- 1Pediatric Heart Center, Children’s Hospital of Fudan University, Shanghai, China
- 2Department of Pediatric, The First Affiliated Hospital of Shandong First Medical University, Jinan, China
Objective: This study aimed to construct and validate a nomogram for predicting cardiovascular mortality (CVM) for child, adolescent, and adult patients with diffuse large B-cell lymphoma (DLBCL).
Materials and methods: Patients with only one primary tumor of DLBCL first diagnosed between 2000 and 2019 in the SEER database were extracted. We used the cumulative incidence function (CIF) to evaluate the cumulative rate of CVM. The outcome of interest was CVM, which was analyzed using a competing risk model, accounting for death due to other causes. The total database was randomly divided into a training cohort and an internal validation cohort at a ratio of 7:3. Adjustments were for demographics, tumor characteristics, and treatment modalities. Nomograms were constructed according to these risk factors to predict CVM risk at 5, 10, and 15 years. Validation included receiver operating characteristic (ROC) curves, time-dependent ROC, C-index, calibration curves, and decision curve analysis.
Results: One hundred four thousand six hundred six patients following initial diagnosis of DLBCL were included (58.3% male, median age 64 years, range 0–80, White 83.98%). Among them, 5.02% died of CVM, with a median follow-up time of 61 (31–98) months. Nomograms based on the seven risk factors (age at diagnosis, gender, race, tumor grade, Ann Arbor stage, radiation, chemotherapy) with hazard ratios ranging from 0.19–1.17 showed excellent discrimination, and calibration plots demonstrated satisfactory prediction. The 5-, 10-, and 15-year AUC and C-index of CVM in the training set were 0.716 (0.714–0.718), 0.713 (0.711–0.715), 0.706 (0.704–0.708), 0.731, 0.727, and 0.719; the corresponding figures for the validation set were 0.705 (0.688–0.722), 0.704 (0.689–0.718), 0.707 (0.693–0.722), 0.698, 0.698, and 0.699. Decision curve analysis revealed a clinically beneficial net benefit.
Conclusions: We first built the nomogram model for DLBCL patients with satisfactory prediction and excellent discrimination, which might play an essential role in helping physicians enact better treatment strategies at the time of initial diagnosis.
Introduction
Diffuse large B-cell lymphoma (DLBCL) is the most common subtype of non-Hodgkin lymphoma (NHL) (1), making up roughly 40% of the total NHL population (2, 3). With improvements in treatment methods and increased survival rates, the number of DLBCL survivors is continually growing (2–4). The conventional treatment regimen for DLBCL commonly incorporates drugs like cyclophosphamide, doxorubicin, and rituximab, all of which carry a heightened risk of cardiac damage (1). Therefore, these survivors face an elevated risk of cardiovascular mortality (CVM) (5–7). While numerous previous studies (8–10) have discussed the incidence of cardiovascular events, there is limited literature addressing the CVM risk in DLBCL patients, especially in children and adolescents. To the best of our knowledge, there are no reported studies that have constructed and validated a nomogram for assessing the CVM risk in children, adolescents, and adults with DLBCL.
In child, adolescent, and adult DLBCL survivors, CVM might be related to disease stages, treatment modalities, and genetic factors (6, 7, 11). The challenges in estimating CVM risks have been highlighted in previous studies (5, 7, 12, 13). Previous studies (5, 14) have primarily utilized Cox regression models to investigate the risk of cardiovascular death and specifically focus on the elderly population. Our research distinctively employs a competing risks model for a more nuanced analysis. This approach incorporates the concept of competitive risks, mitigates the impact of death from other causes on the final outcomes, and includes individuals across the entire age spectrum, which facilitates the attainment of more reliable results (15, 16).
This study aims to construct and validate a nomogram to predict the risk of CVM for child, adolescent, and adult DLBCL patients. By better understanding these relationships, we can provide more targeted guidance for the long-term cardiovascular health management of DLBCL survivors, particularly for the younger age demographic, thereby improving their quality of life and survival prospects.
Materials and methods
Data source and study population
As a network of U.S. population-based incident tumor registries, the Surveillance, Epidemiology, and End Results (SEER) program, which is a public registry maintained by the National Cancer Institute, currently encompasses approximately 27.8% of the cancer patient population (17). The SEER collects patient clinical information data, such as demographics, primary tumor site, stage at diagnosis, initial course of treatment, follow-up time, survival, and economic status of residence. We extracted data from patients with only one primary DLBCL between 2000 and 2019 in the SEER database using the SEER*Stat software (version 8.4.2).
We utilized histology codes from the third edition of the International Classification of Diseases for Oncology (ICD-O-3)—codes 9680/3, 9684/3, and 9688/3—to assemble the cohort of interest, including children, adolescents, and adults aged 0–80 years following initial diagnosis of DLBCL. Patients with a diagnosis at autopsy or death certificate and those with incomplete data on certain variables (survival time, cause of death, race, gender) were excluded (Figure 1).
