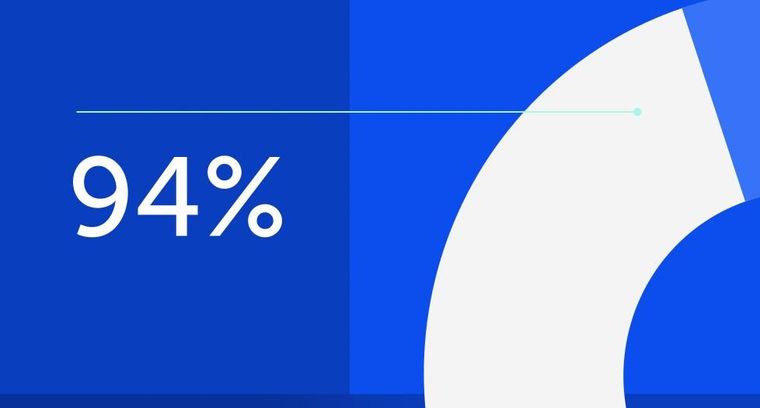
94% of researchers rate our articles as excellent or good
Learn more about the work of our research integrity team to safeguard the quality of each article we publish.
Find out more
ORIGINAL RESEARCH article
Front. Pediatr., 02 April 2024
Sec. Pediatric Surgery
Volume 12 - 2024 | https://doi.org/10.3389/fped.2024.1376196
Objective: This study was conducted to explore the risk factors for the prognosis and recurrence of ureteropelvic junction obstruction (UPJO).
Methods: The correlation of these variables with the prognosis and recurrence risks was analyzed by binary and multivariate logistic regression. Besides, a nomogram was constructed based on the multivariate logistic regression calculation. After the model was verified by the C-statistic, the ROC curve was plotted to evaluate the sensitivity of the model. Finally, the decision curve analysis (DCA) was conducted to estimate the clinical benefits and losses of intervention measures under a series of risk thresholds.
Results: Preoperative automated peritoneal dialysis (APD), preoperative urinary tract infection (UTI), preoperative renal parenchymal thickness (RPT), Mayo adhesive probability (MAP) score, and surgeon proficiency were the high-risk factors for the prognosis and recurrence of UPJO. In addition, a nomogram was constructed based on the above 5 variables. The area under the curve (AUC) was 0.8831 after self cross-validation, which validated that the specificity of the model was favorable.
Conclusion: The column chart constructed by five factors has good predictive ability for the prognosis and recurrence of UPJO, which may provide more reasonable guidance for the clinical diagnosis and treatment of this disease.
Ureteropelvic junction obstruction (UPJO) is the most common cause of congenital hydronephrosis. The prevalence of UPJO ranges from 1:1,500 to 1:500 among newborns, mainly affecting males (with a male-to-female ratio being 2:1) (1–3). Further, left involvement accounts for 60%, and bilateral involvement accounts for 10%–40%. The management of UPJO has posed a challenge for both pediatric and adult urologists. Dissected pyeloplasty for UPJO is considered one of the most common urological reconstruction interventions (4, 5).
However, the postoperative recurrence of UPJO has always been a thorny problem for clinicians. Braga et al. identified the recurrence rate (5.2%) of UPJO after various open surgical procedures in 2008 (6). According to the calculation of Ceyhan et al. in 2019, the recurrence probability of UPJO was 6.7% (7). In recent years, surgical techniques and instruments have been continuously improved (5), and the diagnosis and treatment of UPJO become more reasonable due to the continuous improvement of prenatal diagnosis with the aid of B-ultrasound (8) and the development of MR urography (MRU) (9). However, the postoperative recurrence of UPJO still exists and has not been significantly reduced. Further, there are fewer studies to explore the risk factors of postoperative recurrence of UPJO, which has not been fully explored in the medical circles at home and abroad.
Although Ceyhan (7) and Braga (6) included a sufficient sample size of UPJO, the risk factors associated with the postoperative recurrence after UPJO were not fully clarified. Both of them only conducted a simple controlled study based on clinical case cohorts. Besides, due to the less rigorous statistical methods in their studies, it was still difficult to predict the risks for the recurrence of UPJO to guide clinical diagnosis and treatment.
As a new statistical method to predict the prognosis of diseases in recent years, the nomogram can be used to evaluate the prognosis accurately. In addition, this tool contributes to preventing low-risk patients from unnecessary examinations in the decision-making process and avoiding delayed treatment for patients with a high probability to obtain favorable net benefits (10–12). The nomogram has been employed to predict the prognosis of patients with colorectal cancer (13), prostate cancer (14), and multiple myeloma (15). In addition, some investigators also adopted deep learning (DL) algorithms (16) to predict the recurrence risks of UPJO after surgery. Moreover, some investigators also constructed a clinical prediction model for the reoperation of UPJO after surgery (17). In this study, the prognosis of patients with a surgical history for UPJO was evaluated based on such variables as anteroposterior diameter (APD) of the renal pelvis, preoperative renal parenchymal thickness (RPT), and surgical methods, thus predicting the recurrence risk of UPJO after surgery.
