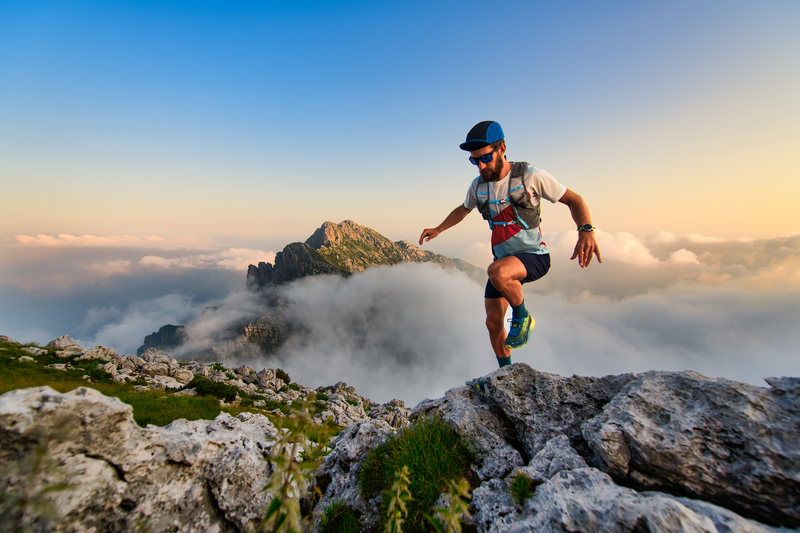
94% of researchers rate our articles as excellent or good
Learn more about the work of our research integrity team to safeguard the quality of each article we publish.
Find out more
ORIGINAL RESEARCH article
Front. Pharmacol. , 24 July 2018
Sec. Ethnopharmacology
Volume 9 - 2018 | https://doi.org/10.3389/fphar.2018.00801
Background: Polypharmacology is emerging as the next paradigm in drug discovery. However, considerable challenges still exist for polypharmacology modeling. In this study, we developed a rational design to identify highly potential targets (HPTs) for polypharmacological drugs, such as berberine.
Methods and Results: All the proven co-crystal structures locate berberine in the active cavities of a redundancy of aromatic, aliphatic, and acidic residues. The side chains from residues provide hydrophobic and electronic interactions to aid in neutralization for the positive charge of berberine. Accordingly, we generated multi-target binding motifs (MBM) for berberine, and established a new mathematical model to identify HPTs based on MBM. Remarkably, the berberine MBM was embodied in 13 HPTs, including beta-secretase 1 (BACE1) and amyloid-β1-42 (Aβ1-42). Further study indicated that berberine acted as a high-affinity BACE1 inhibitor and prevented Aβ1-42 aggregation to delay the pathological process of Alzheimer’s disease.
Conclusion: Here, we proposed a MBM-based drug-target space model to analyze the underlying mechanism of multi-target drugs against polypharmacological profiles, and demonstrated the role of berberine in Alzheimer’s disease. This approach can be useful in derivation of rules, which will illuminate our understanding of drug action in diseases.
Recently, it has been appreciated that single drug acts on multiple rather than single targets, coined as polypharmacology, which opens a new avenue for drug discovery and development (Anighoro et al., 2014; Rastelli and Pinzi, 2015). Current studies in this emerging field focus on two major aspects: (i) polypharmacology across multiple disease-relevant targets can improve therapeutic efficacy (Monteleone et al., 2017); (ii) unintended drug-target interactions can lead to side effects (Peters, 2013). The versatile and promising scaffolds targeting protein–protein interactions offers opportunities for therapeutic intervention for the treatment of human diseases (Liu L.J. et al., 2017; Wang K. et al., 2017). Recent progress in protein labeling technology has enabled the development of probes for the determination of various biological molecules (Kang et al., 2017; Liu J.B. et al., 2017; Vellaisamy et al., 2018). Despite advances in these areas, considerable challenges still exist for polypharmacology modeling. The underlying assumption of the rational design will result from a detailed analysis of the nature of drug-target interactions for the proven effective and safe polypharmacological drugs, such as berberine (Wang W. et al., 2017).
Berberine is a natural isoquinoline alkaloid presented in various medicinal plants, including Berberis vulgaris (Barberry), Coptis chinensis (Coptis), and Hydrastis canadensis (Goldenseal) (Samanani et al., 2005). In traditional medicine, berberine has been widely used to treat diarrhea for a long history (Taylor and Greenough, 1989). Recently, berberine was reported to exhibit favorable therapeutic effects on infection (Chu et al., 2016), cancer (La et al., 2017), diabetes (Gong et al., 2017), obesity (Hvistendahl, 2012), hyperlipidemia (Kong et al., 2004), cardiovascular disease (Tan et al., 2016) and neurodegenerative disorders (Ahmed et al., 2015). The clinical efficacy of berberine is mediated by interacting with a mixture of biological pathways, which are involved in metabolism (Moghaddam et al., 2014; Zhang et al., 2014), transmission of signals (Chen et al., 2017; Spatuzza et al., 2014; Yue et al., 2017) and regulation of gene expression (Bhadra and Kumar, 2011). Increasing evidences indicate that berberine can act on a diverse range of molecular targets by binding to the active cavities that are to have certain structural and physiochemical properties, such as QacR (Schumacher et al., 2001), BmrR (Newberry et al., 2008), RamR (Yamasaki et al., 2013), phospholipase A2 (PLA2) (Chandra et al., 2012) and double helix DNA d(CGTACG)2 (Ferraroni et al., 2011). According to the co-crystal structures of these targets in complex with berberine, berberine binds highly site-specifically by interacting with residues flanking a hydrophobic groove in the pocket. Here, we analyzed and generated a MBM model for berberine to identify HPTs that tend to be nodes positioned in the ‘Goldilocks’ region of biological networks. These findings will provide new insights into the underlying mechanisms of berberine against polypharmacological profiles.
All computational screening methods were performed using the Discovery Studio 2017R2 (DS; BIOVIA-Dassault Systèmes) running on Windows 8.1 operating system in a machine with an Intel® Xeon® E5-2699 v3 2.30 GHz octadeca core processor.
The features of a pharmacophore model reflect the drug-target interaction mode. By fitting berberine against a panel of pharmacophore models, the potential targets for berberine were picked out by employing the Ligand Profiler protocol of DS, which is equipped with two available pharmacophore databases, i.e., PharmaDB and HypoDB. Literature retrieval was simultaneously carried out to refine and supplement the profiling results.