Risk factors
The variables included in this study are based on clinical support (18, 19) and include age at diagnosis (0–18 years, 19–40 years, 41–60 years, 61–80 years), gender, year of diagnosis, tumor grade (no nodal or metastatic disease as local, nodal disease as regional, or any metastatic disease as distant), race (white, black, or other), cause of death, survival time, the Ann Arbor stage (Stage I: Early-stage cancer confined to the organ of origin. Stage II: Local spread, potentially involving nearby lymph nodes. Stage III: More extensive local spread, involving additional lymph nodes. Stage IV: Presence of distant metastasis.), primary site, mean household income (<$60,000, >$60,000), surgery (yes, no), chemotherapy (yes, no/unknown), radiotherapy (yes, no/unknown), and place of residence (rural, urban). Primary site codes were used from SEER*Stat for the “lip, oral, cavity and pharynx” (C0.0–14.9), digestive organs (C15.0–C27.0), “respiratory and intrathoracic organs” (C30.0–C39.9), “bone, joints and articular cartilage” (C40.0–C41.9), hematopoietic (C42.0–C42.9), skin (C44.0–C44.9), nervous system (C47.0–C47.9), peritoneum (C48.0–C48.9), soft tissues (C49.0–C49.9), breast (C50.0–C50.9), female genital organs (C51.0–C57.9), male genital organs (C60.0–C63.9), urinary tract (C64.0–C68.9), “eye, brain and other parts of central nervous system” (C69.0–C72.9), “endocrine system” (C73.0–C76.9), “lymph nodes” (C77.0–C78.0). County median income level was dichotomized into groups based on the SEER-linked county-level data regarding the median household income in the past 12 months using 2019 inflation-adjusted dollars. The initial course of treatment was determined based on whether patients received surgery, chemotherapy, or radiation therapy. Survival time refers to the interval from the diagnosis of cancer to the death of patients due to any cause or the last date of available survival information.
Outcomes
The main outcome of interest was a composite of CVM, defined as any of the following seven causes of death in the SEER database [International Classification of Diseases, 10th Revision (ICD-10) codes]: heart diseases (I00–I09, I11, I13, I20–I51), hypertension without heart disease (I10, I12), cerebrovascular diseases (I60–I69), atherosclerosis (I70), aortic aneurysm and dissection (I71), and other diseases of arteries, arterioles, and capillaries (I72–I78). Competitive risk refers to mortality due to other causes (such as primary diffuse large B-cell lymphoma, infection, and bleeding).
Statistical analysis
For calculating the sample size required for developing a clinical prediction model, the sample size calculation satisfied both the 15 EPV (events per variable) and pmsampsize rule requirements (20) (T1: estimate the overall outcome proportion with sufficient precision at one or more key time-points in follow-up; T2: target a shrinkage factor of 0.9; T3: target small optimism of 0.05 in the apparent R2Nagelkerke) in the training dataset. The R package “pmsampsize” was used, referring to some statistical parameters from a previous study (5). The minimum sample size calculated by the “pmsampsize” package was 1,450 cases. The sample size of the training dataset satisfies this requirement.
Normally distributed data were expressed as mean ± standard deviation. Non-normally distributed data were expressed as medians with interquartile ranges. Categorical data were presented using counts with percentages and compared using the chi-square test, while when frequencies were below 5, Fisher's exact test was applied.
In the competing risk analyses, we used the cumulative incidence function (CIF) to evaluate the cumulative rate of CVM. Multivariable competing risk survival analyses were performed to identify predictors of CVM. The total database was randomly divided into a training cohort and an internal validation cohort at a ratio of 7:3. The training cohort was used for risk factor analysis and nomogram construction. Factors with p-values < 0.05 in univariate competing risk analysis were added to a multivariate competing risk model to detect indicators of death specifically due to CVM. Based on the results of the competing risk analysis of the training cohort, a nomogram was constructed that incorporated all the independent prognostic factors to predict 5-, 10-, and 15-year CVM risk.
We evaluated the accuracy of the nomogram model by examining the 5-, 10-, and 15-year ROC curves. C-index for 5, 10, and 15 years were calculated to measure the predictive ability and accuracy of the model. The predicting outcomes of the nomogram were evaluated in the respective training and validation cohorts by calibrating curves and the decision curve analysis (DCA). DCA is a critical method for assessing the clinical utility of clinical predictive models and can address the limitations of the ROC curve (21).
Ethical approval of this publicly available information provided by the SEER program was not required by our Institutional Review Board. The manuscript has been prepared in accordance with the guideline of Transparent Reporting of a Multivariable Prediction Model for Individual Prognosis or Diagnosis (TRIPOD) (22). For detailed information regarding the TRIPOD checklist, please refer to the online supplementary appendix. Statistical analyses were performed using STATA-MP 17.0 (StataCorp, College Station, TX) and R software (version 4.1.2; R Foundation for Statistical Computing, Vienna, Austria). All statistical tests were two-sided, with the significance level set at a p-value of < 0.05.
Results
Patient characteristics
A total of 104,606 DLBCL were included in subsequent analyses. The median age at diagnosis was 64 (53.72) years. The proportion of male patients was 60,953 (58.27%) and the median follow-up time was 61 (31–98) months. The majority of patients were White 87,845 (83.98%) and 56,080 (53.61%) had distant tumor stage. The most common primary site was lymph nodes (64.46%), followed by the digestive system (9.34%) and nervous system (4.67%). A total of 27,713 (26.49%) patients underwent surgery, 82,825 (79.18%) patients received chemotherapy, and only 18,755 (17.93%) patients underwent radiotherapy. Furthermore, 5,254 (5.02%) patients died of CVD, 4,202 (4.02%) died due to heart diseases, 715 (0.68%) due to cerebrovascular diseases, 53 (0.05%) due to aortic aneurysm and dissection, 180 (0.17%) due to hypertension without heart disease, and 38 (0.04%) due to atherosclerosis. The baseline characteristics are detailed in Tables 1, 2.
