- 1Medicinal Chemistry, Monash Institute of Pharmaceutical Sciences, Monash University, Melbourne, VIC, Australia
- 2Centre de Recherches sur les Macromolécules Végétales, Centre National de la Recherche Scientifique UPR5301, Université Grenoble Alpes, Grenoble, France
- 3Département de Pharmacochimie Moléculaire, Centre National de la Recherche Scientifique, UMR5063, Université Grenoble Alpes, Grenoble, France
- 4School of Science, RMIT University, Melbourne, VIC, Australia
- 5Department of Surgery Austin Health, University of Melbourne, Melbourne, VIC, Australia
- 6Department of Immunology, Central Clinical School, Monash University, Melbourne, VIC, Australia
- 7Burnet Institute, Melbourne, VIC, Australia
Burkholderia ambifaria is an opportunistic respiratory pathogen belonging to the Burkholderia cepacia complex, a collection of species responsible for the rapidly fatal cepacia syndrome in cystic fibrosis patients. A fucose-binding lectin identified in the B. ambifaria genome, BambL, is able to adhere to lung tissue, and may play a role in respiratory infection. X-ray crystallography has revealed the bound complex structures for four fucosylated human blood group epitopes (blood group B, H type 1, H type 2, and Lex determinants). The present study employed computational approaches, including docking and molecular dynamics (MD), to extend the structural analysis of BambL-oligosaccharide complexes to include four additional blood group saccharides (A, Lea, Leb, and Ley) and a library of blood-group-related carbohydrates. Carbohydrate recognition is dominated by interactions with fucose via a hydrogen-bonding network involving Arg15, Glu26, Ala38, and Trp79 and a stacking interaction with Trp74. Additional hydrogen bonds to non-fucose residues are formed with Asp30, Tyr35, Thr36, and Trp74. BambL recognition is dominated by interactions with fucose, but also features interactions with other parts of the ligands that may modulate specificity or affinity. The detailed computational characterization of the BambL carbohydrate-binding site provides guidelines for the future design of lectin inhibitors.
Introduction
Cystic fibrosis morbidity is mostly due to respiratory infection by opportunistic pathogens (Lyczak et al., 2002; O'Sullivan and Freedman, 2009; Ciofu et al., 2013; Caverly et al., 2015). Burkholderia cepacia is one of the most dangerous pathogens isolated from cystic fibrosis patients; 20% of infected individuals succumb to a rapidly fatal pneumonia termed “cepacia syndrome” (Zahariadis et al., 2003; Blackburn et al., 2004; Lynch, 2009). Isolated B. cepacia strains have been classified into a steadily increasing number of species, referred to collectively as the B. cepacia complex (currently consisting of 20 species Vandamme et al., 1997; De Smet et al., 2015; Martinucci et al., 2016). Most members of the complex are resistant to multiple clinically used antibiotics, making the search for new therapeutics more urgent (Zhou et al., 2007; Loutet and Valvano, 2011; Podnecky et al., 2015). Burkholderia ambifaria, a member of the B. cepacia complex, has been isolated from both clinical and environmental samples (Coenye et al., 2001). In addition to infecting human respiratory tissue, B. ambifaria can colonize plant rhizospheres, where it promotes growth and protects against invading fungi (Li et al., 2002; Lee et al., 2006; Parra-Cota et al., 2014).
Previously, a carbohydrate-binding protein (named “BambL”) was identified in the B. ambifaria genome; binding studies using human tissues suggest it may play a role in infection (Audfray et al., 2012). Opportunistic bacteria often adhere to tissues by binding to host carbohydrates using carbohydrate-recognizing proteins (lectins) displayed at the bacterial surface (Bavington and Page, 2005; Imberty and Varrot, 2008; Pieters, 2011; Audfray et al., 2013). Among the many carbohydrates present on human cells, fucose-bearing blood group determinants are often recognized by bacterial lectins (Lindén et al., 2008; Anstee, 2010; Holmner et al., 2010). In the cystic fibrosis respiratory epithelium, cell-surface carbohydrates, present on glycolipids, N-glycoproteins, and mucins, are more fucosylated than in healthy tissue (Rhim et al., 2001; Venkatakrishnan et al., 2015). This increased fucosylation may promote adhesion by fucose-recognizing pathogens (Stoykova and Scanlin, 2008; Audfray et al., 2013). Known cystic fibrosis pathogens Pseudomonas aeruginosa, Burkholderia cenocepacia and Aspergillus fumigatus, all have lectins that bind to fucosylated human blood group carbohydrates (Mitchell et al., 2002; Imberty et al., 2004; Sulak et al., 2010, 2011; Houser et al., 2013, 2015). Significantly, the P. aeruginosa lectins are strongly associated with respiratory tissue damage and bacterial load in a mouse model of lung injury, and treatment with monosaccharides, able to specifically inhibit lectin binding, reduces infection (Chemani et al., 2009). Similar effects have been reported in a human P. aeruginosa infection case study (von Bismarck et al., 2001) suggesting that interfering with lectin-carbohydrate interactions may offer a new frontier in anti-infective treatment (Sharon, 2006; Pera and Peters, 2014). Lectin inhibitor design begins with a thorough understanding of the role of each functional group in the natively recognized carbohydrate (Ernst and Magnani, 2009).
The crystallographic structure of BambL has been solved, revealing a six-bladed β-propeller fold formed by three separate protomers (Audfray et al., 2012). Each subunit contains a single carbohydrate-binding site; upon oligomerization, three additional binding sites are formed at the interfaces between protomers, for a total of six binding sites in the β-propeller fold. The intra- and inter-protomeric sites have similar architectures and (for most blood group carbohydrates) similar binding properties. For this reason, the present work addresses interactions within the intra-protomeric site only. Crystal structures of BambL have also been obtained bound to multiple fucosylated human blood group tetrasaccharides: H type 1, H type 2, B type 2, and Lex (PDB IDs: 3ZW2, 3ZZV, 3ZWE, and 3ZW1; Audfray et al., 2012; Topin et al., 2013; Figure 1). In each case, the carbohydrate is bound via a buried fucose residue, which participates in a network of hydrogen bonds within a tight fucose-binding pocket. Blood group carbohydrate binding specificity has also been determined by glycan array and affinity quantified by titration microcalorimetry: strongest affinity is for H type 2 tetrasaccharide (KD 7.5 μM) and Ley pentasaccharide (KD 11.1 μM; Audfray et al., 2012). This binding preference indicates that BambL is more selective for blood and tissue carbohydrate determinants containing the type 2 epitope Fucα1-2Galβ1-4GlcNAc. Several of the blood group and tissue antigens recognized by BambL have not been structurally characterized in complex with the lectin (e.g., Ley, Leb, and A). Additionally, while existing crystal structures describe static recognition, the dynamic behavior of BambL complexes has not been described. The relative contributions of individual binding interactions to saccharide recognition is also unknown. Extending the structural analysis of BambL-blood group complexes to probe these aspects of recognition will enhance understanding of carbohydrate recognition and facilitate inhibitor design.
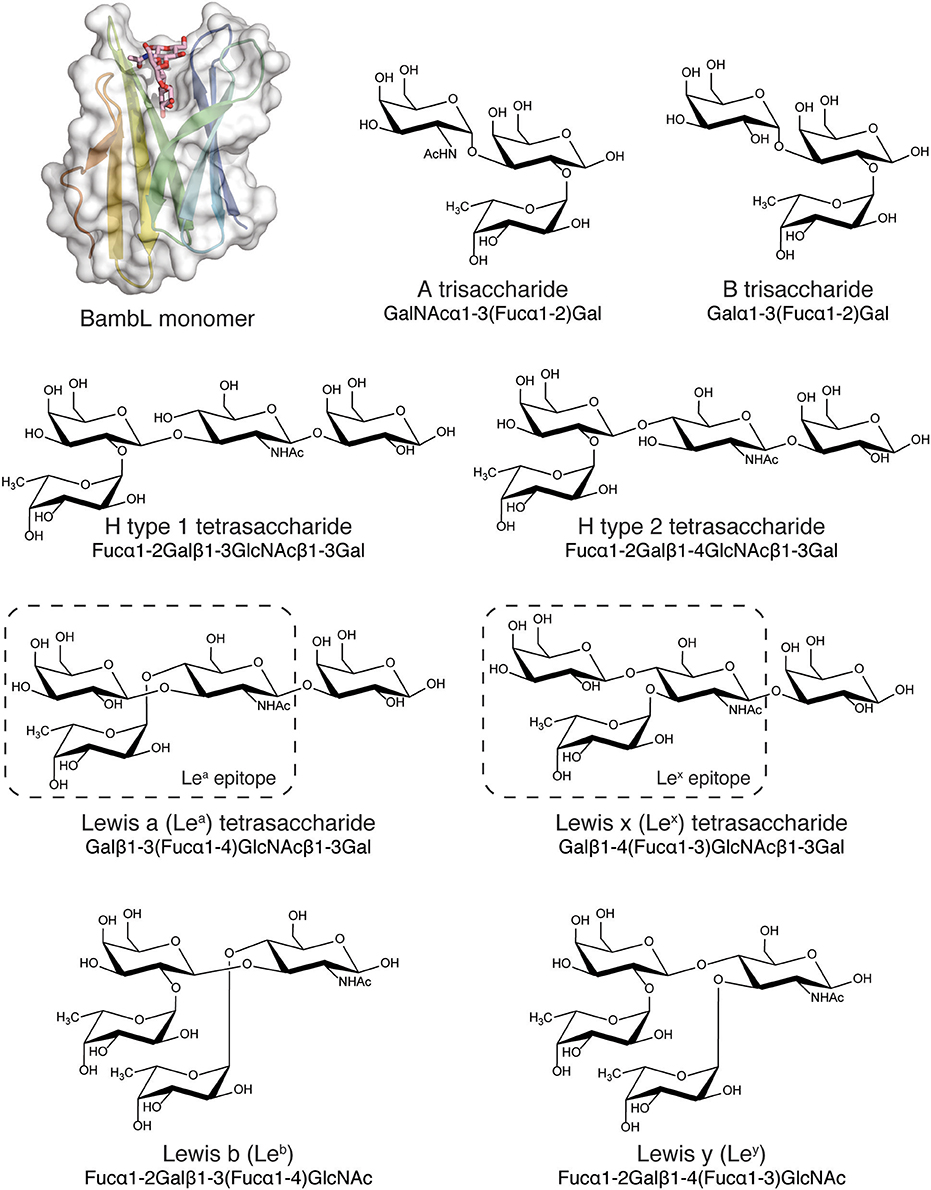
Figure 1. BambL subunit shown with blood group and tissue antigen saccharides (A, B, H, Lea, Leb, Lex, and Ley) used for simulation. BambL structure from PDB ID: 3ZZV, with the intra-protomeric binding site and ligand shown.
