- 1Service de Chirurgie Viscérale Pédiatrique, Hôpital des Enfants, Limoges Cedex, France
- 2Service de Chirurgie Pédiatrique Viscérale, Urologique et Transplantation, Hôpital Necker-Enfants Malades, Assistance Publique-Hôpitaux de Paris, Université Paris Cité, Paris, France
- 3Service de Chirurgie Pédiatrique, CHU Rennes, Institut NuMeCan, INRAe, INSERM, Univ Rennes, Rennes, France
- 4Service d’Oncologie Pédiatrique, Hôpital des Enfants, Limoges Cedex, France
- 5Service de Radiologie Pédiatrique, Hôpital des Enfants, Limoges Cedex, France
Introduction: Preoperative three-dimensional (3D) reconstruction using sectional imaging is increasingly used in challenging pediatric cases to aid in surgical planning. Many case series have described various teams' experiences, discussing feasibility and realism, while emphasizing the technological potential for children. Nonetheless, general knowledge on this topic remains limited compared to the broader research landscape. The aim of this review was to explore the current devices and new opportunities provided by preoperative Computed Tomography (CT) scans or Magnetic Resonance Imaging (MRI).
Methods: A systematic review was conducted to screen pediatric cases of abdominal and pelvic tumors with preoperative 3D reconstruction published between 2000 and 2023.
Discussion: Surgical planning was facilitated through virtual reconstruction or 3D printing. Virtual reconstruction of complex tumors enables precise delineation of solid masses, formulation of dissection plans, and suggests dedicated vessel ligation, optimizing tissue preservation. Vascular mapping is particularly relevant for liver surgery, large neuroblastoma with imaging-defined risk factors (IDRFs), and tumors encasing major vessels, such as complex median retroperitoneal malignant masses. 3D printing can facilitate specific tissue preservation, now accessible with minimally invasive procedures like partial nephrectomy. The latest advancements enable neural plexus reconstruction to guide surgical nerve sparing, for example, hypogastric nerve modelling, typically adjacent to large pelvic tumors. New insights will soon incorporate nerve plexus images into anatomical segmentation reconstructions, facilitated by non-irradiating imaging modalities like MRI.
Conclusion: Although not yet published in pediatric surgical procedures, the next anticipated advancement is augmented reality, enhancing real-time intraoperative guidance: the surgeon will use a robotic console overlaying functional and anatomical data onto a magnified surgical field, enhancing robotic precision in confined spaces.
Introduction
Pediatric oncologic surgery is often considered the pinnacle for pediatric surgeons, given its critical impact on clinical outcomes, particularly patient survival and quality of life (1, 2). Advances in minimally invasive surgery have led to promising improvements in safety and precision, thanks to emerging techniques such as robotic platforms (3) and fluorescence-guided surgery (4). Surgical planning remains crucial to assess the feasibility of adhering to oncologic principles, such as achieving en-bloc macroscopically complete resection or preserving neighboring organs, while also avoiding injury to adjacent vital structures.
3D technology proves to be a valuable tool, as evidenced in cases series, providing additional information and reducing surgical complications compared to relying solely on 2D information (5, 6). Although 3D modelling and printing are not yet standardized in clinical practice, their popularity is on the rise, given their demonstrated positive impact on clinical outcomes in the adult population (7). For pediatric cases, particularly complex ones like neuroblastoma with IDRFs, they are deemed indispensable, because they offer intraoperative tumor anatomy reconstruction and facilitate guidance during procedures (8).
The delineation of tumors and adjacent organs enables a comprehensive understanding of the region of interest through a 3D physical or virtual model. This enhances comprehension of the underlying structures, facilitates simulation of the resection line, and aids in procedure planning.
The initial phase of 3D surgical planning involves the acquisition of images by radiologists. Computed Tomography (CT scan) and Magnetic Resonance Imaging (MRI) images are converted to Digital Imaging and Communications in Medicine (DICOM) format. Specific post-processing medical imaging techniques, such as Multiplanar Reconstruction, Volume Rendering (VolR), and Cinematic Rendering (CR), are then utilized to automatically convert the standardized 2D images into 3D images for projection on a screen (9).
Following image conversion, DICOM data segmentation is performed through collaboration among radiologists, surgeons and bioengineers. This segmentation process involves the use of specific software to reconstruct the DICOM data into 3D models, which can be further utilized for techniques like 3D printing, virtual reality (VR), and augmented reality (AR). Since 2004 (10), numerous surgical practitioners have documented their experiences in surgical planning for abdominal pediatric tumors, utilizing 3D modelling, 3D printing, and VR technologies.
The aim of this review was to describe the various 3D surgical planning techniques and to highlight their respective benefits, limitations, and potential applications.
Material and methods
Search strategy
Two authors (PL and QB) independently conducted a literature search of the MEDLINE/PubMed/Cochrane online databases using the following terms: Term 1—“pediatric oncology” OR “Wilms tumor” OR “pediatric abdominal tumor” OR “pediatric abdominal tumor” OR “Neuroblastoma” OR “retroperitoneal tumor” OR “Hepatoblastoma” OR “Germ cell tumor” AND Term 2—“3D reconstruction” OR “3D modelling” OR “3D printing” OR “augmented reality” OR “virtual reality” or “mixed reality”. All relevant studies published between 2000 and 2023 were retrieved, and duplicates were removed upon identification. Filters were applied to limit results to the English language, human research, and publications from the year 2000 onwards.