This study aimed to incorporate more risk factors that may be associated with the recurrence of UPJO after surgery and conduct relevant explorations. Meanwhile, a clinical prediction model for predicting the recurrence probability of UPJO after surgery was established based on the APD of the renal pelvis, preoperative RPT, surgical methods, and other variables with the aid of various mature and reliable statistical methods. Moreover, this prediction model could be applied to patients with a surgical history for UPJO to predict the recurrence risk after surgery. Furthermore, these efforts are expected to establish a systematic diagnosis and treatment system for the prognosis and recurrence of UPJO, thus reducing the recurrence risks of UPJO after surgery in clinical practice.
This study was approved by the Academic Research Ethics Committee of Shandong University, and the clinical privacy of patients was fully protected from disclosure. In this study, pediatric patients with UPJO who received surgical treatment (open pyeloplasty, laparoscopic pyeloplasty, and robot-assisted pyeloplasty) in the Pediatric Surgery Department of Qilu Hospital of Shandong University from January 2005 to December 2022 were retrieved from the Lianzhong Medical Database of Qilu Hospital of Shandong University as per the names of attending physicians (SUN Fengyin, LI Aiwu, CUI Xinhai, and DONG Zhixing). Eventually, a total of 890 patients with UPJO were identified from January 2005 to December 2022. During the follow-up, the aggravation of collective system separation revealed by CT urography (CTU) and MR urography (MRU) or the aggravation of nephron destruction compared with preoperative conditions revealed by emission computed tomography (ECT) was found in 57 patients. Based on that, a retrospective analysis was conducted (Figure 1). Meanwhile, antibiotics and analgesics were not routinely administered in all patients before and after surgery, and ureteral stents were routinely removed under general anesthesia 6–8 weeks after surgery. This study was designed and implemented in strict accordance with the Transparent Reporting of a Multivariable Prediction Model for Individual Prognosis or Diagnosis (TRIPOD) Statement (18). According to the Event per Variable (EPV) criteria and sample size guidelines for logistic regression of observational studies, a minimum sample size of 800 patients was required (19). The exclusion criteria included: (1) patients without other congenital malformations of the urinary system, such as horseshoe kidney, duplicate kidney, and double ureter; (2) patients without other chronic diseases unrelated to this disease (excluding hypertension, renal injury, and preoperative UTI); (3) patients with incomplete clinical data or a loss to follow-up. According to the exclusion criteria, 8 patients with secondary conditions, 8 patients with horseshoe kidney, and 22 patients with a loss to follow-up were excluded. Statistical results were expressed based on two patterns, namely “recurrence” and “no recurrence”. Specifically, recurrence indicated that the patient received a second surgical procedure except for ureteral stent removal (salvage pyeloplasty performed with the above three different approaches). Non-recurrence indicated that the patient did not undergo any additional surgery related to the urinary system (such as balloon dilatation, ureteral stent implantation, laser intrapelvic pyeloplasty, or other repetitive pyeloplasty) within 30 months after the initial operation.
The clinical characteristics of these patients for the evaluation were classified into the following three categories. (1) Individual factors of patients included gender, age (3 grades: 0–1 years old, 1–4 years old, and >4 years old), body weight (4 grades: 0–10 kg, 10–30 kg, 30–50 kg, and >50 kg), initial operation age (3 grades: 0–1 years old, 1–4 years old, and >4 years old), left or right involvement, unilateral or bilateral involvement, preoperative APD of the affected renal pelvis (4 grades: 0–20 mm, 20–40 mm, 40–60 mm, and >60 mm), preoperative international Grignon grading, RPT (3 grades: 0–0.5 cm, 0.5–1 cm, and >1 cm), stenosis mode (stenosis renal pelvis, vascular compression renal pelvis, renal pelvis polyp, or intrarenal renal pelvis), APD differences compared with the contralateral side (3 grades: 0%–10%, 10%–20%, 30%–40%, and >40%), the presence or absence of UVJO, and symptoms (abdominal pain, hematuria, and stones). (2) UTI-related factors included preoperative ureteral width (with 7 mm as the division), circumcision for male pediatric patients (Yes/No), preoperative UTI (Yes/No), preoperative intraudio videoenous urography (IVU), postoperative UTI (except for positive bacterial culture results, the colony counting should be >105 CFU/ml for the bacterial culture of the urinary tract of asymptomatic patients; If the patient presented with symptoms, the colony counting can be >104 CFU/ml; If the urine culture was performed through bladder puncture catheterization, the colony counting can be >103 CFU/ml). (3) Technical factors included preoperative diagnosis (Yes/No), surgical methods (laparoscopic pyeloplasty, open pyeloplasty, and robot-assisted pyeloplasty), double J implantation or nephrostomy (Yes/No), MAP score, surgical time (3 grades 0–120 min, 120–180 min, and >180 min), intraoperative blood loss (3 grades: 0–50 ml, 50–150 ml, and >150 ml), postoperative analgesia (Yes/No), postoperative complications (except for UTI), postoperative drainage (3 grades: 0–50 ml, 50–100 ml, and >100 ml), postoperative urinary fistula, surgeon proficiency (with the lower limit being 50 cases for laparoscopic pyeloplasty and open pyeloplasty and 31 cases for robot-assisted pyeloplasty). In this study, patients were divided into two categories by the model, namely “recurrent” and “non-recurrent” patients. Recurrence indicated that the patient received a second surgical procedure except for ureteral stent removal. Non-recurrence indicated that the patient did not undergo any additional surgery related to the urinary system within 30 months after the initial operation. The nomogram was constructed based on these two categories.