Univariate and multivariable analyses on the cardiovascular mortality
Risk factor analyses were based on the training set of 73,224 patients. The results of the univariate analysis are summarized in Table 3. In the univariate analysis, factors including age at diagnosis, gender, race, tumor grade, Ann Arbor stage, chemotherapy, and radiation were found to be associated with the CVM risk in child, adolescent, and adult patients with DLBCL.
Risk factor analysis
The multivariate analysis of CVM conducted by competing risk analysis in the training cohort is summarized in Table 4. Multivariate analysis revealed that the following characteristics were associated with risk of CVM: age at diagnosis ([0–18 vs. 61–80] HR: 0.19, 95% CI: 0.15–0.23; [19–40 vs. 61–80] HR: 0.34, 95% CI: 0.32–0.36; [41–60 vs. 61–80] HR: 0.57, 95% CI: 0.55–0.58), gender [(Male vs. Female) HR: 1.17, 95% CI: 1.15–1.21], race [(White vs. Black) HR: 0.73, 95% CI:0.69–0.76], tumor grade ([Localized vs. Distant] HR: 0.62, 95% CI: 0.58–0.65; [Regional vs. Distant] HR: 0.72, 95% CI: 0.68–0.76), Ann Arbor stage ([Stage II vs. Stage I] HR: 0.89, 95% CI: 0.82–0.96; [Stage III vs. Stage I] HR: 0.82, 95% CI: 0.76–0.89; [Stage IV vs. Stage I] HR: 1.05, 95% CI: 0.98–1.11). In terms of treatment, radiation [(Yes vs. No) HR: 0.85, 95% CI: 0.82–0.88] and chemotherapy [(Yes vs. No) HR: 0.56, 95% CI: 0.55–0.57] were associated with CVM in DLBCL patients.
Developing nomograms
The results of the CIF curves of cause of death in DLBCL patients are illustrated in Figure 2 and show that CVM increases with survival time.
In the training cohort, prognostic factors were used to construct the nomogram of CVM for predicting 5-, 10-, and 15-year CVM risk, as presented in Figure 3. In this nomogram, each variable corresponds to a point on the axis of the nomogram, and the corresponding score of the variable was obtained. The sum score of each variable was obtained, the total score corresponded to the point on the risk axis, and the risk value of CVM was obtained.
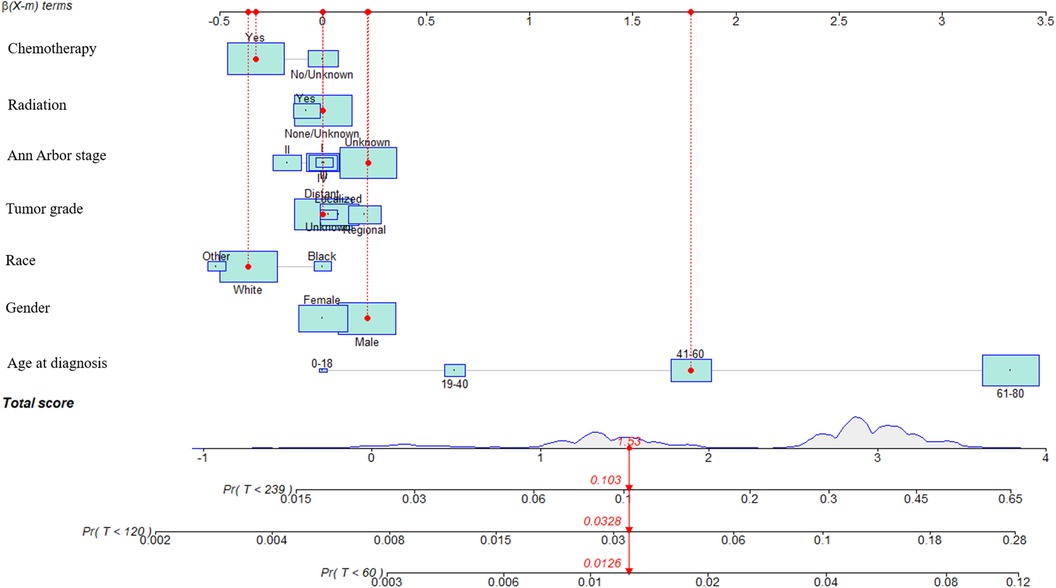
Figure 3. Nomograms to predict CVM for patients with DLBCL and risk stratification. In this nomogram, each variable corresponds to a point on the axis of the nomogram, and the corresponding score of the variable was obtained. The sum score of each variable was obtained, the total score corresponded to the point on the risk axis, and the risk value of CVM was obtained.