Publication selection
The inclusion criteria for the search were as follows: (i) mean participant age under 18 years; (ii) patient population undergoing a surgical procedure; (iii) 3D reconstruction focusing on preoperative tumor visualization; (iv) tumor located in the abdominal or pelvic region.
Exclusion criteria included review articles and conference abstracts, studies related to thoracic, limb, or brain tumors, as well as those concerning protheses and tissue constructs. Duplicates were removed upon identification. Publications written in languages other than English or without full paper available were excluded based on the abstract.
Data extraction
Two reviewers (PL, QB) conducted independent searches and compared results after assessing all identified abstracts for compliance with the review criteria. In cases where agreement could not be reached, a third independent reviewer (AB) was consulted. Reasons for exclusion were documented.
The following data were extracted from the eligible studies: sample size, mean age, country of origin of the study population, study design, type of imaging performed, tumor and tumor location, type of surgery performed, and complications.
Results
Our search across the various databases yielded 86 articles, with 3 duplicates. Of these, 71 were assessed for eligibility, but only 13 met our criteria (Figure 1). No cases involving virtual or mixed reality were found in the literature. We did not uncover any instances of surgical resection using robotic laparoscopic assistance or cases of augmented reality applied to abdominal tumor resection in children. There were no documented cases of fetal tumor 3D exploration using MRI. Among the 13 articles identified, 4 pertained to virtual reconstruction (5, 10–12), and 10 articles focused on 3D printing, potentially in conjunction with virtual reconstruction (13–22). In the first group of studies, the surgical team did not have access to physical models of the tumor or anatomical structures. In the second group, no standardized printing systems were recommended. These pediatric studies are detailed in Table 1, comprising retrospective cases series with over 20 patients, totaling 87 cases with a mean age of 40.5 (±27.2) months. Three patients from our centers were included in this series to illustrate the discussion (Figures 2–4), bringing the total number of patients to 90. Four main groups of abdominal tumors were identified: neuroblastoma, Wilms Tumor, liver tumors, and others such as germ cell tumors and myofibroblastic inflammatory tumors.
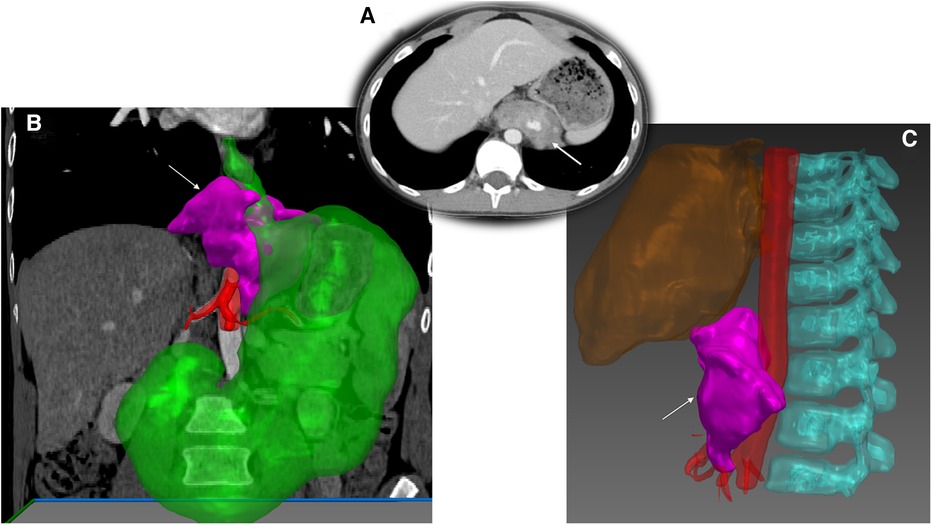
Figure 2. Abdominal inflammatory myofibroblastic tumor and 3D modelling. (A) Computed tomography acquisition of a retroperitoneal mass in a 12-year-old boy. (B) Segmentation of the tumor (arrow) using Medical Imaging Interaction Toolkit (MITK°-free open source software-German Cancer Research-Germany) showing close contact with abdominal aorta (C).
