- College of Pharmacy and Graduate School of Pharmaceutical Sciences, Ewha Womans University, Seoul, South Korea
The primary goal of rational drug discovery is the identification of selective ligands which act on single or multiple drug targets to achieve the desired clinical outcome through the exploration of total chemical space. To identify such desired compounds, computational approaches are necessary in predicting their drug-like properties. G Protein-Coupled Receptors (GPCRs) represent one of the largest and most important integral membrane protein families. These receptors serve as increasingly attractive drug targets due to their relevance in the treatment of various diseases, such as inflammatory disorders, metabolic imbalances, cardiac disorders, cancer, monogenic disorders, etc. In the last decade, multitudes of three-dimensional (3D) structures were solved for diverse GPCRs, thus referring to this period as the “golden age for GPCR structural biology.” Moreover, accumulation of data about the chemical properties of GPCR ligands has garnered much interest toward the exploration of GPCR chemical space. Due to the steady increase in the structural, ligand, and functional data of GPCRs, several cheminformatics approaches have been implemented in its drug discovery pipeline. In this review, we mainly focus on the cheminformatics-based paradigms in GPCR drug discovery. We provide a comprehensive view on the ligand– and structure-based cheminformatics approaches which are best illustrated via GPCR case studies. Furthermore, an appropriate combination of ligand-based knowledge with structure-based ones, i.e., integrated approach, which is emerging as a promising strategy for cheminformatics-based GPCR drug design is also discussed.
Introduction
Rational drug design is the inventive process of identifying pharmaceutically-relevant drug candidates based on the information garnered from a biological target (Jazayeri et al., 2015). Discovery of ligands that modulate a target's activity has contributed largely to the understanding of both physiological and pathological processes (Wacker et al., 2017a). Navigating vast chemical space to identify such ligands seems a daunting task (Oprea and Gottfries, 2001; Lipinski and Hopkins, 2004). Techniques including medicinal chemistry, combinatorial chemistry, and high-throughput screening (HTS) are helpful in the identification of ligands, which can serve as effective modulators for pharmaceutically attractive targets. However, considering the astronomical number of possible drug-like candidates (~1023-1060), chemical space assessed by experimental techniques is still limited (Rodríguez et al., 2016; Mullard, 2017). In such a scenario, cheminformatics, which belongs to a part of the in silico realm, dominates in the exploration of a larger fraction of the chemical space.
Cheminformatics was defined by Brown (1998) as the combination of all available information that can be used in the optimization of a ligand to a potential drug candidate (Bajorath, 2004). This method aids in storing, searching, managing, and analyzing huge amount of chemical data, thereby expediting the development of novel ligand phenotypes (Bajorath, 2004; Valerio and Choudhuri, 2012). Additionally, the extraction of information and knowledge from chemical data could be helpful in the modeling of relationships between chemical structures and biological activities, and in the bioactivity prediction of other compounds from their structures (Schuffenhauer et al., 2006; Humbeck and Koch, 2017). Interestingly, cheminformatics fuses both chemical and biological data from drug candidates and drug targets, respectively, for the identification of new chemical entities (NCEs) and improvement of the reliability of data outcomes.
In the drug discovery pipeline, several cheminformatics approaches play a potent role in the identification of drug target and lead compounds, as well as in the prediction of ADMET properties (Figure 1). Chemogenomics-based databases, as well as computational polypharmacological analyses, have increased in popularity over the last several years as a supplementary method in the identification and validation of potential drug targets (Xie et al., 2014). Once a drug target is identified, the lead candidates with desirable properties are screened out of huge chemical compound libraries, thus underscoring the importance of cheminformatics tools in virtual screening (VS) (Varnek and Tropsha, 2008). Another potent cheminformatics approach, machine-learning is employed for the identification of novel drug candidates from lead compounds via generation of computational models (Lee et al., 2010, 2017; Varnek and Baskin, 2012; Mitchell, 2014). Other cheminformatics approaches including similarity and substructure searching could be utilized for the identification of novel scaffolds from large compounds repositories (Vass et al., 2016). The candidate compounds retrieved could be further docked onto the target protein to propose their possible binding affinities toward the target (Lenselink et al., 2016b). Upon identification of the drug-like candidates, these could be further evaluated for ADMET properties using computational models, thus helping in the elimination of undesired compounds at an early stage of drug discovery, and minimizing the time and costs involved.
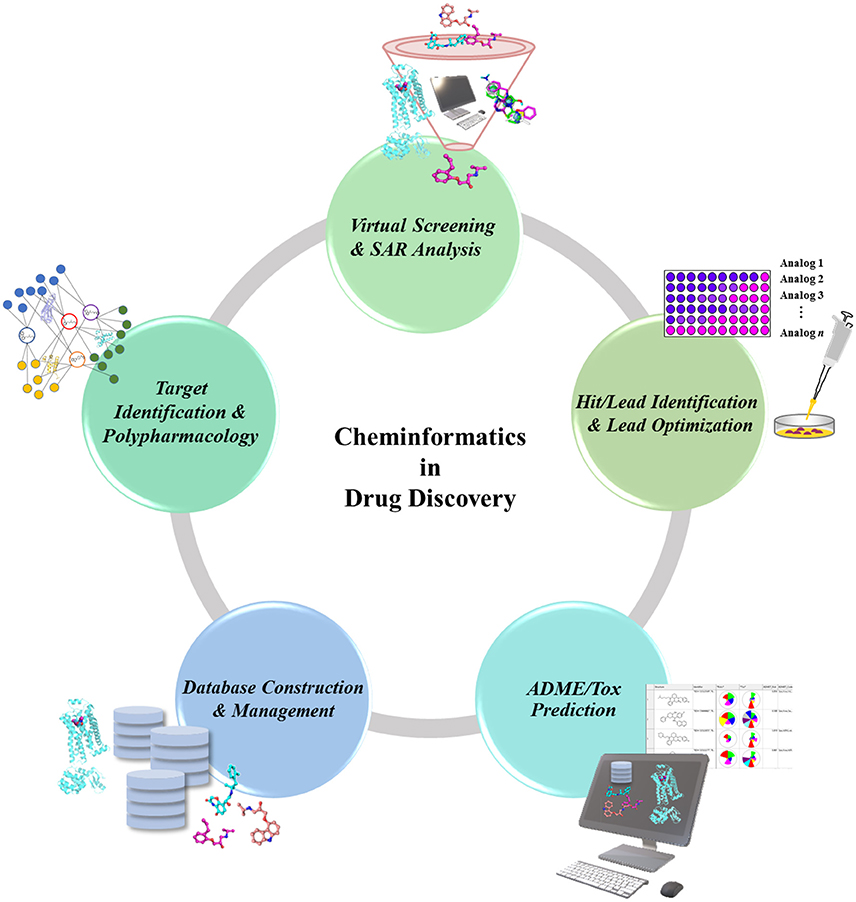
Figure 1. Role of Cheminformatics in the drug discovery process. Cheminformatics is involved in almost every step of the drug discovery pipeline due to the employment and analysis of available data to translate into valuable knowledge, which can in turn be used as a data for further studies.
G protein-coupled receptors (GPCRs) belong to a large family of signaling proteins that mediate cellular responses to most hormones, metabolites, cytokines, and neurotransmitters, and therefore serve as “fruitful targets” for drug discovery (Shoichet and Kobilka, 2012). More than 800 genes comprise this receptor family, which modulate several signaling processes involved in behavior, blood pressure regulation, cognition, immune response, mood, smell, and taste (Thomsen et al., 2005). GPCRs are categorized into six classes based on sequence and function, namely Class A—rhodopsin-like receptors, Class B—secretin family, Class C—metabotropic glutamate receptors, Class D—fungal mating pheromone receptors, Class E—cAMP receptors, and Class F—frizzled (FZD) and smoothened (SMO) receptors (Lee et al., 2018). All GPCR members share a common seven transmembrane (7TM) architecture linked by three extracellular (ECL) and three intracellular (ICL) loops (Ciancetta et al., 2015). However, they have low sequence identity and possess different extracellular N-terminal domains and diverse ligand-binding pockets (Figure 2). In case of class A GPCRs, the endogenous ligand is recognized by a ligand-binding site in the 7TM region. For class B GPCRs, the ligand is recognized by both extracellular and 7TM domains. For class C GPCRs, the ligand-binding pocket is found in the extracellular domain (ECD) that contains a Venus flytrap (VFT) module. In case of class F GPCRs, both SMO and FZD receptors possess an ECD that is comprised of an extracellular cysteine-rich domain (CRD) and an ECD linker domain. The endogenous lipoglycoprotein ligand, Wnt binds to the CRD of the FZD receptors (Wang et al., 2013; Wu et al., 2014). Upon ligand binding, GPCRs activate at least one of the two signaling partners, namely heterotrimeric GTP-binding proteins (G-proteins) or β-arrestins, and mediate signal flow via modulation of various downstream effectors.
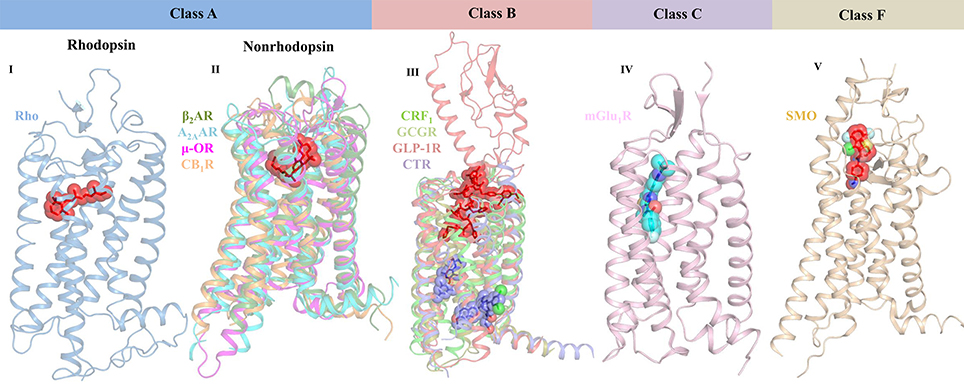
Figure 2. Crystal structures of representative GPCR-ligand complexes from classes A, B, C, and F presenting diverse ligand-binding sites. Class A GPCRs are classified into rhodopsin (bRho, PDB ID: 2HPY) and nonrhodopsin GPCRs. The representative structures of class A nonrhodopsin GPCRs which are further subdivided into aminergic-like (β2AR, PDB ID: 3P0G), nucleotide-like (A2AAR, PDB ID: 3QAK), peptide-like (μ-OR, PDB ID: 5C1M), and lipid-like receptors (CB1R, PDB ID: 5XRA) along with their bound ligands are shown. Similarly, representative structures for class B (CRF1 [PDB ID: 4K5Y], GCGR [PDB ID: 5EE7], full-length GLP-1R [PDB ID: 5NX2], and CTR [5UZ7]), class C (mGlu1R [PDB ID: 4OR2]), and class F (SMO [PDB ID: 4QIN] bound to negative allosteric modulator) are shown. Receptors are shown in cartoon representation and the ligands are shown as stick models with transparent surfaces. Agonists are represented as red sticks, antagonists are shown as purple sticks, and negative allosteric modulator is shown as blue stick model.
GPCR drug discovery has been successful and many of the world's top-selling drugs have targeted this receptor family (Sriram and Insel, 2018). Class A GPCRs are the most immensely investigated GPCR drug target within the drug market due to their centrality in diseases, structural availability, and relative ease of accessibility. The high druggability of GPCRs and its central role in diseases (including alzheimer's disease, cancer, diabetes, obesity, and psychiatric disorders) provide a strong spearhead for its continuous efforts in drug discovery and development (Tautermann, 2016). A recent study of all GPCR drugs and agents currently in clinical trials revealed that 475 drugs (i.e., ~34% of all drugs approved by Food and Drug administration [FDA]) mediate their effects through 108 unique GPCRs (Hauser et al., 2017). Additionally, the success rates for GPCR-targeted agents in the last 5 years were 78% (phase I), 39% (phase II), and 29% (phase III) (Hauser et al., 2017). The most recently FDA approved GPCR-targeted drug is Zilretta (triamcinolone acetonide extended-release injectable suspension), a glucocorticoid receptor agonist, which is used for the pain management of knee osteoarthritis (https://www.drugs.com/history/zilretta.html).
To utilize cheminformatics approaches in GPCR drug design, understanding the nature of the ligands, structural intricacies of the receptor, ligand-receptor interactions, and interaction of the receptors with downstream signaling complexes or other signaling partners is essential. Additionally, unveiling the relationships among ligand, receptor, and effector is necessary to investigate positive and negative allosterism, inverse agonism, biased signaling, and multimeric receptor pharmacology (Lane et al., 2017). Recent upsurge in the crystal structures of GPCRs provides a robust, 3D structural framework for identification of pharmaceutically-relevant ligands using ligand– and structure-based computational approaches, including molecular modeling of receptor dynamics, ligand docking, and virtual ligand screening (VLS) (Coudrat et al., 2017a). Following the successful application of VLS approaches in targets such as kinases, proteases, and other enzyme families, it is also becoming a popular ligand screening tool for GPCRs (Heifetz, 2018). The success of structure-based VLS could be visualized by the encouragingly high hit-rates ranging from 20 to 70% in the identification of novel ligands for several class A GPCRs (Table 1).
In this review, we deliver a comprehensive assessment on the state-of-the-art cheminformatics approaches for GPCR drug discovery with successful models from literature. Firstly, insights on GPCR ligand space and its recent structural advances are summarized. Subsequently, the key principles and boundaries of ligand–, structure-based, and integrated cheminformatics approaches in GPCR drug discovery are discussed in the main text. We also shed some light on the contemporary cheminformatics tools utilized in GPCR drug discovery. Additionally, the limitations associated with cheminformatics approaches have been discussed, which could assist the reader to rationale the best in silico tool during their research. Lastly, we conclude with a summary of the review contents and prospects of the cheminformatics approaches in GPCR drug discovery.