Internal validation
Internal validation was performed in a cohort of 31,382 patients. Patient baseline characteristics between the two cohorts were well-balanced (Supplementary Table S1). Validation methods demonstrated efficacy and stability. The 5-, 10-, and 15-year ROC curves of CVM and time-dependent ROC curves of the CVM nomogram were displayed (Figures 4, 5). The 5-, 10-, and 15-year AUC of CVM in the training set were 0.716 (0.714–0.718), 0.713 (0.711–0.715), and 0.706 (0.704–0.708), with C-index 0.731, 0.727, and 0.719, respectively; the corroding figures for the validation set were 0.705 (0.688–0.722), 0.704 (0.689–0.718), and 0.707 (0.693–0.722), with C-index 0.698, 0.698, and 0.699, respectively (Table 5). The calibration curves were very close to the diagonal (Supplementary Figure S1) showed high consistencies between the predicted and observed CVM probability in the cohorts. This demonstrates good predictive ability and accuracy of the model. The decision curve analysis for the model is shown in Figure 6. The X-axis indicates the threshold probability for CVM, while the Y-axis indicates the net benefit. The DCA indicated a significantly better net benefit, indicating the effective use of the model in achieving net clinical benefit.
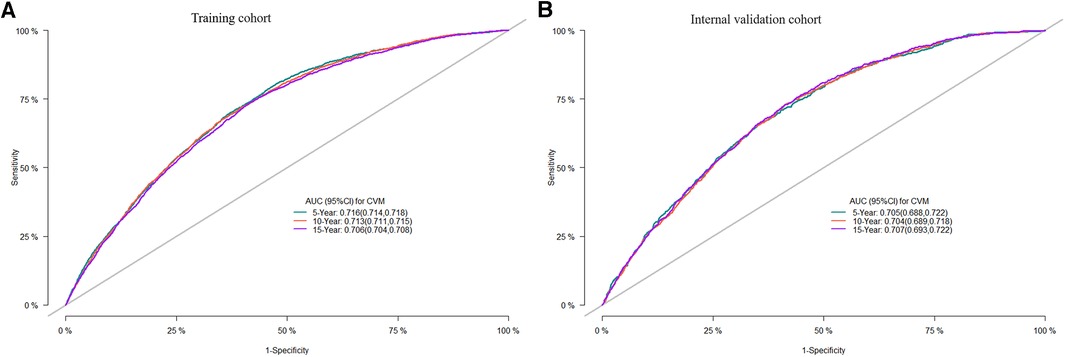
Figure 4. ROC curves of the nomogram of DLBCL patients for 5-, 10-, and 15-year CVM rates in the training cohort (A), the internal validation cohort (B); ROC, receiver operating characteristic; AUC, area under the curve.
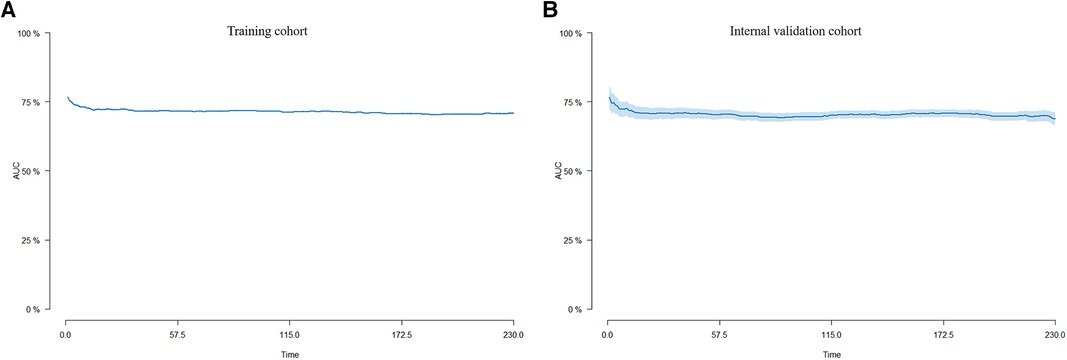
Figure 5. Time-dependent AUC curves for the nomogram of CVM in the training cohort (A) and the internal validation cohort (B).
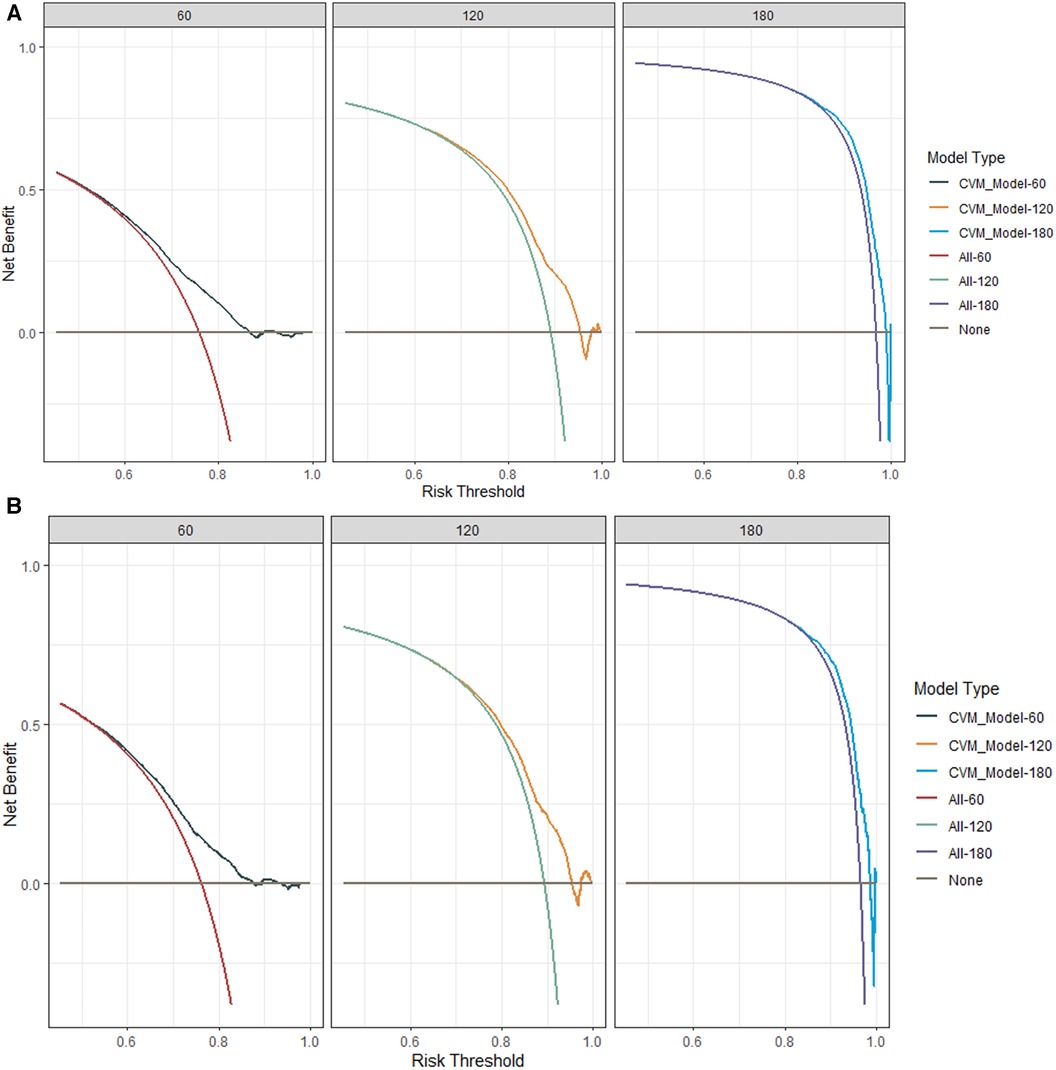
Figure 6. The decision-curve analysis (DCA) plots of the nomogram of DLBCL patients for 60-, 120-, and 180-month CVM rates in the training cohort (A) and the internal validation cohort (B).
Discussion
Utilizing the SEER database of more than 10,000 patients first diagnosed with DLBCL between the ages of 0 and 80 years, we built the first nomogram to predict the risk of CVM, with excellent results in internal validation. This research reports a 5.02% cumulative occurrence rate of cardiovascular death in diffuse large B-cell lymphoma patients, identifying significant risk factors for predicting CVM, including age at diagnosis, gender, tumor grade, Ann Arbor stage, radiation, and chemotherapy. The reported AUC exceeds 0.70, indicating good predictive ability. The risk of CVM decreases with earlier diagnosis, as segmented by age groups.