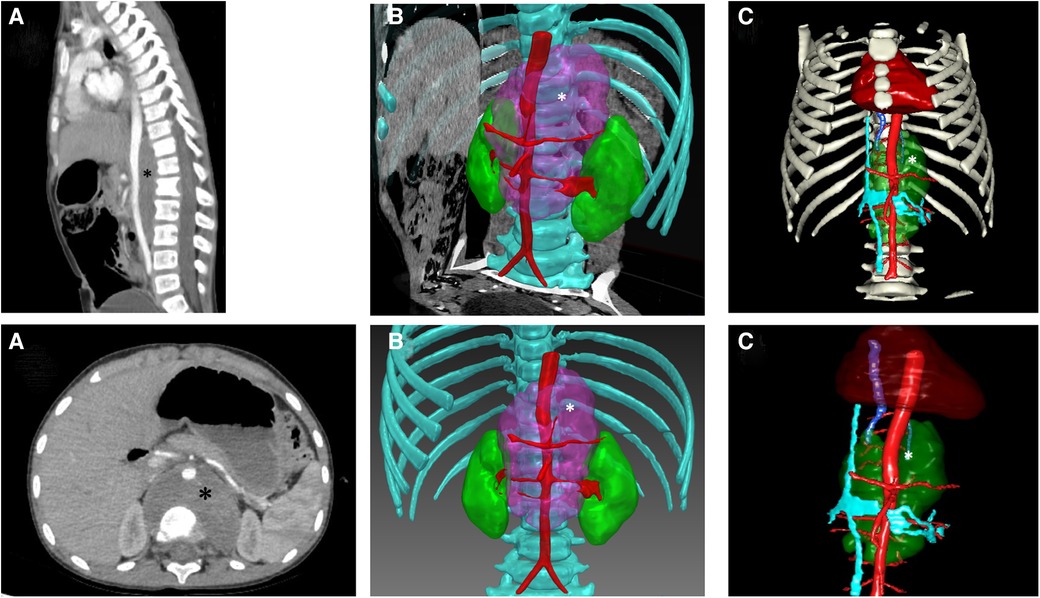
Figure 3. Abdominal neuroblastoma and 3D modelling. (A) Computed tomography acquisition of an abdominal median neuroblastoma (*) in a 4-year-old boy. (B) Segmentation of the tumor (*) using Medical Imaging Interaction Toolkit (MITK°-free open source software-German Cancer Research-Germany) located between vertebra and aorta. (C) Segmentation of the neuroblastoma (*) and surrounding structures by Visible Patient° software (Visible Patient°-Strasbourg-France).
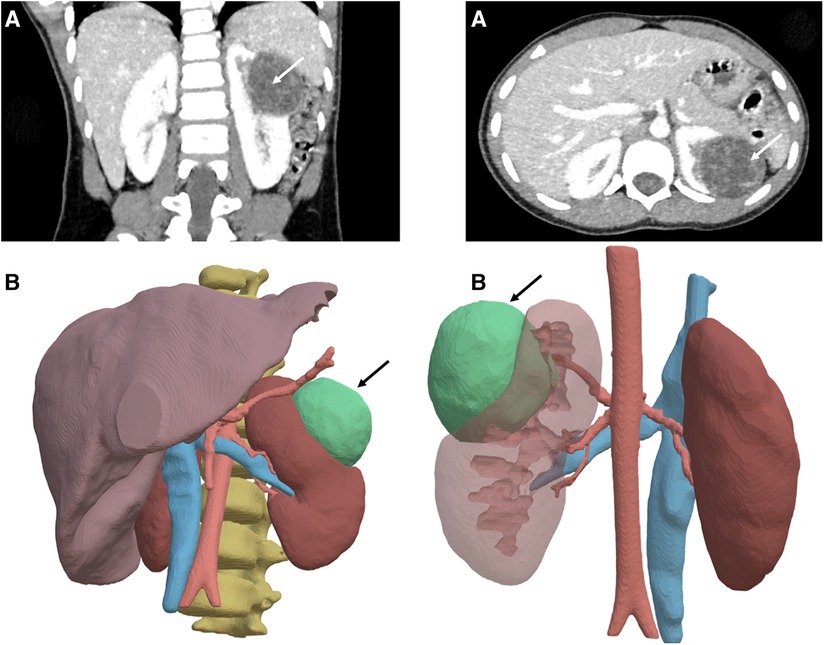
Figure 4. Left renal tumor and 3D modelling. (A) Computed tomography acquisition of a cystic nephroma in a 5-year-old boy with DICER1 syndrome. (B) Segmentation of the tumor (arrow) using semi-automatic methods in the IMAG2 laboratory-Paris-France.
All authors reached the consensus that the utilization of both virtual reconstruction technology and physical model printing proved to be beneficial tools for enhancing surgical planning and improving patient outcomes in cases of complex tumors. However, to date, there is a lack of evidence-based medicine arguments to substantiate these conclusions or to support the funding of such technologies and the selection of candidate patients.
Discussion
3D modelling
3D virtual reconstruction offers numerous applications, including monitoring tumor volume during chemotherapy (11), adjusting the target irradiation volume (23), and providing precise preoperative visualization of the tumor for the surgical team. This process can be performed “in situ” by radiologists, resulting in the projection of 3D images on the screen (9). The primary technology used for this propose is VolR, available with conventional software (24). It serves as an effective tool, as demonstrated in pelvic region analysis for adults, where it provides additional information beyond standard MRI (25). However, the limitation of this pseudo-3D technology lies in the lack of accurate tissue differentiation, such as distinguishing between normal tissue and tumors. It often necessitates cross-referencing with CT or MRI images (Figure 2).
A more comprehensive process is offered by engineering companies providing remote modeling services like “Visible Patient°” (11). Technicians delineate various anatomical structures and return files with reconstructed tumors and surrounding anatomical features. This service offers a precise vascularization map, providing valuable information about potential encasement of large vessels encasement that is crucial for surgical planning and the mental preparation of the surgical team. Figure 3 illustrates the segmentation of a median neuroblastoma with IDRFs.