Booming Age of GPCR Structural Biology
The pioneering study of two-dimensional (2D) structure for bovine rhodopsin (bRho) in 1983 marked the beginning of GPCR structural biology (Hargrave et al., 1983). A decade later, 2D projection map was calculated from the solved 2D crystals of bRho using electron cryomicroscopy, which served as the basis for the construction of the receptor molecular model (Baldwin, 1993; Schertler et al., 1993). However, the first three-dimensional (3D) structure of bRho in its inactive state was released only in 2000 (Palczewski et al., 2000). Despite relentless efforts, elucidation of GPCR structures remained challenging due to several factors, including maintenance of structural integrity of the receptors by embedding in a membrane-like environment, presence of flexible ECLs and ICLs, low expression level of the receptor, and displaying basal signaling activity even in the absence of a ligand. However, all the aforementioned problems have been circumvented with the advances in GPCR crystallography, protein engineering, and innovations in biotechnology. Introduction of small, stable fusion proteins (T4 lysozyme and b562RIL) decreased the flexibility of the receptor regions (ICL3, ICL2, and N-terminal regions), and improved the crystal contacts. Likewise, antibody fragments or nanobodies improved the conformational stability of the receptors. Insertion of mutations (stabilized receptor (StaR) approach) enhanced the receptor thermostability in a particular conformational state and increased the protein expression levels.
The first structural breakthrough of a human GPCR, i.e., β2-adrenergic receptor (β2AR with a diffusible ligand), using different crystallization techniques came in 2007 (Cherezov et al., 2007). Moreover, the first crystal structures for GPCR classes B, C, and F have been solved (Hollenstein et al., 2013; Wang et al., 2013; Wu et al., 2014). So far, experimental structures of 44 distinct GPCRs and ~205 ligand-receptor complexes covering all the four classes, A–C, and F are available, of which most belong to the Class A subfamily (Hauser et al., 2017). It is to be noted that most of the existing GPCR structures are inactive ones, bound to an inhibitor. In the last year (2017) alone, more than 40 GPCR crystal structures have been determined which are listed in Table 2. GPCR structural studies have revealed the arrangement of the TM domains, location of the orthosteric, allosteric, bitopic, and biased ligand binding sites, homo– or heterooligomerization of receptors, and structural rearrangements involved in conformational changes upon GPCR activation or inactivation (Manglik and Kruse, 2017; Schrage and Kostenis, 2017). Besides garnering these 3D structural insights, the molecular basis of GPCR signal transduction coupled to G-proteins or β-arrestins were elucidated through X-ray crystallography and electron cryomicroscopy techniques. Oligomeric complex structures of bRho coupled to G-protein peptide (Rho/GαCT) (Scheerer et al., 2008), human Rho coupled to visual arrestins (Kang et al., 2015; Zhou et al., 2017), β2AR coupled to Gs-protein (Rasmussen et al., 2011) and β-arrestin 1 (Shukla et al., 2014), A2A adenosine receptor (A2AAR) in complex with a mini-Gs protein (Carpenter et al., 2016), glucagon-like peptide 1 receptor (GLP-1R) in complex with a Gs-protein (Zhang et al., 2017), and calcitonin receptor (CTR) coupled to Gs-protein (Liang et al., 2017) have been elucidated. These complex structures provide full mechanistic insights into GPCR and biased signaling, thus underpinning their functional significance and pharmacological targeting.
GPCRs are known to exist or function as monomers, dimers, and/or higher order oligomers, including homo– or hetero– dimers/oligomers (Guo et al., 2017). In addition to the accumulated experimental data through biochemical and biophysical techniques, the structural information on GPCR dimers or higher order oligomers were provided by X-ray crystallography. The first reported higher-order crystal structure of Rho and opsin in native membranes were reported in 2003 (Liang et al., 2003). Consequently, several structures including rhodopsin and nonrhodopsin class A GPCRs were elucidated (Lee et al., 2018). The oligomeric structures of GPCRs are essential for modulation of receptor function, mediation of cross-talk between GPCRs or other signaling pathways, and cellular trafficking, hence they have been associated with specific functional effects. Moreover, targeting these oligomeric structures as drug candidates could provide a new arena for drug development and specificity. The wealth of information supporting the existence of homo- and heterooligomers of GPCRs can be retrieved from the RCSB PDB (https://www.rcsb.org/pdb/home/home.do) or GPCR Oligomerization Knowledge Base (Khelashvili et al., 2010). In addition to these structural intricacies, GPCR signaling is also modulated by the presence of ligands other than orthosteric, which will be discussed in the following sections. Furthermore, adding details like GPCR dynamics to the structural information would provide a bigger picture to the biomedical researchers in this field. Such dynamic events triggered upon receptor activation or inhibition mechanisms could be covered by powerful methodologies including, bottom-up Hydrogen Deuterium eXchange Mass Spectrometry (HDX-MS) and resonance energy transfer (RET) (Li et al., 2015; Zhang, 2017). These important structural tools aid in better GPCR drug design by adding valuable information to our understanding of GPCR function, dynamics, protein-protein interactions, and receptor-ligand interactions (Vilardaga, 2011; Kauk and Hoffmann, 2017). Collectively, all the structural studies provide unprecedented insights into the structural and functional diversity of this receptor family. The wealth of structural information on all GPCRs is invaluable for ligand-based drug design (LBDD), structure-based drug design (SBDD), and integrated paradigms which complement traditional drug discovery efforts.
Insights Into GPCR Ligand Space
Various signaling pathways involve several GPCRs whose activities are mediated by ligand binding. Based on activation intensity, GPCR modulators can be divided into agonists, partial agonists, antagonists, and inverse agonists. Full agonists can stimulate maximal GPCR activity leading to recruitment of downstream proteins for signal transduction. Partial agonists, on the other hand, cannot induce 100% activation of receptors and acts as a type of antagonist while in the presence of full agonists. However, it can act as full agonists when there are excess receptors and in the absence of actual full agonists. Antagonists act as agonist blockers and can be divided into neutral antagonists and inverse agonists. Neutral antagonists can bind to GPCRs but do not affect the receptor's constitutive activity, whereas inverse agonists can block agonist effects. These modulators can directly interact with the orthosteric binding site of GPCRs (Wacker et al., 2017a).
While structural architecture of the TM region is largely conserved, the remarkable diversity in GPCR sequences are most notable in the ECL and ICL regions. This leads credence to the capacity of the GPCR family to interact with a wide range of ligands that vary in size, shape, and physicochemical properties, most of which bind to the orthosteric site to modulate receptor activity. In the ECL region, ECL2 plays a critical role in ligand recognition, access, and selectivity (Dror et al., 2011; Kruse et al., 2012; Zhang H. T. et al., 2015). For Class A GPCRs, lipophilic ligands often come from the lipid membrane and access the orthosteric site through the “lid” formed by the N-terminus and ECL2. In the case of hydrophilic ligands, ECL2 of different receptors only partially covers the ECL region through a variety of structures that shapes the entrance to the binding pocket (Venkatakrishnan et al., 2013). On the other hand, modulators of class B GPCRs are frequently peptide ligands, which possess large volume and high flexibility, requiring a more solvent-accessible orthosteric binding pocket (Liang et al., 2017).
Increase in static GPCR structures and advances in MD facilities have assisted in the elucidation of GPCR-ligand binding interaction. A thorough investigation of the ligand binding pocket of several GPCRs indicated the presence of multiple topologically equivalent residues that forms a consensus ligand binding network in almost all Class A receptors, providing an explanation for cross-reactivity and polypharmacology. Moreover, deviations from these consensus binding residues can account for ligand specificity in different GPCR members, and can thus be exploited in the design of specific and potent ligands (Venkatakrishnan et al., 2013). Regardless of the upsurge in information in the last few decades, it is still difficult to understand the differences in ligand binding requirements for agonists, antagonists, and inverse agonists of a given receptor, despite having almost identical structures (Figure 3). This calls for more studies focused on identifying key residues for agonism and antagonism, not only ligand binding specificity. Along with this, it is important to scrutinize activity cliffs of ligands as significant shifts in modulation type could be observed through small changes in ligand structures.
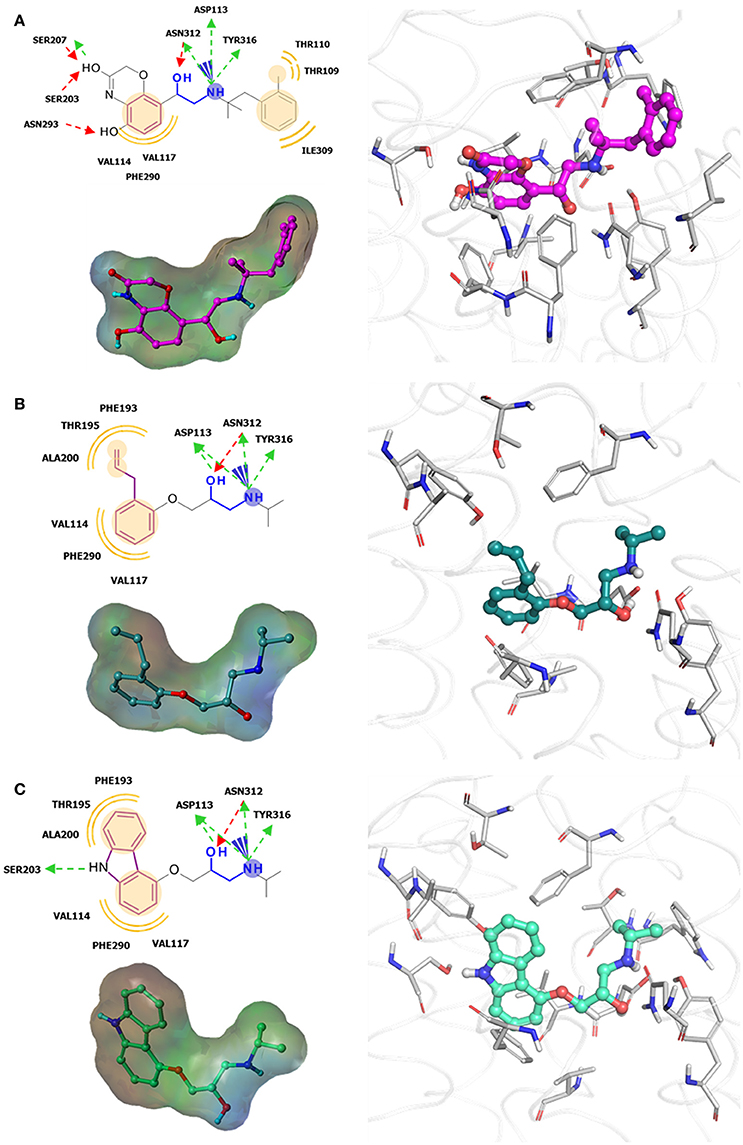
Figure 3. Examples of β2-adrenergic receptor (β2AR) orthosteric ligands with similar structures but possess different activities. (A) BI167,107 acts an agonist (PDB ID: 4LDE) (Ring et al., 2013), (B) alprenolol acts an antagonist (PDB ID: 3NYA) (Wacker et al., 2010), and (C) carazolol acts as an inverse agonist (PDB ID: 2RH1) (Cherezov et al., 2007).
Besides the orthosteric site, GPCR ligands can also bind to allosteric pockets and indirectly modulate receptor activity. Allosteric modulators can be divided into two types: (a) positive allosteric modulators (PAMs), which increases agonist affinity, and (b) negative allosteric modulators (NAMs), which acts as an allosteric antagonist or inverse agonist to decrease agonist affinity (Christopher et al., 2013, 2015; Kenakin, 2016). Additionally, there are some molecules that can both interact with orthosteric and allosteric sites, known as bitopic modulators (Dror et al., 2013; Fronik et al., 2017). Allosteric modulators can be either endogenous molecules, like sodium and cholesterol (Katritch et al., 2014), or exogenous molecules like natural products and synthetic compounds. Since allosteric modulators bind to sites other than the orthosteric site, they can co-bind with the putative ligand on the receptor to alter conformation and activity, thus affecting downstream signaling.
In case of CC chemokine receptor type 9 (CCR9), vercirnon (antagonist) was co-crystallized and unexpectedly found to interact with the intracellular binding site, blocking G-protein coupling (Oswald et al., 2016). Another example of an allosteric modulator is 1-(2-(2-(tert-butyl)phenoxy)pyridin-3-yl)-3-(4-(trifluoromethoxy)phenyl)urea (BPTU), which binds outside the purinergic P2Y1 receptor, flanking the TM bundle inside the lipid bilayer. While BPTU shows lower potency than known orthosteric antagonist, MRS2500, its allosteric interactions allow higher selectivity for the P2Y1 receptor (Zhang D. et al., 2015). Apart from small molecule compounds, ions can also function as an allosteric modulator, as illustrated by the discovery of the conserved allosteric binding pocket for Na+ in Class A GPCRs (Katritch et al., 2014).
The current rising star in GPCR research is biased signaling. Previously, GPCRs were presumed to exist as a simple two-state receptor model [“on” (activation) and “off” (inactivation)]. However, extensive analyses of different signaling pathways paved way to an exciting discovery that GPCRs have multiple conformations, each tailored to a specific response and downstream effect. Different ligands induce different receptor conformations, and each conformational state could initiate a specific downstream signal. While this finding increases the difficulty in drug discovery and design, there is also an opportunity to selectively block pathways implicated in various pathologies, while leaving normal homeostatic processes intact (Bologna et al., 2017). Typically, G protein signaling occurs upon agonist binding, whereas arrestin-mediated signaling occurs through arrestin binding. In this instance, GPCR drug design strategy could be dependent on identifying agonists biased for either G protein or arrestin signaling, leading to higher drug efficacy and diminished adverse effects (DeWire and Violin, 2011). Some excellent examples of biased ligands include lysergic acid diethylamide (LSD) (Wacker et al., 2017b), a well-known hallucinogen which appears to display bias toward β-arrestin signaling, and synthetic opioids TRV-130 (DeWire et al., 2013) and PZM-21 (Manglik et al., 2016), which are biased toward G protein signaling. Altogether, these accumulated data may provide extremely beneficial hints in the discovery and design of GPCR ligands based on the intended activity and targeted pathology. Figure 4 depicts some of the common GPCR modulators that are distinguished by activity types.
Cheminformatics-Based Paradigms in GPCR Drug Discovery
Cheminformatics Approaches Based on the Knowledge Derived From GPCR Ligands
Cheminformatics tools are frequently utilized in GPCR research due to the enormous amount of GPCR ligand data. Difficulties in crystallizing membrane proteins and receptor flexibility hindered structural elucidation and drug discovery research for this receptor. Due to these shortcomings, ligand-based approaches started to thrive in order to provide a better understanding of GPCR function and pharmacology. Some of the major ligand-based cheminformatics approaches are detailed below.