The goal of this computational study was to characterize BambL-saccharide binding modes and to inform future in silico or structure-based design of inhibitors for this bacterial lectin. We were interested in identifying lectin residues that are critical for ligand recognition and thus could be used as constraints in prospective virtual screening. In particular, we investigated whether the BambL binding site is restricted to recognizing fucose or is capable of engaging non-fucose saccharides using additional interactions. We first used docking and site mapping to study binding modes in complexes featuring A, B, O (H), and Lewis fucosylated carbohydrates and a library of blood-group-related saccharides. The dynamic behavior of these systems was then explored by molecular dynamics (MD) simulations. The recognition of fucose-containing saccharides by BambL is accomplished by a hydrogen-bonding network between fucose and Arg15, Glu26, Trp79, and to a lesser extent Ala38. A hydrophobic contact is made between the fucose non-polar face and the Trp79 imidazole. Additional hydrogen bonds outside the fucose-binding pocket to Asp30, Thr36, Trp74, and Tyr35 are formed in complex with multiple blood group and blood-group-related saccharides. Residues involved in these interactions are consistently engaged by blood-group-related saccharides, suggesting they may be valuable interaction targets for BambL inhibitors.
Materials and Methods
A single BambL subunit containing an intra-protomeric (Audfray et al., 2012) binding site was used in the below computational studies.
Blood-Group and Blood-Group-Related Carbohydrate Structure Generation
Low energy blood-group and blood-group-related carbohydrate structures were generated and simulation parameters produced using the GLYCAM web portal (Woods, 2005; Kirschner et al., 2008). The A and B determinants were modeled as trisaccharides for comparison to previous binding data for the soluble type A determinant (Audfray et al., 2012). The H type 1, H type 2, Lea and Lex determinants were modeled as tetrasaccharides for consistency to previously determined binding data (Audfray et al., 2012) and the Leb and Ley determinants were modeled as tetrasaccharides to encompass the entire epitope. The library of blood-group-related structures is shown in Supplementary Figure 1.
Docking
Docking experiments were performed using the docking program Glide 6.8 (Friesner et al., 2004, 2006; Halgren et al., 2004; Schrödinger, 2014a) available within the molecular modeling package Maestro (Schrödinger, 2014a,b). The BambL crystallographic complexes were downloaded from the Protein Data Bank, PDB (Berman et al., 2000), and the protein structures prepared using the Protein Preparation Wizard tool (Madhavi Sastry et al., 2013; Schrödinger, 2014b). During this step, structural details required for the docking calculation were specified. Double bond orders were applied for backbone carbonyl and aromatic side chain moieties, hydrogen atoms were added to the structure, water molecules removed, and disulfide bonds created between cysteine side chain sulfur atoms in close proximity. Missing atoms and side chains were added based on the protein's primary sequence using the Prime tool (Schrödinger, 2014c). To remove steric clashes between added hydrogen atoms, a minimization step was then conducted on hydrogen atoms only, using the OPLS2005 forcefield (Banks et al., 2005). A receptor grid was generated using default settings, with the binding site box centered on the crystallographic ligand. Ligands were docked into the receptor grid using Standard Precision mode with default settings. All carbohydrate atoms were treated flexibly during docking, including all glycosidic linkages and exocyclic groups. The lowest-energy docked poses were retained for MD simulation. Docked poses were filtered by glycosidic dihedral angle to exclude unfavorable high energy carbohydrate conformations. Cutoff values for dihedral filtering were chosen for each glycosidic linkage based on isoenergy contours previously calculated with the MM3 force field from Imberty et al. (1995). Conformations with dihedrals in the following ranges were removed from the analysis: Fucα1-2Gal φ < −130° & 180° < ψ <360°; GalNAcα1-3Gal φ > 240°; Galβ1-3GlcNAc φ > 0° & 180° < ψ < −60°. Thus, we have used energy maps to post-filter docked poses as a means of retaining reasonable conformations. These energy maps have been commonly used to evaluate carbohydrate conformations obtained from simulations and experimental work [for example Jackson et al. (2014) and Tempel et al. (2002)]. Hydrogen bonds and contacts were tallied using MDAnalysis (Michaud-Agrawal et al., 2011; distance = 3.0 Å, angle = 120).
Site Mapping
All BambL-blood group carbohydrate complexes were examined using LigPlot (Wallace et al., 1995; Laskowski and Swindells, 2011). Only poses that passed the glycosidic torsion filter requirements (see above), were used for site mapping, following a previously developed method (Yuriev et al., 2001; Agostino et al., 2009b, 2011, 2013; Dingjan et al., 2015a). In brief, each individual hydrogen bond made by a particular BambL residue was counted toward the hydrogen-bond tally. Non-polar vdW interactions between a specific BambL residue and a carbohydrate residue were counted as a single interaction toward the tally. The tallies were normalized to percentages of the total number of hydrogen bond or vdW interactions. Site maps were generated using residue inclusion cutoff values for lectin-carbohydrate complexes of 90% for hydrogen bonds, 0% for vdW interactions (Agostino et al., 2013). Site map images were rendered using PyMOL (Schrödinger, 2014d).
Molecular Dynamics
MD simulations were performed using Gromacs 5.0.4 (Berendsen et al., 1995; Van Der Spoel et al., 2005; Hess et al., 2008; Pronk et al., 2013). Proteins were parameterized using the AMBER99SB-ILDN (Lindorff-Larsen et al., 2010) forcefield. Carbohydrate topologies were generated using the GLYCAM06 (Kirschner et al., 2008) force field via the glycam.org web portal. The resulting AMBER-formatted topology was converted to GROMACS format using the “acpype” tool (Sousa da Silva and Vranken, 2012). The correctly formatted carbohydrate topology was then combined with the protein topology to describe the entire protein-carbohydrate system. Protein-carbohydrate docked complexes were placed in a rhombic dodecahedral box with a 10 Å minimum distance between solute and box wall, and subsequently solvated using the TIP3P water model. To maintain electrostatic neutrality, Na+ and Cl− counterions were added by the genion module. To remove steric clashes between nearby atoms, the system contents were minimized using the steepest descent algorithm (maximum steps: 50,000). The positions and velocities of the solvent molecules and ions were then equilibrated at constant volume and temperature (NVT ensemble) using three restraint settings: with all protein heavy atoms restrained for 100 ps, then with only backbone atoms restrained for 100 ps (both at 10 K), followed by a 100 ps equilibration without restraints at 300 K. Finally, the pressure of the system was equilibrated for 300 ps without restraints at constant atmospheric pressure (NPT ensemble) at 310 K. During all equilibration steps, positional restraints were applied to protein residues using LINCS (Hess, 2007). The coordinates from the final equilibration step were used to begin production simulation, which was conducted for 400 ns.
For all MD simulations in the NPT ensemble, temperature was kept constant using the velocity rescaling thermostat coupled with a time constant of 0.1 ps. Pressure was held constant at 1 bar using the Parrinello-Rahman barometer, coupled with a time constant of 2 ps. Equations of motion were integrated using a leap-frog integrator with a 2 fs timestep. Long-range electrostatics were evaluated using the Particle Mesh Ewald method. Cutoff values for Coulomb and vdW interactions were set to 1.0 nm. Complexes with blood group carbohydrate ligands were simulated in triplicate, complexes with blood-group-related carbohydrate ligands were simulated in singlicate. Each replicate was commenced using randomized velocities, resulting in independent simulations with different initial velocities.
Analysis of MD Simulations
Hydrogen bonds in MD simulations were analyzed using the Baker-Hubbard method implemented in the MDTraj (McGibbon et al., 2015) software library. An occupancy value was assigned to each hydrogen bond by calculating the percentage of simulation frames in which the bond was present. Glycosidic dihedral angles were measured using MDTraj and compared to calculated isoenergy contours (see above). Carbohydrate ring conformations were analyzed using Best Four-Member Plane method from GLYCAM (Makeneni et al., 2014). CH-π interactions were represented by measuring a shortest distance from either of the fucose atoms C3, C4, C5, or C6 to atoms of the indole ring of Trp74. Atom labeling corresponds to the conventions of the PDB exchange dictionary (Berman et al., 2003).
Results
Generation of BambL-Blood Group Complexes by Docking
To decide which of the crystallographic BambL receptor structures to use in this study, we compared complex structures predicted by re-docking with respective crystallographic complexes. The results of these cognate and cross-docking experiments are shown in Table 1, Figure 2. The Lex tetrasaccharide was poorly docked (RMSD > 2 Å) into all BambL structures. However, all four lectin structures afforded approximately equal performance when used as a receptor for the other three carbohydrate ligands: overall RMSD values of 1.09–2.62 and 0.14–0.56 Å for the buried fucose (Fucα1-2Gal) were observed. The crystallographic BambL structure from the PDB ID: 3ZZV complex was used as the receptor structure for site mapping and MD with all carbohydrates shown in Figure 1.
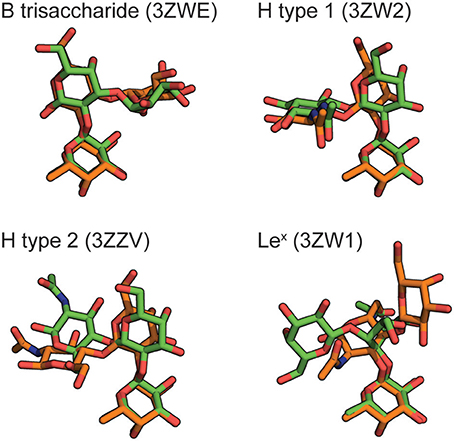
Figure 2. Blood group carbohydrates docked into the BambL binding site of PDB ID: 3ZZV (orange), compared to their respective experimentally determined poses (green). The PDB IDs for experimental poses are indicated. For clarity, all carbohydrates are shown as the non-reducing-end trisaccharide without hydrogen atoms.