Vessels can be delineated within the application, allowing identification of the feeding artery corresponding to the tumor. Simulations of vessel clamping with tissue ischemia can be performed. This technology has been reported to prevent cold intraoperative ischemia in adult patients (26) and can also estimate the resultant organ volume for hepatectomy (27). More recently, the IMAG2 laboratory (Imagine Institute, Necker Enfants Malades Hospital, APHP and Université Paris Cité-France) has developed semi-automatic segmentation methods, combining artificial intelligence methods (knowledge representation, spatial reasoning and deep learning) making it possible to obtain automatic 3D modeling of bones, bladder, colon, vessels, pelvic muscles (obturator, levator ani, piriformis), genital tract in adolescents (ovaries, vagina, uterus), and nerves in a few clicks. This methodology also applied to renal tumor CT scan images making it possible to quickly model 3D structures which cannot benefit from automatic learning due to their excessive inter-individual variations, like tumors (Figure 4) (24, 28, 29). All the aforementioned points contribute to the preoperative liver 3D evaluation being covered by universal health care insurance in Japan since 2012 (30).
Some masses are embryonal abdominal tumors and can be detected prenatally. Fetal MRI is more precise than sonography, and while T2 sequences are well-developed for placental research, they are not superficially outlined for fetal applications. Consequently, even though MRI is the most efficient tool for describing prenatal malformations and providing accurate anatomical delineation of cystic lesions (31), 3D fetal tumor reconstruction is not commonly performed.
3D printing
Physical model printing is an additional option after 3D reconstruction. Initially developed for bones tissues and prothesis implantation, it is now common in adult surgeries such as orthopedics (32). The first surgical 3D printing pediatric case involved a heart model before transplantation (33) and has since expanded to utilize multiple materials. In case of tissue lesions, segmentation allows for precise delineation from other soft tissues like vascular structures. It has been reported as useful in enhancing partial nephrectomy in adults (34), with volumetric precision sufficient for distinguishing between nephron sparing surgery or total nephrectomy (15, 19). Real-scale models can facilitate familial preoperative counseling and, from an educational perspective, can be manipulated by the entire surgical team to improve understanding of abdominal anatomy (35). Most importantly, it can be used for realistic surgical laparoscopic simulation (13) through the assembly of several semi- transparent materials.
The main limitations include its difficulty in use during the intraoperative process without the distracting intervention of a non-sterile person, the lack of reusability, its availability including printing duration (ranging from 4 h to 5 days), and its cost (ranging from $30 to $900) (36). Although a recent study proposed a recommendation guide for sterilization methods of 3D-printed materials (37), its advantages compared to 3D virtual reconstruction are expected to be proven with large series.
Augmented reality
AR involves integrating information about the surgical field into the surgeon's mind to assist with the procedure, while allowing the surgeon to maintain direct contact with the environment. On the other hand, VR remains a futuristic concept for open surgical processes, as it requires full immersion with a headset (Figure 5). To the best of our knowledge, this approach has not yet been published for pediatric patients, but it could have potential applications in open surgery. AR allows for overlaying digital information onto the physical real world, making it useful for surgical navigation (38) and education.
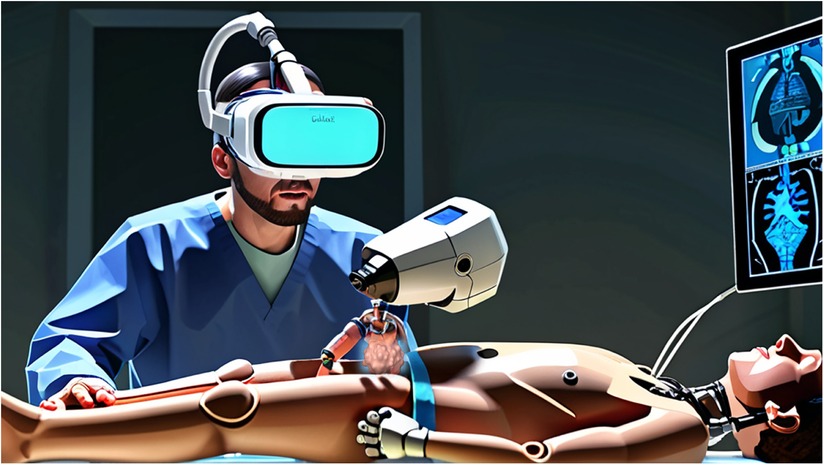
Figure 5. Augmented reality. Illustration of a potential operating room equipped with augmented reality for a pediatric surgery case in the future.