Cheminformatics and Virtual Screening
In silico screening method started to become popularly used after the integration of high throughput screening (HTS) and information technology (Coudrat et al., 2017b). Several computational and VS methods are frequently utilized in different stages of drug discovery and development, but some of the earliest and most commonly used ones are similarity- and QSAR-based strategies due to their efficiency and capability in analyzing simple 2D structures. These strategies are dependent on the principle that similar structures are predicted to display similar activities. Similarity-based methods need at least one established hit whose chemical structure is used to calculate pertinent molecular fingerprints, which is then employed to screen chemical libraries for compounds containing similar structure or fragments. On the other hand, QSAR-based strategies rely on the developed mathematical models which require an adequate number of biologically active compounds with activities covering a wide span of concentration. In this case, screening is dependent on the quality of the dataset used and the accuracy of the developed model (Luo et al., 2016).
Similarity-based VS was applied in a recent study for the discovery of a novel series of cannabinoid receptor 2 (CB2R) agonists (Gianella-Borradori et al., 2015). CB2R is a class A, lipid-like GPCR that regulates the effects of endogenously produced cannabinoid receptor ligands and has been implicated in several inflammatory diseases. In this study, an in-house database containing around 25,000 compounds was screened based on 40 low-energy conformations of known active and selective compound HU-308. Compounds were ranked based on their similarity with any of the 40 conformers of HU-308, and the top 94 were selected for biochemical screening based on the combined color score, which refers to chemistry alignment akin to pharmacophore features, and shape Tanimoto score, which accounts for 3D conformer overlay. From the initial hits, the top 16 active compounds displayed 6 new core scaffolds. Upon combined inspection of bioactivity, molecular weight, and lipophilicity, DIAS1 was chosen and used for further mining of the in-house library with the help of the newly identified scaffold. The second VS led to the discovery of DIAS2, which exhibited better activity and reduced lipophilicity as compared to DIAS1. Further structure-activity relationship (SAR) studies were performed for the optimization of the lead compound to improve potency, selectivity, and pharmacokinetic properties, resulting in candidate compounds that show nanomolar activity and selectivity for CB2R.
Another study used the US EPA's ToxCast database to develop QSAR models for 18 aminergic GPCRs (Mansouri and Judson, 2016). While the ToxCast program can screen hundreds of compounds in vitro to determine toxicity, the chemical space covered by their database is not enough to include all compounds of interest. However, the database can be employed in the development of predictive QSAR models. Two QSAR models were developed during the study, a qualitative (active vs. non-active) and a quantitative (potency value prediction) model. Various descriptors were calculated from the 2D structures of the compounds in the database and were subjected to genetic algorithms (GAs) to identify the best and most predictive descriptors. Several model-fitting methods, including PLSDA (partial least square discriminant analysis), SVMs (support vector machines), kNNs (k-nearest neighbors), and PLSs (partial least squares), were used to generate the QSAR models, which were later evaluated for accuracy and predictability. As a result, they were able to produce suitable models for aminergic GPCR assays and demonstrate the reliability of QSAR-based methods for analysis.
Cheminformatics and de Novo Ligand Design
Typically, ligand-based de novo drug design utilizes approved drugs or known inhibitors as reference structures or a source of pharmacophores that are relevant for bioactivity to build new chemical structures. While novelty and potency are always favored in drug discovery research, de novo structures should also have desirable pharmacokinetic properties (Kawashita et al., 2015). The combination of de novo drug design and computer-aided VS, along with the application of ADME/Tox models for the prediction of pharmacokinetic properties, has the capability of more effectively identifying NCEs with the desirable pharmacological activity profiles. In this sense, de novo drug design approach has become the forerunner of the long-envisioned personalized medicine where patients can be given custom-tailored drugs with increased efficacy and reduced adverse effects.
Rodrigues et al. worked on 5-hydroxytryptamine receptor subtype 2B (5-HT2B) drug discovery and were able to identify selective ligands through multidimensional de novo design (Rodrigues et al., 2015). In the Molecular Ant Algorithm (MAntA) software (Reutlinger et al., 2014), chemically advanced template search version 2 (CATS2), pharmacophores, and Morgan substructure fingerprints were employed to generate 5-HT2B selective ligands via reductive amination, resulting in over 5,000 new compound structures from which 4 were selected based on calculated 5-HT2B selectivity. To further improve selectivity and increase the scaffold diversity, de novo design software DOGS (Hartenfeller et al., 2012) and FDA-approved drug molecule structures were utilized to produce NCEs. The resulting compounds were screened with PAINS (Baell and Holloway, 2010) and ADMET filters (Lagorce et al., 2008) to remove undesirable molecules before performing experimental validation assays. Finally, four more compounds were obtained and among them, one compound showed promising selectivity for the 5-HT2B receptor. Even though the newly designed compound was not comparable in potency with the most potent existing antagonists, this study still provides an excellent application of de novo drug design in GPCR drug discovery field.
Cheminformatics and Chemical Genomics
While the number of currently available GPCR structures is increasing, it only covers a small portion of this protein superfamily and several other pharmaceutically relevant members are not yet elucidated. Chemical genomics can be applied to overcome the difficulty of target and drug identification by screening small molecule libraries and measuring their effects on entire biological systems or a specific group of targets, such as GPCRs. This combines the strength of traditional pharmaceutical techniques and genomics to facilitate discovery and validation of therapeutic targets, as well as identification of potential drug candidates for optimization (Hauser et al., 2018). Moreover, application of this strategy provides information concerning activated signaling pathways and biological effects through measurable gene expressions, leading to relevant data about target specificity and noninteraction pairs. In this sense, chemical genomics works on mining huge chemical data with the help of structural bioinformatics to rapidly identify target structure-function relationships (Valerio and Choudhuri, 2012). One of the most popular chemical genomics-based database found online is GLIDA (GPCR-Ligand Database), a publicly available Chemical Genomics database that can be used for GPCR drug discovery (Okuno et al., 2008). It contains GPCR biological and ligand information, as well as GPCR-ligand binding data. Therefore, it can be utilized for LBDD with the help of techniques such as machine learning-based classification and similarity-based search.
Shiraishi et al. reported an interesting research wherein chemical genomics approach was employed to predict GPCR-ligand interaction for class A GPCRs (Shiraishi et al., 2013). GPCR-ligand interaction data was collected from GVK Biosciences database and kernel methods were applied to evaluate compound-protein interaction (CPI) pair similarities based on Extended Connectivity Fingerprint (ECFP) and Dragon software descriptors generated for the ligands, along with target specific regions, such as full structure, loop region, and TM region. The results showed that compared to kernels accounting for the full structure and loop regions, kernels for the TM region showed significantly improved performance, which agrees with experimental findings that the TM region of class A GPCRs plays a critical role in ligand binding. Reliability of the machine learning model was improved with the addition of negative noninteraction pairs. Careful investigation of GPCR-ligand pairs revealed that high co-occurrence of residue-fragment pairs may be indicative of importance in ligand binding and specificity, as well as conservation of binding modes among Class A GPCRs. Key interactions identified in their study can be used for future VS and lead optimization studies and is beneficial when employed in combination with structure-based studies.
Cheminformatics, Polypharmacology, Drug Repositioning, and Repurposing
Recently, pharmaceutical research focuses not only on the discovery of novel compounds for a known target but also on the discovery of new indications for currently approved drugs. Polypharmacology has quickly emerged as a critical part of drug discovery research with the knowledge of how interconnected pathways in biological systems are. Though this field is most often used to investigate adverse effects and toxicity, information garnered from possible off-target effects can also offer information about new drug indications or cross-reactivity leading to higher drug efficacy (Jacobson et al., 2014). With the upsurge of polypharmacological information, it is no surprise that it is now frequently combined with cheminformatics strategies to predict off-target effects ahead of extensive biochemical analyses in order to save time and resources.
Xie et al. reported an interesting chemical genomics-based polypharmacology study focusing on GPCR-related drug abuse problem (Xie et al., 2014). Initially, a drug-abuse domain specific chemogenomics knowledgebase (DA-KB) was built to consolidate chemogenomics data regarding drug abuse and CNS diseases. This database was later used to investigate molecular interaction networks that encompass both drug abuse and GPCR modulation. Upon identifying 85 drug abuse-related GPCRs, distribution information of these receptors was collected and studied from the MetaCore database (Ekins et al., 2006). Using HTDocking (https://omictools.com/htdocking-tool) and GPCRDocking programs, polypharmacology and polydrug addiction analyses were performed to investigate the interactions between drug abuse-related receptors and ligands, along with cross-reactivities. As a result, the DA-KB became a powerful tool that has the capability of transforming data to useable polypharmacology knowledge. Moreover, TargetHunter server was also developed and can be used for target or off-target discovery.
Cheminformatics Approaches Based on the GPCR Structural Data
SBDD is one of the potent tools in lead discovery and optimization (Andrews et al., 2014). The application of SBDD is proven to be more efficient than traditional methods due to its working principle, which includes understanding the molecular basis of the disease and utilizing the 3D structural data of the target protein in the drug discovery pipeline (Cavasotto and Palomba, 2015). It has played a valuable role in several drug discovery projects involving enzyme targets (Wlodawer and Vondrasek, 1998; Varghese, 1999). Due to the difficulties in the expression and crystallography of GPCRs, there was only limited information available for SBDD of such targets. However, methodological advances in GPCR crystallography have paved way for the elucidation of several GPCR structures in the recent past. The availability of GPCR structures led to increased application of structure-based approaches in GPCR drug design, an area which has long been dominated by ligand-based ones. Breakthroughs in GPCR structural biology provide invaluable insights into the GPCR structure, function, and polypharmacology. The abundance of ligand-bound GPCR structures unveils the intricacies of ligand-receptor interactions, thus triggering a shift from conventional HTS techniques to less cost and highly efficient SBDD approaches for the design and discovery of potent ligands with improved pharmacological profiles. The main drawback of SBDD approaches lies on the scoring functions used by docking algorithms, wherein numerous approximations and restraints to protein flexibility are applied to expedite the process (Kim and Cho, 2016). In the following section, we briefly discuss the structure-based cheminformatics approaches for identifying novel GPCR ligands targeting ligand- and/or allosteric binding sites with few thriving models from the literature.
Identification of GPCR Novel Chemotypes via Structure-Based Virtual Screening
Utilizing crystal structures or homology models of target proteins in rational drug design is considered as the most powerful and popular method of choice in the design and/or screening of new lead compounds. In the early phase of drug discovery pipeline, structure-based virtual screening (SBVS) or docking-based VS has been utilized for the prediction of novel bioactive compounds from large and chemically diverse libraries (Cheng et al., 2012). In general, SBVS requires knowledge about the target's (protein or receptor) 3D structural information determined through experimental (X-ray or NMR) or in silico methods (homology modeling). Procedure involves docking of large chemical libraries of small compounds into crystal structure or homology model of the receptor. The selection criteria of small compounds for further experimental testings are based on the docking score, which assesses the binding affinity of protein-ligand complexes, predicted binding poses, chemical diversity, interactions with key residues, etc. (Ngo et al., 2016). The small compounds that cause a biological response are known as hits, which act as new chemical scaffolds for hit-to-lead development. The general VS workflow applied in several GPCR VS studies is shown in Figure 5.
SBVS studies for the first crystal structures of GPCRs, including β2AR, A2AAR, dopamine D3R, and histamine (H1R) have shown high hit rates. The pioneering study of SBVS for a druggable GPCR using the β2AR crystal structure was reported (Cherezov et al., 2007). In another SBVS, the authors utilized the inactive structure of β2AR/carazolol (PDB code: 2RH1) (Sabio et al., 2008) and screened proprietary and public databases for the identification of β2AR ligands. The hit rates obtained were 36 and 12%, respectively. Similarly, Kolb et al. (2009) docked ~1 × 106 commercially available compounds onto the same crystal structure and the top 25 virtual hits were selected based on their commercial availability, chemical diversity, and complementarity to the binding sites, and subjected for biological testings. Among them, six compounds had detectable binding affinities with the best one showing a Ki of 9 nM. All six hit molecules had novel chemotypes, and five of them were confirmed as inverse agonists. Apart from the reported VS studies using crystal structures, there were also few reports using receptor homology models. Langmead et al. identified highly potent and novel chemotype 1,3,5-triazine derivatives using A2AAR homology models (Langmead et al., 2012). A virtual library of 5.45 × 105 compounds was screened and the initial hits were selected based on the shape geometry and electrostatic properties of the orthosteric site. A hit rate of 9% was obtained and the structures were modified and optimized using X-ray crystallography and structure-based optimization techniques. This series of optimization led to the successful identification of AZD4635 (HTL-1071), which is in phase 1 clinical trials for immunooncology (Jazayeri et al., 2017).
Interestingly, a large-scale VS study was carried out by Lane et al. (2013) for the identification of both orthosteric and allosteric ligands of D3R. Based on the crystal structure of D3R, two optimized D3R models were prepared. To account for protein flexibility, conformers of D3R models were generated and subsequently evaluated by VS performance, i.e., conformers that can separate D3R actives from decoys were selected for the following analyses. The Molsoft Screen Pub database, which contains 4.1 × 106 compounds, was virtually screened using docking calculations. Top 300 hits in each model were selected and clustered by chemical similarity (0.3 Tanimoto distance). The top 25 compounds selected did not have a positively charged amine forming a conserved salt bridge to D1103.32, which is contrary to D3R apo model, but has interactions with TM1, 2, 3, and 7 as well as ECL1 and ECL2. These hits also reach dopamine and D1103.32 at the end of the orthosteric pocket. Finally, the predicted novel allosteric ligands were experimentally validated, showing distinct functional profiles on dopamine-signaling efficiency. Another SBVS approach identified nanomolar lead compounds for the melanin-concentrating hormone-1 receptor (MCH-1R) (Lionta et al., 2014). This approach combines GPCR molecular modeling, antagonist binding site prediction, design, synthesis, and a focused library screening. A primary hit compound from a pyranose-based VAST library was initially used for the construction of a high quality MCH-1R model. Furthermore, the model validation was performed using a virtual enrichment experiment, along with the model-driven structure-based expansion of the initial hit, for identification of potent interactions in the binding site. A SBVS of a library with ≤0.7 Tanimoto similarity to existing MCH-1R ligands provided a 14% hit rate and 10 unique chemotypes of potent MCH-1R inhibitors, including two nanomolar leads (Lionta et al., 2014).