In a second step, all blood group saccharides were docked in BambL (PDB ID: 3ZZV) and the top docked poses were analyzed for structural features relevant to recognition (Table 2). In all cases except Lex, the majority of binding interactions were made via a single buried fucose residue (Figure 3). The difucosylated Leb and Ley possess two fucose residues (Fucα1-2Gal and Fucα1-4GlcNAc in Leb or Fucα1-3GlcNAc in Ley) and therefore may occupy the fucose-binding pocket in two ways. Of the docked Leb poses produced here, only the Fucα1-2Gal residue was predicted in the binding pocket. As for the docked Ley poses, all of the top 20 ranked poses positioned the Fucα1-2Gal residue in the pocket, with the exception of poses at rank 5 and 6 that predicted the Fucα1-3GlcNAc residue in the fucose binding pocket.
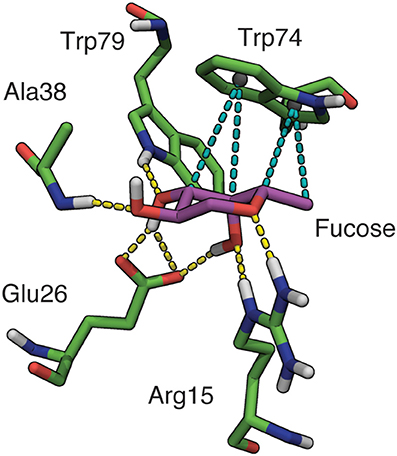
Figure 3. Binding site interactions involving fucose in BambL-blood group saccharide docked poses. Hydrogen bonds shown as yellow dashes, hydrophobic interactions shown as teal dashes. Non-polar hydrogens omitted for clarity.
As expected, recognition of the buried fucose (Fucα1-2Gal) was governed by a conserved hydrogen-bonding network and a single hydrophobic stacking interaction (Supplementary Table 1). Rather than interacting via a buried fucose, the Lex top docked pose was placed “back-to-front” with the reducing end galactose in the fucose-binding pocket, and the fucose directed away from the protein.
Apart from interactions with the buried fucose residue, additional hydrogen bonds are made between non-fucose residues and amino acids in the four β-turn loops surrounding the fucose-binding pocket (Table 2). The most frequently participating residue, Asp30, interacts with non-fucose portions of multiple saccharides (B, H1, Lea, Leb, and Ley). The imidazole side-chain of Trp74 (which stacks against the buried fucose) also donates a hydrogen bond to non-fucose residues in several cases. In each case, the hydrogen bond is accepted by atoms in a similar location: two residues away from the buried fucose, at the GlcNAc 6-position (Leb, Ley), Gal/GalNAc 3-position (A, B), or GlcNAc 2-position (H1). The presence of hydrogen bonds between non-fucose portions and loop residues suggests that BambL recognition may not rely solely on interactions with a single buried fucose.
Glycosidic dihedral angles in top docked poses lie close to global or secondary minima in previously calculated (Imberty et al., 1995) energy maps (see Supplementary Figures 2, 3). An exception is the Fucα1-2Gal linkage, which is positioned in between minima in the H type 1, H type 2, Leb and Ley top poses. In the A and B trisaccharide complexes, the Fucα1-2Gal linkage adopted the lowest energy conformation. These results agree with earlier BambL-blood group docking by Topin et al. (2013) in which top docked pose glycosidic linkages also occupied a range of energetic minima.
Site Mapping of BambL-Blood Group Complexes
Site mapping reveals binding site residues that are frequently involved in interactions throughout an ensemble of docked poses. Site maps for BambL-blood group complexes are shown in Figure 4. These maps are based on docking results for all carbohydrates shown in Figure 1. The BambL site maps agree with crystallographic complexes, identifying multiple residues in the fucose binding pocket known to interact with fucose in crystallographic structures (PDB IDs: 3ZW2, 3ZZV, 3ZWE, and 3ZW1; Audfray et al., 2012; Topin et al., 2013). Across the docked pose ensemble, hydrogen bonds were frequently formed to Arg15 (27.9%), Ala38 (11.6%), and Glu26 (13.7%), all located within the fucose-binding pocket. Surprisingly, Trp79 (4.9%), also in the crystallographic fucose pocket, was not often involved throughout the docked pose ensemble. van der Waals (vdW) interactions were frequently made with Trp74 (14.6%) in the fucose pocket, in close agreement with crystallographic bound complexes. Site maps also revealed new interactions not seen in crystal structures, identifying hydrogen bonding to Asp30 (7.1%) and vdW interaction with Tyr35 (11.1%) as regularly occurring across all docked poses.
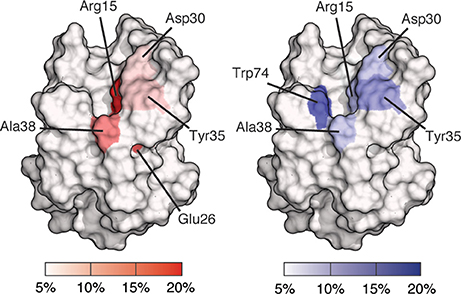
Figure 4. Site maps of a BambL subunit showing binding site residues involved in docked pose interactions. Residues involved in 5% or fewer interactions are colored white; residues involved in 20% or greater interactions are colored red (for hydrogen bonding) or blue (for van der Waals). Residues with intermediate involvement are shaded according to the color scale.
Molecular Dynamics Simulations of BambL-Blood Group Complexes
To investigate the dynamic behavior of BambL-blood group complexes, the lowest-energy poses generated by docking were simulated in explicit solvent. For difucosylated Leb and Ley, the lowest-energy poses with the Fucα1-2Gal residue in the fucose-binding pocket were used. The poorly docked Lex complex was also simulated, but quickly dissociated from the protein or was unstable in the binding site (see Supplementary Figure 4). To probe the dynamic behavior of the Lex binding interactions, the crystallographic complex was used instead (PDB ID: 3ZW1).
During MD simulations, all fucose-anchored blood group saccharides (A, B, H type 1, H type 2, Lea, Leb, Ley) remained bound to BambL without dissociation for the entire duration (400 ns). Structural fluctuations in ligand RMSD were below 2 Å in all bound complexes, reflecting relatively small changes in ligand positions and geometries during the MD simulations (see Supplementary Figure 5). Carbohydrate ring conformations were found to generally adopt one of the two chair conformations (1C4 or 4C1), while the GlcNAc rings in the H type 2, Lea, and Lex exhibited some variation (see Supplementary Figure 6). A similar hydrogen-bonding pattern was observed across all blood group simulations (Figures 5, 6), featuring interactions between the buried fucose residue and the fucose-binding pocket: Glu26 acidic group to O3 and O4 hydroxyl protons, Arg15 guanidinium to O4 and O5 oxygen atoms, and Trp79 indole to O3 oxygen atom. These hydrogen bonds were highly occupied (between 60 and 90% of simulation frames), with the exception of the Glu26 hydrogen bonds in the Leb complex (50–60%). The high occupancy of these hydrogen bonds indicates the dominant role played by fucose in BambL-carbohydrate binding.
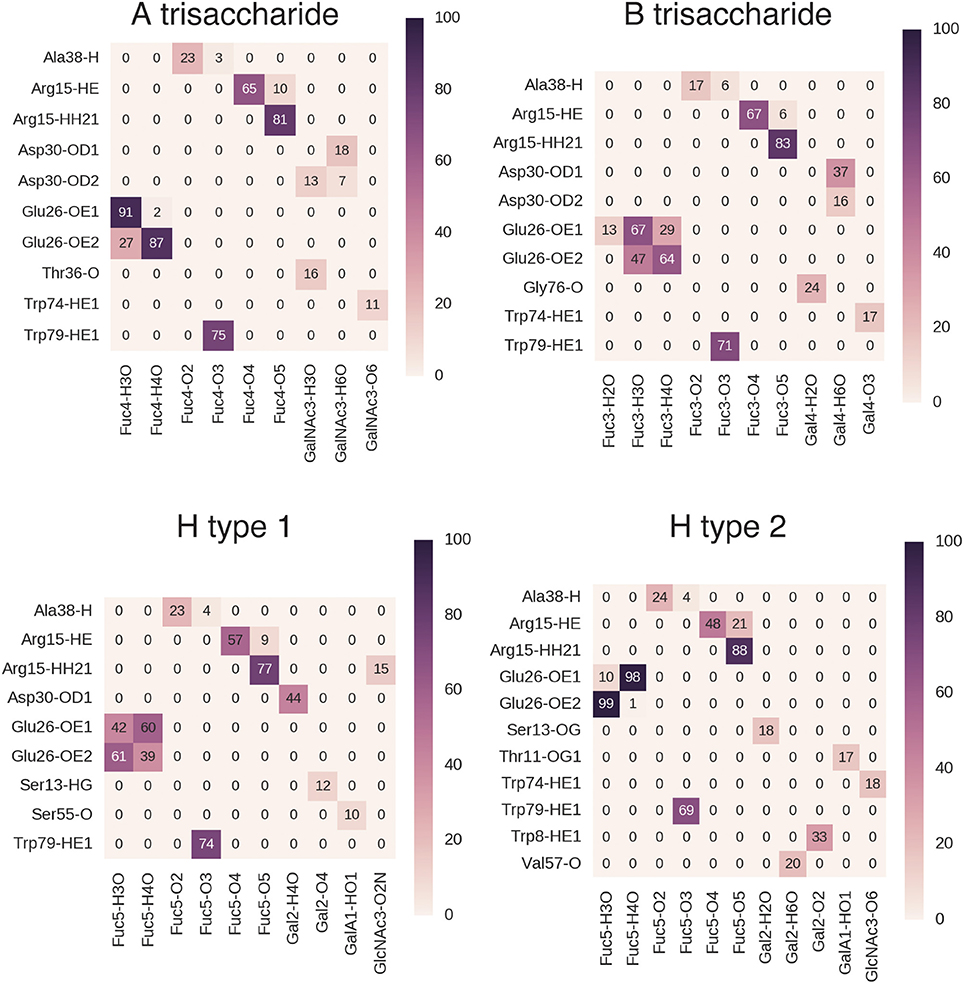
Figure 5. Hydrogen bond interactions in BambL-saccharide complexes during MD simulations shown for A trisaccharide, B trisaccharide, H type 1 and H type 2 blood groups. Atoms are named to the conventions of the PDB exchange dictionary. Grid cells are colored and labeled by average occupancy from three replicate simulations. Occupancy values were calculated by dividing the number of frames in which the hydrogen bond exists by the total number of simulation frames.