The process flow of this study is shown in Figure 1. In the first step, relevant data were collected. In the process of data collection, unnecessary data were deleted in strict accordance with the above standards. In the second step, the collected data were collated according to the variables that were assumed to be related to UPJO recurrence, so that these data can be used for the subsequent statistical calculation. In the third step, the rms (6.4.0) and ResourceSelection (0.3–5) packages in R (4.2.1) were used for binary and multivariate logistic regression analyses. In the processing process, data cleaning was carried out first; Then, the glm function was used to screen variables by single-factor binary logistic regression. Next, the multivariate binary logistic regression was conducted, and the model correlation test was performed. In terms of the variable screening strategy, the single-factor sample would be included in the multi-factor model if it met the p-value threshold (<0.05). Eventually, the risk factors with the most significant correlation with UPJO recurrence were screened out. After data cleaning, the binary logistic model was constructed with the aid of the glm function. Moreover, the rms package was employed to construct and visualize nomogram-related models. As a result, a nomogram based on 5 risk factors related to UPJO recurrence was constructed. In the fourth step, the Bootstrap sampling method was adopted. First, the data (S) of 852 samples from the overall sample were obtained. Then, these 852 original sample data were subjected to sampling with replacement to obtain a sample with a size of 100, which was repeated 1,000 times. The sample in each sampling was called a Bootstrap sample, and a total of 1,000 Bootstrap samples were obtained. After that, the statistics of each Bootstrap sample were estimated, and 1,000 statistics in total were obtained. Finally, the sampling distribution was constructed based on these 1,000 Bootstrap statistics. The ROC analysis of these data was performed using the pROC (1.18.0) package, and the results were visualized using ggplot2 (3.3.6). Among them, the pROC package could correct the ending order of data by default (ensuring that the result was convex upwards). Besides, the 95% confidence interval (CI) was set, and 2.5% of quantiles were taken at both ends of the sorted sampling distribution, thus completing the confidence interval estimation of the overall median. In the fifth step, the binary classification model and survival model were fitted with the logistic model and logistic-LASSO (least absolute shrinkage and selection operator) model, respectively. The leave-one-out (LOO) risk score was calculated over a range of model complexity parameters (lambda λ). The lambda values with the highest AUROC and consistency, respectively, were selected for the construction of the final model. The bootstrap sampling on the empirical percentile (1,000 times of sampling) was utilized to infer the point estimation. Additionally, parametric reasoning of model coefficients was performed through selective inference (SI) designed based on the LASSO model. The binary Logistic model was constructed with the aid of the glm function. Moreover, the rms package was adopted to perform calibration analyses and visualization. Meanwhile, the glm function was employed to construct a binary Logistic model, and the rmda package was utilized to calculate the corresponding net return rate and perform visualization.
A total of 852 patients with UPJO who underwent dissected pyeloplasty over an established period were explored. Among them, 57 patients underwent a second pyeloplasty after surgery, and the median time from the recurrence to the initial operation was 17 months. Among these 852 patients, the median age of patients at their initial operation was 40 months. Among them, there were 144 (17%) female patients; The average body weight was 16.4 kg. Besides, there were 465 (54%) patients with left involvement and 537 (63%) patients with unilateral involvement. The average APD before surgery was 3.16 cm. The median GRI grade was rated as 3 before surgery. The average RPT measured by preoperative imaging was 1.15 cm. The average surgical time was 73.27 min and the average blood loss was 84.58 ml. There were 555, 64, and 233 patients undergoing laparoscopic pyeloplasty, robot-assisted pyeloplasty, and open pyeloplasty, respectively. All data were evenly distributed.
The data of 852 patients were included, and most of the included variables were evenly distributed. The binary logistic model was constructed to select variables. As a result, 36 variables were initially screened, of which 5 variables were reserved for the construction of the prediction model (Table 1; Figure 2). Based on these 5 variables, the prediction model was established with the assistance of the logistic regression equation. The parameters of the ROC curve at the best cut-off value in different models were recorded. The results demonstrated that the AUC of the model was 0.883, which exhibited high sensitivity and specificity (Figure 3). In addition, the calibration curve revealed that the fitting degree of the model was high (Figure 4).
Figure 2. Predicting nomogram of postoperative recurrence in UPJO through preapd, preuti, PT, MAP, and proficiency. Odds represents the probability of UPJO prognosis recurrence corresponding to the obtained score. Patient prognosis values are located on the axis of each variable; Then draw a line upwards at a 90 angle to determine the number of points for that specific variable. The sum of these numbers is located on the total score axis and plotted downwards at a 90° angle along the UPJO prognostic recurrence risk axis to determine the likelihood of UPJO prognostic recurrence.
Figure 3. The ROC curve obtained through internal validation after establishing the model. The value of AUC in the figure indicates that the model has good diagnostic ability.
Figure 4. The calibration curves for the nomogram. The x-axis represents the nomogram-predicted probability and y-axis represents the actual probability of recurrence of UPJO. Perfect prediction would correspond to the 45°dashed line (Ideal). The Apparent line represents the entire cohort (n ¼ 852), and the blue solid line is bias-corrected by bootstrapping (B ¼ 1,000 repetitions), indicating observed nomogram performance.
Finally, 5 predictive factors were selected as the prognostic characteristics of the nomogram (Figure 2), including preoperative APD, preoperative UTI, preoperative RPT, MAP score, and surgeon proficiency. Based on the nomogram, patients can roughly estimate the risk of secondary surgery in a treatment evaluation program. This nomogram can be used to predict the individualized risk for the recurrence of UPJO after surgery.