In silico screening territory for classes B, C, and F largely remains uncharted due to the limited number of crystal structures available. Using SBVS approach, noncompetitive ligands (allosteric modulators) of related class B GPCRs, namely glucagon receptor (GLR) and glucagon-like peptide 1 receptor (GLP-1R), were identified (de Graaf et al., 2011b). Based on the crystal structure of corticotropin-releasing factor 1 receptor (CRF1R), a homology model for GLR was constructed. A database containing 1.9 × 106 compounds was assessed for chemical similarity to the current GLR noncompetitive inhibitors and docked onto the TM cavity of GLR. Based on the protein-ligand interaction fingerprints (IFPs), 23 compounds were selected and subjected for in vitro evaluations. Only two compounds were found to dose-dependently inhibit the effect of glucagon. One hit that was predicted as inactive for GLR bound to GLP-1R and potentiated a response similar to the endogenous GLP-1 ligand. For class C GPCRs, successful in silico VS studies were carried out against the VFT crystal structures (orthosteric N-terminal domain) of metabotropic glutamate receptor subtypes, mGlu3R and mGlu4R (Selvam et al., 2010). Besides the above-mentioned studies of VS campaigns, there are several computational works reported in the literature to discover novel orthosteric ligands for various GPCRs (which is well summarized in several review articles; Andrews et al., 2014; Cavasotto and Palomba, 2015; Shonberg et al., 2015; Ngo et al., 2016; Lee et al., 2018). Since SBVS on GPCRs is too broad to cover in this section, we have summarized representative case studies reported in the last 5 years (2013–2017) in Table 1.
Relevance of Fragment-Based Drug Discovery (FBDD) on GPCR Targets
Sequential piecing of fragments together to develop a novel lead compound is known as fragment-based drug discovery (FBDD) or fragment-based lead discovery (FBLD). FBDD is a potent scaffold-hopping and lead structure optimization tool for drug discovery projects and serves as an alternative to HTS (Matricon et al., 2017). The success of this approach in drug discovery campaign could be visualized by the increase in the number of compounds (originated from virtual fragment screens) entering clinical trials. A remarkable example of drugs identified via FBDD approach is vemurafenib, which was approved for the treatment of metastatic melanoma in 2011 (Baker, 2013). FBDD uses small molecules comprising ≤20 heavy atoms as a starting fragment for effective hit optimization. The main concept of this approach is to discover ligands that are smaller than a regular drug compound. The enlarged coverage of uncharted chemical space in fragment databases provides an exciting opportunity to find ligands after screening only a few thousand compounds (Chen et al., 2013). A fragment library can be designed and screened using molecular docking studies (Lee et al., 2018). The retrieved fragments could be further optimized using other computational approaches for growing, linking, or both.
Strategies utilized in the development of fragments into a lead compound include fragment growing, fragment linking, sequential docking, and group-based QSAR techniques. Fragment growth strategy initially begins with a fragment in the receptor' active site and allows extension of the fragment to maximize its interaction with the residues in the binding pocket. Fragment linking refers to the covalent linking of two or more fragments to form a single molecule which provides a new chemical scaffold in the active site. The application of FBDD to SBVS increases the structural space of hit-to-lead compounds. Even though ligands retrieved from fragment libraries lack selectivity and exhibit low affinity, they can be used as starting points for novel lead discovery. Despite its numerous advantages, there are still limitations associated with this approach, such as low accuracy prediction of fragment binding modes and rapid accumulation of errors. However, this approach proves to be useful when complemented with experimental techniques. Fragment screening of GPCR ligands via experimental methods (NMR, SPR, and X-ray crystallography) is challenging due to the difficulties in obtaining substantial amounts of functional protein, inherent conformational flexibility of the receptors outside the membrane, and low expression of the receptors (Lee et al., 2018). Therefore, in silico FBDD approaches could be utilized for GPCRs and other therapeutic targets. In the following paragraphs, we discuss the successful application of FBDD on GPCR drug discovery from literature.
The importance of in silico screening against GPCR protein structures or homology models to investigate novel fragment-like ligand chemical space is applicable for several GPCR targets. One of the first successful virtual fragment screening was developed by de Graaf et al. against doxepin bound human H1R crystal structure (de Graaf et al., 2011a; Shimamura et al., 2011). In this approach, molecular docking and receptor-ligand IFP protocols were combined to discover a chemically diverse set of new fragment-like H1R ligands. Out of 26 fragment-like compounds, 19 showed high binding affinity at the receptor level (hit rate 73%). Similarly, another structure-based virtual fragment screening (SBVFS) was performed against two GPCR targets, namely dopamine (D3R) crystal structure and H4R homology model structure, and an in-house fragment library of 12,905 fragments (Vass et al., 2014b). Additionally, molecular dynamics (MD) simulations were performed to represent different conformational states of the receptor orthosteric site (Vass et al., 2014b). Single structure- and ensemble docking screens were carried out for both receptors. The resulting 50 virtual hits were subjected for in vitro studies. Both the single and ensemble structures were found to be suitable for docking-based VS of fragments against GPCR targets. Chen et al. complemented in silico SBVFS with experimental biophysical screening to test the efficiency of their developed method (Chen et al., 2013). Initially, a set of 500 fragments were docked onto the orthosteric pocket of antagonist-bound A2AAR crystal structure (Jaakola et al., 2008) and ranked by affinity prior to target immobilized NMR screening of the same library (TINS). TINS resulted in 94 hits, where five fragments were identified to exceed the threshold affinity for the GPCR target. In the in silico screening, four out of five compounds were found in the top 50 fragments. Apart from these four fragments, the remaining 46 fragments also showed high binding affinities. Thus, a second computational screening approach using commercially available fragments (3.28 × 105) was performed and the 22 top-ranked compounds were tested experimentally. Among them, 14 fragments were identified as A2AAR ligands. Furthermore, QSAR studies were performed for three potent A2AAR ligands followed by optimization of the fragments by MD simulations and free-energy calculations. Similarly, another successful application of fragment-based screening and lead optimization using both biophysical and in silico techniques was shown in β1AR target leading to the discovery of novel high affinity leads (Christopher et al., 2013).
Verheij et al. studied target selectivity against histamine subtype H4R and 5-HT3A (ion channel) homology models using SBVFS approach (Verheij et al., 2011). The results of fragment-based screening showed that both receptors yielded a common pool of hit fragments, thus underlining remarkable similarities in ligand recognition. This knowledge could assist in efficiently navigating chemical space during hit optimization. Besides the orthosteric binding site (primary), allosteric sites (secondary) have also been targeted for identification of novel compounds by SBVFS approach. Vass et al. applied a sequential docking protocol to predict starting points for fragment linking using D3R crystal structure and D2R homology model to identify subtype selectivity (Vass et al., 2014a). Two in-house focused fragment libraries (196 fragments function as primary binding site ligands for D2 and D3 receptors and 266 fragments function as secondary binding site ligands for D3R) were docked in the orthosteric and allosteric binding sites and the best fragment combinations were listed. Similar top-scoring fragments were identified for the orthosteric site, whereas allosteric site fragments showed subtype selectivity. Three fragment-linked compounds that showed 9-, 39-, and 55-fold selectivity for D3R were synthesized, and docking results were validated by the experimental data.
In tandem with SBDD, FBDD has also been successfully applied to other GPCR classes. Novel mGlu5R NAMs were identified through combination of fragment-based screening and medicinal chemistry approaches (Christopher et al., 2015). In addition, the binding modes of NAMs with the receptor were crystallographically solved. Recently, an in silico fragment-based approach was applied on the crystal structures of mGlu5R (Doré et al., 2014; Christopher et al., 2015) for the design of novel allosteric modulators (Bian et al., 2017). Initially, a fragment library for reported GPCR allosteric modulators was constructed using the data from Allosteric Database (ASD). Subsequently, the novel compounds were generated and analyzed using retrosynthetic combinatorial analysis procedure (RECAP). Molecular docking was applied to screen the hits for the target by docking the in silico generated compounds into the binding pocket. Additionally, other computational methodologies, such as benchmark dataset verification, docking, QSAR model simulations, etc., were performed to assess validation of the hits. Twenty structurally diverse hits were predicted as potential mGlu5 allosteric modulators based on the binding energies and docking scores. This study highlights the importance of purely computational FBDD approach for facilitating the design of novel compounds for other targets as well. In addition to the above-mentioned GPCR case studies on SBVFS campaigns, there are several other in silico reports available regarding the discovery of novel ligands which are summarized elsewhere (Hubbard and Murray, 2011; Murray et al., 2012; Shoichet and Kobilka, 2012; Visegrády and Keseru, 2013; Andrews et al., 2014; Lee et al., 2018).
Integration of Ligand- and Structure-Based Cheminformatics Approaches
The use of cheminformatics in drug discovery provides an excellent foundation for the integration of structure- and ligand-based strategies due to its application in different stages of drug discovery. With the rising number of available structures, biological databases, and in silico techniques for cheminformatics and modern drug discovery, it is not surprising that ligand- and structure-based approaches are used in combination to take advantage of the abundant GPCR ligand information while employing recently elucidated crucial protein structural information to aid in increasing success in GPCR drug discovery research. Furthermore, integration of LBDD and SBDD complements strengths and weaknesses of each method, leading to better insights in critical ligand functionalities and receptor-ligand interaction information. Researchers are now able to use 3D protein structures to predict binding modes and study the pharmacology of known drugs and their analogs through docking, providing rationalization of ligand activity and useful SAR information for the design and optimization of new agonists and antagonists (Munk et al., 2016). In addition, rapid innovation of hardware and computing power allows the use of MD simulations for more in-depth study of GPCR ligand binding and activity modulation (McRobb et al., 2016; Clark, 2017).
An excellent case of ligand- and structure-based integration in GPCR drug discovery is shown in studies involving A2AAR, an attractive drug target for the treatment of Parkinson's disease. Since A2AAR receptor was one among the first GPCRs to be crystallized, it has become one of the most extensively studied drug target. The later release of a high-resolution A2AAR structure, which revealed the presence of water in the binding site, further increased the efforts for drug design and optimization. Over the years, most of A2AAR antagonists, such as istradefylline (Jenner, 2005) and preladenant (Neustadt et al., 2007), have been designed based on the purine scaffold and other related heterocycles. Although the abundance in ligand information for A2AAR helps in the elucidation of important chemical fingerprints and ligand binding interactions, it has become difficult to discover novel entities for drug development. In a study by Lenselink et al. (2016a), they performed VS using an ensemble of A2A receptor structures split into a structure-based decision tree (Lenselink et al., 2014). Ligands were docked to each protein structure and proceeded to the next receptor docking based on a GlideScore cut-off of the previous procedure. The resulting ligands were filtered using Rapid Elimination of Swill (REOS) (Walters and Namchuk, 2003) and re-scored using MM-GBSA. Consequently, similarity-based analysis (against compounds tested for A2AAR activity recorded in ChEMBL) was performed to determine the structural novelty of the remaining hits and select the most unique compounds to be tested experimentally. Out of 71 novel ligands, only 2 compounds displayed suitable A2AAR binding affinity. They also performed a retrospective analysis of the current A2AAR ligands to determine novelty in structure and its relation to observed A2AAR activity. Decades of research efforts for this target left little room for discovery of new ligand scaffolds, as seen in previous VS studies showing ligand Tanimoto similarity in the range of 0.19–0.68 (Carlsson et al., 2010; Katritch et al., 2010; Langmead et al., 2012; Rodriguez et al., 2015), with the lowest similarity showing the least activity. While most of the virtual hits were found to be similar in structure to experimentally validated compounds from ChEMBL, it should be noted that several of the tested compounds or scaffold structures were also discovered using computational methods, highlighting the value of in silico approaches in drug discovery and design.
Aside from combining known structure- and ligand-based methods, hybrid tools that assimilate features from both approaches have been developed to afford computational chemists other strategies which can compensate current individual limitations of SBDD and LBDD. One of the hybrid methods that has gained popularity in recent years is proteochemometric (PCM) modeling. PCM modeling is similar to traditional QSAR studies since both methods require descriptors, bioactivity data, and machine learning functions for model development (Qiu et al., 2017). However, a cross-term descriptor is also required in PCM modeling to consider amino acids and ligand functional groups that are crucial for binding interaction of the complex (Lapinsh et al., 2001; van Westen et al., 2011; Qiu et al., 2017). This method has been found to be useful on polypharmacological studies as it can provide information on target selectivity (Cortes-Ciriano et al., 2015), especially in large protein families like GPCRs. In a recent study by Gao et al. (2013), 24 PCM models were developed for amine GPCRs and their corresponding ligands using machine learning methods, support vector regression (SVR), and Gaussian processes (GP). Two typical descriptors were generated per receptor: z-scale and transmembrane identity descriptors, and two typical descriptors were generated for each ligand: general (atomic contributions, logP, etc.,) and drug-like index descriptors. These descriptors were first used to build 24 PCM models, which were validated using a test-set. Although, most of the models showed strong goodness-of-fit (R2) and predictivity (Q2), the addition of cross-terms led to a lower predictive capability of the PCM models. This may be because it is still difficult to fully translate receptor-ligand interfaces to a descriptor value. Despite this, their PCM models showed great potential in predicting cross interactions between GPCRs and ligands.
Summary of Cheminformatics Softwares/Tools Utilized in GPCR Drug Discovery
HTS has undergone technological advances and innovations that has rendered it as the principal method of drug discovery for years. However, it did not necessarily lead to a great leap forward in the discovery of NCEs as the hit rate for this method is frequently low, in addition to the enormous costs and efforts involved. In turn, computer-aided drug design (CADD) have been recognized and continuously receives increase in interest and usage such that most of GPCR drug discovery research efforts make use of one or more computational tools, especially in the initial stages of drug design. Due to the complexities of experimental GPCR research, it is of no surprise that CADD has emerged as a method of choice to expedite GPCR drug discovery and design. Furthermore, increasing knowledge of GPCR systems has led to the rising popularity of cheminformatics and chemogenomics as evidenced by the growing number of publicly available databases, which can provide structural or interaction information regarding receptor and its associated ligands.