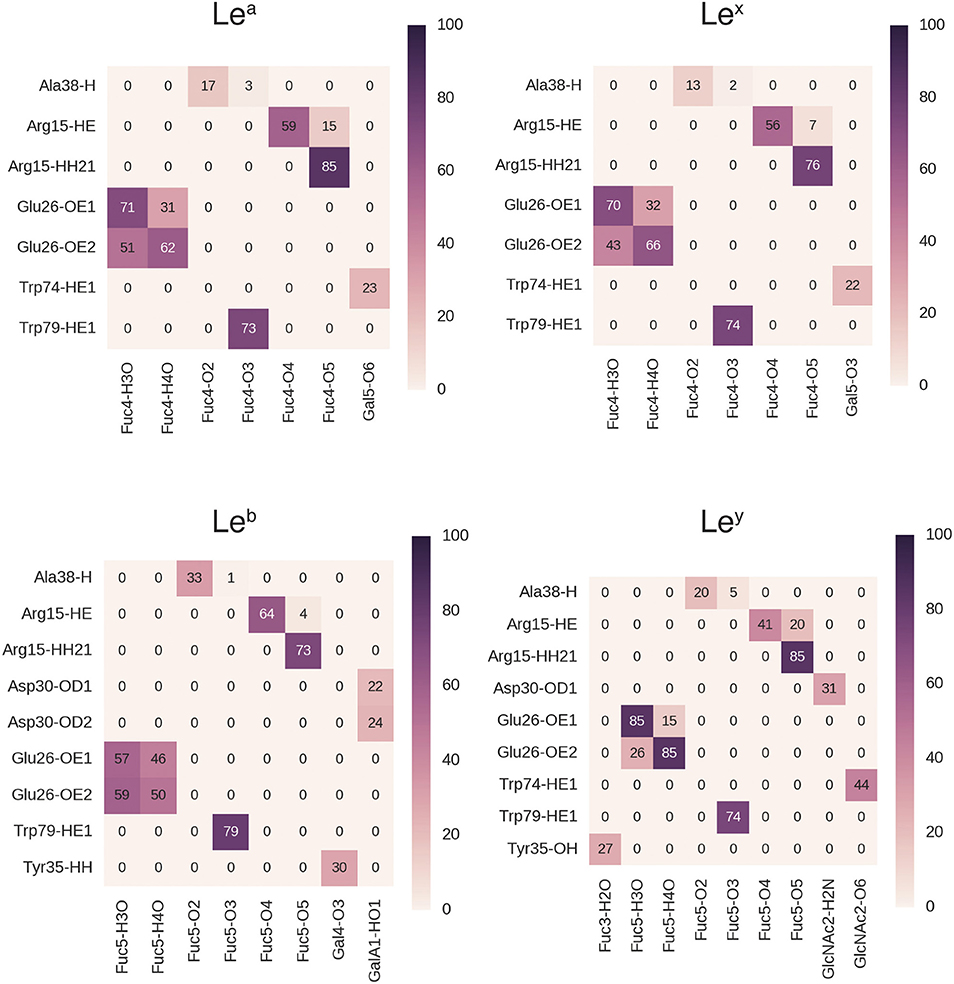
Figure 6. Hydrogen bond interactions in BambL-saccharide complexes during MD simulations shown for Lewis group saccharides. Atoms are named to the conventions of the PDB exchange dictionary. Grid cells are colored and labeled by average occupancy from three replicate simulations. Occupancy values were calculated by dividing the number of frames in which the hydrogen bond exists by the total number of simulation frames.
In addition to the above interactions, a low-occupancy (up to 30% of simulation frames) hydrogen bond was observed between the Ala38 backbone amide proton and the buried fucose 2-position hydroxyl oxygen atom. In contrast to the highly occupied hydrogen bonds, this interaction engages a backbone proton rather than a side-chain; combined with the low occupancy, this suggests a less significant contribution by this hydrogen bond to carbohydrate binding. Alongside hydrogen-bonding interactions, stacking of the fucose C3-C4-C5-C6 hydrophobic face against the Trp74 indole ring was consistently maintained during simulation (see Supplementary Figure 7).
Hydrogen bonds to non-fucose portions of the carbohydrate ligands were formed at low to moderate occupancies (20–50%) with fucose-binding residue Trp74 (Ley: 44%, Lea: 23%, Lex: 22%) and surface residue Asp30 (B: 37%, H type 1: 44%, Leb: 24%, Ley: 31%).
Glycosidic linkage conformations explored during MD simulations occupy global, and occasionally secondary, minima (Figure 7). As observed in docking, the Fucα1-2Gal linkage is again an exception, adopting a position intermediate between the two minima for the entire duration of simulation in the H type 1, H type 2, Leb and Ley complexes. In the H type 1 and Leb complexes, this linkage explores a narrower range of higher-energy conformations compared to H type 2 and Ley. It is possible that this difference between the calculated energetic minima and the conformations observed in simulation is due to the presence of the protein. Force field-based energy contours describe the energetic behavior of each linkage as an unbound disaccharide in vacuum (Imberty et al., 1995), while simulation of the bound complex introduces protein, water, and other saccharide units within the tri- or tetrasaccharide, all of which influence conformational behavior. A recent example of the influence of protein binding on carbohydrate conformation is the Lex saccharide, which occupies well-characterized “closed” conformations in solution and “open” conformations when bound to the RSL lectin (Topin et al., 2016; defined by the relative positions of the fucose and galactose rings). In the present study, the Lex saccharide maintained an open conformation during MD simulation, corresponding to shapes “Open V” and “Open II” in the scheme defined by Topin et al. (2016) consistent with its continuous occupation of the binding site during simulation (see Supplementary Figure 8).
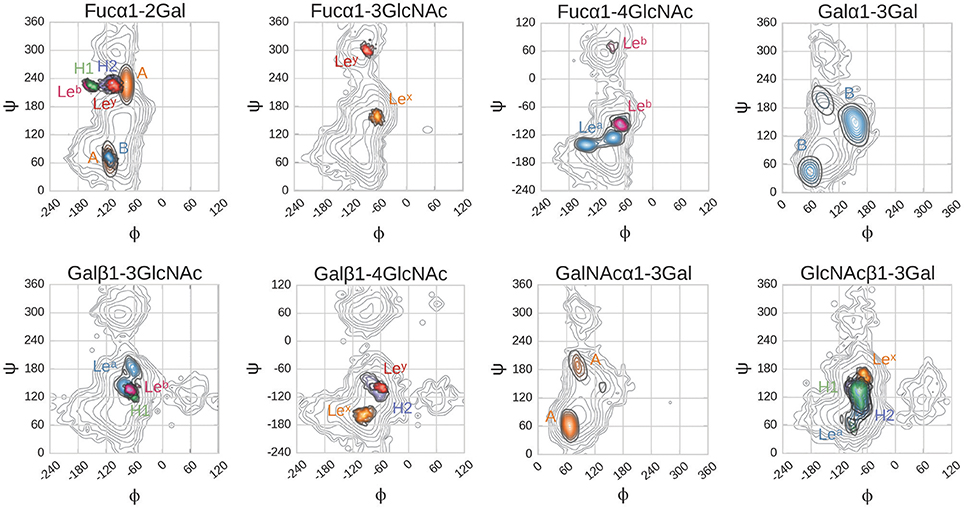
Figure 7. Glycosidic dihedral angles in BambL-blood group saccharide complexes during MD simulations. Dihedrals are defined as: φ, O5-C1-O1-Cx; ψ, C1-O1-Cx-Cx+1. Contour plots color coding: gray, calculated energy landscapes of constituting linkages; brown, A; light blue B; green H1; dark blue, H2; cyan Lea; pink, Leb; orange Lex; red Ley. Contour plot lines mark intervals of 1 kcal/mol.
In the A and B trisaccharide simulations, the N-acetylgalactosamine and non-reducing end galactose move more freely than the saccharide occupying the same position in the other ligands. The Fucα1-2Gal glycosidic linkage in these two saccharides occupies two conformations, defined by variation in the ψ-angle between −60° and +100°. The A trisaccharide explores both, while the B trisaccharide only occupies the former conformation (Figure 7).
Docking and MD Simulations of Complexes with Blood-Group-Related Carbohydrates
Interactions between BambL and blood group/tissue carbohydrates was mediated mainly via the single buried fucose, with occasional hydrogen bonds formed between non-fucose atoms and residues on loops surrounding the binding pocket. Identifying these non-fucose binding interactions may provide opportunities to improve inhibitor affinity for BambL beyond the current fucose-based inhibitors.
The potential for non-fucose binding interactions to form in BambL-saccharide complexes was explored by simulating complexes of 36 blood-group-related carbohydrates to the protein (i.e., a focused carbohydrate library). The related carbohydrates ranged in size from di- to heptasaccharides and were composed of fragments of blood group and tissue determinant carbohydrates and elongated versions of blood group carbohydrates bearing additional saccharides (for structures of all library members, see Supplementary Figure 1). Most of these structures contain fucose moieties and were expected to interact with BambL via the fucose-dominated mode observed in crystallographic structures. To explore how non-fucose residues (such as galactose and N-acetylgalactosamine) might occupy the fucose-binding site, a selection of di- and trisaccharides lacking fucose were also evaluated. Complexes with BambL were assembled by docking and simulated in explicit solvent for 400 ns.