The three-dimensional crystal structures of the proven targets for berberine were retrieved from the Protein Data Bank1, including QacR (PDB ID: 1JUM), BmrR (PDB ID: 3D6Y), RamR (PDB ID: 3VW2), PLA2 (PDB ID: 2QVD), and double helix DNA d(CGTACG)2 (PDB ID: 3NP6). The structures were prepared and optimized using the Prepare Protein and Minimization protocols of DS (Chu et al., 2018). After optimization, the binding-site spheres were subsequently defined around the location of berberine. Based on the steric and electronic features in the binding sites of multiple targets with berberine, the MBM was generated using the Receptor-Ligand Pharmacophore Generation module in DS.
The MBM-based screening starts with the analysis of potential targets by the DS package. The optimized berberine was docked into the refined potential targets using LigandFit. The LigandScore can define the binding sites, generate ligand conformations, dock each conformation, save the top docked structures, and apply scoring function to each docked structure for the best binding mode (Chu et al., 2016). The pharmacophore models were subsequently generated using the Receptor-Ligand Pharmacophore Generation module. The binding potential (BP) was calculated using the following equation: BP = nα × RMSDβ, where BP is also equal to the one-KD-minus-κ power of ten, n is the number of pharmacophore features matched with MBM, RMSD is the average distance in three-dimensional structure between pharmacophore and MBM. The values of α, β, and κ were determined as 2.599, -5.234, and -5.905, respectively, which was simulated from a series of proven KD values of berberine. The fit value is a combined measure of the similarity between pharmacophore and MBM, and computed by the following equation.
Here, BP0 was taken as the minimum BP of proven targets, i.e., BmrR with a value of 49.243. The fit value of BmrR (Fit value = 0.5) was used as a predetermined threshold to select HPTs of berberine from the potential pharmacophores.
The selected poses of berberine with BACE1 (5ENM) and Aβ1-42 (2NAO) were subjected to 10 ns molecular dynamics (MD) simulations using DS. The stability of the complex was analyzed and confirmed by plotting root mean square deviation (RMSD). The RMSD is a measure of the deviation of the conformational stability of the proteins from backbone structure to the early starting structure and fundamental property investigation in MD. The binding energy of the stable complex was calculated using force field CHARMm which showed the sum of electrostatic and van de Waals interaction terms.
The interaction of berberine (Sigma) with BACE1 (Sigma) and Aβ1-42 (Sigma) was analyzed by SPR spectroscopy with a Biacore T200 biosensor instrument (GE Healthcare) (Yamasaki et al., 2013). Each target was immobilized onto flow cells in a CM5 chip using an amine-coupling method. Binding analyses were carried out at 25°C and a flow rate of 30 μl min-1. Berberine in running buffer (1 × PBS, 3 mM DTT and 5% dimethyl sulfoxide, pH = 7.4) was run over each target at gradient concentrations as indicated. An empty flow cell, without any immobilized protein, was used as a reference. The binding curves were analyzed using the steady-state affinity analysis supplied with the BIA evaluation software (GE Healthcare).
The inhibitory property of berberine on BACE1 was evaluated by a fluorescence resonance energy transfer (FRET) assay (Sigma) (Kennedy et al., 2016). Briefly, BACE1 enzyme and substrate were diluted in a Fluorescent Assay Buffer (Sigma) to produce 10× working solutions. Berberine was diluted into different concentrations in deionized water. This assay was performed in 96-well microplates using 100 μl, which comprised of 20 μl substrate solution, 2 μl BACE1 enzyme solution, and 5 μl berberine solution. The reaction was allowed to proceed for 2 h in the dark at 37°C. The product fluorescence before and after the reaction was measured by a POLARstar Omega multimode microplate spectrophotometer (BMG LABTECH) using 320 nm excitation and 405 nm emission wavelengths. The percentage of inhibition was calculated using the following equation: [1 - (S -S0)/(C -C0)] × 100, where S and C represent the fluorescence intensities in the presence and absence of berberine, respectively. Inhibition curves were created by graphing the percentage of inhibition versus the inhibitor concentration using linear regression.
Aβ1-42 was initially dissolved in HFIP (Sigma), evaporated, and re-dissolved in DMSO (Sigma) to a final concentration of 5 mM. To examine the effect of berberine on Aβ1-42 aggregation, peptide solutions containing Aβ1-42 monomers (10 μM) with and without berberine were incubated at 37°C for 48 h.
The morphological changes of Aβ1-42 aggregation in the presence and absence of berberine were characterized by TEM using a JEM-1011 transmission microscope (JOEL, Tokyo, Japan). Each sample was spotted onto glow-discharged, formvar-coated 300 mesh copper grids (Ted Pella Inc.), incubated for 1 min, dried, and then negatively stained with 2% uranyl acetate (Chu et al., 2016).
The aggregation kinetics of Aβ1-42 were monitored by incorporation of ThT. ThT solution was diluted in phosphate buffer (10 mM phosphate, 150 mM NaCl, pH = 7.0) to a final concentration of 20 μM. The fluorescence intensity for 20 μM Aβ1-42 incubated with and without berberine was measured at 37°C using a POLARstar Omega multimode microplate spectrophotometer (BMG LABTECH) under kinetic fluorometric mode. Measurements were carried out at an excitation wavelength of 450 nm and an emission of 485 nm. To account for background fluorescence, ThT intensity from solution without Aβ1-42 was subtracted from solution containing Aβ1-42. Raw data were fitted using BMG LABTECH’s MARS Data Analysis Software.
Thirty 120-day male APP/PS1 transgenic mice were purchased from the Jackson Laboratory (Bar Harbor, ME, United States). All mice were housed at 25°C under a 12-h light-dark cycle and given free access to standard laboratory food and water. The mice were randomly assigned into three groups: control group (n = 10), 50 mg/kg berberine (n = 10) and 100 mg/kg berberine (n = 10). Berberine was administrated to these mice in their drinking water for 4 months, starting from 4 months of age just before they developed cognitive impairment and key pathologic features. The dose of berberine was chosen according to previous studies with no gender differences (Kong et al., 2004). All experiments were conducted in compliance with the Animal Care and Institutional Ethical Guidelines and approved by the Biomedical Ethical Committee of Peking University.