There are several cheminformatics softwares and web servers available to identify lead compounds targeting GPCRs (Khan et al., 2011; Yadav et al., 2016). As mentioned previously, in silico approaches are classified into two approaches: SBDD and LBDD. If there are already known NMR and X-ray crystal structures or reliable homology models available, computational methods based on target protein structures can be exploited (Lyne, 2002). These tools are related with several computational approaches, including molecular docking, VS, pharmacophore generation, and binding pocket detection. As shown in Table 3, several in silico cheminformatics methods have been applied for GPCR targeted drug discovery. In cases where no protein structures are available, ligand-based virtual screening (LBVS) can be utilized. LBVS can be further sub-classified into three: pharmacophore-, similarity-, and machine learning-based VS (Basith et al., 2016). As shown in Table 4, several in silico cheminformatics methods could be exploited for generation of pharmacophores, searching 3D similarity, and identifying targets (polypharmacology). Moreover, commercially available chemical libraries for VS are shown in Table 5.
Limitations of Cheminformatics Approaches in GPCR Drug Discovery
In the last several years, the increasing number of high resolution GPCR structures has unlocked new avenues for structure-based GPCR drug discovery and design. However, several obstacles remain, including rapid identification of novel fragment-like compounds and structure-based elucidation of GPCR ligand function to name a few.
With the recent innovations in high-throughput, computer, and software technologies, as well as the upsurge of publicly available data, cheminformatics methodologies has no doubt become an essential part of most drug discovery efforts to date. However, a major flaw is seen during cheminformatics model development, wherein the experimental data used is assumed to be correct. In contrast to this assumption, databases can contain errors for ligand structures, bioactivity, activity types, and other information, which often results in ambiguous models leading to erroneous findings. Several recent articles (Fourches et al., 2010, 2016; Williams and Ekins, 2011; Williams et al., 2012) have discussed this topic at length and how it can have a negative effect on model development and performance. A study by Olah et al. (2005) mentioned that there were two molecules with incorrect structures on average for each medicinal chemistry journal, indicating a total error percentage of 8% in the WOMBAT database. Another more recent study by Tiikkainen et al. (2013), estimated the ligand error rates in ChEMBL, Liceptor, and WOMBAT databases to be 5, 7, and 6%, respectively. Error values for activity values in the three databases ranged from 1 to 2%. It is therefore important to carefully and manually curate chemical and biological databases, since even minor errors can cause a substantial decrease in the predictive capability of generated models. Moreover, while the increasing sophistication of computer programs has allowed researchers an atomistic view of several GPCR systems, approximations of crucial energy terms that cannot be computationally explored at present has greatly limited the accuracy in the perception of these systems. Because of these, researchers should constantly gauge findings against their own scientific knowledge to see whether the results are significant or not. It should always be remembered that computational tools are created and continuously developed to assist in making the drug discovery process more efficient, but nothing can replace a researcher's own knowledge and experience.
Moreover, insights about GPCR structure, function, and binding partners have increased significantly compared to a few decades ago. Despite this, a great deal of information is still beyond our fingertips, such as protein structures of hundreds of unique GPCRs and ligand information for orphan GPCRs. It is imperative not lose fervor in gathering new knowledge to further enhance our understanding of GPCR structures and functions.
Conclusions
In the nineteenth century, chemical space exploration was initiated as a counting game to estimate its size (Reymond, 2015). However, the advent of cheminformatics field and powerful in silico technologies assisted in the exploration of uncharted ligand space from large chemical libraries. The availability of large public and commercial chemical databases, as well as ligand chemical space exploration tools, provide researchers the ease of accessibility to handle and explore huge chemical data. Cheminformatics is a complex field of study that translates large data into useful knowledge for drug design and optimization protocols. The expansion of GPCR structures and ligands over the past decade is mainly due to the progress in its structural biology and theoretical advancements. These structural and in silico breakthroughs have led to the implementation of cheminformatics approaches in GPCR drug discovery pipeline. In the GPCR drug discovery protocol, ligand- and structure-based approaches are the most commonly applied ones. LBDD is known as a fast and simple technique for the identification of vital chemical functionalities required for biological activity. However, absence of binding pocket information limits its ability in incorporating several important factors, such as receptor flexibility and ligand bioactive conformation, thereby restricting the discovery of candidate leads to only the ligand classes used in model development (Saxena et al., 2017). But due to the prolonged absence of GPCR structures, researchers relied heavily on ligand-based methods for drug discovery and lead optimization, leading to copious ligand structural information for these targets. Following the crystallization of bRho in 2000 (Palczewski et al., 2000) and β2AR in 2007 (Rasmussen et al., 2007), a striking increase in GPCR structural information have been observed in the last several years. While the current available structures are unable to cover the structural diversity of GPCR protein family members, there is enough that can be used as templates for homology modeling to perform SBDD. In contrast to ligand-based techniques, SBDD can be used to predict ligand bioactive conformation, thus providing a better understanding of receptor-ligand interactions and allowing the discovery of NCEs. Furthermore, recent researches underpin the significance of emerging integrated approaches in GPCR drug design and discovery. Assimilating LBDD and SBDD methods, as well as the use of integrated approaches, has proven to increase the success rate of finding promising leads, especially for well-studied targets such as GPCRs. All the cheminformatics approaches discussed in this review are focused toward the identification of novel ligands for GPCR targets based on the structural and ligand data, where several case studies signify the importance of VS. The evolution of cheminformatics techniques and their synergy in GPCR drug discovery pipeline is the driving force that will facilitate cost-effective and prolific outcomes in the exploration of uncharted GPCR ligand space. Yet, an expert human touch is entailed to authenticate and tame the computer-generated outcome.
Author Contributions
SB and MC summarized the literature, wrote the manuscript, and prepared the figures. SM wrote part of the manuscript, prepared the figures, and revised the manuscript. JP and NC prepared the tables. SK and SC supervised all the works, provided critical comments, and wrote the manuscript.
Conflict of Interest Statement
The authors declare that the research was conducted in the absence of any commercial or financial relationships that could be construed as a potential conflict of interest.
Acknowledgments
This work was supported by the Mid-career Researcher Program (NRF-2017R1A2B4010084) funded by the Ministry of Science and ICT (MSIT) through the National Research Foundation of Korea (NRF).
References
Andrews, S. P., Brown, G. A., and Christopher, J. A. (2014). Structure-based and fragment-based GPCR drug discovery. ChemMedChem 9, 256–275. doi: 10.1002/cmdc.201300382
Baell, J. B., and Holloway, G. A. (2010). New substructure filters for removal of pan assay interference compounds (PAINS) from screening libraries and for their exclusion in bioassays. J. Med. Chem. 53, 2719–2740. doi: 10.1021/jm901137j
Bajorath, J. (2004). Chemoinformatics: Concepts, Methods, and Tools for Drug Discovery. New Jersey, NJ: Humana Press.
Baker, M. (2013). Fragment-based lead discovery grows up. Nat. Rev. Drug Discov. 12, 5–7. doi: 10.1038/nrd3926
Baldwin, J. M. (1993). The probable arrangement of the helices in G protein-coupled receptors. EMBO J. 12, 1693–1703.
Basith, S., Cui, M., Macalino, S. J. Y., and Choi, S. (2016). Expediting the design, discovery, and development of anticancer drugs using computational approaches. Curr. Med. Chem. 24, 4753–4778. doi: 10.2174/0929867323666160902160535
Bian, Y., Feng, Z., Yang, P., and Xie, X. Q. (2017). Integrated in silico fragment-based drug design: case study with allosteric modulators on metabotropic glutamate receptor 5. AAPS J. 19, 1235–1248. doi: 10.1208/s12248-017-0093-5
Bologna, Z., Teoh, J. P., Bayoumi, A. S., Tang, Y., and Kim, I. M. (2017). Biased G protein-coupled receptor signaling: new player in modulating physiology and pathology. Biomol. Ther. 25, 12–25. doi: 10.4062/biomolther.2016.165
Bresso, E., Togawa, R., Hammond-Kosack, K., Urban, M., Maigret, B., and Martins, N. F. (2016). GPCRs from fusarium graminearum detection, modeling and virtual screening - the search for new routes to control head blight disease. BMC Bioinform. 17:463. doi: 10.1186/s12859-016-1342-9
Brown, F. K. (1998). Chemoinformatics: what is it and how does it impact drug discovery. Ann. Rep. Med. Chem. 33, 375–384. doi: 10.1002/0471250953.bi1401s18
Carlsson, J., Yoo, L., Gao, Z. G., Irwin, J. J., Shoichet, B. K., and Jacobson, K. A. (2010). Structure-based discovery of A2A adenosine receptor ligands. J. Med. Chem. 53, 3748–3755. doi: 10.1021/jm100240h
Carpenter, B., Nehmé, R., Warne, T., Leslie, A. G., and Tate, C. G. (2016). Structure of the adenosine A(2A) receptor bound to an engineered G protein. Nature 536, 104–107. doi: 10.1038/nature18966
Cavasotto, C. N., and Palomba, D. (2015). Expanding the horizons of G protein-coupled receptor structure-based ligand discovery and optimization using homology models. Chem. Commun. 51, 13576–13594. doi: 10.1039/C5CC05050B
Chen, D., Ranganathan, A., Ijzerman, A. P., Siegal, G., and Carlsson, J. (2013). Complementarity between in silico and biophysical screening approaches in fragment-based lead discovery against the A(2A) adenosine receptor. J. Chem. Inf. Model. 53, 2701–2714. doi: 10.1021/ci4003156
Cheng, T., Li, Q., Zhou, Z., Wang, Y., and Bryant, S. H. (2012). Structure-based virtual screening for drug discovery: a problem-centric review. AAPS J. 14, 133–141. doi: 10.1208/s12248-012-9322-0
Cherezov, V., Rosenbaum, D. M., Hanson, M. A., Rasmussen, S. G., Thian, F. S., Kobilka, T. S., et al. (2007). High-resolution crystal structure of an engineered human beta2-adrenergic G protein-coupled receptor. Science 318, 1258–1265. doi: 10.1126/science.1150577
Cho, N. C., Seo, S. H., Kim, D., Shin, J. S., Ju, J., Seong, J., et al. (2016). Pharmacophore-based virtual screening, biological evaluation and binding mode analysis of a novel protease-activated receptor 2 antagonist. J. Comput. Aided Mol. Des. 30, 625–637. doi: 10.1007/s10822-016-9937-9
Christopher, J. A., Aves, S. J., Bennett, K. A., Doré, A. S., Errey, J. C., Jazayeri, A., et al. (2015). Fragment and structure-based drug discovery for a class C GPCR: discovery of the mGlu5 negative allosteric modulator HTL14242 (3-Chloro-5-[6-(5-fluoropyridin-2-yl)pyrimidin-4-yl]benzonitrile). J. Med. Chem. 58, 6653–6664. doi: 10.1021/acs.jmedchem.5b00892