Of the 36 complexes simulated, 28 remained stably engaged without dissociation of the ligand into bulk solvent. Multiple binding modes were observed among the stable complexes, exhibiting different hydrogen-bonding patterns (Figure 8). In some complexes (2, 6, 34, 30), very few hydrogen bonds were formed and were observed for only up to 30% of MD runs. These binding modes, while stable, did not feature significant hydrogen-bonding interactions with BambL.
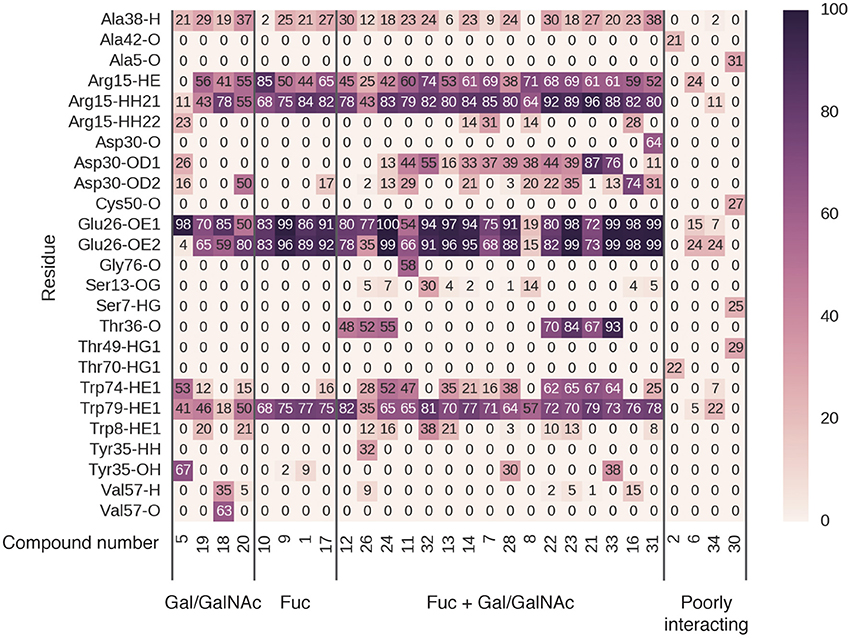
Figure 8. Hydrogen bonding occupancy of blood-group-related saccharides during MD simulations. Saccharide names indicate the ligand moieties interacting with BambL during simulation.
In four cases (5, 19, 18, 20), the ligand was found to interact with the fucose-binding pocket via a non-fucose saccharide (galactose or N-acetylgalactosamine). While these non-fucose binding modes do include hydrogen bonds to the three fucose pocket residues (Arg15, Glu26, and Trp79), these interactions are not as highly occupied as those made by fucose-containing saccharides (10, 9, 1, 17). In non-fucose binding modes, hydrogen-bond occupancies over 70% were observed for only one or two interactions per ligand; for fucose-mediated binding, all three pocket residues are engaged more than 70% of the time.
The remaining 20 carbohydrates bound in a fucose-dominated manner, forming hydrogen bonds at over 70% occupancy between a fucose and all three residues of the fucose-binding pocket. In most cases, additional hydrogen bonds were formed with loop residues outside the fucose-binding pocket, with occupancies ranging from 10 to 90%. The highly stable (>70% occupancy) non-fucose hydrogen bonds involved residues Asp30 and Thr36, located on loop 4. The acidic sidechain of Asp30 projects toward the fucose-binding pocket, accepting hydrogen bonds from saccharides not directly bonded to the buried fucose. Thr36 is located further away from the fucose-binding pocket, and accepts hydrogen bonds via the backbone carbonyl oxygen atom. A less-occupied hydrogen bond (up to 67%) is formed to the indole nitrogen of Trp74, concurrent with hydrophobic stacking against a buried fucose. Finally, Tyr35 donates a hydrogen bond via the phenolic hydroxyl to compound 28 and 33 (and additionally to the non-fucose compound 5). The fucose-dominated binding modes featuring highest occupancy of non-fucose hydrogen bonds involved carbohydrates 21 and 33, illustrated in Supplementary Figure 9.
Combining all the BambL residues involved in hydrogen bonds to fucose and non-fucose saccharides presents a perspective of the target site that incorporates a wider view of BambL-saccharide recognition, considering multiple interaction points across the protein surface (Figure 9). This view of the BambL binding site presents opportunities for future inhibitor design to consider regions outside the fucose-binding pocket.
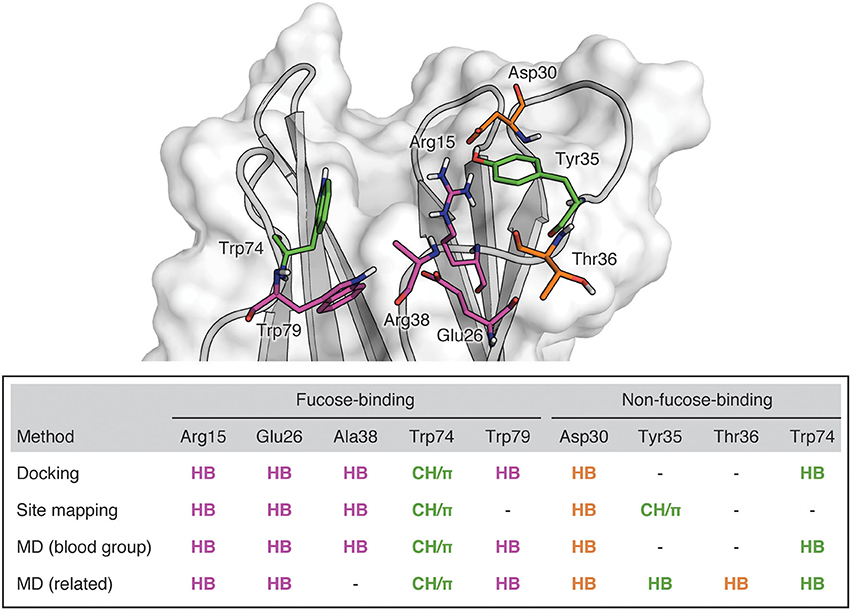
Figure 9. BambL binding site showing residues implicated in saccharide binding. Purple: Residues which form hydrogen bonds with the buried fucose saccharide. Orange: Residues which form hydrogen bonds with non-fucose saccharides. Green: Residues which participate in both hydrophobic and hydrogen bonding interactions.
Discussion
We have investigated the molecular aspects of carbohydrate recognition of the B. ambifaria lectin by computational methods: docking, site mapping, and MD. Molecular docking has been shown to be extremely useful for structural predictions, if not affinity calculations (Yuriev et al., 2015). However, docking carbohydrate ligands presents a number of challenges stemming from their extreme flexibility, a large number of hydroxyl groups, leading to the formation of (often) extensive hydrogen-bonding networks, and the formation of crucial CH/π stacking interactions between the C-H bonds of the carbohydrates (on their hydrophobic faces) and aromatic side chains of the protein (Agostino et al., 2009a, 2012a). Also, carbohydrate ligands are modular, and different residues (e.g., galactose vs. glucose) are able to establish highly similar interactions with the binding site. We have previously validated Glide and tested a range of other docking programs for structural prediction of carbohydrate complexes with antibodies (Agostino et al., 2009a, 2012b) and lectins (Agostino et al., 2011). We have demonstrated that, as the result of all the above-mentioned challenges, docking programs and scoring functions are not always able to predict the native binding pose faithfully as the top docked pose. To overcome this shortcoming and to harness the recognition information embedded in the docking output, we have developed a site mapping methodology that takes into account an ensemble of docked poses and identifies binding site residues critically involved in recognition of a ligand or ligand family (Yuriev et al., 2001, 2002; Agostino et al., 2013; Dingjan et al., 2015a).
In this study, docking with Glide produced reasonable top poses for a range of BambL complexes with blood group carbohydrates (Table 2). Using the BambL structure from PDB ID: 3ZZV gave accurate complex prediction for the B, H type 1 and H type 2 saccharides and accurate fucose placement for the A, Lea, Leb, and Ley determinants. All these complexes featured a buried fucose residue (Fucα1-2Gal), providing the majority of hydrogen-bonding interactions, and conformational ranges reflective of predicted energetic minima (Imberty et al., 1995) and relevant experimental structures (Yuriev et al., 2005; Dingjan et al., 2015b). Notably, the distances between fucose carbon atoms and the geometric centers of the imidazole phenyl and pyrrole component ring systems of Trp74 (Supplementary Material, Table S1) are similar to reported geometries for fucose CH/π dispersion interactions of a closely related lectin, RSL (Wimmerova et al., 2012). As in the RSL-fucose complex, the C6 atom interacts with the pyrrole part of the imidazole ring (distance of 3.76 ± 0.3 Å), while C3 is further than 4 Å away. Unlike the RSL complex, C5 also interacts with the pyrrole ring (distance of 3.83 ± 0.1 Å), rather than the phenyl ring, which is further than 4 Å from the entire non-polar plane.
Detailed elaboration of structural aspects of molecular recognition requires expanding the single snapshot view afforded by crystal structures or top docked poses. To that effect, we have undertaken site mapping and MD investigations in order to identify BambL residues critical for recognition of blood group carbohydrates. The advantage of site mapping lies in its ability to consider alternative binding modes while MD also explicitly accounts for the role of water, mediating interactions of BambL to carbohydrates.
We have identified the atomic scale binding interactions that facilitate recognition of fucosylated human blood group saccharides by BambL. A network of hydrogen bonds combined with a single hydrophobic stacking interaction between the buried fucose and amino acids in the fucose-binding pocket account for the majority of binding interactions (Figure 3). These structural features of the fucose-driven recognition closely agree with experimental characterization of BambL-carbohydrate binding profile by glycan array, which has demonstrated a preference for short, fucose-bearing saccharides, with the fucose monosaccharide among the most highly ranked binders (Audfray et al., 2012). However, this fucose-driven recognition motif does not explain the specificity profile of BambL compared to other related fucose-binding lectins. Namely, the interactions between BambL and fucosylated saccharides are highly similar to those found in complexes featuring other six-bladed β-propeller fucose-binding lectins: found in fungi [Aleuria aurantia lectin, AAL (Fujihashi et al., 2003; Wimmerova et al., 2003); Aspergillus fumigatus lectin, AFL (Houser et al., 2013); Aspergillus oryzae lectin, AOL (Makyio et al., 2016)] and bacteria [Ralstonia solanacearum lectin, RSL (Kostlánová et al., 2005)]. Members of this lectin family bind fucose via the same interactions: hydrogen bonds between O2 and a backbone amide proton, O3 and indole nitrogen, O3 and O4 to a shared carboxylate moiety, and O4 and O5 to a shared guanidinium moiety. In a previous docking study of RSL-fucose recognition by Mishra et al. (2012) the same suite of interactions was reported.