All mice at 8 months of age were subjected to the Morris water maze task for 5 days for evaluation on their learning and memory abilities (Vorhees and Williams, 2006). The apparatus consisted of a circular white metal pool (160 cm in diameter and 50 cm in height) filled with 26 cm deep water at constant temperature (22 ± 1°C) throughout the experiment. The water pool was divided into four quadrants by the water maze software and had a translucent acrylic platform (12 cm in diameter and 24 cm in height) placed in the center of the northwest quadrant and 1.0–2.0 cm below the water surface. The acquisition trial for the mice was the last training trials to find the hidden platform at a target quadrant. The escape latency was recorded by the finding-platform time. At day 5, the mice were subjected to the probe trial to access spatial memory. The time in the target quadrant and crossing the former platform area were recorded for testing spatial memory.
Five-micrometer-thick sagittal paraffin sections of mouse brain were mounted on glass slides. The tissue sections were deparaffinized and rehydrated through graded ethanol washes. Antigen retrieval was conducted in 10 mM citrate buffer (pH = 6.0) and boiled for 2 min. The slides were incubated with 3% hydrogen peroxide for 10 min, and then blocked by 10% normal goat serum for 10 min at room temperature. After removing excess blocking buffer, indirect immunohistochemical staining was performed with anti-Aβ1-42 antibody (1:100, Covance Research Products, Berkeley, CA, United States) at 37°C for 1 h, washed thoroughly, and then incubated with biotinylated anti-mouse IgG antibody (1:100, Dako, Denmark) at 37°C for 45 min. The slides were detected using diaminobenzidine.
The results were conducted using Student’s t-test with SPSS 13.0 software (Chu et al., 2014). The data were expressed as means ± standard deviation (SD) of three independent experiments. Values of p < 0.05 were considered to be statistically significant.
Berberine is a natural isoquinoline alkaloid drawing increased attention for its favorable therapeutic effects on various diseases. The clinical efficacy of berberine is determined by its activity across multiple targets, including QacR, PLA2, BmrR, RamR, and d(CGTACG)2 DNA. Since the co-crystal structures of these targets in complex with berberine have been reported in the PDB database, these targets are selected as proven targets to generate MBM model for berberine. Two binding sites obtained for berberine consist in RamR, including site I and site II (Figure 1A). The co-crystal structures locate berberine close to the negatively charged binding sites (Figure 1B). The distinguishing feature appears to be the presence of buried acidic residues, such as Glu, Asp, and nucleic acids, which provide electrostatic interactions with the positively charged nitrogen (N+) of berberine. Distances between the N+ of berberine and the key anions in QacR, PLA2, BmrR, RamR site I, RamR site II and d(CGTACG)2 DNA are 4.29, 5.28, 4.88, 3.56, 3.75, and 3.79 Å, respectively (Figure 1B). Berberine has a large hydrophobic surface, which is ideal for interacting with the aromatic and aliphatic residues, constituting the hydrophobic pockets (Figure 1C). The binding pose of berberine and surrounding residues within the multi-target binding pockets are nearly identical (Figure 2A). The side chains from residues provide π–π and π-alkyl interactions to aid in electrostatic neutralization for the positive charge of berberine (Supplementary Figure S1). Molecular interactions reveal that berberine can establish strong attractive charge contacts with the hydrophobic moieties of neighboring residues within 7 Å (Figure 2B). Accordingly, pharmacophores for multiple targets were generated based on the atomic details of the binding features (Figure 2C). Through essential pharmacophore superimposition, a predetermined MBM model of berberine was developed for further target screening (Figure 2D).
FIGURE 1. The physicochemical properties of multi-target binding pockets with berberine. Berberine is shown as sticks with carbon, oxygen and nitrogen colored gray, red and blue, respectively. (A) The substrate-binding sites of QacR, PLA2, BmrR, RamR, and d(CGTACG)2 DNA in complex with berberine. Two binding sites appeared in RamR, including site I and site II. (B) The chemical properties of multi-target binding pockets in complex with berberine. The surfaces are shown in gradient colors, where electropositivity is colored blue, and electronegativity is colored red. The key anions are labeled and depicted as sticks. (C) The physical properties of multi-target binding pockets in complex with berberine. The surfaces are shown in gradient colors, where hydrophobia is colored brown, and hydrophile is colored blue.
FIGURE 2. The MBM model of berberine. The berberine and key residues are shown as sticks with carbon, oxygen and nitrogen colored gray, red, and blue. Electrostatic interactions are shown as dashed lines with π–π, π-alkyl and hydrogen bonds colored purple, pink, and green, respectively. (A) The binding motifs of berberine within QacR, PLA2, BmrR, RamR, and d(CGTACG)2 DNA. Two binding sites appeared in RamR, including site I and site II. Secondary structural elements are depicted as ribbons (coils, α-helices; arrows, β-sheets). Color is based on secondary structures (α-helices, red; β-sheets, skyblue; loops, green). (B) The molecular interactions of berberine with surrounding residues. Hydrophobic, acidic and excluded residues are colored brown, red and blue, respectively. (C) The multi-target pharmacophore models of berberine. The colored spheres identify the position and the type of binding features (aromatic rings, orange; hydrophobic portions, cyan; cations, red; anions, blue; hydrogen bond acceptors, green). (D) MBM model obtained for berberine. For clarity, the binding features are represented as circles. Color-coding is given as indicated.
Multi-target binding motifs-based screening started with the choice of potential targets for berberine by target fishing with two available pharmacophore databases, i.e., PharmaDB and HypoDB. Literature retrieval was simultaneously carried out to refine and supplement the profiling results. A complete list of 86 potential targets involved with 38 diseases was summarized in the Supplementary Table S1. According to the MBM-based comparison function, a fit value equal to or greater than 0.50 was used as a heuristic threshold to select HPTs of berberine from the hit pharmacophores (Table 1). Among the 13 candidates, there are two HPTs associated with AD, including BACE1 and Aβ1-42, which indicated that berberine tended to be beneficial for the patients with AD. To validate the stability of berberine with BACE1 (5ENM) and Aβ1-42 (2NAO), we performed standardized MD stimulations through Pipeline Pilot using the CHARMm component in DS. As shown in the Supplementary Figure S2, the most plausible models of berberine with BACE1 and Aβ1-42 were stable.
The Divine Farmer’s Classic of Materia Medica, finished in the Han Dynasty (206 BC-220 AD), documented that “Chinese goldthread can prevent the loss of memory with extended treatment,” which is described as an “upper herb.” The major active principle, i.e., berberine, has recently been demonstrated as an effective therapeutic remedy to prevent or delay the pathogenesis of AD. However, the underlying mechanisms of berberine against AD are poorly understood.
