and the association to neuropsychiatric symptoms in three Norwegian nursing home cohorts between 2004 and 2011. BMC Geriatr. 16, 115. doi: 10.1186/s12877-016-0287-1
Hattink, B. J., Meiland, F. J., Overmars-Marx, T., de Boer, M., Ebben, P. W., van Blanken, M., et al. (2016). The electronic, personalizable Rosetta system for dementia care: exploring the user-friendliness, usefulness and impact. Disabil. Rehabil. Assist. Technol. 11 (1), 61–71. doi: 10.3109/17483107.2014.932022
Holm, S., Ploug, T. (2013). Nudgingand informed consent revisited: why “nudging” fails in the clinical context. Am. J. Bioeth 13 (6), 29–31. doi: 10.1080/15265161.2013.781713
Holthe, T., Casagrande, F. D., Halvorsrud, L., Lund, A. (2018). The assisted living project: a process evaluation of implementation of sensor technology in community assisted living. A feasibility study. Disabil. Rehabil. Assist. Technol. 15 (1), 29–36. doi: 10.1080/17483107.2018.1513572
Hsu, Y. L., Chung, P. C., Wang, W. H., Pai, M. C., Wang, C. Y., Lin, C. W., et al. (2014). Gait and balance analysis for patients with Alzheimer’s disease using an inertial-sensor-based wearable instrument. IEEE J. Biomed. Health Inform. 18 (6), 1822–1830. doi: 10.1109/JBHI.2014.2325413
Husebo, B. S., Ballard, C., Aarsland, D., Selbaek, G., Slettebo, D. D., Gulla, C., et al. (2019). The effect of a multicomponent intervention on quality of life in residents of nursing homes: a randomized controlled trial (COSMOS). J. Am. Med. Dir. Assoc. 20 (3), 330–339. doi: 10.1016/j.jamda.2018.11.006
Jansen, C. P., Diegelmann, M., Schnabel, E. L., Wahl, H. W., Hauer, K. (2017). Life-space and movement behavior in nursing home residents: results of a new sensor-based assessment and associated factors. BMC Geriatr. 17, 36. doi: 10.1186/s12877-017-0430-7
Jekel, K., Damian, M., Storf, H., Hausner, L., Frolich, L. (2016). Development of a proxy-free objective assessment tool of instrumental activities of daily living in mild cognitive impairment using smart home technologies. J. Alzheimers. Dis. 52 (2), 509–517. doi: 10.3233/JAD-151054
Kang, H. G., Mahoney, D. F., Hoenig, H., Hirth, V. A., Bonato, P., Hajjar, I. (2010). Innovative technology working group on advanced approaches to physiologic monitoring for the, A. (2010). In situ monitoring of health in older adults: technologies and issues. J. Am. Geriatr. Soc. 58 (8), 1579–1586. doi: 10.1111/j.1532-5415.2010.02959.x
Khan, S. S., Ye, B., Taati, B., Mihailidis, A. (2018). Detecting agitation and aggression in people with dementia using sensors-A systematic review. Alzheimers Dement 14 (6), 824–832. doi: 10.1016/j.jalz.2018.02.004
Khosla, R., Nguyen, K., Chu, M.-T. (2017). Human robot engagement and acceptability in residential aged care. Int. J. Human–Computer Interact. 33 (6), 510–522. doi: 10.1080/10447318.2016.1275435
Kikhia, B., Stavropoulos, T. G., Andreadis, S., Karvonen, N., Kompatsiaris, I., Savenstedt, S., et al. (2016). Utilizing a wristband sensor to measure the stress level for people with dementia. Sensors 16 (12), 24. doi: 10.3390/s16121989
Landau, R., Auslander, G. K., Werner, S., Shoval, N., Heinik, J. (2010). Families’ and professional caregivers’ views of using advanced technology to track people with dementia. Qual. Health Res. 20 (3), 409–419. doi: 10.1177/1049732309359171
Landau, R., Werner, S. (2012). Ethical aspects of using GPS for tracking people with dementia: recommendations for practice. Int. Psychogeriatr 24 (3), 358–366. doi: 10.1017/S1041610211001888
Lariviere, M. J. (2017). Exaine current technology – enabled care practices for people with dementia in the UK: findings from accommodate. A collaborative community-based ethnography of people wliving with dementia using assistive technology and telecare at home. Alzheimer Dement 13 (7), P157. doi: 10.1016/j.jalz.2017.06.2595