DLBCL is a type of non-Hodgkin's lymphoma, of which complications such as cardiomyopathy and rhythm disturbances are frequently documented in children, adolescents, and adults (3, 12). In most studies (8, 23), the prevalence of cardiovascular disease is described rather than the cardiovascular disease mortality rate. In our current study, we observed a CVM rate of 5.02% among DLBCL patients. This finding is corroborated by the work of Jurczak et al. (24), who reported a 4.59% rate of cardiovascular-related deaths in DLBCL patients undergoing treatment. Additionally, our research demonstrates that for diffuse large B-cell lymphoma patients, an early diagnosis in childhood correlates with a reduced risk of cardiovascular death. A recent study disclosed that among the causes of cardiovascular death, heart-related conditions were the most predominant, accounting for 79.4% of cases, followed by cerebrovascular diseases, at 14.4% (5). These findings are consistent with our own results.
Our findings emphasize that age at diagnosis has a notable correlation with the risk of CVM, in line with previous studies (25–27). This association is particularly pronounced among older individuals, who frequently present with an advanced stage of the disease. Additionally, factors such as limited tolerance to adequate chemotherapy and the presence of pre-existing comorbidities, especially cardiac conditions, exacerbate the risk within this demographic (28). The heightened treatment toxicity observed in elderly patients is further influenced by the diminished reserve capacity of organs susceptible to these toxic effects.
When comparing gender, we observed a higher risk among men than among women. This is consistent with the findings reported in other literature (5, 27, 29–31). This may be attributed to men's metabolism of chemotherapy drugs, which leads to a lower response and survival (32). Race was also identified as a predictor of CVM in our study. This is consistent with the findings of previous studies (27, 30), which reported that black patients had a higher risk of CVM compared to white DLBCL patients. This could be due to disparities in access to healthcare and treatment.
The tumor grade was also identified as a significant predictor of CVM in our study. The findings from the study conducted by Kamel et al. (5) are in alignment with our observations, revealing that patients with a high tumor grade are at an elevated risk of CVM. This could be due to the aggressive nature of high-tumor grade, which often requires intensive treatment that can increase the risk of cardiovascular disease. Simultaneously, in our research, our study revealed that the Ann Arbor stage was correlated with the risk of CVM. This result has also been observed in other research articles (5, 33). Additional clinical research is required to elucidate the predictive roles of tumor grade and Ann Arbor stage in assessing the risk of CVM among DLBCL patients.