According to MBM-based screening, BACE1 and Aβ1-42 were predicted as HPTs for berberine, which lead to the aberrant production and aggregation of amyloid plaques in Alzheimer’s brains. Through application of molecular modeling, berberine was docked into the electronegative binding pocket of BACE1 (Supplementary Figure S3). The N+ of berberine engaged in electrostatic interactions with the key anion (Asp80) of BACE1, and the phenyl groups created π–π stacking with the Tyr119 active site residue (Figure 3A). A pharmacophore model was subsequently generated based on the interactions between berberine and BACE1 (Figure 3B). By fitting the pharmacophore against MBM, BACE1 was picked out as a HPT for berberine with a value of 0.788156 (Table 1). The complex of BACE1 with compound 10 (5ENM) was calculated using force field CHARMm which showed the binding energy to be -110.2 kcal/mol (Wu et al., 2016). The docked complex of BACE1 with berberine was more structurally stable and energetically favorable than the compound 10, with the binding energy reaching to -123.6 kcal/mol. Furthermore, the binding affinity of berberine to BACE1 was tested by Surface Plasmon Resonance (SPR). The equilibrium dissociation constant (KD) was calculated from SPR data to be 1.261 μM (Figure 3C). Moreover, we demonstrated that berberine effectively inhibited the activity of BACE1 with an IC50 of 62.96 μM, thereby decreasing the generation of Aβ1-42 (Figure 3D). Thus, BACE1 was identified as a new target of berberine.
FIGURE 3. The action of berberine on BACE1. (A) The binding motif of berberine within BACE1. Secondary structural elements are depicted as ribbons (coils, α-helices; arrows, β-sheets). Color-coding is based on the secondary structures (α-helices, red; β-sheets, skyblue; loops, green). The berberine and key residues are shown as sticks with carbon, oxygen and nitrogen colored gray, red, and blue. Electrostatic interactions are shown as dashed lines with π–π and π-alkyl colored purple and pink, respectively. (B) The pharmacophore model of berberine obtained for BACE1. The colored spheres identify the position and the type of binding features (aromatic rings, orange; hydrophobic portions, cyan; cations, red; anions, blue). (C) The binding of berberine to BACE1. The association and dissociation of berberine with immobilized BACE1 was analyzed using SPR. (D) The inhibition of BACE1 enzymatic activity by berberine in a concentration-dependent manner. The data are represented as the mean ± SD of five replicates.
With respect to Aβ1-42, berberine was docked into a large hydrophobic surface of the oligomer (Supplementary Figure S4). Molecular modeling revealed that berberine interacted with the residues by establishing hydrophobic bonds with Phe19 and Val24 (Figure 4A). The binding motif of berberine in Aβ1-42 was outlined from MBM-based screening with a fit value of 0.906099 (Figure 4B and Table 1). The docked complex of Aβ1-42 with berberine was calculated using force field CHARMm which showed the binding energy to be -73.6 kcal/mol. Moreover, the SPR responses indicated that berberine bound to Aβ1-42 oligomer with a KD value of 1.491 μM (Figure 4C). The aggregation kinetics of Aβ1-42 in the absence or presence of berberine were monitored by incorporation of Thioflavin T (ThT). ThT fluorescence intensity showed that berberine was able to inhibit the seeding capacity of Aβ1-42 in a dose-dependent manner (Figure 4D). Furthermore, transmission electron microscope (TEM) was employed to observe Aβ1-42 aggregates. TEM image of Aβ1-42 alone appeared characteristic fibrils after 48 h incubation at 37°C (Figure 4E). The fibrillization was disturbed when co-incubated with increasing concentration of berberine, indicating that Aβ1-42 tended to be a new target of berberine (Figure 4E).
FIGURE 4. The action of berberine on Aβ1-42 oligomer. (A) The binding motif of berberine within Aβ1-42 oligomer. Secondary structures are depicted as ribbons (coils, α-helices; arrows, β-sheets). Color-coding is based on the secondary structures (α-helices, red; β-sheets, skyblue; loops, green). The berberine and key residues are shown as sticks with carbon, oxygen and nitrogen colored gray, red, and blue. Electrostatic interactions are shown as dashed lines with π-alkyl groups colored pink. (B) The pharmacophore model of berberine obtained for Aβ1-42 oligomer. The colored spheres identify the position and the type of binding features (hydrophobic portions, cyan). (C) The binding of berberine to Aβ1-42 oligomer. The association and dissociation of berberine with immobilized Aβ1-42 oligomer was analyzed using SPR. (D) The inhibition of Aβ1-42 seeding capacity by berberine. The aggregation kinetics of Aβ1-42 in the absence or presence of berberine were monitored by incorporation of ThT. Error bars represent the SD of eight replicates. (E) The TEM images of Aβ1-42 aggregation with or without berberine at 37°C for 48 h. The scale bars indicate 100 nm. Magnification: 50,000×.
Furthermore, we studied the role of berberine in the prevention and treatment of AD. Spatial learning ability was observed on the basis of the time required to find the hidden platform. The berberine-treated APP/PS1 transgenic mice effectively improved their spatial learning ability in terms of daily escape latency through the 5-day consecutive training, compared with the control group (Figure 5A). However, no significant difference was assessed in swimming speed between the berberine-treated and the control groups (Figure 5B). Following the 5-day training, probe trials were conducted to assess both short-term (24 h) and long-term (72 h) memory tests on the 6th and 8th days, respectively. Significant differences were observed between the berberine-treated and the control mice (Figure 5C). In addition, the berberine-treated mice showed a significant improvement in the time spent searching for the pre-placed platform in both the short-term (p < 0.05) and long-term (p < 0.05) memory tests (Figure 5D). More importantly, the formation of amyloid plaque in the brain tissue was significantly inhibited for all the 50 mg/kg berberine (n = 10) and 100 mg/kg berberine (n = 10) treated mice, compared to the control group (n = 10) (Figure 5E). No significant difference was observed between 50 mg/kg berberine (n = 10) and 100 mg/kg berberine (n = 10) treated mice.
FIGURE 5. The neuroprotective properties of berberine in AD. Effect of berberine on spatial learning and memory of APP/PS1 mice were assessed by Morris water maze task. (A) The escape latency of mice were measured in 5-day training trials. Significant improvement in escape latency were observed in the berberine-treated mice (p < 0.05). (B) The swimming speed of mice during training trails. (C) The number of times crossing the target quadrant in both short-term memory and long-term memory. (D) The time of mice searching for the pre-placed platform in the target quadrant, in both short-term memory and long-term memory. Data shown are means ± SEM (n = 10). Significance was calculated by Student’s t-test. ∗p < 0.05. (E) Immunohistochemistry staining of the mice brain tissues with anti-Aβ1-42 antibody (4G8). Scale bar is 200 μm.