The DCA results confirmed that the net benefit of the prediction model was improved compared with the default strategy. In the default strategy, it was assumed that all or no patients among these 852 patients needed centralized interventions (Figure 5). The DCA results were also verified by transforming net benefits into reduced interventions per 100 patients. As shown in the DCA diagram, the clinical strategy based on the nomogram would reduce the number of unnecessary interventions with a wide range of threshold probabilities in the training set and the test set.
Figure 5. Decision curve analysis for predicting prognosis recurrence of UPJO based on nomograms. The figure represents the decision benefits.
The postoperative recurrence of ureteropelvic junction obstruction (UPJO) has always been a thorny problem for clinicians. Braga analyzed the recurrence rate (5.2%) of UPJO and proposed that not performing retrograde pyelography or selecting the lumbar dorsal incision in open pyeloplasty was independently associated with the high risk of UPJO recurrence (6). Ceyhan et al. confirmed that the recurrence rate of UPJO and the incidence of complications were 6.7% and 11.4%, respectively. Urinary tract infection (UTI) (7.8%), complications associated with urinary diversion (1.8%), and urethral polyps (1.4%) are the most common complications. Preoperative shunt (P = 0.020) and early complications after pyeloplasty (P < 0.001) are significantly associated with the recurrence of UPJO (7). As revealed in previous studies, the overall postoperative recurrence rate of UPJO is about 5%–10% (20–22). However, the postoperative recurrence of UPJO still exists and has not been significantly reduced.
Besides, there are significant differences in the risk factors for the recurrence of UPJO after surgery between different reports. It has been reported that vascular compression and tortuous stenosis of the proximal ureter are also the causes of UPJO recurrence after surgery (23). However, after the literature on the salvage surgery for UPJO was reviewed, dense fibrous tissues and scarring around anastomosis were recognized as the main causes of UPJO recurrence (24–30). Besides, there were incomplete indicators in previous studies. The main risk factors included urinary fistula, inappropriate conditions at the anastomotic stoma, anastomotic stoma, scar hyperplasia, iatrogenic valve, anastomotic adhesion, non-absorption of silk thread, high ureteral anastomosis and so on. Meanwhile, in the research on other aspects of UPJO, some investigators selected the age at the initial operation, BMI, gender, unilateral or bilateral involvement, and left or right involvement as the basic evaluation indicators for patients who did not achieve favorable outcomes in the initial operation (31, 32). However, Lim et al. reported that the age at the initial operation was a factor affecting the surgical outcome (33). Both Braga and Ceyhan reported that age was not associated with the surgical outcome. The results of this study demonstrated that the age at the initial operation was not significantly related to the prognosis and recurrence of UPJO. WENBIN FU also mentioned that calculus, a complication of UPJO, may be a risk factor for the prognosis and recurrence of this disease. Silay MS et al. maintained that UVJO exerted certain impacts on postoperative remission of UPJO (34). In this study, the risk factors related to the prognosis and recurrence of UPJO were obtained based on the above reports and the research on salvage surgery for recurrent UPJO. Additionally, these authors only adopted the statistical method of cohort studies to explore the risk factors for the prognosis and recurrence of UPJO. They did not apply systematic statistical methods, nor did they carry out verification. Therefore, more rigorous, systematic, and convincing statistical methods, such as clinical prediction models, were employed to analyze all statistical indicators. Under this circumstance, the indicators related to UPJO recurrence can be explored more comprehensively, and a more practical clinical prediction model was also established.
In recent years, clinical prediction models have been used in clinical research in the form of nomograms (35). In addition to the above application in clinical cases, Ruo-Yang Chen, Jie Wu, Yu-xiang Song and other investigators also applied nomograms to clinical research (36–38), and reliable clinical prediction models were also constructed. In this study, the data of 852 patients with UPJO were collected to construct a clinical prediction model for predicting the postoperative recurrence of UPJO. Besides, 5 risk factors for the prognosis and recurrence of UPJO were screened by single-factor logistic regression and multivariate regression analyses, including APD of the renal pelvis, RPT, MAP score, preoperative UTI, and surgeon proficiency. In addition, a nomogram was constructed based on the multivariate logistic regression analysis results. Moreover, the ROC curve was plotted to verify the discriminability of the model, with the AUC of this model being 0.883. All the combinations of sensitivity and specificity of the whole probability range were included in the AUC calculation. The calculation results indicated that the model had favorable discriminability. Furthermore, a calibration curve was plotted to evaluate the fitting degree of the model, and it was found that the model had a high fitting degree. This suggested that there was no significant systematic difference between the data after internal sampling and those in the clinical prediction model. Such common calibration errors were not observed. The above results demonstrated that the model had high clinical application value.
Jiayi Li et al. (17) performed univariate and multivariate logistic analyses and maintained that patient weight, preoperative APD of the renal pelvis, and difficulty in ureteral D-J stent implantation were independent risk factors for surgical failure. They constructed a clinical prediction model with high diagnostic specificity and high fitting degree. The APD of the renal pelvis was also selected as a risk factor for the prognosis and recurrence of UPJO in their study. However, the difference lay in that it was concluded in our study that the body weight of children may not be an independent factor directly affecting the effectiveness of surgery. The weight gain with the growth and development of children and the thickening of the perirenal fascia were the factors that may directly affect the postoperative recurrence of UPJO. Additionally, the MAP score commonly used in adult urology was also adopted to perform quantitative analyses, thus more intuitively reflecting that weight gain brought more difficulties to the surgical treatment of UPJO. Erik Drysdale et al. (16) adopted AI (deep learning) to identify the risk of UPJO recurrence after dissected pyeloplasty. They found that APD and renal parenchyma function before and after surgery were positively correlated with the recurrence of UPJO after surgery, and they are independent risk factors for UPJO recurrence after dissected pyeloplasty. Their findings were consistent with our results.