Before AR was generated by specific software, blending the surgical field with additional information was done intraoperatively by the surgeon's brain. Robotic gamma detection of neuroblastoma was reported for intraoperative resection support before the laparoscopic era (39). Some methods are now available using the laparoscopy screen. Fluorescence-guided surgery (4) using indocyanine green enhances perception of vascularization in the operative field, and a dedicated robotic interface for near-infrared fluorescence-guided surgery has been reported to be effective for partial nephrectomy and tumor excision guidance (40). Intraoperative use of an ultrasound probe has been reported for pancreatic surgery, providing both visual and ultrasound images (41) integrated into the robotic console. In a conference paper, Stafman et al. reported that intraoperative ultrasound guidance facilitates laparoscopic resection of smaller, non-visible tumors and optimizes negative margins (42). Others technologies like photodynamic therapy and near-infrared photoimmunotherapy show promising results (43). However, integrating these devices into standard laparoscopy seems less pertinent due to the lack of 3D visualization and precision of movements compared to robotic surgery. In the only case included in this review that was operated with the robot, AR was not available at the console.
Mixed reality combines AR and VR to create a new operating field (Figure 6). Its integration into the robotic console would allow blending anatomical structures and simulated digital elements like nervous plexi, tumor delineation, and vascularization, synchronized with respiratory movements. The concept of cybernetic surgery was first proposed in a robotic liver segmentectomy using augmented reality (44). Machine learning has been demonstrated to be relevant to human expertise for real-time anatomy segmentation during laparoscopy (45), particularly for nervous structures (46). All these supportive devices will enhance the level of assistance for the procedure. The next step will be the integration of artificial intelligence in near-real-time surgery (47), enabling semi-autonomous robotic surgery under human supervision.
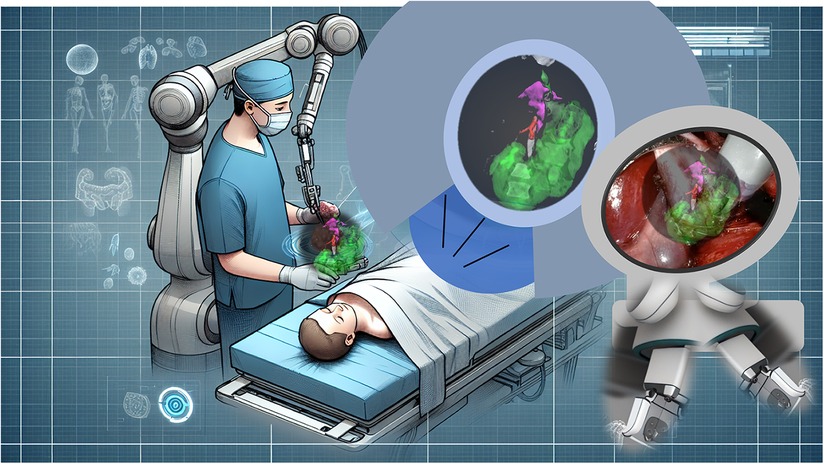
Figure 6. Mixed reality. Illustration depicting a potential scenario of mixed reality support for a pediatric surgery case in the future.
Conclusion
While there is currently no evidence to suggest that 3D technology offers a positive impact on clinical outcomes for pediatric oncology, it is widely recognized by most pediatric surgeons as a key future tool, especially when used in conjunction with dedicated small instruments and robotic platforms. In the near future, pediatric patients are expected to benefit from systematic surgical planning, which may include 3 modeling prior to minimally invasive resection procedures under mixed reality, facilitated by artificial intelligence algorithms.
Author contributions
PL: Data curation, Formal Analysis, Methodology, Software, Validation, Visualization, Writing – original draft, Writing – review & editing. AB: Data curation, Formal Analysis, Software, Validation, Visualization, Writing – review & editing. SS: Data curation, Software, Validation, Visualization, Writing – review & editing. AA: Data curation, Software, Validation, Writing – review & editing. JH: Data curation, Software, Validation, Visualization, Writing – review & editing. CP: Data curation, Methodology, Software, Validation, Visualization, Writing – review & editing. MB: Data curation, Software, Writing – review & editing. LF: Methodology, Software, Validation, Visualization, Writing – review & editing. TL: Data curation, Software, Validation, Writing – review & editing. QB: Data curation, Formal Analysis, Software, Validation, Visualization, Writing – review & editing, Conceptualization, Investigation, Methodology, Project administration, Supervision, Writing – original draft.
Funding
The author(s) declare that no financial support was received for the research, authorship, and/or publication of this article.
Acknowledgments
Special thanks to Dr Sophie Domingues-Montanari for editing the manuscript. Special thanks to Mr Xavier Caquot from Johnson and Johnson Medtech° company for his assistance of the French hospitals in the connecting process with segmentation tools, like Visible Patients°. Some images included in Figures 5 and 6 were AI generated using OpenAI. (2024). Chat GPT-4, Professional Version [Software]. Retrieved from https://openai.com/gpt-4.
Conflict of interest
The authors declare that the research was conducted in the absence of any commercial or financial relationships that could be construed as a potential conflict of interest.
Publisher's note
All claims expressed in this article are solely those of the authors and do not necessarily represent those of their affiliated organizations, or those of the publisher, the editors and the reviewers. Any product that may be evaluated in this article, or claim that may be made by its manufacturer, is not guaranteed or endorsed by the publisher.