Christopher, J. A., Brown, J., Doré, A. S., Errey, J. C., Koglin, M., Marshall, F. H., et al. (2013). Biophysical fragment screening of the beta1-adrenergic receptor: identification of high affinity arylpiperazine leads using structure-based drug design. J. Med. Chem. 56, 3446–3455. doi: 10.1021/jm400140q
Ciancetta, A., Sabbadin, D., Federico, S., Spalluto, G., and Moro, S. (2015). Advances in Computational techniques to study GPCR-ligand recognition. Trends Pharmacol. Sci. 36, 878–890. doi: 10.1016/j.tips.2015.08.006
Clark, T. (2017). G-Protein coupled receptors: answers from simulations. Beilstein J. Org. Chem. 13, 1071–1078. doi: 10.3762/bjoc.13.106
Cortes-Ciriano, I., Ul Ain, Q., Subramanian, V., Lenselink, E. B., Mendez-Lucio, O., IJzerman, A. P., et al. (2015). Polypharmacology modelling using proteochemometrics (PCM): recent methodological developments, applications to target families, and future prospects. MedChemComm 6, 24–50. doi: 10.1039/C4MD00216D
Coudrat, T., Christopoulos, A., Sexton, P. M., and Wootten, D. (2017a). Structural features embedded in G protein-coupled receptor co-crystal structures are key to their success in virtual screening. PLoS ONE 12:e0174719. doi: 10.1371/journal.pone.0174719
Coudrat, T., Simms, J., Christopoulos, A., Wootten, D., and Sexton, P. M. (2017b). Improving virtual screening of G protein-coupled receptors via ligand-directed modeling. PLoS Comput. Biol. 13:e1005819. doi: 10.1371/journal.pcbi.1005819
Cramer, R. D. (2003). Topomer CoMFA: a design methodology for rapid lead optimization. J. Med. Chem. 46, 374–388. doi: 10.1021/jm020194o
Daga, P. R., Polgar, W. E., and Zaveri, N. T. (2014). Structure-based virtual screening of the nociceptin receptor: hybrid docking and shape-based approaches for improved hit identification. J. Chem. Inf. Model. 54, 2732–2743. doi: 10.1021/ci500291a
de Graaf, C., Kooistra, A. J., Vischer, H. F., Katritch, V., Kuijer, M., Shiroishi, M., et al. (2011a). Crystal structure-based virtual screening for fragment-like ligands of the human histamine H(1) receptor. J. Med. Chem. 54, 8195–8206. doi: 10.1021/jm2011589
de Graaf, C., Rein, C., Piwnica, D., Giordanetto, F., and Rognan, D. (2011b). Structure-based discovery of allosteric modulators of two related class B G-protein-coupled receptors. ChemMedChem 6, 2159–2169. doi: 10.1002/cmdc.201100317
DeWire, S. M., and Violin, J. D. (2011). Biased ligands for better cardiovascular drugs: dissecting G-protein-coupled receptor pharmacology. Circ. Res. 109, 205–216. doi: 10.1161/CIRCRESAHA.110.231308
DeWire, S. M., Yamashita, D. S., Rominger, D. H., Liu, G., Cowan, C. L., Graczyk, T. M., et al. (2013). A G protein-biased ligand at the mu-opioid receptor is potently analgesic with reduced gastrointestinal and respiratory dysfunction compared with morphine. J. Pharmacol. Exp. Ther. 344, 708–717. doi: 10.1124/jpet.112.201616
Doré, A. S., Okrasa, K., Patel, J. C., Serrano-Vega, M., Bennett, K., Cooke, R. M., et al. (2014). Structure of class C GPCR metabotropic glutamate receptor 5 transmembrane domain. Nature 511, 557–562. doi: 10.1038/nature13396
Dror, R. O., Green, H. F., Valant, C., Borhani, D. W., Valcourt, J. R., Pan, A. C., et al. (2013). Structural basis for modulation of a G-protein-coupled receptor by allosteric drugs. Nature 503, 295–299. doi: 10.1038/nature12595
Dror, R. O., Pan, A. C., Arlow, D. H., Borhani, D. W., Maragakis, P., Shan, Y., et al. (2011). Pathway and mechanism of drug binding to G-protein-coupled receptors. Proc. Natl. Acad. Sci. U.S.A. 108, 13118–13123. doi: 10.1073/pnas.1104614108
Ekins, S., Bugrim, A., Brovold, L., Kirillov, E., Nikolsky, Y., Rakhmatulin, E., et al. (2006). Algorithms for network analysis in systems-ADME/Tox using the MetaCore and MetaDrug platforms. Xenobiotica 36, 877–901. doi: 10.1080/00498250600861660
Fourches, D., Muratov, E., and Tropsha, A. (2010). Trust, but verify: on the importance of chemical structure curation in cheminformatics and QSAR modeling research. J. Chem. Inf. Model. 50, 1189–1204. doi: 10.1021/ci100176x
Fourches, D., Muratov, E., and Tropsha, A. (2016). Trust, but verify II: a practical guide to chemogenomics data curation. J. Chem. Inf. Model. 56, 1243–1252. doi: 10.1021/acs.jcim.6b00129
Frandsen, I. O., Boesgaard, M. W., Fidom, K., Hauser, A. S., Isberg, V., Bräuner-Osborne, H., et al. (2017). Identification of histamine H3 receptor ligands using a new crystal structure fragment-based method. Sci. Rep. 7:4829. doi: 10.1038/s41598-017-05058-w
Friesner, R. A., Murphy, R. B., Repasky, M. P., Frye, L. L., Greenwood, J. R., Halgren, T. A., et al. (2006). Extra precision glide: docking and scoring incorporating a model of hydrophobic enclosure for protein-ligand complexes. J. Med. Chem. 49, 6177–6196. doi: 10.1021/jm051256o
Fronik, P., Gaiser, B. I., and Sejer Pedersen, D. (2017). Bitopic ligands and metastable binding sites: opportunities for G protein-coupled receptor (GPCR) medicinal chemistry. J. Med. Chem. 60, 4126–4134. doi: 10.1021/acs.jmedchem.6b01601
Gandhimathi, A., and Sowdhamini, R. (2016). Molecular modelling of human 5-hydroxytryptamine receptor (5-HT2A) and virtual screening studies towards the identification of agonist and antagonist molecules. J. Biomol. Struct. Dyn. 34, 952–970. doi: 10.1080/07391102.2015.1062802
Gao, J., Huang, Q., Wu, D., Zhang, Q., Zhang, Y., Chen, T., et al. (2013). Study on human GPCR-inhibitor interactions by proteochemometric modeling. Gene 518, 124–131. doi: 10.1016/j.gene.2012.11.061
Gianella-Borradori, M., Christou, I., Bataille, C. J., Cross, R. L., Wynne, G. M., Greaves, D. R., et al. (2015). Ligand-based virtual screening identifies a family of selective cannabinoid receptor 2 agonists. Bioorg. Med. Chem. 23, 241–263. doi: 10.1016/j.bmc.2014.11.002
Grigoryan, A. V., Kufareva, I., Totrov, M., and Abagyan, R. A. (2010). Spatial chemical distance based on atomic property fields. J. Comput. Aided Mol. Des. 24, 173–182. doi: 10.1007/s10822-009-9316-x
Guo, H., An, S., Ward, R., Yang, Y., Liu, Y., Guo, X. X., et al. (2017). Methods used to study the oligomeric structure of G-protein-coupled receptors. Biosci. Rep. 37:BSR20160547. doi: 10.1042/BSR20160547
Hargrave, P. A., McDowell, J. H., Curtis, D. R., Wang, J. K., Juszczak, E., Fong, S. L., et al. (1983). The structure of bovine rhodopsin. Biophys. Struct. Mech. 9, 235–244. doi: 10.1007/BF00535659
Hartenfeller, M., Zettl, H., Walter, M., Rupp, M., Reisen, F., Proschak, E., et al. (2012). DOGS: reaction-driven de novo design of bioactive compounds. PLoS Comput. Biol. 8:e1002380. doi: 10.1371/journal.pcbi.1002380
Hauser, A. S., Attwood, M. M., Rask-Andersen, M., Schiöth, H. B., and Gloriam, D. E. (2017). Trends in GPCR drug discovery: new agents, targets and indications. Nat. Rev. Drug Discov. 16, 829–842. doi: 10.1038/nrd.2017.178
Hauser, A. S., Chavali, S., Masuho, I., Jahn, L. J., Martemyanov, K. A., Gloriam, D. E., et al. (2018). Pharmacogenomics of GPCR drug targets. Cell 172, 41–54. doi: 10.1016/j.cell.2017.11.033
Hawkins, P. C., Skillman, A. G., and Nicholls, A. (2007). Comparison of shape-matching and docking as virtual screening tools. J. Med. Chem. 50, 74–82. doi: 10.1021/jm0603365
Hollenstein, K., Kean, J., Bortolato, A., Cheng, R. K., Doré, A. S., Jazayeri, A., et al. (2013). Structure of class B GPCR corticotropin-releasing factor receptor 1. Nature 499, 438–443. doi: 10.1038/nature12357
Hubbard, R. E., and Murray, J. B. (2011). Experiences in fragment-based lead discovery. Methods Enzymol. 493, 509–531. doi: 10.1016/B978-0-12-381274-2.00020-0
Humbeck, L., and Koch, O. (2017). What can we learn from bioactivity data? Chemoinformatics tools and applications in chemical biology research. ACS Chem. Biol. 12, 23–35. doi: 10.1021/acschembio.6b00706
Irwin, J. J., Sterling, T., Mysinger, M. M., Bolstad, E. S., and Coleman, R. G. (2012). ZINC: a free tool to discover chemistry for biology. J. Chem. Inf. Model. 52, 1757–1768. doi: 10.1021/ci3001277
Jaakola, V. P., Griffith, M. T., Hanson, M. A., Cherezov, V., Chien, E. Y., Lane, J. R., et al. (2008). The 2.6 angstrom crystal structure of a human A2A adenosine receptor bound to an antagonist. Science 322, 1211–1217. doi: 10.1126/science.1164772
Jacobson, K. A., Costanzi, S., and Paoletta, S. (2014). Computational studies to predict or explain G protein coupled receptor polypharmacology. Trends Pharmacol. Sci. 35, 658–663. doi: 10.1016/j.tips.2014.10.009
Jain, A. N. (2007). Surflex-Dock 2.1: robust performance from ligand energetic modeling, ring flexibility, and knowledge-based search. J. Comput. Aided Mol. Des. 21, 281–306. doi: 10.1007/s10822-007-9114-2
Jang, J. W., Cho, N. C., Min, S. J., Cho, Y. S., Park, K. D., Seo, S. H., et al. (2016). Novel scaffold identification of mGlu1 receptor negative allosteric modulators using a hierarchical virtual screening approach. Chem. Biol. Drug Des. 87, 239–256. doi: 10.1111/cbdd.12654
Jazayeri, A., Andrews, S. P., and Marshall, F. H. (2017). Structurally enabled discovery of adenosine A2A receptor antagonists. Chem. Rev. 117, 21–37. doi: 10.1021/acs.chemrev.6b00119
Jazayeri, A., Dias, J. M., and Marshall, F. H. (2015). From G protein-coupled receptor structure resolution to rational drug design. J. Biol. Chem. 290, 19489–19495. doi: 10.1074/jbc.R115.668251
Jenner, P. (2005). Istradefylline, a novel adenosine A2A receptor antagonist, for the treatment of Parkinson's disease. Expert Opin. Investig. Drugs 14, 729–738. doi: 10.1517/13543784.14.6.729
Jones, G., Willett, P., Glen, R. C., Leach, A. R., and Taylor, R. (1997). Development and validation of a genetic algorithm for flexible docking. J. Mol. Biol. 267, 727–748. doi: 10.1006/jmbi.1996.0897
Kaczor, A. A., Silva, A. G., Loza, M. I., Kolb, P., Castro, M., and Poso, A. (2016). Structure-based virtual screening for dopamine D2 receptor ligands as potential antipsychotics. ChemMedChem 11, 718–729. doi: 10.1002/cmdc.201500599
Kang, Y., Zhou, X. E., Gao, X., He, Y., Liu, W., Ishchenko, A., et al. (2015). Crystal structure of rhodopsin bound to arrestin by femtosecond X-ray laser. Nature 523, 561–567. doi: 10.1038/nature14656
Kastner, K. W., Shoue, D. A., Estiu, G. L., Wolford, J., Fuerst, M. F., Markley, L. D., et al. (2014). Characterization of the Anopheles gambiae octopamine receptor and discovery of potential agonists and antagonists using a combined computational-experimental approach. Malar. J. 13:434. doi: 10.1186/1475-2875-13-434
Katritch, V., Fenalti, G., Abola, E. E., Roth, B. L., Cherezov, V., and Stevens, R. C. (2014). Allosteric sodium in class A GPCR signaling. Trends Biochem. Sci. 39, 233–244. doi: 10.1016/j.tibs.2014.03.002
Katritch, V., Jaakola, V. P., Lane, J. R., Lin, J., Ijzerman, A. P., Yeager, M., et al. (2010). Structure-based discovery of novel chemotypes for adenosine A(2A) receptor antagonists. J. Med. Chem. 53, 1799–1809. doi: 10.1021/jm901647p
Kauk, M., and Hoffmann, C. (2017). Intramolecular and intermolecular FRET sensors for GPCRs - monitoring conformational changes and beyond. Trends Pharmacol. Sci. 39, 123–135. doi: 10.1016/j.tips.2017.10.011
Kawashita, N., Yamasaki, H., Miyao, T., Kawai, K., Sakae, Y., Ishikawa, T., et al. (2015). A mini-review on chemoinformatics approaches for drug discovery. J. Comput. Aided Chem. 16, 15–29. doi: 10.2751/jcac.16.15
Kelemen, Á. A., Kiss, R., Ferenczy, G. G., Kovács, L., Flachner, B., Lorincz, Z., et al. (2016). Structure-based consensus scoring scheme for selecting class A aminergic GPCR fragments. J. Chem. Inf. Model. 56, 412–422. doi: 10.1021/acs.jcim.5b00598
Kenakin, T. P. (2016). Pharmacology in Drug Discovery and Development: Understanding Drug Response. Boston, MA: Elsevier; Academic Press.