Based on relationship between drugs and targets, we built a space model for drug-target network. In the drug-target space, MBM represents a two-dimensional plane across all targets for one drug, which reveals that (i) single drug against multiple targets; (ii) multiple drugs against single target (Figure 6A). The resulting interactomes will connect all drug targets into a highly interlinked space, with strong local clustering of targets for each drug based on MBM modeling. To study the space, we developed a novel approach on the basis of enormous information on drug-target interactions. The rational design strategy includes the choice of potential targets, the generation of a dedicated MBM model, the MBM-based screening of HPTs, and the identification of new targets (Figure 6B). The candidates lead continue in an iterative process of reentering MBM determination and reevaluation for further optimization (Figure 6B). Key principles in this emerging field were tested through integrating the network pharmacology for berberine based on MBM screening (Figure 6C). Overall, 13 HPTs were predicted, with 2 targets verified experimentally, including BACE1 and Aβ1-42 involved with AD (Table 1). Our results indicated that berberine was beneficial to decrease the generation of Aβ1-42 via inhibiting the enzymatic activity of BACE1, and disrupt the aggregation of Aβ1-42 into amyloid plaques (Figure 6D). Further studies on these HPTs will elucidate the underlying mechanism of berberine against polypharmacological profiles.
FIGURE 6. Multi-target binding motifs (MBM)-based network pharmacology. (A) Drug-target space. Two-dimensional plans and circles correspond to MBMs and targets, respectively. Color-coding is given according to the spatial relationship between drug-MBMs and targets. (B) The workflow of MBM-based screening. (C) Network pharmacology obtained for berberine. A network view of berberine is generated by mapping drug-target networks onto biological networks on the basis of KEGG pathway database. Circles, squares, and triangles represent targets, biological pathways and diseases, respectively. Diseases are classified according to the International Classification of Diseases 10th Revision. Color codes are given in the legend. (D) The underlying mechanisms of berberine against AD. Berberine prevents the aberrant production and aggregation of Aβ1-42 in the pathogenesis of AD.
In drug discovery and development, the common analogy of drug action is that of a lock and key, with a correctly sized key (drug) fitting into the specific key hole (active cavity) of a lock (target). In recent years, it has been appreciated that a single drug can act as a ‘skeleton key’ that fits into multiple ‘locks’ of selective targets, coined as polypharmacology. Current studies in this emerging field focus on two major aspects, i.e., drug repurposing and multi-target drug design (March-Vila et al., 2017). The strategy relies on the knowledge of three-dimensional structures of biological targets, which is characterized as structure-based drug design (SBDD). During the past few decades, there has been a steep rise in the computer-aided drug design (CADD) that can assist in carrying out the process of SBDD from the selection of a target to the generation and evaluation of lead compounds effectively (Ramsay and Di Giovanni, 2017). Meanwhile, the explosion of structural information has provided exciting opportunities for SBDD (Réau et al., 2018). Herein we developed a novel approach for tackling the major sources of attrition in drug discovery, i.e., fact or fiction.
The strategy relies on the knowledge of drugs that bind highly site-specifically to the active cavity of targets. Prerequisite for an optimal supramolecular interaction is a perfect structural and physicochemical complementarity of drug and target. As a polypharmacological drug can act on multiple targets, the binding features of drugs are transformed into multi-target pharmacophores. The conformation that includes all the features is defined as a MBM model. The MBM theory explains how structurally diverse targets can bind with a common drug. According to the MBM, closely related targets identified through pharmacophore homology have the highest chance of cross reactivity and hence highest binding potential. Furthermore, the MBM model in turn can be used to design drugs that match the specific binding motif of a drug-target superfamily.
The inventive process of MBM-based screening starts with the choice of targets for a polypharmacological drug by target fishing with the pharmacophore databases. The hit targets are in need of literature evidence, and consequently classified into proven targets and potential targets. After analysis and curation of the binding motifs within the proven targets that have been co-crystallized with a common drug, a dedicated MBM model will be generated through essential pharmacophore superimposition. A MBM model is an ensemble of steric and electronic features that are necessary for recognition of a specific ligand by a broad and related category of molecules. A predetermined MBM model can be used to identify HPTs from the potential targets through pharmacophore comparison. Experiments are to be carried out to verify the affinity and efficacy. The approved targets will require co-crystallization with the drug to determine the binding modes. As increasing new targets become available, the MBM model will be updated to further refine it.
In this study, we gained insights into the network pharmacology for berberine by the application of MBM-based screening. Current studies have reported the co-crystal structures of five molecules in complex with berberine, including QacR, BmrR, RamR, PLA2 and d(CGTACG)2 DNA. Based on a detailed analysis of the interactions between berberine and its multiple proven targets, we generated a dedicated MBM model for berberine to identify HPTs from the 86 potential targets, including BACE1 and Aβ1-42. Each HPT is a node in a biological network that is linked to a particular disease, e.g., AD. AD is a progressive neurodegenerative disease, and the deposition of Aβ1-42 is the typical hallmark of AD pathology. In the amyloidogenic pathway, the amyloid precursor protein (APP) is sequentially cleaved by BACE1 to produce C-terminal fragment (C99), followed by cleavage by γ-secretase to produce Aβ peptides, including Aβ1-42 (Zhang et al., 2017). Recent studies have indicated that berberine could inhibit Aβ production (Cai et al., 2016). In particular, Zhang et al. (2017) indicated that berberine could reduce Aβ generation via decreasing the expression of BACE1. Herein, we focused mainly on the potential targets of berberine in AD. Consequently, we indicated that berberine could act as a high-affinity BACE1 inhibitor and prevented Aβ1-42 aggregation to delay the pathological process of AD, which further demonstrated that berberine tended to be beneficial for the patients with AD. These findings will provide new insights into the underlying mechanisms of berberine in the treatment of AD.
More importantly, a network view revealed that berberine can act on a node or combination of nodes whose perturbation results in a desired therapeutic outcome. Recent studies have identified several HPTs of berberine, including AMPK and MD-2, which confirmed the results of MBM-based screening (Chu et al., 2014; Zhang et al., 2017). Mapping the polypharmacology network for multi-target drugs onto the human disease network will enable a new network pharmacology approach to drug discovery. We believe that MBM-based network pharmacology will illuminate our understanding of drug action in diseases.