Lazarou, I., Karakostas, A., Stavropoulos, T. G., Tsompanidis, T., Meditskos, G., Kompatsiaris, I., et al. (2016). A Novel and Intelligent Home Monitoring System for Care Support of Elders with Cognitive Impairment. J. Alzheimers Dis. 54 (4), 1561–1591. doi: 10.3233/JAD-160348
Lehoux, P., Grimard, D. (2018). When robots care: Public deliberations on how technology and humans may support independent living for older adults. Soc Sci.Med. 211, 330–337. doi: 10.1016/j.socscimed.2018.06.038
Lin, Q., Zhang, D., Chen, L., Ni, H., Zhou, X. (2014). Managing Elders’ Wandering Behavior Using Sensors-based Solutions: A Survey. Int. J. Gerontol 8 (2), 49–55. doi: 10.1016/j.ijge.2013.08.007
Livingston, G., Sommerlad, A., Orgeta, V., Costafreda, S. G., Huntley, J., Ames, D., et al. (2017). Dementia prevention, intervention, and care. Lancet 390 (10113), 2673–2734. doi: 10.1016/S0140-6736(17)31363-6
Lyketsos, C. G., Colenda, C. C., Beck, C., Blank, K., Doraiswamy, M. P., Kalunian, D. A., et al. (2006). Position statement of the American Association for Geriatric Psychiatry regarding principles of care for patients with dementia resulting from Alzheimer disease. Am. J. Geriatr. Psychiatry 14 (7), 561–572. doi: 10.1097/01.JGP.0000221334.65330.55
Mangini, L., Wick, J. Y. (2017). Wandering: Unearthing New Tracking Devices. Consult. Pharm. 32 (6), 324–331. doi: 10.4140/TCP.n.2017.324
Martin, S., Augusto, J. C., McCullagh, P., Carswell, W., Zheng, H., Wang, H., et al. (2013). Participatory research to design a novel telehealth system to support the night-time needs of people with dementia: NOCTURNAL. Int. J. Environ. Res. Public Health 10 (12), 6764–6782. doi: 10.3390/ijerph10126764
Martinez-Alcala, C. I., Pliego-Pastrana, P., Rosales-Lagarde, A., Lopez-Noguerola, J. S., Molina-Trinidad, E. M. (2016). Information and Communication Technologies in the Care of the Elderly: Systematic Review of Applications Aimed at Patients With Dementia and Caregivers. JMIR Rehabil. Assist. Technol. 3 (1), e6. doi: 10.2196/rehab.5226
Mehrabian, S., Extra, J., Wu, Y.-H., Pino, M., Traykov, L., Rigaud, A.-S. (2014). The perceptions of cognitively impaired patients and their caregivers of a home telecare system. Med. Devices 8, 21–29. doi: 10.2147/MDER.S70520
Meiland, F. J., Hattink, B. J., Overmars-Marx, T., de Boer, M. E., Jedlitschka, A., Ebben, P. W., et al. (2014). Participation of end users in the design of assistive technology for people with mild to severe cognitive problems; the European Rosetta project. Int. Psychogeriatr. 26 (5), 769–779. doi: 10.1017/S1041610214000088
Melander, C., Martinsson, J., Gustafsson, S. (2017). Measuring Electrodermal Activity to Improve the Identification of Agitation in Individuals with Dementia. Dement. Geriatr. Cogn. Dis. Extra 7 (3), 430–439. doi: 10.1159/000484890
Melander, C. A., Kikhia, B., Olsson, M., Walivaara, B. M., Savenstedt, S. (2018). The Impact of Using Measurements of Electrodermal Activity in the Assessment of Problematic Behaviour in Dementia. Dement. Geriatr. Cogn. Dis. Extra 8 (3), 333–347. doi: 10.1159/000493339
Mendelson, D. (2018). The European Union General Data Protection Regulation (EU 2016/679) and the Australian My Health Record Scheme - A Comparative Study of Consent to Data Processing Provisions. J. Law Med. 26 (1), 23–38. doi: 10.2139/ssrn.3225047
Merilahti, J., Viramo, P., Korhonen, I. (2016). Wearable Monitoring of Physical Functioning and Disability Changes, Circadian Rhythms and Sleep Patterns in Nursing Home Residents. IEEE J. Biomed. Health Inform. 20 (3), 856–864. doi: 10.1109/JBHI.2015.2420680
