- 1Department of Pediatric Hematology and Oncology, University Hospital Centre Zagreb, Zagreb, Croatia
- 2Primary Health Care Pediatrician, Šibenik, Croatia
- 3University Clinic for Children’s Disease, Medical Faculty, Ss. Cyril and Methodius University in Skopje, Skopje, North Macedonia
- 4Macedonian Academy of Sciences and Arts, Skopje, Republic of Macedonia
- 5Croatian Academy of Medical Sciences, Zagreb, Croatia
- 6School of Medicine, University of Zagreb, Zagreb, Croatia
Introduction: This cross-sectional study enrolled a group of 271 children with microcytic anemia in order to test the performance of 41 single and 2 composite formulas andindices in distinguishing between β-thalassemia (β-thal) and iron deficiency anemia (IDA) in the pediatric population.
Methods: Optimal pediatric cut-off values from the previously published formulas and indices were generated using ROC analysis. Logistic regression in R using generalized linear models (GLM) generated two new indices.
Results: Formulas and indices with optimal cut-offvalues in children with accuracy ≥90% were (in descending order): Matos & Carvalho index, MDHL(Telmissani) formula, England and Fraser formula, Pornprasert index, Sirachainan index, Telmissani (MCHD) formula, CRUISE index, Hameed index, Sargolzaie formula and Zaghloul II index. The CroThalDD-LM1 index has an accuracy of 93.36% (AUC 0.986, 95% CI 0.975–0.997), while the second CroThalDD-LM2 index utilizes absolute reticulocyte count alongside CBC variables, with an accuracy of 96.77% (AUC 0.985, 95% CI 0.988–0.999).
Discussion and conclusion: We recommend using aforementioned formulas and indices with corrected cut-off values and accuracy >90% alongside two new proposed indices. A comparison of both native and these new indices is encouraged. These are the first discrimination indices generated and designed precisely for the pediatric population, which includes preschool children.
1 Introduction
The discrimination between β-thalassemia (β-thal) and iron deficiency anemia (IDA) is of great socioeconomic importance. A recent multicenter study reports a 32.4% prevalence of IDA among infants in northwestern and 12% in children one year and older in central Croatia (1). Accurate and early diagnosis reduces costly and unnecessary laboratory testing. Furthermore, inappropriate empirical supplementary iron treatment, could be detrimental to the child's health. Many developing countries have limited resources for the genetic testing of β-thal, but have standard laboratory techniques for its detection. Since hemoglobin electrophoresis is not always readily available in developing countries, such discriminating formulas and indices can reduce their economic burden. Using accurate formula or index also provides an early screening with a high probability of β-thal or IDA in children. In this article, our goal was to determine the accuracy of the existing single and composite formulasand indices in distinguishing between these two diseases and, if possible, generate a new one(s). Previous adult indices and formulas with their original cut-off values have unsatisfactory diagnostic accuracy in distinguishing β-thal from IDA in children. Since children are the most vulnerable age group, especially in developing countries, creating a pediatric index that includes school and preschool children is necessary. The importance of this topic is proven by the latest works (1, 2).
2 Methods
To this end, we extensively searched three available databases (Pubmed, Scopus, Web of Science) to find all available published formulasand indices distinguishing β-thal from IDA. We tested the accuracy of forty-one single formulas andindices and two composite indices. The accuracy of these aforementioned formulasand indices has not been tested in children thus far. The following are shown in alphabetical order:
Alparslan [log10 (MCH × MCHC × RDW/RBC)], Bessman (RDW), Bordbar (80−MCV│ × │27−MCH), CRUISE (MCHC + 0.603 × RBC + 0.523 × RDW), Das Gupta (1.89 × RBC-0.33 × RDW-3.28), Ehsani (MCV−(10 × RBC), England & Fraser [MCV−RBC−(5 × Hb)−3.4], Green & King (MCV × MCV × RDW/Hb × 100), Hameed (MCV2 × RDW/(RBC2 × Hb), Hisham (Hb × MCV × RDW/RBC2), Huber-Herklotz (MCH × RDW × 0.1/RBC) + RDW), Index26, Jayabose (RDWI) (MCV × RDW/RBC), Janel (11T), Kandhro (RBC/HCT + 0.5 × RDW), Kandhro-2 (RDW × 5)/RBC), Keikhaei [Hb × RDW × 100/(RBC)2 × MCHC], Kerman I (MCV × MCH/RBC), Kerman II (MCV × MCH × 10/RBC × MCHC), Matos-Carvalho (1.91 × RBC) + (0.44 × MCHC), Mentzer (MCV/RBC), Merdin-1(RDW × RBC/MCV), Merdin-2 (RDW × RBC × Hb/MCV), Pornprasert (MCHC), Ravanbakhsh F1 (MCV/HCT), Ravanbakhsh F2 (RDW−3 × RBC), Ravanbakhsh F3 (MCV × RDW−100 RBC), Ravanbakhsh F4 (MCV × Hb/RDW × RBC), RBC (RBC), Ricerca (RDW/RBC), Sargolzaie (125.6 + (44.3 × RBC)−(20.9 × Hb)−(2.5 × MCV) + (20.3 × MCH)−12.18 × MCHC), Seghal (MCV2 /RBC), Shine-Lal (MCV × MCV × MCH/100), Sirachainan (1.5Hb-0.05 × MCV), Sirdah (MCV−RBC−(3 × Hb), Srivastava (MCH/RBC), SVM (1.45 × (MCV−82.8)/10.28 + 0.66 × (MCH−27.0)/3.9 + 0.98), Telmissani MCHD (MCH/MCV), Telmissani MDHL (MCH × RBC/MCV), Thal-index (Nishad) (0.615 × MCV) + (0.51 × MCH) + (0.446 × RDW), Wongprachum (MCV × RDW/RBC)−10Hb), Zaghloul I (Hb + Hct + RBC), Zaghloul II (Hb + Hct + RBC−RDW).