Despite the common binding mode, these lectins prefer different blood group determinants: AAL exhibits broad specificity, while AFL prefers Ley, and RSL prefers saccharides featuring Fucα1-2 and Fucα1-6 moieties (blood group A, B, and H and core of N-glycans). Varied blood group specificity has been proposed to arise from steric hindrance around the fucose-binding pocket, preventing strong binding to most branched carbohydrate structures (Fujihashi et al., 2003). Glycan array screening shows generally decreased binding to branched carbohydrates compared to mono- and disaccharides for these lectins, emphasizing the importance of steric effects (Houser et al., 2013). Additionally, the non-selective AAL lacks steric hindrance around the fucose-binding pocket: in a bound complex featuring the disaccharide Fucα1-6GlcNAcβ1-OMe, transferred NOE experiments confirmed conformational flexibility around the glycosidic linkage (Weimar and Peters, 1994). However, steric hindrance alone does not fully explain blood group selectivity in this lectin family. AFL binds the difucosylated Ley more strongly than the corresponding monofucosylated saccharide, H-type 2, despite similar steric complementarity to the binding site (Houser et al., 2013). We suggest that stabilizing interactions outside the fucose-binding pocket (as observed in simulations of BambL complexed with blood-group-related saccharides) play a role in saccharide binding in the 6-bladed β-propeller lectin family more generally.
Interactions with non-fucose residues are not as highly occupied as interactions with the fucose. However, they contribute to a wider view of BambL-carbohydrate recognition, considering multiple interaction points across the protein surface. They include hydrogen bonding to Asp30, Tyr35, Thr36, and Trp74 and hydrophobic contacts with Tyr35 (Figure 9). These contacts outside the fucose-binding pocket could be employed in future inhibitor design for BambL to address issues of opportunistic infections.
Conclusion
In summary, the present work details the recognition of fucosylated human blood group determinants by BambL, quantifies the occupancy of hydrogen bonding interactions, and identifies opportunities for targeting residues outside the fucose-binding pocket. Recognition mainly involves the fucose monosaccharide through a network of highly occupied hydrogen-bonding interactions to Arg15, Glu26, and Trp79, and a lower occupancy interaction with Ala38. An additional stacking interaction between the fucose hydrophobic face and Trp74 is also highly occupied in MD simulations. Hydrogen bonds to non-fucose saccharides were formed in complexes with Ley, Leb, Lea, H1, H2, and B trisaccharide and in multiple complexes involving blood-group-related saccharides. The most occupied interactions involved Asp30, Thr36, Trp74, and to a lesser degree Tyr35. Carbohydrate recognition by BambL is therefore proposed to be driven by interactions in the fucose-binding site and further stabilized by satellite interactions between non-fucose saccharides and surface residues outside the fucose-binding pocket. The analysis of carbohydrate recognition by BambL presented in this study lays the foundation for the development of fucomimetic molecules able to bind to BambL. Such molecules have potential as anti-adhesives for the treatment of B. ambifaria infection in cystic fibrosis patients.
Author Contributions
Each author has contributed significantly to the submitted work. TD and EY conceived and designed the experiments. TD performed the experiments. TD, EY, and PR analyzed the data. TD, AI, SP, EY, and PR wrote the paper. All authors read and approved the final manuscript.
Funding
This work was supported by a Victorian Life Sciences Computation Initiative (VLSCI) grant number VR0250 on its Peak Computing Facility at the University of Melbourne, an initiative of the Victorian Government, Australia, and with the assistance of resources from the National Computational Infrastructure (NCI), which is supported by the Australian Government, and the Multi-modal Australian ScienceS Imaging and Visualization Environment (MASSIVE) via grant Y96. TD is supported by an Australian Postgraduate Award (APA) scholarship. AI and SP are supported by CNRS, Université Grenoble Alpes through Glyco@Alps (ANR-15-IDEX-02) and Labex ARCANE (ANR-11-LABX-0003-01). PR is supported by an RMIT University Vice Chancellor's Senior Research Fellowship.
Conflict of Interest Statement
The authors declare that the research was conducted in the absence of any commercial or financial relationships that could be construed as a potential conflict of interest.
Supplementary Material
The Supplementary Material for this article can be found online at: http://journal.frontiersin.org/article/10.3389/fphar.2017.00393/full#supplementary-material
Abbreviations
BambL, Burkholderia ambifaria lectin; H1, H type 1; H2, H type 2; Lea, Lewis a; Leb, Lewis b; Lex, Lewis x; Ley, Lewis y; MD, Molecular Dynamics; PDB, Protein Data Bank; vdW, van der Waals; RMSD, root mean square deviation
References
Agostino, M., Jene, C., Boyle, T., Ramsland, P. A., and Yuriev, E. (2009a). Molecular docking of carbohydrate ligands to antibodies: structural validation against crystal structures. J. Chem. Inf. Model. 49, 2749–2760. doi: 10.1021/ci900388a
Agostino, M., Mancera, R. L., Ramsland, P. A., and Yuriev, E. (2013). AutoMap: A tool for analyzing protein-ligand recognition using multiple ligand binding modes. J. Mol. Graphics Modell. 40, 80–90. doi: 10.1016/j.jmgm.2013.01.001
Agostino, M., Ramsland, P. A., and Yuriev, E. (2012a). “Docking of carbohydrates into protein binding sites,” in Structural Glycobiology, eds E. Yuriev and P. A. Ramsland (Boca Raton, FL: CRC Press), 111–138.
Agostino, M., Sandrin, M. S., Thompson, P. E., Yuriev, E., and Ramsland, P. A. (2009b). In silico analysis of antibody-carbohydrate interactions and its application to xenoreactive antibodies. Mol. Immunol. 47, 233–246. doi: 10.1016/j.molimm.2009.09.031
Agostino, M., Yuriev, E., and Ramsland, P. A. (2011). A computational approach for exploring carbohydrate recognition by lectins in innate immunity. Front. Immunol. 2:23. doi: 10.3389/fimmu.2011.00023
Agostino, M., Yuriev, E., and Ramsland, P. A. (2012b). Antibody recognition of cancer-related gangliosides and their mimics investigated using in silico site mapping. PLoS ONE 7:e35457. doi: 10.1371/journal.pone.0035457
Anstee, D. J. (2010). The relationship between blood groups and disease. Blood 115, 4635–4643. doi: 10.1182/blood-2010-01-261859
Audfray, A., Claudinon, J., Abounit, S., Ruvoen-Clouet, N., Larson, G., Smith, D. F., et al. (2012). Fucose-binding lectin from opportunistic pathogen Burkholderia ambifaria binds to both plant and human oligosaccharidic epitopes. J. Biol. Chem. 287, 4335–4347. doi: 10.1074/jbc.M111.314831
Audfray, A., Varrot, A., and Imberty, A. (2013). Bacteria love our sugars: interaction between soluble lectins and human fucosylated glycans, structures, thermodynamics and design of competing glycocompounds. C. R. Chim. 16, 482–490. doi: 10.1016/j.crci.2012.11.021
Banks, J. L., Beard, H. S., Cao, Y., Cho, A. E., Damm, W., Farid, R., et al. (2005). Integrated modeling program, applied chemical theory (IMPACT). J. Comput. Chem. 26, 1752–1780. doi: 10.1002/jcc.20292
Bavington, C., and Page, C. (2005). Stopping bacterial adhesion: a novel approach to treating infections. Respiration 72, 335–344. doi: 10.1159/000086243
Berendsen, H. J. C., van der Spoel, D., and van Drunen, R. (1995). GROMACS: a message-passing parallel molecular dynamics implementation. Comput. Phys. Commun. 91, 43–56. doi: 10.1016/0010-4655(95)00042-E
Berman, H. M., Westbrook, J., Feng, Z., Gilliland, G., Bhat, T. N., Weissig, H., et al. (2000). The protein data bank. Nucleic Acids Res. 28, 235–242. doi: 10.1093/nar/28.1.235
Berman, H., Henrick, K., and Nakamura, H. (2003). Announcing the worldwide protein data bank. Nat. Struct. Mol. Biol. 10, 980–980. doi: 10.1038/nsb1203-980
Blackburn, L., Brownlee, K., Conway, S., and Denton, M. (2004). ‘Cepacia syndrome’ with Burkholderia multivorans, 9 years after initial colonization. J. Cyst. Fibros. 3, 133–134. doi: 10.1016/j.jcf.2004.03.007
Caverly, L. J., Zhao, J., and LiPuma, J. J. (2015). Cystic fibrosis lung microbiome: opportunities to reconsider management of airway infection. Pediatr. Pulmonol. 50, S31–S38. doi: 10.1002/ppul.23243
Chemani, C., Imberty, A., de Bentzmann, S., Pierre, M., Wimmerova, M., Guery, B. P., et al. (2009). Role of LecA and LecB lectins in Pseudomonas aeruginosa-induced lung injury and effect of carbohydrate ligands. Infect. Immun. 77, 2065–2075. doi: 10.1128/IAI.01204-08
Ciofu, O., Hansen, C. R., and Hoiby, N. (2013). Respiratory bacterial infections in cystic fibrosis. Curr. Opin. Pulm. Med. 19, 251–258. doi: 10.1097/MCP.0b013e32835f1afc
Coenye, T., Mahenthiralingam, E., Henry, D., LiPuma, J. J., Laevens, S., Gillis, M., et al. (2001). Burkholderia ambifaria sp nov., a novel member of the Burkholderia cepacia complex including biocontrol and cystic fibrosis-related isolates. Int. J. Syst. Evol. Microbiol. 51, 1481–1490. doi: 10.1099/00207713-51-4-1481