Khan, F., Yadav, D. K., Maurya, A., Sonia, and Srivastava, S. K. (2011). Modern methods & web resources in drug design & discovery. Lett. Drug Des. Discov. 8, 469–490. doi: 10.2174/157018011795514249
Khelashvili, G., Dorff, K., Shan, J., Camacho-Artacho, M., Skrabanek, L., Vroling, B., et al. (2010). GPCR-OKB: the G protein coupled receptor oligomer knowledge base. Bioinformatics 26, 1804–1805. doi: 10.1093/bioinformatics/btq264
Kim, M., and Cho, A. E. (2016). Incorporating, QM and solvation into docking for applications to GPCR targets. Phys. Chem. Chem. Phys. 18, 28281–28289. doi: 10.1039/C6CP04742D
Kolb, P., Rosenbaum, D. M., Irwin, J. J., Fung, J. J., Kobilka, B. K., and Shoichet, B. K. (2009). Structure-based discovery of beta2-adrenergic receptor ligands. Proc. Natl. Acad. Sci. U.S.A. 106, 6843–6848. doi: 10.1073/pnas.0812657106
Kooistra, A. J., Vischer, H. F., McNaught-Flores, D., Leurs, R., de Esch, I. J. P., and de Graaf, C. (2016). Function-specific virtual screening for GPCR ligands using a combined scoring method. Sci. Rep. 6:28288. doi: 10.1038/srep28288
Kramer, B., Rarey, M., and Lengauer, T. (1999). Evaluation of the FLEXX incremental construction algorithm for protein-ligand docking. Proteins 37, 228–241. doi: 10.1002/(SICI)1097-0134(19991101)37:2<228::AID-PROT8>3.0.CO;2-8
Kruse, A. C., Hu, J., Pan, A. C., Arlow, D. H., Rosenbaum, D. M., Rosemond, E., et al. (2012). Structure and dynamics of the M3 muscarinic acetylcholine receptor. Nature 482, 552–556. doi: 10.1038/nature10867
Kruse, A. C., Weiss, D. R., Rossi, M., Hu, J., Hu, K., Eitel, K., et al. (2013). Muscarinic receptors as model targets and antitargets for structure-based ligand discovery. Mol. Pharmacol. 84, 528–540. doi: 10.1124/mol.113.087551
Kufareva, I., Ilatovskiy, A. V., and Abagyan, R. (2012). Pocketome: an encyclopedia of small-molecule binding sites in 4D. Nucleic Acids Res. 40, D535–D540. doi: 10.1093/nar/gkr825
Lagorce, D., Sperandio, O., Galons, H., Miteva, M. A., and Villoutreix, B. O. (2008). FAF-Drugs2: free ADME/tox filtering tool to assist drug discovery and chemical biology projects. BMC Bioinformatics 9:396. doi: 10.1186/1471-2105-9-396
Lane, J. R., Chubukov, P., Liu, W., Canals, M., Cherezov, V., Abagyan, R., et al. (2013). Structure-based ligand discovery targeting orthosteric and allosteric pockets of dopamine receptors. Mol. Pharmacol. 84, 794–807. doi: 10.1124/mol.113.088054
Lane, J. R., May, L. T., Parton, R. G., Sexton, P. M., and Christopoulos, A. (2017). A kinetic view of GPCR allostery and biased agonism. Nat. Chem. Biol. 13, 929–937. doi: 10.1038/nchembio.2431
Langmead, C. J., Andrews, S. P., Congreve, M., Errey, J. C., Hurrell, E., Marshall, F. H., et al. (2012). Identification of novel adenosine A(2A) receptor antagonists by virtual screening. J. Med. Chem. 55, 1904–1909. doi: 10.1021/jm201455y
Lapinsh, M., Prusis, P., Gutcaits, A., Lundstedt, T., and Wikberg, J. E. (2001). Development of proteo-chemometrics: a novel technology for the analysis of drug-receptor interactions. Biochim. Biophys. Acta 1525, 180–190. doi: 10.1016/S0304-4165(00)00187-2
Launay, G., Téletchéa, S., Wade, F., Pajot-Augy, E., Gibrat, J. F., and Sanz, G. (2012). Automatic modeling of mammalian olfactory receptors and docking of odorants. Protein Eng. Des. Sel. 25, 377–386. doi: 10.1093/protein/gzs037
Lee, G. R., and Seok, C. (2016). Galaxy7TM: flexible GPCR-ligand docking by structure refinement. Nucleic Acids Res. 44, W502–W506. doi: 10.1093/nar/gkw360
Lee, J. H., Basith, S., Cui, M., Kim, B., and Choi, S. (2017). In silico prediction of multiple-category classification model for cytochrome P450 inhibitors and non-inhibitors using machine-learning method. SAR QSAR Environ. Res. 28, 863–874. doi: 10.1080/1062936X.2017.1399925
Lee, J. H., Lee, S., and Choi, S. (2010). in silico classification of adenosine receptor antagonists using Laplacian-modified naive Bayesian, support vector machine, and recursive partitioning. J. Mol. Graph. Model. 28, 883–890. doi: 10.1016/j.jmgm.2010.03.008
Lee, Y., Basith, S., and Choi, S. (2018). Recent advances in structure-based drug design targeting class A G protein-coupled receptors utilizing crystal structures and computational simulations. J. Med. Chem. 61, 1–46. doi: 10.1021/acs.jmedchem.6b01453
Le Guilloux, V., Schmidtke, P., and Tuffery, P. (2009). Fpocket: an open source platform for ligand pocket detection. BMC Bioinformatics 10:168. doi: 10.1186/1471-2105-10-168
Lemmen, C., Lengauer, T., and Klebe, G. (1998). FLEXS: a method for fast flexible ligand superposition. J. Med. Chem. 41, 4502–4520. doi: 10.1021/jm981037l
Lenselink, E. B., Beuming, T., Sherman, W., van Vlijmen, H. W., and IJzerman, A. P. (2014). Selecting an optimal number of binding site waters to improve virtual screening enrichments against the adenosine A2A receptor. J. Chem. Inf. Model. 54, 1737–1746. doi: 10.1021/ci5000455
Lenselink, E. B., Beuming, T., van Veen, C., Massink, A., Sherman, W., van Vlijmen, H. W., et al. (2016a). In search of novel ligands using a structure-based approach: a case study on the adenosine A2A receptor. J. Comput. Aided Mol. Des. 30, 863–874. doi: 10.1007/s10822-016-9963-7
Lenselink, E. B., Louvel, J., Forti, A. F., van Veldhoven, J. P. D., de Vries, H., Mulder-Krieger, T., et al. (2016b). Predicting binding affinities for GPCR ligands using free-energy perturbation. ACS Omega 1, 293–304. doi: 10.1021/acsomega.6b00086
Li, S., Lee, S. Y., and Chung, K. Y. (2015). Conformational analysis of g protein-coupled receptor signaling by hydrogen/deuterium exchange mass spectrometry. Methods Enzymol. 557, 261–278. doi: 10.1016/bs.mie.2014.12.004
Liang, Y., Fotiadis, D., Filipek, S., Saperstein, D. A., Palczewski, K., and Engel, A. (2003). Organization of the G protein-coupled receptors rhodopsin and opsin in native membranes. J. Biol. Chem. 278, 21655–21662. doi: 10.1074/jbc.M302536200
Liang, Y. L., Khoshouei, M., Radjainia, M., Zhang, Y., Glukhova, A., Tarrasch, J., et al. (2017). Phase-plate cryo-EM structure of a class B GPCR-G-protein complex. Nature 546, 118–123. doi: 10.1038/nature22327
Lionta, E., Spyrou, G., Vassilatis, D. K., and Cournia, Z. (2014). Structure-based virtual screening for drug discovery: principles, applications and recent advances. Curr. Top. Med. Chem. 14, 1923–1938. doi: 10.2174/1568026614666140929124445
Lipinski, C., and Hopkins, A. (2004). Navigating chemical space for biology and medicine. Nature 432, 855–861. doi: 10.1038/nature03193
Luo, M., Wang, X. S., Roth, B. L., Golbraikh, A., and Tropsha, A. (2014). Application of quantitative structure-activity relationship models of 5-HT1A receptor binding to virtual screening identifies novel and potent 5-HT1A ligands. J. Chem. Inf. Model. 54, 634–647. doi: 10.1021/ci400460q
Luo, M., Wang, X. S., and Tropsha, A. (2016). Comparative analysis of QSAR-based vs. chemical similarity based predictors of GPCRs binding affinity. Mol. Inform. 35, 36–41. doi: 10.1002/minf.201500038
Lyne, P. D. (2002). Structure-based virtual screening: an overview. Drug Discov. Today 7, 1047–1055. doi: 10.1016/S1359-6446(02)02483-2
Manglik, A., and Kruse, A. C. (2017). Structural basis for G protein-coupled receptor activation. Biochemistry 56, 5628–5634. doi: 10.1021/acs.biochem.7b00747
Manglik, A., Lin, H., Aryal, D. K., McCorvy, J. D., Dengler, D., Corder, G., et al. (2016). Structure-based discovery of opioid analgesics with reduced side effects. Nature 537, 185–190. doi: 10.1038/nature19112
Mansouri, K., and Judson, R. S. (2016). in silico study of in vitro GPCR assays by QSAR modeling. Methods Mol. Biol. 1425, 361–381. doi: 10.1007/978-1-4939-3609-0_16
Matricon, P., Ranganathan, A., Warnick, E., Gao, Z. G., Rudling, A., Lambertucci, C., et al. (2017). Fragment optimization for GPCRs by molecular dynamics free energy calculations: probing druggable subpockets of the A 2A adenosine receptor binding site. Sci. Rep. 7:6398. doi: 10.1038/s41598-017-04905-0
McGann, M. (2012). FRED and HYBRID docking performance on standardized datasets. J. Comput. Aided Mol. Des. 26, 897–906. doi: 10.1007/s10822-012-9584-8
McRobb, F. M., Negri, A., Beuming, T., and Sherman, W. (2016). Molecular dynamics techniques for modeling G protein-coupled receptors. Curr. Opin. Pharmacol. 30, 69–75. doi: 10.1016/j.coph.2016.07.001
Miao, Y., Goldfeld, D. A., Moo, E. V., Sexton, P. M., Christopoulos, A., McCammon, J. A., et al. (2016). Accelerated structure-based design of chemically diverse allosteric modulators of a muscarinic G protein-coupled receptor. Proc. Natl. Acad. Sci. U.S.A. 113, E5675–E5684. doi: 10.1073/pnas.1612353113
Mitchell, J. B. (2014). Machine learning methods in chemoinformatics. Wiley Interdiscip. Rev. Comput. Mol. Sci. 4, 468–481. doi: 10.1002/wcms.1183
Morris, G. M., Huey, R., Lindstrom, W., Sanner, M. F., Belew, R. K., Goodsell, D. S., et al. (2009). AutoDock4 and AutoDockTools4: automated docking with selective receptor flexibility. J. Comput. Chem. 30, 2785–2791. doi: 10.1002/jcc.21256
Munk, C., Harpsøe, K., Hauser, A. S., Isberg, V., and Gloriam, D. E. (2016). Integrating structural and mutagenesis data to elucidate GPCR ligand binding. Curr. Opin. Pharmacol. 30, 51–58. doi: 10.1016/j.coph.2016.07.003
Murray, C. W., Verdonk, M. L., and Rees, D. C. (2012). Experiences in fragment-based drug discovery. Trends Pharmacol. Sci. 33, 224–232. doi: 10.1016/j.tips.2012.02.006
Neustadt, B. R., Hao, J., Lindo, N., Greenlee, W. J., Stamford, A. W., Tulshian, D., et al. (2007). Potent, selective, and orally active adenosine A2A receptor antagonists: arylpiperazine derivatives of pyrazolo[4,3-e]-1,2,4-triazolo[1,5-c]pyrimidines. Bioorg. Med. Chem. Lett. 17, 1376–1380. doi: 10.1016/j.bmcl.2006.11.083
Neves, M. A., Totrov, M., and Abagyan, R. (2012). Docking and scoring with ICM: the benchmarking results and strategies for improvement. J. Comput. Aided Mol. Des. 26, 675–686. doi: 10.1007/s10822-012-9547-0
Ngo, T., Kufareva, I., Coleman, J. L. J., Graham, R. M., Abagyan, R., and Smith, N. J. (2016). Identifying ligands at orphan GPCRs: current status using structure-based approaches. Br. J. Pharmacol. 173, 2934–2951. doi: 10.1111/bph.13452
Okuno, Y., Tamon, A., Yabuuchi, H., Niijima, S., Minowa, Y., Tonomura, K., et al. (2008). GLIDA: GPCR–ligand database for chemical genomics drug discovery–database and tools update. Nucleic Acids Res. 36, D907–D912. doi: 10.1093/nar/gkm948
Olah, M., Mracec, M., Ostopovici, L., Rad, R., Bora, A., Hadaruga, N., et al. (2005). “9. WOMBAT: world of molecular bioactivity,” in Chemoinformatics in Drug Discovery, ed T. I. Oprea (Weinheim: WILEY-VCH Verlag GmbH & Co.), 221–239.
Oprea, T. I., and Gottfries, J. (2001). Chemography: the art of navigating in chemical space. J. Comb. Chem. 3, 157–166. doi: 10.1021/cc0000388
Oswald, C., Rappas, M., Kean, J., Doré, A. S., Errey, J. C., Bennett, K., et al. (2016). Intracellular allosteric antagonism of the CCR9 receptor. Nature 540, 462–465. doi: 10.1038/nature20606
Palczewski, K., Kumasaka, T., Hori, T., Behnke, C. A., Motoshima, H., Fox, B. A., et al. (2000). Crystal structure of rhodopsin: a G protein-coupled receptor. Science 289, 739–745. doi: 10.1126/science.289.5480.739
Paul, D. S., and Gautham, N. (2016). MOLS 2.0: software package for peptide modeling and protein-ligand docking. J. Mol. Model. 22, 239. doi: 10.1007/s00894-016-3106-x
Qiu, T., Qiu, J., Feng, J., Wu, D., Yang, Y., Tang, K., et al. (2017). The recent progress in proteochemometric modelling: focusing on target descriptors, cross-term descriptors and application scope. Brief. Bioinform. 18, 125–136. doi: 10.1093/bib/bbw004
Rasmussen, S. G., Choi, H. J., Rosenbaum, D. M., Kobilka, T. S., Thian, F. S., Edwards, P. C., et al. (2007). Crystal structure of the human beta2 adrenergic G-protein-coupled receptor. Nature 450, 383–387. doi: 10.1038/nature06325
Rasmussen, S. G., DeVree, B. T., Zou, Y., Kruse, A. C., Chung, K. Y., Kobilka, T. S., et al. (2011). Crystal structure of the beta2 adrenergic receptor-Gs protein complex. Nature 477, 549–555. doi: 10.1038/nature10361
Renault, N., Laurent, X., Farce, A., El Bakali, J., Mansouri, R., Gervois, P., et al. (2013). Virtual screening of CB(2) receptor agonists from bayesian network and high-throughput docking: structural insights into agonist-modulated GPCR features. Chem. Biol. Drug Des. 81, 442–454. doi: 10.1111/cbdd.12095
Reutlinger, M., Rodrigues, T., Schneider, P., and Schneider, G. (2014). Multi-objective molecular de novo design by adaptive fragment prioritization. Angew. Chem. Int. Ed. Engl. 53, 4244–4248. doi: 10.1002/anie.201310864
Reymond, J. L. (2015). The chemical space project. Acc. Chem. Res. 48, 722–730. doi: 10.1021/ar500432k
Ring, A. M., Manglik, A., Kruse, A. C., Enos, M. D., Weis, W. I., Garcia, K. C., et al. (2013). Adrenaline-activated structure of β(2)-adrenoceptor stabilized by an engineered nanobody. Nature 502, 575–579. doi: 10.1038/nature12572
Rodrigues, T., Hauser, N., Reker, D., Reutlinger, M., Wunderlin, T., Hamon, J., et al. (2015). Multidimensional de novo design reveals 5-HT2B receptor-selective ligands. Angew. Chem. Int. Ed. Engl. 54, 1551–1555. doi: 10.1002/anie.201410201
Rodríguez, D., Chakraborty, S., Warnick, E., Crane, S., Gao, Z. G., O'Connor, R., et al. (2016). Structure-based screening of uncharted chemical space for atypical adenosine receptor agonists. ACS Chem. Biol. 11, 2763–2772. doi: 10.1021/acschembio.6b00357
Rodriguez, D., Gao, Z. G., Moss, S. M., Jacobson, K. A., and Carlsson, J. (2015). Molecular docking screening using agonist-bound GPCR structures: probing the A(2A) adenosine receptor. J. Chem. Inf. Model. 55, 550–563. doi: 10.1021/ci500639g
Roy, U., and Luck, L. A. (2007). Molecular modeling of estrogen receptor using molecular operating environment. Biochem. Mol. Biol. Educ. 35, 238–243. doi: 10.1002/bmb.65
Sabio, M., Jones, K., and Topiol, S. (2008). Use of the X-ray structure of the beta2-adrenergic receptor for drug discovery. Part 2: identification of active compounds. Bioorg. Med. Chem. Lett. 18, 5391–5395. doi: 10.1016/j.bmcl.2008.09.046
Sandal, M., Duy, T. P., Cona, M., Zung, H., Carloni, P., Musiani, F., et al. (2013). GOMoDo: a GPCRs online modeling and docking webserver. PLoS ONE 8:e74092. doi: 10.1371/journal.pone.0074092
Sanders, M. P., Verhoeven, S., de Graaf, C., Roumen, L., Vroling, B., Nabuurs, S. B., et al. (2011). Snooker: a structure-based pharmacophore generation tool applied to class A GPCRs. J. Chem. Inf. Model. 51, 2277–2292. doi: 10.1021/ci200088d
Saxena, A. K., Bhunia, S. S., and Saxena, M. (2017). “Integration on ligand and structure based approaches in GPCRs,” in Topics in Medicinal Chemistry, eds P. R. Bernstein, G. I. Georg, T. Keller, T. Kobayashi, J. A. Lowe, N. A. Meanwell, A. K. Saxena, U. Stilz, C. T. Supuran, and A. Zhang (Berlin; Heidelberg: Springer), 1–61.