Radiotherapy emerged as the pioneering treatment, offering enduring remission and even a potential cure for DLBCL patients (34, 35). Contrary to traditional understanding, mediastinal or thoracic region radiation can inflict considerable harm on the heart and adjacent vasculature, thereby elevating the risk of ischemic heart disease and valvular irregularities. There is controversy around whether radiotherapy is appropriate for DLBCL patients. Additionally, in our study, both univariate and multivariate competing risk models indicated that patients who underwent radiation therapy had lower cardiovascular disease-related mortality compared to those who did not receive radiation treatment. Our findings are consistent with the studies (35, 36), indicating that the mortality rate is minimized through exposure to radiotherapy compared to patients who did not receive radiation. The latter group showed a higher risk of mortality and a lower survival rate. This may suggest that undergoing radiation therapy is associated with reduced cardiovascular-related mortality rates. Zimmermann et al. (37) indicate that the omission of radiation leads not only to a shorter progression-free survival (PFS) but also to worse overall survival (OS). Radiation should therefore remain standard practice. In the SEER program, a detailed radiotherapy regimen was lacking. As such, additional research is necessary to elucidate the impact of radiotherapy on the risk of CVM in patients diagnosed with DLBCL.
Strengths and limitations
The current study offers several notable advantages that contribute to both its credibility and broader applicability. To begin with, our study has verified the risk factors for CVM in children, adolescents, and adults with DLBL and has constructed the first predictive model for all age groups to predict CVM. However, our study has some limitations. First, the subjects included in our study were patients with initial, solitary occurrences of diffuse large B-cell lymphoma, representing a selective approach. Second, some information associated with CVM that might influence survival was not available, such as doses of radiation, chemotherapy regimens, smoking, alcohol use, transcriptomic or genomic data, and history of cardiovascular diseases. Third, because our study patients were predominantly White, the generalizability of our findings to other populations and ethnicities warrants further investigation.
Conclusions
We first built the nomogram model for DLBCL patients, with satisfactory prediction and excellent discrimination, which might play an essential role in helping physicians enact better treatment strategies at the time of initial diagnosis. Age of diagnosis, gender, race, tumor grade, Ann Arbor stage, radiation, and chemotherapy were predictors for risk of CVM. Clinical variables at diagnosis can identify DLBCL patients at high risk of CVM, for whom preventive interventions should be considered.
Data availability statement
The data set analysis of this study can be found in SEER database. The datasets analyzed in this study are available in the SEER repository and can be obtained from: https://seer.cancer.gov/data/.
Ethics statement
The studies involving humans were approved by Ethics Committee of the First Affiliated Hospital of Shandong First Medical University. The studies were conducted in accordance with the local legislation and institutional requirements. Written informed consent for participation in this study was provided by the participants’ legal guardians/next of kin.
Author contributions
KM: Data curation, Formal Analysis, Software, Validation, Visualization, Writing – original draft, Writing – review & editing. JZ: Data curation, Formal Analysis, Investigation, Validation, Writing – original draft. YG: Data curation, Formal Analysis, Writing – original draft. GH: Conceptualization, Formal Analysis, Funding acquisition, Investigation, Software, Validation, Visualization, Writing – original draft, Writing – review & editing.
Funding
The author(s) declare financial support was received for the research, authorship, and/or publication of this article.
This research was supported by the Shandong Provincial Medical Association Clinical Research Funds Qilu Special (YXH2022ZX02181), the Jinan Science and Technology Plan- Clinical Medical Science and Technology Innovation Program (No. 202134003, No. 202225057), Shandong Medical Association Cord Blood Research Program (YXH2020zx005), and the First Affiliated Hospital of Shandong First Medical University National Natural Science Foundation Cultivation Program (QYPY2020NSFC0616).
Conflict of interest
The authors declare that the research was conducted in the absence of any commercial or financial relationships that could be construed as a potential conflict of interest.
Publisher's note
All claims expressed in this article are solely those of the authors and do not necessarily represent those of their affiliated organizations, or those of the publisher, the editors and the reviewers. Any product that may be evaluated in this article, or claim that may be made by its manufacturer, is not guaranteed or endorsed by the publisher.
Supplementary material
The Supplementary Material for this article can be found online at: https://www.frontiersin.org/articles/10.3389/fped.2024.1346006/full#supplementary-material
Supplementary Figure 1
Calibration curves of the nomogram of DLBCL patients for 5-, 10-, and 15-year CVM rates in the training cohort (A–C) and the internal validation cohort (D–F).