De Smet, B., Mayo, M., Peeters, C., Zlosnik, J. E. A., Spilker, T., Hird, T. J., et al. (2015). Burkholderia stagnalis sp. nov., and Burkholderia territorii sp. nov., two novel Burkholderia cepacia complex species from environmental and human sources. Int. J. Syst. Evol. Microbiol. 65, 2265–2271. doi: 10.1099/ijs.0.000251
Dingjan, T., Agostino, M., Ramsland, P. A., and Yuriev, E. (2015a). “Antibody-carbohydrate recognition from docked ensembles using the automap procedure,” in Carbohydrate-Based Vaccines, ed B. Lepenies (New York, NY: Springer), 41–55. doi: 10.1007/978-1-4939-2874-3_4
Dingjan, T., Spendlove, I., Durrant, L. G., Scott, A. M., Yuriev, E., and Ramsland, P. A. (2015b). Structural biology of antibody recognition of carbohydrate epitopes and potential uses for targeted cancer immunotherapies. Mol. Immunol. 67, 75–88. doi: 10.1016/j.molimm.2015.02.028
Ernst, B., and Magnani, J. L. (2009). From carbohydrate leads to glycomimetic drugs. Nat. Rev. Drug Disc. 8, 661–677. doi: 10.1038/nrd2852
Friesner, R. A., Banks, J. L., Murphy, R. B., Halgren, T. A., Klicic, J. J., Mainz, D. T., et al. (2004). Glide: a new approach for rapid, accurate docking and scoring. 1. method and assessment of docking accuracy. J. Med. Chem. 47, 1739–1749. doi: 10.1021/jm0306430
Friesner, R. A., Murphy, R. B., Repasky, M. P., Frye, L. L., Greenwood, J. R., Halgren, T. A., et al. (2006). Extra precision Glide: docking and scoring incorporating a model of hydrophobic enclosure for protein−ligand complexes. J. Med. Chem. 49, 6177–6196. doi: 10.1021/jm051256o
Fujihashi, M., Peapus, D. H., Kamiya, N., Nagata, Y., and Miki, K. (2003). Crystal structure of fucose-specific lectin from Aleuria aurantia binding ligands at three of its five sugar recognition sites. Biochemistry 42, 11093–11099. doi: 10.1021/bi034983z
Halgren, T. A., Murphy, R. B., Friesner, R. A., Beard, H. S., Frye, L. L., Pollard, W. T., et al. (2004). Glide: a new approach for rapid, accurate docking and scoring. 2. enrichment factors in database screening. J. Med. Chem. 47, 1750–1759. doi: 10.1021/jm030644s
Hess, B. (2007). P-LINCS: a parallel linear constraint solver for molecular simulation. J. Chem. Theory Comput. 4, 116–122. doi: 10.1021/ct700200b
Hess, B., Kutzner, C., van der Spoel, D., and Lindahl, E. (2008). GROMACS 4: algorithms for highly efficient, load-balanced, and scalable molecular simulation. J. Chem. Theory Comput. 4, 435–447. doi: 10.1021/ct700301q
Holmner, Å., Mackenzie, A., and Krengel, U. (2010). Molecular basis of cholera blood-group dependence and implications for a world characterized by climate change. FEBS Lett. 584, 2548–2555. doi: 10.1016/j.febslet.2010.03.050
Houser, J., Komarek, J., Cioci, G., Varrot, A., Imberty, A., and Wimmerova, M. (2015). Structural insights into Aspergillus fumigatus lectin specificity: AFL binding sites are functionally non-equivalent. Acta Crystallogr. D Biol. Crystallogr. 71, 442–453. doi: 10.1107/S1399004714026595
Houser, J., Komarek, J., Kostlanova, N., Cioci, G., Varrot, A., Kerr, S. C., et al. (2013). A soluble fucose-specific lectin from Aspergillus fumigatus conidia - structure, specificity and possible role in fungal pathogenicity. PLoS ONE 8:e83077. doi: 10.1371/journal.pone.0083077
Imberty, A., and Varrot, A. (2008). Microbial recognition of human cell surface glycoconjugates. Curr. Opin. Struct. Biol. 18, 567–576. doi: 10.1016/j.sbi.2008.08.001
Imberty, A., Mikros, E., Koca, J., Mollicone, R., Oriol, R., and Perez, S. (1995). Computer simulation of histo-blood group oligosaccharides: energy maps of all constituting disaccharides and potential energy surfaces of 14 ABH and Lewis carbohydrate antigens. Glycoconj. J. 12, 331–349. doi: 10.1007/BF00731336
Imberty, A., Wimmerova, M., Mitchell, E. P., and Gilboa-Garber, N. (2004). Structures of the lectins from Pseudomonas aeruginosa: insights into the molecular basis for host glycan recognition. Microbes Infect. 6, 221–228. doi: 10.1016/j.micinf.2003.10.016
Jackson, T. A., Robertson, V., and Auzanneau, F. I. (2014). Evidence for two populated conformations for the Dimeric Le(X) and Le(A)Le(X) tumor-associated carbohydrate antigens. J. Med. Chem. 57, 817–827. doi: 10.1021/jm401576x
Kirschner, K. N., Yongye, A. B., Tschampel, S. M., González-Outeiriño, J., Daniels, C. R., Foley, B. L., et al. (2008). GLYCAM06: a generalizable biomolecular force field. Carbohydrates. J. Comput. Chem. 29, 622–655. doi: 10.1002/jcc.20820
Kostlánová, N., Mitchell, E. P., Lortat-Jacob, H., Oscarson, S., Lahmann, M., Gilboa-Garber, N., et al. (2005). The fucose-binding Lectin from Ralstonia solanacearum: a new type of β-propeller architecture formed by oligomerization and interacting with fucoside, fucosyllactose, and plant xyloglucan. J. Biol. Chem. 280, 27839–27849. doi: 10.1074/jbc.M505184200
Laskowski, R. A., and Swindells, M. B. (2011). LigPlot+: multiple ligand-protein interaction diagrams for drug discovery. J. Chem. Inf. Model. 51, 2778–2786. doi: 10.1021/ci200227u
Lee, C. H., Kim, M., Kim, H., Ahn, J. H., Yi, Y., Kang, K., et al. (2006). An antifungal property of Burkholderia ambifaria against phytopathogenic fungi. J. Microbiol. Biotechnol. 16, 465–468.
Li, W., Roberts, D. P., Dery, P. D., Meyer, S. L. F., Lohrke, S., Lumsden, R. D., et al. (2002). Broad spectrum anti-biotic activity and disease suppression by the potential biocontrol agent Burkholderia ambifaria BC-F. Crop Prot. 21, 129–135. doi: 10.1016/S0261-2194(01)00074-6
Lindén, S., Mahdavi, J., Semino-Mora, C., Olsen, C., Carlstedt, I., Borén, T., et al. (2008). Role of ABO secretor status in mucosal innate immunity and H. pylori Infection. PLoS Pathog. 4:e2. doi: 10.1371/journal.ppat.0040002
Lindorff-Larsen, K., Piana, S., Palmo, K., Maragakis, P., Klepeis, J. L., Dror, R. O., et al. (2010). Improved side-chain torsion potentials for the Amber ff99SB protein force field. Proteins 78, 1950–1958. doi: 10.1002/prot.22711
Loutet, S. A., and Valvano, M. A. (2011). Extreme antimicrobial Peptide and polymyxin B resistance in the genus burkholderia. Front. Microbiol. 2:59. doi: 10.3389/fmicb.2011.00159
Lyczak, J. B., Cannon, C. L., and Pier, G. B. (2002). Lung infections associated with cystic fibrosis. Clin. Microbiol. Rev. 15, 194–222. doi: 10.1128/CMR.15.2.194-222.2002
Lynch, J. P. III. (2009). Burkholderia cepacia complex: impact on the cystic fibrosis lung lesion. Sem. Resp. Crit. Care Med. 30, 596–610. doi: 10.1055/s-0029-1238918
Madhavi Sastry, G., Adzhigirey, M., Day, T., Annabhimoju, R., and Sherman, W. (2013). Protein and ligand preparation: parameters, protocols, and influence on virtual screening enrichments. J. Comput. Aided Mol. Des. 27, 221–234. doi: 10.1007/s10822-013-9644-8
Makeneni, S., Foley, B. L., and Woods, R. J. (2014). BFMP: a method for discretizing and visualizing pyranose conformations. J. Chem. Inf. Model. 54, 2744–2750. doi: 10.1021/ci500325b
Makyio, H., Shimabukuro, J., Suzuki, T., Imamura, A., Ishida, H., Kiso, M., et al. (2016). Six independent fucose-binding sites in the crystal structure of Aspergillus oryzae lectin. Biochem. Biophys. Res. Commun. 477, 477–482. doi: 10.1016/j.bbrc.2016.06.069
Martinucci, M., Roscetto, E., Iula, V. D., Votsi, A., Catania, M. R., and De Gregorio, E. (2016). Accurate identification of members of the Burkholderia cepacia complex in cystic fibrosis sputum. Lett. Appl. Microbiol. 62, 221–229. doi: 10.1111/lam.12537
McGibbon, R. T., Beauchamp, K. A., Harrigan, M. P., Klein, C., Swails, J. M., Hernández, C. X., et al. (2015). MDTraj: a modern open library for the analysis of molecular dynamics trajectories. Biophys. J. 109, 1528–1532. doi: 10.1016/j.bpj.2015.08.015
Michaud-Agrawal, N., Denning, E. J., Woolf, T. B., and Beckstein, O. (2011). MDAnalysis: a toolkit for the analysis of molecular dynamics simulations. J. Comput. Chem. 32, 2319–2327. doi: 10.1002/jcc.21787
Mishra, S. K., Adam, J., Wimmerova, M., and Koca, J. (2012). In silico mutagenesis and docking study of Ralstonia solanacearum RSL lectin: performance of docking software to predict saccharide binding. J. Chem. Inf. Model. 52, 1250–1261. doi: 10.1021/ci200529n
Mitchell, E., Houles, C., Sudakevitz, D., Wimmerova, M., Gautier, C., Perez, S., et al. (2002). Structural basis for oligosaccharide-mediated adhesion of Pseudomonas aeruginosa in the lungs of cystic fibrosis patients. Nat. Struct. Biol. 9, 918–921. doi: 10.1038/nsb865
O'Sullivan, B. P., and Freedman, S. D. (2009). Cystic fibrosis. Lancet 373, 1891–1904. doi: 10.1016/S0140-6736(09)60327-5
Parra-Cota, F. I., Pena-Cabriales, J. J., de los Santos-Villalobos, S., Martinez-Gallardo, N. A., and Delano-Frier, J. P. (2014). Burkholderia ambifaria and B. caribensis promote growth and increase yield in grain Amaranth (Amaranthus cruentus and A-hypochondriacus) by improving plant nitrogen uptake. PLoS ONE 9:14. doi: 10.1371/journal.pone.0088094
Pera, N. P., and Peters, R. J. (2014). Towards bacterial adhesion-based therapeutics and detection methods. Med. Chem. Comm. 5, 1027–1035. doi: 10.1039/C3MD00346A
Pieters, R. J. (2011). “The role of carbohydrates in bacterial infections,” in Carbohydrate Recognition, eds B. Wang and G. J. Boons (Hoboken: John Wiley & Sons, Inc.), 93–106.