Scheerer, P., Park, J. H., Hildebrand, P. W., Kim, Y. J., Krauss, N., Choe, H. W., et al. (2008). Crystal structure of opsin in its G-protein-interacting conformation. Nature 455, 497–502. doi: 10.1038/nature07330
Schertler, G. F., Villa, C., and Henderson, R. (1993). Projection structure of rhodopsin. Nature 362, 770–772. doi: 10.1038/362770a0
Schneidman-Duhovny, D., Dror, O., Inbar, Y., Nussinov, R., and Wolfson, H. J. (2008). Deterministic pharmacophore detection via multiple flexible alignment of drug-like molecules. J. Comput. Biol. 15, 737–754. doi: 10.1089/cmb.2007.0130
Schrage, R., and Kostenis, E. (2017). Functional selectivity and dualsteric/bitopic GPCR targeting. Curr. Opin. Pharmacol. 32, 85–90. doi: 10.1016/j.coph.2016.12.001
Schuffenhauer, A., Brown, N., Selzer, P., Ertl, P., and Jacoby, E. (2006). Relationships between molecular complexity, biological activity, and structural diversity. J. Chem. Inf. Model. 46, 525–535. doi: 10.1021/ci0503558
Selvam, C., Oueslati, N., Lemasson, I. A., Brabet, I., Rigault, D., Courtiol, T., et al. (2010). A virtual screening hit reveals new possibilities for developing group III metabotropic glutamate receptor agonists. J. Med. Chem. 53, 2797–2813. doi: 10.1021/jm901523t
Shimamura, T., Shiroishi, M., Weyand, S., Tsujimoto, H., Winter, G., Katritch, V., et al. (2011). Structure of the human histamine H1 receptor complex with doxepin. Nature 475, 65–70. doi: 10.1038/nature10236
Shiraishi, A., Niijima, S., Brown, J. B., Nakatsui, M., and Okuno, Y. (2013). Chemical genomics approach for GPCR-ligand interaction prediction and extraction of ligand binding determinants. J. Chem. Inf. Model. 53, 1253–1262. doi: 10.1021/ci300515z
Shoichet, B. K., and Kobilka, B. K. (2012). Structure-based drug screening for G-protein-coupled receptors. Trends Pharmacol. Sci. 33, 268–272. doi: 10.1016/j.tips.2012.03.007
Shonberg, J., Kling, R. C., Gmeiner, P., and Löber, S. (2015). GPCR crystal structures: medicinal chemistry in the pocket. Bioorg. Med. Chem. 23, 3880–3906. doi: 10.1016/j.bmc.2014.12.034
Shukla, A. K., Westfield, G. H., Xiao, K. H., Reis, R. I., Huang, L. Y., Tripathi-Shukla, P., et al. (2014). Visualization of arrestin recruitment by a G-protein-coupled receptor. Nature 512, 218–222. doi: 10.1038/nature13430
Spitzer, R., and Jain, A. N. (2012). Surflex-dock: docking benchmarks and real-world application. J. Comput. Aided Mol. Des. 26, 687–699. doi: 10.1007/s10822-011-9533-y
Sriram, K., and Insel, P. A. (2018). GPCRs as targets for approved drugs: how many targets and how many drugs? Mol. Pharmacol. 93, 251–258. doi: 10.1124/mol.117.111062
Tautermann, C. S. (2016). Impact, determination and prediction of drug-receptor residence times for GPCRs. Curr. Opin. Pharmacol. 30, 22–26. doi: 10.1016/j.coph.2016.07.004
Thomsen, W., Frazer, J., and Unett, D. (2005). Functional assays for screening GPCR targets. Curr. Opin. Biotechnol. 16, 655–665. doi: 10.1016/j.copbio.2005.10.008
Tiikkainen, P., Bellis, L., Light, Y., and Franke, L. (2013). Estimating error rates in bioactivity databases. J. Chem. Inf. Model. 53, 2499–2505. doi: 10.1021/ci400099q
Trauelsen, M., Rexen Ulven, E., Hjorth, S. A., Brvar, M., Monaco, C., Frimurer, T. M., et al. (2017). Receptor structure-based discovery of non-metabolite agonists for the succinate receptor GPR91. Mol. Metab. 6, 1585–1596. doi: 10.1016/j.molmet.2017.09.005
Trott, O., and Olson, A. J. (2010). AutoDock Vina: improving the speed and accuracy of docking with a new scoring function, efficient optimization, and multithreading. J. Comput. Chem. 31, 455–461. doi: 10.1002/jcc.21334
Tsai, T. Y., Chang, K. W., and Chen, C. Y. (2011). iScreen: world's first cloud-computing web server for virtual screening and de novo drug design based on TCM database@Taiwan. J. Comput. Aided Mol. Des. 25, 525–531. doi: 10.1007/s10822-011-9438-9
Valerio, L. G. Jr., and Choudhuri, S. (2012). Chemoinformatics and chemical genomics: potential utility of in silico methods. J. Appl. Toxicol. 32, 880–889. doi: 10.1002/jat.2804
van Westen, G. J. P., Wegner, J. K., Geluykens, P., Kwanten, L., Vereycken, I., Peeters, A., et al. (2011). Which compound to select in lead optimization? Prospectively validated proteochemometric models guide preclinical development. PLoS ONE 6:e27518. doi: 10.1371/journal.pone.0027518
Varghese, J. N. (1999). Development of neuraminidase inhibitors as anti-influenza virus drugs. Drug Dev. Res. 46, 176–196. doi: 10.1002/(SICI)1098-2299(199903/04)46:3/4<176::AID-DDR4>3.0.CO;2-6
Varnek, A., and Baskin, I. (2012). Machine learning methods for property prediction in chemoinformatics: Quo Vadis? J. Chem. Inf. Model. 52, 1413–1437. doi: 10.1021/ci200409x
Varnek, A., and Tropsha, A. (2008). Chemoinformatics Approaches to Virtual Screening. Cambridge: RSC Publishing.
Vass, M., Agai-Csongor, E., Horti, F., and Keseru, G. M. (2014a). Multiple fragment docking and linking in primary and secondary pockets of dopamine receptors. ACS Med. Chem. Lett. 5, 1010–1014. doi: 10.1021/ml500201u
Vass, M., Kooistra, A. J., Ritschel, T., Leurs, R., de Esch, I. J., and de Graaf, C. (2016). Molecular interaction fingerprint approaches for GPCR drug discovery. Curr. Opin. Pharmacol. 30, 59–68. doi: 10.1016/j.coph.2016.07.007
Vass, M., Schmidt, É., Horti, F., and Keseru, G. M. (2014b). Virtual fragment screening on GPCRs: a case study on dopamine D3 and histamine H4 receptors. Eur. J. Med. Chem. 77, 38–46. doi: 10.1016/j.ejmech.2014.02.034
Venkatakrishnan, A. J., Deupi, X., Lebon, G., Tate, C. G., Schertler, G. F., and Babu, M. M. (2013). Molecular signatures of G-protein-coupled receptors. Nature 494, 185–194. doi: 10.1038/nature11896
Verheij, M. H., de Graaf, C., de Kloe, G. E., Nijmeijer, S., Vischer, H. F., Smits, R. A., et al. (2011). Fragment library screening reveals remarkable similarities between the G protein-coupled receptor histamine H(4) and the ion channel serotonin 5-HT(3)A. Bioorg. Med. Chem. Lett. 21, 5460–5464. doi: 10.1016/j.bmcl.2011.06.123
Vilardaga, J. P. (2011). Studying ligand efficacy at G protein-coupled receptors using FRET. Methods Mol. Biol. 756, 133–148. doi: 10.1007/978-1-61779-160-4_6
Visegrády, A., and Keseru, G. M. (2013). Fragment-based lead discovery on G-protein-coupled receptors. Expert Opin. Drug. Discov. 8, 811–820. doi: 10.1517/17460441.2013.794135
Wacker, D., Fenalti, G., Brown, M. A., Katritch, V., Abagyan, R., Cherezov, V., et al. (2010). Conserved binding mode of human β(2) adrenergic receptor inverse agonists and antagonist revealed by X-ray crystallography. J. Am. Chem. Soc. 132, 11443–11445. doi: 10.1021/ja105108q
Wacker, D., Stevens, R. C., and Roth, B. L. (2017a). How ligands illuminate GPCR molecular pharmacology. Cell 170, 414–427. doi: 10.1016/j.cell.2017.07.009
Wacker, D., Wang, S., McCorvy, J. D., Betz, R. M., Venkatakrishnan, A. J., Levit, A., et al. (2017b). Crystal structure of an LSD-bound human serotonin receptor. Cell 168, 377 e312–389 e312. doi: 10.1016/j.cell.2016.12.033.
Walters, W. P., and Namchuk, M. (2003). Designing screens: how to make your hits a hit. Nat. Rev. Drug Discov. 2, 259–266. doi: 10.1038/nrd1063
Wang, C., Wu, H., Katritch, V., Han, G. W., Huang, X. P., Liu, W., et al. (2013). Structure of the human smoothened receptor bound to an antitumour agent. Nature 497, 338–343. doi: 10.1038/nature12167
Weiss, D. R., Ahn, S., Sassano, M. F., Kleist, A., Zhu, X., Strachan, R., et al. (2013). Conformation guides molecular efficacy in docking screens of activated beta-2 adrenergic G protein coupled receptor. ACS Chem. Biol. 8, 1018–1026. doi: 10.1021/cb400103f
Williams, A. J., and Ekins, S. (2011). A quality alert and call for improved curation of public chemistry databases. Drug Discov. Today 16, 747–750. doi: 10.1016/j.drudis.2011.07.007
Williams, A. J., Ekins, S., and Tkachenko, V. (2012). Towards a gold standard: regarding quality in public domain chemistry databases and approaches to improving the situation. Drug Discov. Today 17, 685–701. doi: 10.1016/j.drudis.2012.02.013
Wlodawer, A., and Vondrasek, J. (1998). Inhibitors of HIV-1 protease: a major success of structure-assisted drug design. Annu. Rev. Biophys. Biomol. Struct. 27, 249–284. doi: 10.1146/annurev.biophys.27.1.249
Wolber, G., and Langer, T. (2005). LigandScout: 3-D pharmacophores derived from protein-bound ligands and their use as virtual screening filters. J. Chem. Inf. Model. 45, 160–169. doi: 10.1021/ci049885e
Wu, H., Wang, C., Gregory, K. J., Han, G. W., Cho, H. P., Xia, Y., et al. (2014). Structure of a class C GPCR metabotropic glutamate receptor 1 bound to an allosteric modulator. Science 344, 58–64. doi: 10.1126/science.1249489
Xie, X. Q., Wang, L., Liu, H., Ouyang, Q., Fang, C., and Su, W. (2014). Chemogenomics knowledgebased polypharmacology analyses of drug abuse related G-protein coupled receptors and their ligands. Front. Pharmacol. 5:3. doi: 10.3389/fphar.2014.00003
Xu, W., Lim, J., Goh, C. Y., Suen, J. Y., Jiang, Y., Yau, M. K., et al. (2015). Repurposing registered drugs as antagonists for protease-activated receptor 2. J. Chem. Inf. Model. 55, 2079–2084. doi: 10.1021/acs.jcim.5b00500
Yadav, D. K., Rai, P., Pratap, R., and Singh, H. (2016). “Software and web resources for computer-aided molecular modeling and drug discovery,” in Chemometrics Applications and Research: QSAR in Medicinal Chemistry, eds A. G. Mercader, P. R. Duchowicz, and P. M. Sivakumar (Oakville, ON: Apple Academic Press), 33–99.
Zhang, D., Gao, Z. G., Zhang, K., Kiselev, E., Crane, S., Wang, J., et al. (2015). Two disparate ligand-binding sites in the human P2Y1 receptor. Nature 520, 317–321. doi: 10.1038/nature14287
Zhang, G., Wang, K., Li, X. D., Zhang, D. L., and Xu, F. (2016). Discovery of novel antagonists of human neurotensin receptor 1 on the basis of ligand and protein structure. Biomed. Pharmacother. 84, 147–157. doi: 10.1016/j.biopha.2016.09.025
Zhang, H., Unal, H., Desnoyer, R., Han, G. W., Patel, N., Katritch, V., et al. (2015). Structural basis for ligand recognition and functional selectivity at angiotensin receptor. J. Biol. Chem. 290, 29127–29139. doi: 10.1074/jbc.M115.689000
Zhang, X. (2017). Seven perspectives on GPCR H/D-exchange proteomics methods. F1000Res 6:89. doi: 10.12688/f1000research.10667.1
Zhang, Y., Sun, B., Feng, D., Hu, H., Chu, M., Qu, Q., et al. (2017). Cryo-EM structure of the activated GLP-1 receptor in complex with a G protein. Nature 546, 248–253. doi: 10.1038/nature22394
Zhou, X. E., He, Y., de Waal, P. W., Gao, X., Kang, Y., Van Eps, N., et al. (2017). Identification of phosphorylation codes for arrestin recruitment by G protein-coupled receptors. Cell 170, 457 e413–469 doi: 10.1016/j.cell.2017.07.002
Keywords: GPCR, cheminformatics, drug discovery, ligand-based drug design, structure-based drug design
Citation: Basith S, Cui M, Macalino SJY, Park J, Clavio NAB, Kang S and Choi S (2018) Exploring G Protein-Coupled Receptors (GPCRs) Ligand Space via Cheminformatics Approaches: Impact on Rational Drug Design. Front. Pharmacol. 9:128. doi: 10.3389/fphar.2018.00128
Received: 08 December 2017; Accepted: 06 February 2018;
Published: 09 March 2018.
Edited by:
Leonardo G. Ferreira, University of São Paulo, BrazilReviewed by:
Doriano Lamba, Consiglio Nazionale Delle Ricerche (CNR), ItalyDharmendra Kumar Yadav, Gachon University of Medicine and Science, South Korea
Ana Carolina Rennó Sodero, Universidade Federal do Rio de Janeiro, Brazil
Copyright © 2018 Basith, Cui, Macalino, Park, Clavio, Kang and Choi. This is an open-access article distributed under the terms of the Creative Commons Attribution License (CC BY). The use, distribution or reproduction in other forums is permitted, provided the original author(s) and the copyright owner are credited and that the original publication in this journal is cited, in accordance with accepted academic practice. No use, distribution or reproduction is permitted which does not comply with these terms.
*Correspondence: Soosung Kang, c3NrYW5nQGV3aGEuYWMua3I=
Sun Choi, c3VuY2hvaUBld2hhLmFjLmty
†These authors have contributed equally to this work.