The RPT can directly reflect the degree of renal compression and the severity of renal injury. Josefin Nordenstrom et al. confirmed that the severity of RPT damage was an independent risk factor for the fetus to receive surgical treatment after birth (39). This may explain the view of this study that the severity of renal injury in children was closely related to a second operation. The results revealed that preoperative UTI was a risk factor for the recurrence of UPJO. Meanwhile, other researchers also proposed that infection was an important reason for the failure of the initial operation of UPJO (40, 41), which may be related to anastomotic adhesion caused by infection (42). However, it was also confirmed that the administration of antibiotics (43, 44) was not effective in preventing UTI after the surgical treatment of UPJO. Therefore, further exploration is required to identify whether antibiotics should be routinely used to control infection before surgery. However, some researchers also confirmed that infection was only related to the ureteral width (7 mm) (45).
As revealed in several studies (46–53), open pyeloplasty, laparoscopic pyeloplasty, and robot-assisted laparoscopic pyeloplasty may generate different curative effects. Meanwhile, the proficiency of surgeons was also one of the factors affecting the curative effects. Compared with conventional surgery, the other two surgical methods have certain requirements for the proficiency of surgeons. The consensus published by European Association of Urology (EAU) showed that surgeons could proficiently perform laparoscopic pyeloplasty after implementing the surgery for 50 cases. Niklas Pakkasjärvi (46) found that surgeons were adept at robotic-assisted laparoscopic pyeloplasty after implementing the surgery for 31 cases. This suggested that surgeon proficiency in both procedures should also be regarded as a risk factor for the prognosis and recurrence of UPJO (54, 55). In this study, these two thresholds were selected as a division to identify the proficiency of surgeons.
The recurrence risk of patients with UPJO can be obtained by evaluating the APD of the renal pelvis, RPT, MAP score, preoperative UTI, and surgeon proficiency. As illustrated in the model-related decision curve, the recurrence risks of UPJO are related to the overall clinical benefits and losses of interventions, which further highlights that the model can more effectively predict the risks or benefits of readmission for patients with UPJO. Meanwhile, it is also proved that the model can improve the benefits of patients and reduce the loss of patients after the actual clinical intervention. This contributes to obtaining more benefits in clinical diagnosis and treatment, surgical procedures, surgical timing, and surgical mode improvement when applying this model in practice. With the assistance of this model, patients can be provided with a more individualized diagnosis and treatment regimen, which may affect decision-making. Moreover, this may also reduce unnecessary examinations and treatment procedures, which would further reduce the treatment costs, thus exerting far-reaching social impacts. In clinical practice, it can be maintained that the average cost for the re-admission of patients may be reduced based on this model, and the losses and expected benefits of patients may be calculated more accurately. On that basis, a more feasible prediction model may be constructed for clinical practice. Nevertheless, only internal sampling verification has been performed in this study, and external verification is not conducted. Hence, the clinical practicability of the model has not been further verified. It is necessary to conduct external verification in subsequent clinical studies to verify the clinical practicability of this model.
The original contributions presented in the study are included in the article/Supplementary Material, further inquiries can be directed to the corresponding author.
The studies involving humans were approved by Ethics committee of Qilu Hospital of Shandong University. The studies were conducted in accordance with the local legislation and institutional requirements. Written informed consent for participation in this study was provided by the participants’ legal guardians/next of kin.
WM: Writing – original draft. HG: Writing – original draft. MC: Writing – review & editing, Data curation. ZL: Writing – review & editing, Writing – original draft, Data curation. DL: Writing – review & editing, Formal Analysis. CD: Writing – review & editing, Formal Analysis. DB: Writing – review & editing. FS: Writing – review & editing, Supervision, Project administration, Formal Analysis, Conceptualization.
The author(s) declare that no financial support was received for the research, authorship, and/or publication of this article.
The authors declare that the research was conducted in the absence of any commercial or financial relationships that could be construed as a potential conflict of interest.
All claims expressed in this article are solely those of the authors and do not necessarily represent those of their affiliated organizations, or those of the publisher, the editors and the reviewers. Any product that may be evaluated in this article, or claim that may be made by its manufacturer, is not guaranteed or endorsed by the publisher.