Podnecky, N. L., Rhodes, K. A., and Schweizer, H. P. (2015). Efflux pump-mediated drug resistance in Burkholderia. Front. Microbiol. 6:305. doi: 10.3389/fmicb.2015.00305
Pronk, S., Páll, S., Schulz, R., Larsson, P., Bjelkmar, P., Apostolov, R., et al. (2013). GROMACS 4.5: a high-throughput and highly parallel open source molecular simulation toolkit. Bioinformatics 29, 845–854. doi: 10.1093/bioinformatics/btt055
Rhim, A. D., Stoykova, L., Glick, M. C., and Scanlin, T. F. (2001). Terminal glycosylation in cystic fibrosis (CF): a review emphasizing the airway epithelial cell. Glycoconj. J. 18, 649–659. doi: 10.1023/A:1020815205022
Schrödinger (2014a). “Glide”, in Small-Molecule Drug Discovery Suite 2014-1, 6.2 Edn. (New York, NY: Schrödinger, LLC)
Schrödinger (2014b). “Maestro,” in Schrödinger Release 2014-1, 9.7 Edn. (New York, NY: Schrödinger, LLC).
Schrödinger (2014c). “Prime,” in Schrödinger Release 2014-1, 4.4 Edn. (New York, NY: Schrödinger, LLC).
Sharon, N. (2006). Carbohydrates as future anti-adhesion drugs for infectious diseases. Biochim. Biophys. Acta Gen. Subj. 1760, 527–537. doi: 10.1016/j.bbagen.2005.12.008
Sousa da Silva, A., and Vranken, W. (2012). ACPYPE—AnteChamber PYthon Parser interfacE. BMC Res. Notes 5:367. doi: 10.1186/1756-0500-5-367
Stoykova, L. I., and Scanlin, T. F. (2008). Cystic fibrosis (CF), Pseudomonas aeruginosa, CFTR and the CF glycosylation phenotype: a review and update. Curr. Org. Chem. 12, 900–910. doi: 10.2174/138527208784892169
Sulak, O., Cioci, G., Delia, M., Lahmann, M., Varrot, A., Imberty, A., et al. (2010). A TNF-like trimeric lectin domain from Burkholderia cenocepacia with specificity for fucosylated human histo-blood group antigens. Structure 18, 59–72. doi: 10.1016/j.str.2009.10.021
Sulak, O., Cioci, G., Lameignere, E., Balloy, V., Round, A., Gutsche, I., et al. (2011). Burkholderia cenocepacia BC2L-C is a super lectin with dual specificity and proinflammatory activity. PLoS Pathog. 7:e1002238. doi: 10.1371/journal.ppat.1002238
Tempel, W., Tschampel, S., and Woods, R. J. (2002). The xenograft antigen bound to Griffonia simplicifolia lectin 1-B-4—X-ray crystal structure of the complex and molecular dynamics characterization of the binding site. J. Biol. Chem. 277, 6615–6621. doi: 10.1074/jbc.M109919200
Topin, J., Arnaud, J., Sarkar, A., Audfray, A., Gillon, E., Perez, S., et al. (2013). Deciphering the glycan preference of bacterial lectins by glycan array and molecular docking with validation by microcalorimetry and crystallography. PLoS ONE 8:e71149. doi: 10.1371/journal.pone.0071149
Topin, J., Lelimousin, M., Arnaud, J., Audfray, A., Pérez, S., Varrot, A., et al. (2016). The hidden conformation of Lewis x, a human histo-blood group antigen, is a determinant for recognition by pathogen lectins. ACS Chem. Biol. 11, 2011–2020. doi: 10.1021/acschembio.6b00333
Van Der Spoel, D., Lindahl, E., Hess, B., Groenhof, G., Mark, A. E., and Berendsen, H. J. C. (2005). GROMACS: fast, flexible, and free. J. Comput. Chem. 26, 1701–1718. doi: 10.1002/jcc.20291
Vandamme, P., Holmes, B., Vancanneyt, M., Coenye, T., Hoste, B., Coopman, R., et al. (1997). Occurrence of multiple genomovars of Burkholderia cepacia in cystic fibrosis patients and proposal of Burkholderia multivorans sp. nov. Int. J. Syst. Bacteriol. 47, 1188–1200. doi: 10.1099/00207713-47-4-1188
Venkatakrishnan, V., Thaysen-Andersen, M., Chen, S. C. A., Nevalainen, H., and Packer, N. H. (2015). Cystic fibrosis and bacterial colonization define the sputum N-glycosylation phenotype. Glycobiology 25, 88–100. doi: 10.1093/glycob/cwu092
von Bismarck, P., Schneppenheim, R., and Schumacher, U. (2001). Successful treatment of Pseudomonas aeruginosa respiratory tract infection with a sugar solution–a case report on a lectin based therapeutic principle. Klin. Padiatr. 213, 285–287. doi: 10.1055/s-2001-17220
Wallace, A. C., Laskowski, R. A., and Thornton, J. M. (1995). LIGPLOT—a program to generate schematic diagrams of protein ligand interactions. Protein Eng. 8, 127–134. doi: 10.1093/protein/8.2.127
Weimar, T., and Peters, T. (1994). Aleuria aurantia agglutinin recognizes multiple conformations of α-L-Fuc-(1→6)-β-D-GlcNAc-OMe. Angew. Chem. Int. Ed. Engl. 33, 88–91. doi: 10.1002/anie.199400881
Wimmerova, M., Kozmon, S., Necasova, I., Mishra, S. K., Komarek, J., and Koca, J. (2012). Stacking interactions between carbohydrate and protein quantified by combination of theoretical and experimental methods. PLoS ONE 7:e46032. doi: 10.1371/journal.pone.0046032
Wimmerova, M., Mitchell, E., Sanchez, J.-F., Gautier, C., and Imberty, A. (2003). Crystal structure of fungal lectin: six-bladed β-propeller fold and novel fucose recognition mode for Aleuria aurantia lectin. J. Biol. Chem. 278, 27059–27067. doi: 10.1074/jbc.M302642200
Woods, R. J. (2005). GLYCAM Web [Online]. Complex Carbohydrate Research Center, University of Georgia, Athens, G. A. Available Online at: http://www.glycam.com (Accessed June 1 2015).
Yuriev, E., Farrugia, W., Scott, A. M., and Ramsland, P. A. (2005). Three-dimensional structures of carbohydrate determinants of Lewis system antigens: implications for effective antibody targeting of cancer. Immunol. Cell Biol. 83, 709–717. doi: 10.1111/j.1440-1711.2005.01374.x
Yuriev, E., Holien, J., and Ramsland, P. A. (2015). Improvements, trends, and new ideas in molecular docking: 2012–2013 in review. J. Mol. Recognit. 28, 581–604. doi: 10.1002/jmr.2471
Yuriev, E., Ramsland, P. A., and Edmundson, A. B. (2001). Docking of combinatorial peptide libraries into a broadly cross-reactive human IgM. J. Mol. Recognit. 14, 172–184. doi: 10.1002/jmr.533
Yuriev, E., Ramsland, P. A., and Edmundson, A. B. (2002). Recognition of IgG-derived peptides by a human IgM with an unusual combining site. Scand. J. Immunol. 55, 242–255. doi: 10.1046/j.0300-9475.2002.01032.x
Zahariadis, G., Levy, M. H., and Burns, J. L. (2003). Cepacia-like syndrome caused by Burkholderia multivorans. Can. J. Infect. Dis. 14, 123–125. doi: 10.1155/2003/675159
Keywords: blood group determinants, Burkholderia ambifaria, docking, fucose, molecular dynamics
Citation: Dingjan T, Imberty A, Pérez S, Yuriev E and Ramsland PA (2017) Molecular Simulations of Carbohydrates with a Fucose-Binding Burkholderia ambifaria Lectin Suggest Modulation by Surface Residues Outside the Fucose-Binding Pocket. Front. Pharmacol. 8:393. doi: 10.3389/fphar.2017.00393
Received: 12 March 2017; Accepted: 06 June 2017;
Published: 21 June 2017.
Edited by:
Leonardo G. Ferreira, University of São Paulo, BrazilReviewed by:
Hugo Verli, Universidade Federal do Rio Grande do Sul, BrazilDavid Antony Morton-Blake, Trinity College, Dublin, Ireland
Copyright © 2017 Dingjan, Imberty, Pérez, Yuriev and Ramsland. This is an open-access article distributed under the terms of the Creative Commons Attribution License (CC BY). The use, distribution or reproduction in other forums is permitted, provided the original author(s) or licensor are credited and that the original publication in this journal is cited, in accordance with accepted academic practice. No use, distribution or reproduction is permitted which does not comply with these terms.
*Correspondence: Elizabeth Yuriev, ZWxpemFiZXRoLnl1cmlldkBtb25hc2guZWR1
Paul A Ramsland, cGF1bC5yYW1zbGFuZEBybWl0LmVkdS5hdQ==