References
1. Tavakkoli M, Barta SK. 2024 update: advances in the risk stratification and management of large B-cell lymphoma. Am J Hematol. (2023) 98(11):1791–805. doi: 10.1002/ajh.27075
2. Zhang N, Wu J, Wang Q, Liang Y, Li X, Chen G, et al. Global burden of hematologic malignancies and evolution patterns over the past 30 years. Blood Cancer J. (2023) 13:82. doi: 10.1038/s41408-023-00853-3
3. Kambhampati S, Herrera AF, Rhee JW. How to treat diffuse large B-cell lymphoma: oncologic and cardiovascular considerations. JACC CardioOncol. (2023) 5:281–91. doi: 10.1016/j.jaccao.2023.05.001
4. Lee SF, Vellayappan BA, Wong LC, Chiang CL, Chan SK, Wan EY, et al. Cardiovascular diseases among diffuse large B-cell lymphoma long-term survivors in Asia: a multistate model study. ESMO Open. (2022) 7:100363. doi: 10.1016/j.esmoop.2021.100363
5. Kamel MG, El-Qushayri AE, Thach TQ, Huy NT. Cardiovascular mortality trends in non-Hodgkin’s lymphoma: a population-based cohort study. Expert Rev Anticanc. (2018) 18:91–100. doi: 10.1080/14737140.2018.1409626
6. Ocier K, Abdelaziz S, Kim S, Rowe K, Snyder J, Deshmukh V, et al. Cardiovascular disease risks in younger versus older adult B-cell non-Hodgkin’s lymphoma survivors. Cancer Med. (2021) 10:4117–26. doi: 10.1002/cam4.3934
7. Zhu C, Shi T, Jiang C, Liu B, Baldassarre LA, Zarich S. Racial and ethnic disparities in all-cause and cardiovascular mortality among cancer patients in the U.S. JACC CardioOncol. (2023) 5:55–66. doi: 10.1016/j.jaccao.2022.10.013
8. Upshaw JN, Nelson J, Rodday AM, Kumar AJ, Klein AK, Konstam MA, et al. Association of preexisting heart failure with outcomes in older patients with diffuse large B-cell lymphoma. Jama Cardiol. (2023) 8:453–61. doi: 10.1001/jamacardio.2023.0303
9. Barac A. Assessing heart failure vs lymphoma treatment risks and benefits-it takes two to tango. JAMA Cardiol. (2023) 8:462–3. doi: 10.1001/jamacardio.2023.0312
10. Maier RH, Plummer C, Kasim AS, Akhter N, Ogundimu E, Maddox J, et al. Preventing cardiotoxicity in patients with breast cancer and lymphoma: protocol for a multicentre randomised controlled trial (PROACT). Bmj Open. (2022) 12:e66252. doi: 10.1136/bmjopen-2022-066252
11. Salz T, Zabor EC, Brown PN, Dalton SO, Raghunathan NJ, Matasar MJ, et al. Cardiovascular risk factors, radiation therapy, and myocardial infarction among lymphoma survivors. Acta Oncol. (2022) 61:1064–8. doi: 10.1080/0284186X.2022.2107402
12. Moser EC, Noordijk EM, van Leeuwen FE, le Cessie S, Baars JW, Thomas J, et al. Long-term risk of cardiovascular disease after treatment for aggressive non-Hodgkin lymphoma. Blood. (2006) 107:2912–9. doi: 10.1182/blood-2005-08-3392
13. Ruiz-Soto R, Sergent G, Gisselbrecht C, Larghero J, Ertault M, Hennequin C, et al. Estimating late adverse events using competing risks after autologous stem-cell transplantation in aggressive non-Hodgkin lymphoma patients. Cancer Am Cancer Soc. (2005) 104:2735–42. doi: 10.1002/cncr.21492
14. Zhang Z, Geskus RB, Kattan MW, Zhang H, Liu T. Nomogram for survival analysis in the presence of competing risks. Ann Transl Med. (2017) 5:403. doi: 10.21037/atm.2017.07.27
15. Austin PC, Lee DS, Fine JP. Introduction to the analysis of survival data in the presence of competing risks. Circulation. (2016) 133:601–9. doi: 10.1161/CIRCULATIONAHA.115.017719
16. Austin PC, Fine JP. Practical recommendations for reporting fine-gray model analyses for competing risk data. Stat Med. (2017) 36:4391–400. doi: 10.1002/sim.7501
17. Murphy CC, Tortolero GA, Gerber DE, Pruitt SL. An updated report on the prevalence of prior cancer among persons newly diagnosed with cancer in the surveillance, epidemiology, and end results program. JAMA Oncol. (2023) 9:1147–50. doi: 10.1001/jamaoncol.2023.1723
18. Mohan SSR, Ahmad M. Novel technologies must be considered for childhood cancer survivors at risk for cardiomyopathy. JACC CardioOncol. (2023) 5:156. doi: 10.1016/j.jaccao.2022.10.012
19. Leong DP, Mukherjee SD. The European society of cardiology cardio-oncology guidelines: evidence base, actionability, and relevance to clinical practice. JACC CardioOncol. (2023) 5:137–40. doi: 10.1016/j.jaccao.2022.10.009
20. Riley RD, Ensor J, Snell KIE, Harrell FE, Martin GP, Reitsma JB, et al. Calculating the sample size required for developing a clinical prediction model. Br Med J. (2020) 18:368. doi: 10.1136/bmj.m441
21. Fitzgerald M, Saville BR, Lewis RJ. Decision curve analysis. JAMA. (2015) 313:409–10. doi: 10.1001/jama.2015.37
22. Debray T, Collins GS, Riley RD, Snell K, Van Calster B, Reitsma JB, et al. Transparent reporting of multivariable prediction models developed or validated using clustered data: TRIPOD-cluster checklist. Br Med J. (2023) 380:e71018. doi: 10.1136/bmj-2022-071018