1. Tan BJ, Smith AD. Ureteropelvic junction obstruction repair: when, how, what? Curr Opin Urol. (2004) 14:55–9. doi: 10.1097/00042307-200403000-00002
2. Khawaja AR, Dar TI, Bashir F, Sharma A, Tyagi V, Bazaz MS. Stentless laparoscopic pyeloplasty: a single center experience. Urol Ann. (2014) 6:202–7. doi: 10.4103/0974-7796.134258
3. Mendrek M, Vögeli TA, Bach C. Recent advances in urologic surgical techniques for pyeloplasty. F1000Res. (2019) 8:F1000 Faculty Rev-295. doi: 10.12688/f1000research.15866.1
4. Halachmi S, Pillar G. Congenital urological anomalies diagnosed in adulthood—management considerations. J Pediatr Urol. (2008) 4:2–7. doi: 10.1016/j.jpurol.2007.03.001
5. Nerli RB, Reddy M, Prabha P, Ashish Koura A, Praveen Patne P, Ganesh MK. Complications of laparoscopic pyeloplasty in children. Pediatr Surg Int. (2009) 25:343–7. doi: 10.1007/s00383-009-2341-y
6. Braga LHP, Lorenzo AJ, Bägli DJ, Keays M, Farhat WA, Khoury AE, et al. Risk factors for recurrent ureteropelvic JunctionObstruction after open pyeloplasty in a large pediatric cohort. J Urol. (2008) 180:1684–7. discussion 1687-8. doi: 10.1016/j.juro.2008.03.086
7. Ceyhan E, Ileri F, Aydin CT, Dogan AM, Tekgul S. Predictors of recurrence and complications in pediatrics pyeloplasty pediatric U. Urology. (2019) 126:187–91. doi: 10.1016/j.urology.2019.01.014
8. Li B, McGrath M, Farrokhyar F, Braga LH. Ultrasound-based scoring systemfor indication of pyeloplasty in patients with UPJO-like hydronephrosis. Front Pediatr. (2020) 8:353. doi: 10.3389/fped.2020.00353
9. Scalabre A, Clément Y, Guillière F, Ayciriex S, Gaillard S, Demède D, et al. Early detection of ureteropelvic junction obstruction in neonates with prenatal diagnosis of renal pelvis dilatation using 1 H NMR urinary metabolomic. Sci Rep. (2022) 12:13406. doi: 10.1038/s41598-022-17664-4
10. Alba AC, Agoritsas T, Walsh M, Hanna S, Iorio A, Devereaux PJ, et al. Discrimination and calibration of clinical prediction models: users’ guides to the medical literature. JAMA. (2017) 318:1377–84. doi: 10.1001/jama.2017.12126
11. Diamond GA. What price perfection? Calibration and discrimination of clinical prediction models. J Clin Epidemiol. (1992) 45:85–9. doi: 10.1016/0895-4356(92)90192-p
12. Walsh CG, Sharman K, Hripcsak G. Beyond discrimination: a comparison of calibration methods and clinical usefulness of predictive models of readmission risk. J Biomed Inform. (2017) 76:9–18. doi: 10.1016/j.jbi.2017.10.008
13. Yu C, Zhang Y. Establishment of prognostic nomogram for elderly colorectal cancer patients: a SEER database analysis. BMC gastroenterol. (2020) 20:347. doi: 10.1186/s12876-020-01464-z
14. Rocco B, Sighinolfi MC, Sandri M, Puliatti S, Bianchi G. A novel nomogram for predicting ECE of prostate cancer. BJU Int. (2018) 122:916–8. doi: 10.1111/bju.14503
15. Huang HY, Wang Y, Wang WD, Wei XL, Gale RP, Li JY, et al. A prognostic survival model based on metabolism-related gene expression in plasma cell myeloma. Leukemia. (2021) 35:3212–22. doi: 10.1038/s41375-021-01206-4
16. Drysdale E, Khondker A, Kim JK, Kwong JCC, Erdman L. Personalized application of machine learning algorithms to identify pediatric patients at risk for recurrent ureteropelvic junction obstruction after dismembered pyeloplasty. World J Urol. (2022) 40:593–9. doi: 10.1007/s00345-021-03879-z
17. Li J, Li Z, He Y, Fan S, Liu P. Development of the prediction model for negative outcomes after primary laparoscopic pyeloplasty in children: a retrospective study of 535 patients. Transl Androl Urol. (2022) 11:1680–90. doi: 10.21037/tau-22-327
18. Collins GS, Reitsma JB, Altman DG, Moons KG. Transparent reporting of a MUltivariable prediction model for individual prognosis or diagnosis (TRIPOD): the TRIPOD statement. Br Med J. (2015) 350:g7594. doi: 10.1136/bmj.g7594
19. Bujang MA, Sa’at N, Sidik TMITAB, Joo LC. Sample size guidelines for logistic regression from observational studies with large population: emphasis on the accuracy between statistics and parameters based on real life clinical data malays. JMed Sci. (2018) 25:122–30. doi: 10.21315/mjms2018.25.4.12
20. Salem YH, Majd M, Rushton HG, Belman AB. Outcome analysis of pediatric pyeloplasty as a function of patient age, presentation and differential renal function. J Urol. (1995) 154:1889–93. doi: 10.1016/S0022-5347(01)66819-8
21. Seixas-Mikelus SA, Jenkins LC, Williot P, Greenfield SP. Pediatricpyeloplasty: comparison of literature meta-analysis of laparoscopic and open techniques with open surgery at a single institution. J Urol Nov. (2009) 182:2428–32. doi: 10.1016/j.juro.2009.07.051