Nijhof, N., van Gemert-Pijnen, L. J., Woolrych, R., Sixsmith, A. (2013). An evaluation of preventive sensor technology for dementia care. J. Telemed. Telecare 19 (2), 95–100. doi: 10.1258/jtt.2012.120605
Nishikata, S., Yamakawa, M., Shigenobu, K., Suto, S., Makimoto, K. (2013). Degree of ambulation and factors associated with the median distance moved per day in Alzheimer’s disease patients. Int. J. Nurs. Pract. 19 Suppl 3, 56–63. doi: 10.1111/ijn.12174
Olsson, A., Persson, A. C., Bartfai, A., Boman, I. L. (2018). Sensor technology more than a support. Scand. J. Occup. Ther. 25 (2), 79–87. doi: 10.1080/11038128.2017.1293155
Pillai, J. A., Bonner-Jackson, A. (2015). Review of information and communication technology devices for monitoring functional and cognitive decline in Alzheimer’s disease clinical trials. J. Health Eng 6 (1), 71–83. doi: 10.1260/2040-2295.6.1.71
Ploug, T., Holm, S. (2013). Informed consent and routinisation. J. Med. Ethics 39 (4), 214. http://jme.bmj.com/content/39/4/214.abstract. doi: 10.1136/medethics-2012-101056
Robinson, L., Gibson, G., Kingston, A. (2013). Assistive technologies in caring for the oldest old: A review of current practice and future directions. Aging Health 9 (4), 365–375. doi: 10.2217/ahe.13.35
Rostill, H., Nilforooshan, R., Morgan, A., Barnaghi, P., Ream, E., Chrysanthaki, T. (2018). Technology integrated health management for dementia. Br. J. Community Nurs. 23 (10), 502–508. doi: 10.12968/bjcn.2018.23.10.502
Rowe, M. A., Kelly, A., Horne, C., Lane, S., Campbell, J., Lehman, B., et al. (2009). Reducing dangerous nighttime events in persons with dementia by using a nighttime monitoring system. Alzheimers Dement. 5 (5), 419–426. doi: 10.1016/j.jalz.2008.08.005
Sacco, G., Joumier, V., Darmon, N., Dechamps, A., Derreumaux, A., Lee, J. H., et al. (2012). Detection of activities of daily living impairment in Alzheimer’s disease and mild cognitive impairment using information and communication technology. Clin. Interv. Aging 7, 539–549. doi: 10.2147/CIA.S36297
Sorell, T., Draper, H. (2012). Telecare, surveillance, and the welfare state. Am. J. Bioeth 12 (9), 36–44. doi: 10.1080/15265161.2012.699137
Stucki, R. A., Urwyler, P., Rampa, L., Muri, R., Mosimann, U. P., Nef, T. (2014). A web-based non-intrusive ambient system to measure and classify activities of daily living. J. Med. Internet. Res. 16 (7), e175. doi: 10.2196/jmir.3465
Teipel, S., Konig, A., Hoey, J., Kaye, J., Kruger, F., Robillard, J. M., et al. (2018). Use of nonintrusive sensor-based information and communication technology for real-world evidence for clinical trials in dementia. Alzheimers Dement. 14 (9), 1216–1231. doi: 10.1016/j.jalz.2018.05.003
Timmers, M., Van Veen, E. B., Maas, A. I. R., Kompanje, E. J. O. (2019). Will the Eu Data Protection Regulation 2016/679 Inhibit Critical Care Research? Med. Law Rev. 27 (1), 59–78. doi: 10.1093/medlaw/fwy023
Vahia, I. V., Kamat, R., Vang, C., Posada, C., Ross, L., Oreck, S., et al. (2017). Use of tablet devices in the management of agitation among inpatients with dementia: an open-label study. Am. J. Geriatr. Psychiatry 25 (8) 860–864. doi: 10.1016/j.jagp.2016.07.011
van Haeften-van Dijk, A. M., Meiland, F. J., van Mierlo, L. D., Droes, R. M. (2015). Transforming nursing home-based day care for people with dementia into socially integrated community day care: process analysis of the transition of six day care centres. Int. J. Nurs. Stud. 52 (8), 1310–1322. doi: 10.1016/j.ijnurstu.2015.04.009
van Hoof, J., Verboor, J., Weernink, C. E. O. (2018). Real-Time Location Systems for Asset Management in Nursing Homes: An Explorative Study of Ethical Aspects. Information 9 (4), 80. doi: 10.3390/info9040080