This study was carried out in accordance with the recommendations of the Animal Care and Institutional Ethical Guidelines in China. The protocol was approved by the Biomedical Ethical Committee of Peking University.
MC designed the experiments. MC, XC, JW, LG, QW, ZG, and JF performed the experiments. MC, XC, LG, JK, MZ, QG, BL, CZ and XG analyzed the results. MC wrote the manuscript. ZC and YDW revised the manuscript. All authors read and approved the final manuscript.
This work was supported by National Natural Science Foundation of China (81603119), Natural Science Foundation of Beijing Municipality (7174316), Leading Academic Discipline Project of Beijing Education Bureau (BMU20110254), Fostering Talents in Basic Science of the National Natural Science Foundation of China (J1030831/J0108), and Non-profit Central Research Institute Fund of Chinese Academy of Medical Sciences.
The authors declare that the research was conducted in the absence of any commercial or financial relationships that could be construed as a potential conflict of interest.
The Supplementary Material for this article can be found online at: https://www.frontiersin.org/articles/10.3389/fphar.2018.00801/full#supplementary-material
FIGURE S1 | Electrostatic interactions between berberine and key residues. The berberine and key residues are labeled and shown as sticks with carbon, oxygen, and nitrogen colored gray, red, and blue, respectively. The side chains from the residues provide π–π (A) and π-alkyl (B) interactions to aid in electrostatic neutralization for the positive charge of berberine. Electrostatic interactions are shown as dashed lines with π–π and π-alkyl colored purple and pink.
FIGURE S2 | Molecular dynamics of berberine with BACE1 and Aβ1-42. The stability of berberine with BACE1 (A) and Aβ1-42 (B) was validated using a standardized MD protocol through Pipeline Pilot using the CHARMm component in DS.
FIGURE S3 | The binding pocket of BACE1 with berberine. The binding surface is shown in gradient colors, where electropositivity is colored blue, and electronegativity is red. The berberine and Asp80 are shown as sticks with carbon, oxygen, and nitrogen colored gray, red, and blue, respectively. Distance between the N+ of berberine and Asp80 in BACE1 is 3.67 Å.
FIGURE S4 | The binding pocket of Aβ1-42 oligomer in complex with berberine. The binding surface is shown in gradient colors, where hydrophobia is colored brown, and hydrophile is colored blue. The berberine are shown as sticks with carbon, oxygen, and nitrogen colored gray, red, and blue, respectively.
TABLE S1 | Potential targets of berberine.
Ahmed, T., Gilani, A. U., Abdollahi, M., Daglia, M., Nabavi, S. F., and Nabavi, S. M. (2015). Berberine and neurodegeneration: a review of literature. Pharmacol. Rep. 67, 970–979. doi: 10.1016/j.pharep.2015.03.002
Anighoro, A., Bajorath, J., and Rastelli, G. (2014). Polypharmacology: challenges and opportunities in drug discovery. J. Med. Chem. 57, 7874–7887. doi: 10.1021/jm5006463
Battistutta, R., Mazzorana, M., Cendron, L., Bortolato, A., Sarno, S., Kazimierczuk, Z., et al. (2007). The ATP-binding site of protein kinase CK2 holds a positive electrostatic area and conserved water molecules. Chembiochem 8, 1804–1809. doi: 10.1002/cbic.200700307
Bernotas, R. C., Singhaus, R. R., Kaufman, D. H., Travins, J. M., Ullrich, J. W., Unwalla, R., et al. (2010). 4-(3-Aryloxyaryl)quinoline sulfones are potent liver X receptor agonists. Bioorg. Med. Chem. Lett. 20, 209–212. doi: 10.1016/j.bmcl.2009.10.132
Bhadra, K., and Kumar, G. S. (2011). Therapeutic potential of nucleic acid-binding isoquinoline alkaloids: binding aspects and implications for drug design. Med. Res. Rev. 31, 821–862. doi: 10.1002/med.20202
Cai, Z., Wang, C., and Yang, W. (2016). Role of berberine in Alzheimer’s disease. Neuropsychiatr. Dis. Treat. 12, 2509–2520. doi: 10.2147/NDT.S114846
Chandra, D. N., Abhilash, J., Prasanth, G. K., Sabu, A., Sadasivan, C., and Haridas, M. (2012). Inverted binding due to a minor structural change in berberine enhances its phospholipase A2 inhibitory effect. Int. J. Biol. Macromol. 50, 578–585. doi: 10.1016/j.ijbiomac.2012.01.029
Chappie, T. A., Humphrey, J. M., Allen, M. P., Estep, K. G., Fox, C. B., Lebel, L. A., et al. (2007). Discovery of a series of 6,7-dimethoxy-4-pyrrolidylquinazoline PDE10A inhibitors. J. Med. Chem. 50, 182–185. doi: 10.1021/jm060653b
Chen, Q., Mo, R., Wu, N., Zou, X., Shi, C., Gong, J., et al. (2017). Berberine ameliorates diabetes-associated cognitive decline through modulation of aberrant inflammation response and insulin signaling pathway in DM rats. Front. Pharmacol. 8:334. doi: 10.3389/fphar.2017.00334
Chu, M., Ding, R., Chu, Z. Y., Zhang, M. B., Liu, X. Y., Xie, S. H., et al. (2014). Role of berberine in anti-bacterial as a high affinity LPS antagonist binding to TLR4/MD-2 receptor. BMC Complement. Altern. Med. 14:89. doi: 10.1186/1472-6882-14-89
Chu, M., Zhang, M. B., Liu, Y. C., Kang, J. R., Chu, Z. Y., Yin, K. L., et al. (2016). Role of berberine in the treatment of methicillin-resistant Staphylococcus aureus infections. Sci. Rep. 6:24748. doi: 10.1038/srep24748
Chu, M., Zhou, M., Jiang, C., Chen, X., Guo, L., Zhang, M., et al. (2018). Staphylococcus aureus phenol-soluble modulins α1-α3 act as novel Toll-like receptor (TLR)4 antagonists to inhibit HMGB1/TLR4/NF-κB signaling pathway. Front. Immunol. 9:862. doi: 10.3389/fimmu.2018.00862
Crawford, J. M., Korman, T. P., Labonte, J. W., Vagstad, A. L., Hill, E. A., Kamari-Bidkorpeh, O., et al. (2009). Structural basis for biosynthetic programming of fungal aromatic polyketide cyclization. Nature 461, 1139–1143. doi: 10.1038/nature08475
Ferraroni, M., Bazzicalupi, C., Billa, A. R., and Gratteri, P. (2011). X-Ray diffraction analyses of the natural isoquinoline alkaloids berberine and sanguinarine complexed with double helix DNA d(CGTACG). Chem. Commun. 47, 4917–4919. doi: 10.1039/c1cc10971
Gong, J., Hu, M., Huang, Z., Fang, K., Wang, D., Chen, Q., et al. (2017). Berberine attenuates intestinal mucosal barrier dysfunction in type 2 diabetic rats. Front. Pharmacol. 8:42. doi: 10.3389/fphar.2017.00042
Hvistendahl, M. (2012). My microbiome and me. Science 336, 1248–1250. doi: 10.1126/science.336.6086.1248
Kang, T. S., Wang, W., Zhong, H. J., Dong, Z. Z., Huang, Q., Mok, S. W., et al. (2017). An anti-prostate cancer benzofuran-conjugated iridium (III) complex as a dual inhibitor of STAT3 and NF-κB. Cancer Lett. 396, 76–84. doi: 10.1016/j.canlet.2017.03.016
Kennedy, M. E., Stamford, A. W., Chen, X., Cox, K., Cumming, J. N., Dockendorf, M. F., et al. (2016). The BACE1 inhibitor verubecestat (MK-8931) reduces CNS β-amyloid in animal models and in Alzheimer’s disease patients. Sci. Transl. Med. 8:363ra150.