References
1. Pio L, Sarnacki S. Editorial: innovative approaches in pediatric surgical oncology. Front Pediatr. (2022) 10:989822. doi: 10.3389/fped.2022.989822
2. Bhakta N, Liu Q, Ness KK, Baassiri M, Eissa H, Yeo F, et al. The cumulative burden of surviving childhood cancer: an initial report from the St Jude lifetime cohort study (SJLIFE). Lancet. (2017) 390(10112):2569–82. doi: 10.1016/S0140-6736(17)31610-0
3. Blanc T, Meignan P, Vinit N, Ballouhey Q, Pio L, Capito C, et al. Robotic surgery in pediatric oncology: lessons learned from the first 100 tumors—a nationwide experience. Ann Surg Oncol. (2022) 29(2):1315–26. doi: 10.1245/s10434-021-10777-6
4. Abdelhafeez A, Talbot L, Murphy AJ, Davidoff AM. Indocyanine green-guided pediatric tumor resection: approach, utility, and challenges. Front Pediatr. (2021) 9:689612. doi: 10.3389/fped.2021.689612
5. Günther P, Ley S, Tröger J, Witt O, Autschbach F, Holland-Cunz S, et al. 3D perfusion mapping and virtual surgical planning in the treatment of pediatric embryonal abdominal tumors. Eur J Pediatr Surg. (2008) 18(1):7–12. doi: 10.1055/s-2007-989374
6. Günther P, Tröger J, Holland-Cunz S, Behnisch W, Hinz U, Romero P, et al. Surgical complications in abdominal tumor surgery in children. Experiences at a single oncological center. Eur J Pediatr Surg. (2009) 19(5):297–303. doi: 10.1055/s-0029-1220681
7. Wake N, Nussbaum JE, Elias MI, Nikas CV, Bjurlin MA. 3D printing, augmented reality, and virtual reality for the assessment and management of kidney and prostate cancer: a systematic review. Urology. (2020) 143:20–32. doi: 10.1016/j.urology.2020.03.066
8. Parthasarathy J, Krishnamurthy R, Ostendorf A, Shinoka T. 3D printing with MRI in pediatric applications. J Magn Reson Imaging. (2020) 51(6):1641–58. doi: 10.1002/jmri.26870
9. Valls-Esteve A, Adell-Gómez N, Pasten A, Barber I, Munuera J, Krauel L. Exploring the potential of three-dimensional imaging, printing, and modeling in pediatric surgical oncology: a new era of precision surgery. Children (Basel). (2023) 10(5):832. doi: 10.3390/children10050832
10. Günther P, Schenk JP, Wunsch R, Tröger J, Waag KL. Abdominal tumours in children: 3-D visualisation and surgical planning. Eur J Pediatr Surg. (2004) 14(5):316–21. doi: 10.1055/s-2004-821042
11. Irtan S, Hervieux E, Boutroux H, Becmeur F, Ducou-le-Pointe H, Leverger G, et al. Preoperative 3D reconstruction images for paediatric tumours: advantages and drawbacks. Pediatr Blood Cancer. (2021) 68(1):e28670. doi: 10.1002/pbc.28670
12. Su L, Dong Q, Zhang H, Zhou X, Chen Y, Hao X, et al. Clinical application of a three-dimensional imaging technique in infants and young children with complex liver tumors. Pediatr Surg Int. (2016) 32(4):387–95. doi: 10.1007/s00383-016-3864-7
13. Souzaki R, Kinoshita Y, Ieiri S, Kawakubo N, Obata S, Jimbo T, et al. Preoperative surgical simulation of laparoscopic adrenalectomy for neuroblastoma using a three-dimensional printed model based on preoperative CT images. J Pediatr Surg. (2015) 50(12):2112–5. doi: 10.1016/j.jpedsurg.2015.08.037
14. Krauel L, Fenollosa F, Riaza L, Pérez M, Tarrado X, Morales A, et al. Use of 3D prototypes for complex surgical oncologic cases. World J Surg. (2016) 40(4):889–94. doi: 10.1007/s00268-015-3295-y
15. Sánchez-Sánchez Á, Girón-Vallejo Ó, Ruiz-Pruneda R, Fernandez-Ibieta M, García-Calderon D, Villamil V, et al. Three-dimensional printed model and virtual reconstruction: an extra tool for pediatric solid tumors surgery. European J Pediatr Surg Rep. (2018) 6(1):e70–e6. doi: 10.1055/s-0038-1672165
16. Tejo A, Castellví A, Lustig P, Fenollosa F, Uceda R, Buj I, et al. 3D printed surgical planning prototype manufactured by a hybrid multi-material 3D printer. IOP Conf Ser Mater Sci Eng. (2021) 1193:01211. doi: 10.1088/1757-899X/1193/1/012116
17. Tejo-Otero A, Fenollosa-Artés A, Uceda R, Castellvi-Fernandez A, Lustig-Gainza P, Valls-Esteve A, et al. 3D printed prototype of a complex neuroblastoma for preoperative surgical planning. Ann 3D Print Med. (2021) 2:100014. doi: 10.1016/j.stlm.2021.100014
18. Girón-Vallejo Ó, García-Calderón D, Ruiz-Pruneda R, Cabello-Laureano R, Doménech-Abellán E, Fuster-Soler JL, et al. Three-dimensional printed model of bilateral Wilms tumor: a useful tool for planning nephron sparing surgery. Pediatr Blood Cancer. (2018) 65(4). doi: 10.1002/pbc.26894