22. Braga LH, Lorenzo AJ, Skeldon S, Dave S, Bagli DJ, Khoury AE, et al. Failed pyeloplasty in children: comparative analysis of retrograde endopyelotomy versus redo pyeloplasty. J Urol. (2007) 178:2571–5. doi: 10.1016/j.juro.2007.08.050
23. Fu W, Chen H, Ren C, Zhao J. Transabdominal laparoscopy and ureteroscopy one-stage surgery in the treatment of bilateral ureteropelvic junction obstruction combined with bilateral renal stones: a case report. Exp Ther Med. (2022) 24:450. doi: 10.3892/etm.2022.11377
24. Kavoussi LR, Meretyk S, Dierks SM, Bigg SW, Gup DI, Clayman R, et al. Endopyelotomy for secondary ureteropelvic junction obstruction in children. J Urol. (1991) 145:345–9. doi: 10.1016/S0022-5347(17)38335-0
25. Demirko MK, Barut O, Şahinkanat T, Rosenzweig B, Zilberman DE. Laparoscopic pyeloplasty in ureteropelvic junction obstruction: a single-center experience. Laparosc Endosc Surg Sci. (2021) 28:24–8. doi: 10.14744/less.2021.58219
26. Baek M, Silay MS, Au JK, Huang GO, Elizondo RA, Puttmann K, et al. Quantifying the additional difficulty of pediatric robot-assisted laparoscopic re-do pyeloplasty: a comparison of primary and Re-do procedures. J Laparoendosc Adv Surg Tech A. (2018) 28(5):610–6. doi: 10.1089/lap.2016.0691
27. Rohrmann D, Snyder HM 3rd, Duckett JW Jr, Canning DA, Zderic SA. The operative management of recurrent ureteropelvic junction obstruction. J Urol Sep. (1997) 158(3 Pt 2):1257–9. doi: 10.1016/S0022-5347(01)64446-X
28. Faerber GJ, Ritchey ML, Bloom DA. Percutaneous endopyelotomy in infants and young children after failed open pyeloplasty. J Urol. (1995) 154:1495–7. doi: 10.1016/S0022-5347(01)66912-X
29. Cromie W. Complications of pyeloplasty. Urol Clin N Am. (1983) 10:385–97. doi: 10.1016/S0094-0143(21)01701-8
30. Williams DI, Kenawi M. The prognosis of pelviureteric obstruction in childhood: a review of 190 cases. Eur Urol. (1976) 2:57–63. doi: 10.1159/000471963
31. Zhang Y, Ouyang W, Xu H, Luan Y, Yang J, Lu YC, et al. Secondary management for recurrent ureteropelvic junction obstruction after pyeloplasty: a comparison of Re-do robot-assisted laparoscopic pyeloplasty and conventional laparoscopic pyeloplasty. Urol Int. (2019) 103:466–72. doi: 10.1159/000503156
32. Powell C, Gatti JM, Juang D, Murphy JPL. Aparoscopic pyeloplasty for ureteropelvic junction obstruction following open pyeloplasty in children. J Laparoendosc Adv Surg Tech A. (2015) 25:858–63. doi: 10.1089/lap.2015.0074
33. Lim DJ, Walker RDI. Management of the failed pyeloplasty. J Urol. (1996) 156(2 Pt 2):738–40. doi: 10.1016/S0022-5347(01)65801-4
34. Silay MS, Turan T, Kayalı Y, Başıbüyük İ, Gunaydin B, Caskurlu T, et al. Comparison of intravesical (Cohen) and extravesical (lich-gregoir) ureteroneocystostomy in the treatment of unilateral primary vesicoureteric reflux in children. J Pediatr Urol. (2018) 14:65.e 1–65.4. doi: 10.1016/j.jpurol.2017.09.014
35. Park SY. Nomogram: an analogue tool to deliver digital knowledge. J Thorac Cardiovasc Surg. (2018) 155:1793. doi: 10.1016/j.jtcvs.2017.12.107
36. Wu J, Zhang H, Li L, Hu M, Chen L, Xu B, et al. A nomogram for predicting overall survival in patients with low-grade endometrial stromal sarcoma: a population-based analysis. Cancer Commun (Lond). (2018) 40:301–12. doi: 10.1002/cac2.12067
37. Liu C, Liu X, Hu M, Li DW, Zhang M, Zhu C, et al. A simple nomogram for predicting infectious diseases in adult kidney transplantation recipients. J Gerontol A Biol Sci Med Sci. (2023) 78:1227–33. doi: 10.3389/fpubh.2022.944137
38. Song YX, Yang XD, Luo YG, Ouyang CL, Yu Y, Ma YL, et al. Comparison of logistic regression and machine learning methods for predicting postoperative delirium in elderly patients: a retrospective study. CNS Neurosci Ther. (2023) 29:158–67. doi: 10.1111/cns.13991
39. Nordenström J, Koutozi G, Holmdahl G, Abrahamsson K, Sixt R, Sjöström S. Changes in differential renal function after pyeloplasty in infants and children. J Pediatr Urol. (2020) 16:329.e 1–329.8. doi: 10.1016/j.jpurol.2020.02.002
40. Chan YY, Rosoklija I, Shannon R, Singal A, D'Oro A, Meade P, et al. MPH urinary tract infection after robot-assisted laparoscopic pyeloplasty: are urine cultures and antibiotics helpful? Urology. (2021) 148:235–42. doi: 10.1016/j.urology.2020.09.054
41. Holzman SA, Braga LH, Zee RS, Davis-Dao CA, Kern NG, Chamberlin JD, et al. Risk of urinary tract infection in patients with hydroureter: an analysis from the society of fetal urology prenatal hydronephrosis registry. J Pediatr Urol. (2021) 17:775–81. doi: 10.1016/j.jpurol.2021.09.001