23. Salz T, Zabor EC, de Nully BP, Dalton SO, Raghunathan NJ, Matasar MJ, et al. Preexisting cardiovascular risk and subsequent heart failure among non-Hodgkin lymphoma survivors. J Clin Oncol. (2017) 35:3837–43. doi: 10.1200/JCO.2017.72.4211
24. Jurczak W, Szmit S, Sobociński M, Machaczka M, Drozd-Sokołowska J, Joks M, et al. Premature cardiovascular mortality in lymphoma patients treated with (R)-CHOP regimen—a national multicenter study. Int J Cardiol. (2013) 168:5212–7. doi: 10.1016/j.ijcard.2013.08.033
25. Rigacci L, Annibali O, Kovalchuk S, Bonifacio E, Pregnolato F, Angrilli F, et al. Nonpeghylated liposomal doxorubicin combination regimen (R-COMP) for the treatment of lymphoma patients with advanced age or cardiac comorbidity. Hematol Oncol. (2020) 38:478–86. doi: 10.1002/hon.2764
26. Zinzani PL, Federico M, Oliva S, Pinto A, Rigacci L, Specchia G, et al. The more patients you treat, the more you cure: managing cardiotoxicity in the treatment of aggressive non-Hodgkin lymphoma. Leuk Lymphoma. (2015) 56:12–25. doi: 10.3109/10428194.2014.894187
27. Zhao J, Su L, Zhong J. Risk factors for cancer-specific mortality and cardiovascular mortality in patients with diffuse large b-cell lymphoma. Clin Lymphoma Myeloma Leuk. (2020) 20:e858–63. doi: 10.1016/j.clml.2020.06.005
28. Volkova M, Russell RR. Anthracycline cardiotoxicity: prevalence, pathogenesis and treatment. Curr Cardiol Rev. (2011) 7:214–20. doi: 10.2174/157340311799960645
29. Bai LY, Yang MH, Chiou TJ, Liu JH, Yen CC, Wang WS, et al. Non-Hodgkin lymphoma in elderly patients: experience at Taipei veterans general hospital. Cancer-Am Cancer Soc. (2003) 98:1188–95. doi: 10.1002/cncr.11609
30. Crozier JA, Sher T, Yang D, Swaika A, Foran J, Ghosh R, et al. Persistent disparities among patients with T-cell non-Hodgkin lymphomas and B-cell diffuse large cell lymphomas over 40 years: a SEER database review. Clin Lymphoma Myeloma Leuk. (2015) 15:578–85. doi: 10.1016/j.clml.2015.06.005
31. Pulte D, Gondos A, Brenner H. Ongoing improvement in outcomes for patients diagnosed as having non-Hodgkin lymphoma from the 1990s to the early 21st century. Arch Intern Med. (2008) 168:469–76. doi: 10.1001/archinternmed.2007.125
32. Pfreundschuh M, Müller C, Zeynalova S, Kuhnt E, Wiesen MH, Held G, et al. Suboptimal dosing of rituximab in male and female patients with DLBCL. Blood. (2014) 123:640–6. doi: 10.1182/blood-2013-07-517037
33. Diamond A, Ayyappan S, Cao S, Tashtish N, Boughan K, Cooper B, et al. Risk factors for cardiovascular events and mortality in patients diagnosed with diffuse large B-cell lymphoma and treated with anthracyclines. Hematol Oncol. (2022) 40:626–36. doi: 10.1002/hon.3034
34. Bush RS, Gospodarowicz M, Sturgeon J, Alison R. Radiation therapy of localized non-Hodgkin’s lymphoma. Cancer Treat Rep. (1977) 61:1129–36.332347
35. Choi KH, Lee SJ, Mun SH, Song JH, Choi BO. Consolidative radiotherapy after complete remission following R-CHOP immunochemotherapy in stage III–IV diffuse large B-cell lymphoma patients: a systematic review and meta-analysis. Cancers (Basel). (2023) 15:3940. doi: 10.3390/cancers15153940
36. Miller TP, Dahlberg S, Cassady JR, Adelstein DJ, Spier CM, Grogan TM, et al. Chemotherapy alone compared with chemotherapy plus radiotherapy for localized intermediate- and high-grade non-Hodgkin’s lymphoma. N Engl J Med. (1998) 339:21–6. doi: 10.1056/NEJM199807023390104
Keywords: pediatric cardiology, cardiovascular mortality, cardio-oncology, diffuse large B-cell lymphoma, nomogram
Citation: Mu K, Zhang J, Gu Y and Huang G (2024) Development and validation of a nomogram for predicting cardiovascular mortality risk for diffuse large B-cell lymphoma in children, adolescents, and adults. Front. Pediatr. 12:1346006. doi: 10.3389/fped.2024.1346006
Received: 28 November 2023; Accepted: 22 January 2024;
Published: 7 February 2024.
Edited by:
Liqun Sun, University of Toronto, CanadaReviewed by:
Bo Zhang, Shanghai Jiao Tong University, ChinaXikun Han, Massachusetts Institute of Technology, United States
Wei Lv, University of Chinese Academy of Sciences, China
© 2024 Mu, Zhang, Gu and Huang. This is an open-access article distributed under the terms of the Creative Commons Attribution License (CC BY). The use, distribution or reproduction in other forums is permitted, provided the original author(s) and the copyright owner(s) are credited and that the original publication in this journal is cited, in accordance with accepted academic practice. No use, distribution or reproduction is permitted which does not comply with these terms.
*Correspondence: Guoying Huang gyhuang@shmu.edu.cn