19. Wellens LM, Meulstee J, van de Ven CP, van Scheltinga CEJ T, Littooij AS, van den Heuvel-Eibrink MM, et al. Comparison of 3-dimensional and augmented reality kidney models with conventional imaging data in the preoperative assessment of children with Wilms tumors. JAMA Netw Open. (2019) 2(4):e192633. doi: 10.1001/jamanetworkopen.2019.2633
20. Souzaki R, Kinoshita Y, Ieiri S, Hayashida M, Koga Y, Shirabe K, et al. Three-dimensional liver model based on preoperative CT images as a tool to assist in surgical planning for hepatoblastoma in a child. Pediatr Surg Int. (2015) 31(6):593–6. doi: 10.1007/s00383-015-3709-9
21. Yang T, Tan T, Yang J, Pan J, Hu C, Li J, et al. The impact of using three-dimensional printed liver models for patient education. J Int Med Res. (2018) 46(4):1570–8. doi: 10.1177/0300060518755267
22. Tejo-Otero A, Lustig-Gainza P, Fenollosa-Artés F, Valls A, Krauel L, Buj-Corral I. 3D printed soft surgical planning prototype for a biliary tract rhabdomyosarcoma. J Mech Behav Biomed Mater. (2020) 109:103844. doi: 10.1016/j.jmbbm.2020.103844
23. Chen MJ, Leao CR, Simoes RCP, Belletti FS, Figueiredo MLS, Cypriano MS. Kidney-sparing whole abdominal irradiation in Wilms tumor: potential advantages of VMAT technique. Pediatr Blood Cancer. (2020) 67(5):e28223. doi: 10.1002/pbc.28223
24. Virzì A, Muller CO, Marret JB, Mille E, Berteloot L, Grévent D, et al. Comprehensive review of 3D segmentation software tools for MRI usable for pelvic surgery planning. J Digit Imaging. (2020) 33(1):99–110. doi: 10.1007/s10278-019-00239-7
25. Fan KS, Durnea C, Nygaard CC, Khalil M, Doumouchtsis SK. Three-dimensional volume rendering of pelvic floor anatomy with focus on fibroids in relation to the lower urogenital tract based on cross-sectional MRI images. J Med Syst. (2023) 47(1):62. doi: 10.1007/s10916-023-01947-y
26. Shao P, Li P, Xu Y, Cao Q, Ju X, Qin C, et al. Application of combined computed tomography arteriography, venography, and urography in laparoscopic partial nephrectomy with segmental artery clamping. Urology. (2014) 84(6):1361–5. doi: 10.1016/j.urology.2014.07.056
27. Hallet J, Gayet B, Tsung A, Wakabayashi G, Pessaux P, Group nICCoLLR. Systematic review of the use of pre-operative simulation and navigation for hepatectomy: current status and future perspectives. J Hepatobiliary Pancreat Sci. 2015;22(5):353–62. doi: 10.1002/jhbp.220
28. La Barbera G, Rouet L, Boussaid H, Lubet A, Kassir R, Sarnacki S, et al. Tubular structures segmentation of pediatric abdominal-visceral ceCT images with renal tumors: assessment, comparison and improvement. Med Image Anal. (2023) 90:102986. doi: 10.1016/j.media.2023.102986
29. Muller C, Mille E, Virzi A, Marret J, Peyrot Q, Delmonte A, et al. Integrating tractography in pelvic surgery: a proof of concept. J Pediatr Surg Case Rep. (2019) 48:101268. doi: 10.1016/j.epsc.2019.101268
30. Oshiro Y, Ohkohchi N. Three-dimensional liver surgery simulation: computer-assisted surgical planning with three-dimensional simulation software and three-dimensional printing. Tissue Eng Part A. (2017) 23(11–12):474–80. doi: 10.1089/ten.tea.2016.0528
31. Davidson JR, Uus A, Matthew J, Egloff AM, Deprez M, Yardley I, et al. Fetal body MRI and its application to fetal and neonatal treatment: an illustrative review. Lancet Child Adolesc Health. (2021) 5(6):447–58. doi: 10.1016/S2352-4642(20)30313-8
32. Martelli N, Serrano C, van den Brink H, Pineau J, Prognon P, Borget I, et al. Advantages and disadvantages of 3-dimensional printing in surgery: a systematic review. Surgery. (2016) 159(6):1485–500. doi: 10.1016/j.surg.2015.12.017
33. Sodian R, Weber S, Markert M, Loeff M, Lueth T, Weis FC, et al. Pediatric cardiac transplantation: three-dimensional printing of anatomic models for surgical planning of heart transplantation in patients with univentricular heart. J Thorac Cardiovasc Surg. (2008) 136(4):1098–9. doi: 10.1016/j.jtcvs.2008.03.055
34. Wake N, Bjurlin MA, Rostami P, Chandarana H, Huang WC. Three-dimensional printing and augmented reality: enhanced precision for robotic assisted partial nephrectomy. Urology. (2018) 116:227–8. doi: 10.1016/j.urology.2017.12.038