von Schomberg, R. (2013). “A vision of responsible research and innovation,” in Responsible Innovation: Managing the Responsible Emergence of Science and Innovation in Society. Eds. Owen, R., Bessant, J., Heintz, M. (London: John Wiley & Sons), 51–74. doi: 10.1002/9781118551424.ch3
Whelan, B. M., Angus, D., Wiles, J., Chenery, H. J., Conway, E. R., Copland, D. A., et al. (2018). Toward the Development of SMART Communication Technology: Automating the Analysis of Communicative Trouble and Repair in Dementia. Innov. Aging 2 (3), igy034. doi: 10.1093/geroni/igy034
Wigg, J. M. (2010). Liberating the wanderers: using technology to unlock doors for those living with dementia. Sociol Health Illness 32 (2), 288–303. doi: 10.1111/j.1467-9566.2009.01221.x
Winblad, B., Amouyel, P., Andrieu, S., Ballard, C., Brayne, C., Brodaty, H., et al. (2016). Defeating Alzheimer’s disease and other dementias: a priority for European science and society. Lancet Neurol. 15 (5), 455–532. doi: 10.1016/S1474-4422(16)00062-4
World Health Organization (2017). 10 facts on dementia. The World Health Organization. Available from: https://www.who.int/features/factfiles/dementia/en/
Wortmann, M. (2012). Dementia: a global health priority - highlights from an ADI and World Health Organization report. Alzheimers Res. Ther. 4 (5), 40. doi: 10.1186/alzrt143
Wu, Y. T., Beiser, A. S., Breteler, M. M. B., Fratiglioni, L., Helmer, C., Hendrie, H. C., et al. (2017). The changing prevalence and incidence of dementia over time - current evidence. Nat. Rev. Neurol. 13 (6), 327–339. doi: 10.1038/nrneurol.2017.63
Yamakawa, M., Suto, S., Shigenobu, K., Kunimoto, K., Makimoto, K. (2012). Comparing dementia patients’ nighttime objective movement indicators with staff observations. Psychogeriatr 12 (1), 18–26. doi: 10.1111/j.1479-8301.2011.00380.x
Yang, Y. T., Kels, C. G. (2017). Ethical Considerations in Electronic Monitoring of the Cognitively Impaired. J. Am. Board. Fam. Med. 30 (2), 258–263. doi: 10.3122/jabfm.2017.02.160219
Yokokawa, K. (2012). Usefulness of video for observing lifestyle impairments in dementia patients. Psychoger 12 (2), 137–141. doi: 10.1111/j.1479-8301.2012.00428.x
Zhou, H., Sabbagh, M., Wyman, R., Liebsack, C., Kunik, M. E., Najafi, B. (2017). Instrumented trail-making task to differentiate persons with no cognitive impairment, amnestic mild cognitive impairment, and alzheimer disease: a proof of concept study. Gerontol 63 (2), 189–200. doi: 10.1159/000452309
Keywords: dementia, sensoring, monitoring, behavior, therapy
Citation: Husebo BS, Heintz HL, Berge LI, Owoyemi P, Rahman AT and Vahia IV (2020) Sensing Technology to Monitor Behavioral and Psychological Symptoms and to Assess Treatment Response in People With Dementia. A Systematic Review. Front. Pharmacol. 10:1699. doi: 10.3389/fphar.2019.01699
Received: 02 July 2019; Accepted: 31 December 2019;
Published: 04 February 2020.
Edited by:
Bjorn Johansson, Karolinska Institutet (KI), SwedenReviewed by:
Dione Kobayashi, Independent Researcher, Cambridge, United StatesMing-Chyi Pai, National Cheng Kung University, Taiwan
Copyright © 2020 Husebo, Heintz, Berge, Owoyemi, Rahman and Vahia. This is an open-access article distributed under the terms of the Creative Commons Attribution License (CC BY). The use, distribution or reproduction in other forums is permitted, provided the original author(s) and the copyright owner(s) are credited and that the original publication in this journal is cited, in accordance with accepted academic practice. No use, distribution or reproduction is permitted which does not comply with these terms.
*Correspondence: Bettina S. Husebo, Bettina.Husebo@uib.no