Kong, W., Wei, J., Abidi, P., Lin, M., Inaba, S., and Li, C. (2004). Berberine is a novel cholesterol-lowering drug working through a unique mechanism distinct from statins. Nat. Med. 10, 1344–1351. doi: 10.1038/nm1135
La, X., Zhang, L., Li, Z., Yang, P., and Wang, Y. (2017). Berberine-induced autophagic cell death by elevating GRP78 levels in cancer cells. Oncotarget 8, 20909–20924. doi: 10.18632/oncotarget.14959
Langendorf, C. G., Ngoei, K. R., Scott, J. W., Ling, N. X., Issa, S. M., Gorman, M. A., et al. (2016). Structural basis of allosteric and synergistic activation of AMPK by furan-2-phosphoic derivative C2 binding. Nat. Commun. 7:10912. doi: 10.1038/ncomms10912
Liu, J. B., Yang, C., Ko, C. N., Kasipandi, V., Yang, B., Lee, M. Y., et al. (2017). A long lifetime iridium (III) complex as a sensitive luminescent probe for bisulfite detection in living zebrafish. Sens. Actuators B Chem. 243, 971–976. doi: 10.1016/j.snb.2016.12.083
Liu, L. J., Wang, W., Huang, S. Y., Hong, Y., Li, G., Lin, S., et al. (2017). Inhibition of the Ras/Raf interaction and repression of renal cancer xenografts in vivo by an enantiomeric iridium (III) metal-based compound. Chem. Sci. 8, 4756–4763. doi: 10.1039/c7sc00311k
March-Vila, E., Pinzi, L., Sturm, N., Tinivella, A., Engkvist, O., Chen, H., et al. (2017). On the integration of in silico drug design methods for drug repurposing. Front. Pharmacol. 8:298. doi: 10.3389/fphar.2017.00298
Mast, N., Annalora, A. J., Lodowski, D. T., Palczewski, K., Stout, C. D., and Pikuleva, I. A. (2011). Structural basis for three-step sequential catalysis by the cholesterol side chain cleavage enzyme CYP11A1. J. Biol. Chem. 286, 5607–5613. doi: 10.1074/jbc.M110.188433
Moghaddam, H. K., Baluchnejadmojarad, T., Roghani, M., Khaksari, M., Norouzi, P., and Ahooie, M. (2014). Berberine ameliorate oxidative stress and astrogliosis in the hippocampus of STZ-induced diabetic rats. Mol. Neurobiol. 49, 820–826. doi: 10.1007/s12035-013-8559-7
Monteleone, S., Fuchs, J. E., and Liedl, K. R. (2017). Molecular connectivity predefines polypharmacology: aliphatic rings, chirality, and sp3 centers enhance target selectivity. Front. Pharmacol. 8:552. doi: 10.3389/fphar.2017.00552
Murray, C. W., Carr, M. G., Callaghan, O., Chessari, G., Congreve, M., Cowan, S., et al. (2010). Fragment-based drug discovery applied to Hsp90. Discovery of two lead series with high ligand efficiency. J. Med. Chem. 53, 5942–5955. doi: 10.1021/jm100059d
Newberry, K. J., Huffman, J. L., Miller, M. C., Vazquez-Laslop, N., Neyfakh, A. A., and Brennan, R. G. (2008). Structures of BmrR-drug complexes reveal a rigid multidrug binding pocket and transcription activation through tyrosine expulsion. J. Biol. Chem. 283, 26795–26804. doi: 10.1074/jbc.M804191200
Ogunjimi, A. A., Zeqiraj, E., Ceccarelli, D. F., Sicheri, F., Wrana, J. L., and David, L. (2012). Structural basis for specificity of TGFβ family receptor small molecule inhibitors. Cell. Signal. 24, 476–483. doi: 10.1016/j.cellsig.2011.09.027
Ohto, U., Fukase, K., Miyake, K., and Satow, Y. (2007). Crystal structures of human MD-2 and its complex with antiendotoxic lipid IVa. Science 316, 1632–1634. doi: 10.1126/science.1139111
Peters, J. U. (2013). Polypharmacology - foe or friend? J. Med. Chem. 56, 8955–8971. doi: 10.1021/jm400856t
Qin, J., Xie, P., Ventocilla, C., Zhou, G., Vultur, A., Chen, Q., et al. (2012). Identification of a novel family of BRAF (V600E) inhibitors. J. Med. Chem. 55, 5220–5230. doi: 10.1021/jm3004416
Ramsay, R. R., and Di Giovanni, G. (2017). Editorial: structure-based drug design for diagnosis and treatment of neurological diseases. Front. Pharmacol. 8:13. doi: 10.3389/fphar.2017.00013
Rastelli, G., and Pinzi, L. (2015). Computational polypharmacology comes of age. Front. Pharmacol. 6:157. doi: 10.3389/fphar.2015.00157
Réau, M., Langenfeld, F., Zagury, J. F., Lagarde, N., and Montes, M. (2018). Decoys selection in benchmarking datasets: overview and perspectives. Front. Pharmacol. 9:11. doi: 10.3389/fphar.2018.00011
Samanani, N., Park, S. U., and Facchini, P. J. (2005). Cell type-specific localization of transcripts encoding nine consecutive enzymes involved in protoberberine alkaloid biosynthesis. Plant Cell 17, 915–926. doi: 10.1105/tpc.104.028654
Schumacher, M. A., Miller, M. C., Grkovic, S., Brown, M. H., Skurray, R. A., and Brennan, R. G. (2001). Structural mechanisms of QacR induction and multidrug recognition. Science 294, 2158–2163. doi: 10.1126/science.1066020
Spatuzza, C., Postiglione, L., Covelli, B., Ricciardone, M., Benvenuti, C., and Mondola, P. (2014). Effects of berberine and red yeast on proinflammatory cytokines IL-6 and TNF-α in peripheral blood mononuclear cells (PBMCs) of human subjects. Front. Pharmacol. 5:230. doi: 10.3389/fphar.2014.00230