42. Krajewski W, Wojciechowska J, Dembowski J, Zdrojowy R, Szydełko T. Hydronephrosis in the course of ureteropelvic junction obstruction: an underestimated problem? Current opinions on the pathogenesis, diagnosis and treatment. Adv Clin Exp Med. (2017) 26:857–64. doi: 10.17219/acem/59509
43. Estrada CR, Peters CA, Retik AB, Nguyen HT. Vesicoureteral reflux and urinary tract infection in children with a history of prenatal hydronephrosis—should voiding cystourethrography be performed in cases of postnatally persistent grade II hydronephrosis? J Urol. (2009) 181:801–6. discussion 806–7. doi: 10.1016/j.juro.2008.10.057
44. Ferroni MC, Lyon TD, Rycyna KJ, Dwyer ME, Schneck FX, Ost MC, et al. The role of prophylactic antibiotics after minimally invasive pyeloplasty with ureteral stent placement in children. Urology. (2016) 89:107–11. doi: 10.1016/j.urology.2015.11.035
45. Neheman A, Sabler IM, Beberashvili I, Goltsman G, Verchovsky G, Kord E, et al. Ureteral stent colonization and urinary tract infection in children undergoing minimally invasive pyeloplasty. Eur J Pediatr Surg. (2023) 33:47–52. doi: 10.1055/a-1905-4460
46. Pakkasjärvi N, Krishnan N, Ripatti L, Anand S. Learning curves in pediatric robot-assisted pyeloplasty: a systematic review. J. Clin. Med. (2022) 11(23):6935. doi: 10.3390/jcm11236935
47. Dothan D, Raisin G, Jaber J, Kocherov S, Chertin B. Learning curve of robotic assisted laparoscopic pyeloplasty (RALP) in children: how to reach a level of excellence? J Robot Surg. (2021) 15:93–7. doi: 10.1007/s11701-020-01082
48. Spampinato G, Binet A, Fourcade L, Mendoza Sagaon M, Villemagne T, Braik K, et al. Comparison of the learning curve for robot-assisted laparoscopic pyeloplasty between senior and junior surgeons. J Laparoendosc Adv Surg Tech A. (2021) 31:478–83. doi: 10.1089/lap.2020.0822
49. Zhu H, Shen C, Li X, Xiao X, Chen X, Zhang Q, et al. Laparoscopic pyeloplasty: a comparison between the transperitoneal and retroperitoneal approach during the learning curve. Urol Int. (2013) 90:130–5. doi: 10.1159/000343989
50. Junejo NN, Alotaibi A, Alshahrani SM, Peters CA, Alhazmi H, Vallasciani SA. The learning curve for robotic assisted pyeloplasty in children: our initial experience from a single center. Urol Ann. (2020) 12:19–24. doi: 10.4103/UA.UA_113_19
51. Tasian GE, Wiebe DJ, Casale P. Learning curve of robotic assisted pyeloplasty for pediatric urology fellows. J Urol. (2013) 190:1622–6. doi: 10.1016/j.juro.2013.02.009
52. Chammas MF Jr, Mitre AI, Arap MA, Hubert N, Hubert J. Learning robotic pyeloplasty without simulators: an assessment of the learning curve in the early robotic era. Clinics (Sao Paulo). (2019) 74:e777. doi: 10.6061/clinics/2019/e777
53. Panek W, Szmer J, Kuijper CF, Chrzan R. Learning curve or experience-related outcome: what really matters in paediatric laparoscopic pyeloplasty. Wideochir Inne Tech Maloinwazyjne. (2020) 15:377–81. doi: 10.5114/wiitm.2019.89391
54. Masieri L, Sforza S, Grosso AA, Lorenzo M, Simone S, Antonio A G, et al. Robot-assisted laparoscopic pyeloplasty in children: a systematic review. Minerva Urol Nefrol. (2020) 72:673–90. doi: 10.23736/S0393-2249.20.03854-0
Keywords: nomogram, predict, prognosis, recurrence, UPJO
Citation: Ma W, Gao H, Chang M, Lu Z, Li D, Ding C, Bi D and Sun F (2024) The construction of a nomogram to predict the prognosis and recurrence risks of UPJO. Front. Pediatr. 12:1376196. doi: 10.3389/fped.2024.1376196
Received: 25 January 2024; Accepted: 13 March 2024;
Published: 2 April 2024.
Edited by:
Simone Sforza, University of Florence, ItalyReviewed by:
Shimin Wu, Huazhong University of Science and Technology, China© 2024 Ma, Gao, Chang, Lu, Li, Ding, Bi and Sun. This is an open-access article distributed under the terms of the Creative Commons Attribution License (CC BY). The use, distribution or reproduction in other forums is permitted, provided the original author(s) and the copyright owner(s) are credited and that the original publication in this journal is cited, in accordance with accepted academic practice. No use, distribution or reproduction is permitted which does not comply with these terms.
*Correspondence: Fengyin Sun dGpjb2xsZWdlMjAxNEAxNjMuY29t
†These authors share first authorship
Disclaimer: All claims expressed in this article are solely those of the authors and do not necessarily represent those of their affiliated organizations, or those of the publisher, the editors and the reviewers. Any product that may be evaluated in this article or claim that may be made by its manufacturer is not guaranteed or endorsed by the publisher.
Research integrity at Frontiers
Learn more about the work of our research integrity team to safeguard the quality of each article we publish.