35. Yang T, Lin S, Tan T, Yang J, Pan J, Hu C, et al. Impact of 3D printing technology on comprehension of surgical anatomy of retroperitoneal tumor. World J Surg. (2018) 42(8):2339–43. doi: 10.1007/s00268-018-4489-x
36. Pereira HR, Barzegar M, Hamadelseed O, Esteve AV, Munuera J. 3D surgical planning of pediatric tumors: a review. Int J Comput Assist Radiol Surg. (2022) 17(4):805–16. doi: 10.1007/s11548-022-02557-8
37. Valls-Esteve A, Lustig-Gainza P, Adell-Gomez N, Tejo-Otero A, Englí-Rueda M, Julian-Alvarez E, et al. A state-of-the-art guide about the effects of sterilization processes on 3D-printed materials for surgical planning and medical applications: a comparative study. Int J Bioprint. (2023) 9(5):756. doi: 10.18063/ijb.756
38. Yeung AWK, Tosevska A, Klager E, Eibensteiner F, Laxar D, Stoyanov J, et al. Virtual and augmented reality applications in medicine: analysis of the scientific literature. J Med Internet Res. (2021) 23(2):e25499. doi: 10.2196/25499
39. Martelli H, Ricard M, Larroquet M, Wioland M, Paraf F, Fabre M, et al. Intraoperative localization of neuroblastoma in children with 123I- or 125I-radiolabeled metaiodobenzylguanidine. Surgery. (1998) 123(1):51–7. doi: 10.1016/S0039-6060(98)70228-4
40. Esposito C, Settimi A, Del Conte F, Cerulo M, Coppola V, Farina A, et al. Image-guided pediatric surgery using indocyanine green (ICG) fluorescence in laparoscopic and robotic surgery. Front Pediatr. (2020) 8:314. doi: 10.3389/fped.2020.00314
41. Schulte Am Esch J, Krüger M, Barthlen W, Förster C, Mohnike K, Empting S, et al. Technical aspects of paediatric robotic pancreatic enucleation based on a case of an insulinoma. Int J Med Robot. (2021) 17(6):e2317. doi: 10.1002/rcs.2317
42. Stafman L, Matthew L, Zamora I, Lovvorn H. Ultrasound-guided laparoscopic resection of pediatric solid tumors. ESPES and IPEG Conference; July 6th 2023; Sorrento Italia (2023).
43. Privitera L, Paraboschi I, Cross K, Giuliani S. Above and beyond robotic surgery and 3D modelling in paediatric cancer surgery. Front Pediatr. (2021) 9:777840. doi: 10.3389/fped.2021.777840
44. Pessaux P, Diana M, Soler L, Piardi T, Mutter D, Marescaux J. Towards cybernetic surgery: robotic and augmented reality-assisted liver segmentectomy. Langenbecks Arch Surg. (2015) 400(3):381–5. doi: 10.1007/s00423-014-1256-9
45. Kolbinger FR, Rinner FM, Jenke AC, Carstens M, Krell S, Leger S, et al. Anatomy segmentation in laparoscopic surgery: comparison of machine learning and human expertise—an experimental study. Int J Surg. (2023) 109(10):2962–74. doi: 10.1097/JS9.0000000000000595
46. Kitaguchi D, Harai Y, Kosugi N, Hayashi K, Kojima S, Ishikawa Y, et al. Artificial intelligence for the recognition of key anatomical structures in laparoscopic colorectal surgery. Br J Surg. (2023) 110(10):1355–8. doi: 10.1093/bjs/znad249
Keywords: pediatric oncology, three-dimensional printing, virtual reality, augmented reality, minimally invasive surgery
Citation: Lopez P, Belgacem A, Sarnacki S, Arnaud A, Houari J, Piguet C, Baudouin M, Fourcade L, Lauvray T and Ballouhey Q (2024) Enhancing surgical planning for abdominal tumors in children through advanced 3D visualization techniques: a systematic review of future prospects. Front. Pediatr. 12:1386280. doi: 10.3389/fped.2024.1386280
Received: 14 February 2024; Accepted: 26 April 2024;
Published: 7 May 2024.
Edited by:
Luca Pio, St. Jude Children’s Research Hospital, United StatesReviewed by:
Daniel Rhee, Johns Hopkins University, United StatesIllya Martynov, University of Marburg, Germany
© 2024 Lopez, Belgacem, Sarnacki, Arnaud, Houari, Piguet, Baudouin, Fourcade, Lauvray and Ballouhey. This is an open-access article distributed under the terms of the Creative Commons Attribution License (CC BY). The use, distribution or reproduction in other forums is permitted, provided the original author(s) and the copyright owner(s) are credited and that the original publication in this journal is cited, in accordance with accepted academic practice. No use, distribution or reproduction is permitted which does not comply with these terms.
*Correspondence: Quentin Ballouhey cS5iYWxsb3VoZXlAZ21haWwuY29t
†ORCID Quentin Ballouhey orcid.org/0000-0002-6817-9946