Tan, H. L., Chan, K. G., Pusparajah, P., Duangjai, A., Saokaew, S., Mehmood Khan, T., et al. (2016). Rhizoma coptidis: a potential cardiovascular protective agent. Front. Pharmacol. 7:362. doi: 10.3389/fphar.2016.00362
Taylor, C. E., and Greenough, W. B. III (1989). Control of diarrheal diseases. Annu. Rev. Public Health 10, 221–244. doi: 10.1146/annurev.pu.10.050189.001253
Vellaisamy, K., Li, G., Ko, C. N., Zhong, H. J., Fatima, S., Kwan, H. Y., et al. (2018). Cell imaging of dopamine receptor using agonist labeling iridium (III) complex. Chem. Sci. 9, 1119–1125. doi: 10.1039/c7sc04798c
Vorhees, C. V., and Williams, M. T. (2006). Morris water maze: procedures for assessing spatial and related forms of learning and memory. Nat. Protoc. 1, 848–858. doi: 10.1038/nprot.2006.116
Walti, M. A., Ravotti, F., Arai, H., Glabe, C. G., Wall, J. S., Böckmann, A., et al. (2016). Atomic-resolution structure of a disease-relevant Aβ(1-42) amyloid fibril. Proc. Natl. Acad. Sci. U.S.A. 113, 4976–4984. doi: 10.1073/pnas.1600749113
Wang, K., Feng, X., Chai, L., Cao, S., and Qiu, F. (2017). The metabolism of berberine and its contribution to the pharmacological effects. Drug Metab. Rev. 49, 139–157. doi: 10.1080/03602532.2017.1306544
Wang, W., Vellaisamy, K., Li, G., Wu, C., Ko, C. N., Leung, C. H., et al. (2017). Development of a long-lived luminescence probe for visualizing β-galactosidase in ovarian carcinoma cells. Anal. Chem. 89, 11679–11684. doi: 10.1021/acs.analchem.7b03114
Warmus, J. S., Flamme, C., Zhang, L. Y., Barrett, S., Bridges, A., Chen, H., et al. (2008). 2-Alkylamino- and alkoxy-substituted 2-amino-1,3,4-oxadiazoles–O-Alkyl benzohydroxamate esters replacements retain the desired inhibition and selectivity against MEK (MAP ERK kinase). Bioorg. Med. Chem. Lett. 18, 6171–6174. doi: 10.1016/j.bmcl.2008.10.015
Wu, Y. J., Guernon, J., Yang, F., Snyder, L., Shi, J., Mcclure, A., et al. (2016). Targeting the BACE1 active site flap leads to a potent inhibitor that elicits robust brain Aβ reduction in rodents. ACS Med. Chem. Lett. 7, 271–276. doi: 10.1021/acsmedchemlett.5b00432
Yamasaki, S., Nikaido, E., Nakashima, R., Sakurai, K., Fujiwara, D., Fujii, I., et al. (2013). The crystal structure of multidrug-resistance regulator RamR with multiple drugs. Nat. Commun. 4:2078. doi: 10.1038/ncomms3078
Yue, S. J., Liu, J., Feng, W. W., Zhang, F. L., Chen, J. X., Xin, L. T., et al. (2017). System pharmacology-based dissection of the synergistic mechanism of huangqi and huanglian for diabetes mellitus. Front. Pharmacol. 8:694. doi: 10.3389/fphar.2017.00694
Zhang, H., Zhao, C., Cao, G., Guo, L., Zhang, S., Liang, Y., et al. (2017). Berberine modulates amyloid-β peptide generation by activating AMP-activated protein kinase. Neuropharmacology 125, 408–417. doi: 10.1016/j.neuropharm.2017.08.013
Keywords: berberine, polypharmacology, multi-target binding motifs, drug-target space, Alzheimer’s disease
Citation: Chu M, Chen X, Wang J, Guo L, Wang Q, Gao Z, Kang J, Zhang M, Feng J, Guo Q, Li B, Zhang C, Guo X, Chu Z and Wang Y (2018) Polypharmacology of Berberine Based on Multi-Target Binding Motifs. Front. Pharmacol. 9:801. doi: 10.3389/fphar.2018.00801
Received: 21 April 2018; Accepted: 03 July 2018;
Published: 24 July 2018.
Edited by:
Vincent Kam Wai Wong, Macau University of Science and Technology, MacauReviewed by:
Dik-Lung Ma, Hong Kong Baptist University, Hong KongCopyright © 2018 Chu, Chen, Wang, Guo, Wang, Gao, Kang, Zhang, Feng, Guo, Li, Zhang, Guo, Chu and Wang. This is an open-access article distributed under the terms of the Creative Commons Attribution License (CC BY). The use, distribution or reproduction in other forums is permitted, provided the original author(s) and the copyright owner(s) are credited and that the original publication in this journal is cited, in accordance with accepted academic practice. No use, distribution or reproduction is permitted which does not comply with these terms.
*Correspondence: Ming Chu, ZmFtb3VzQGJqbXUuZWR1LmNu
†These authors have contributed equally to this work.
Disclaimer: All claims expressed in this article are solely those of the authors and do not necessarily represent those of their affiliated organizations, or those of the publisher, the editors and the reviewers. Any product that may be evaluated in this article or claim that may be made by its manufacturer is not guaranteed or endorsed by the publisher.
Research integrity at Frontiers
Learn more about the work of our research integrity team to safeguard the quality of each article we publish.