2.1 Inclusion and exclusion study criteria
• Inclusion criteria:
○ A complete blood count (CBC) analysis of children with microcytic anemia according to CALIPER hematology reference standards (3).
○ Age of children included ranged from 6 months to 18 years.
○ All patients with β-thal were non-transfusion dependant (NTDT) homozygous or compound heterozygous beta + thalassemia or heterozygous beta0+ thalassemia variants.
• Exclusion criteria:
○ Children under 6 months of age with low serum iron levels,
○ Children with iron supplementation therapy prior to inclusion into the study
○ Children with β-thal and low iron levels which could reduce the HbA2 level
○ Children receving recent blood transfusions
○ Children with other diseases causing microcytic hypochromic anemia (inflammatory, malignant, metabolic, cardiac, nephrological, or gastroenterological diseases, children with chronic illnesses, surgical procedures in children immediately before taking a blood sample for analysis),
CBC and reticulocyte analysis were performed on Sysmex XN-3000 automated blood analyzer for the values of red blood cell count (RBC), hemoglobin (Hb), hematocrit (Hct), mean corpuscular volume (MCV), mean corpuscular hemoglobin (MCH), mean corpuscular hemoglobin concentration (MCHC), red cell distribution width (RDW), reticulocytes (RTIC, per mille and absolute 1012 /L). Peripheral blood samples were obtained by standard venipuncture. The osmotic fragility test assessed red cell osmotic fragility. Hb A, Hb A2, Hb F, and Hb variants were detected using an automated capillary electrophoresis system alongside high-performance liquid chromatography (HPLC) and isoelectric focusing (IEF) for confirmation. Hb A2 values >4% or hemoglobin HbF > 5% indicated β-thal carriers, while HbA values >4% with HbF up to 50% indicated NTDT β-thal. These complementary methods were used simultaneously in the routine laboratory work-up of every sample to ensure that no methodologic or clerical errors were made. IEF and capillary electrophoresis avoided the insufficient distinguishing of Hb Lepore vs. Hb A2 by HPLC due to overlapping retention times. Using manual IEF, we ensured the sharpness of the bands on the gel. The main disadvantage of capillary electrophoresis is the poor separation of Hb S vs. Hb D. To avoid the disadvantages of each method, we decided that all three methods should give the same positive results for β-thal. Iron deficiency anemia was diagnosed by measuring serum iron (Fe), ferritin (FER) [Ferritin ELISA Kit, Demeditec Diagnostics, GmbH], unsaturated iron binding capacity (UIBC), and total iron binding capacity (TIBC). Serum iron levels <4 μmol/L and serum ferritin levels <15 ng/dl indicate IDA (4). Since IDA can reduce HbA2 levels, hemoglobin variant analysis was repeated after IDA was corrected (5). DNA analysis was performed if protein-based methods revealed an unknown variant or ambiguous result. Gene-specific PCR analysis of pediatric Croatian patients was achieved by Q5 High-Fidelity PCR Kit (New England Biolabs) according to the manufacturer's instructions. Direct DNA sequencing of the amplified PCR products was done at Macrogene Europe. DNA analysis revealed the following hemoglobin subunit β-gene mutations: IVS-I-2 (T->C) (HBB:c.92 + 2T>C); 5′UTR; + 20 (C>T) (HBB:c.-31C>T); IVS-II-745 (G->C) (HBB:c.316-106C>G); IVS-I-110 (G->A)beta + (HBB:c.93-21G>A); IVS-I-1(G->A) (HBB:c.92 + 1G>A); IVS-II-666 C>T(HBB:c.316-185C>T); IVS-II-16 G>C (HBB:c.315 + 16G>C); IVS-II-666 C>T (HBB:c.316-185C>T); HBB:c.9T>C; HBB:c.315 + 74T>G; c.316-135het_dupT; c.316-133A>G; c.93-54G>A; c.316-68_316-67het_insCGG; c.316-342delA; c.316-312delT; c.316-209delT. The following mutations in the independent test sample were determined International Reference Laboratory for Haemoglobinopathies, Research Centre for Genetic Engineering and Biotechnology (RCGEB) “Georgi D. Efremov,” Skopje, Republic of Macedonia. Genomic DNA was isolated following a standard phenol extraction/ethanol precipitation protocol. DNA analysis for β-globin gene mutations was performed via ABI PRISM SNaPshot Multiplex Kit (Life Technologies) and ABI PRISM™ Big Dye Terminator v.1.1 Kit (Life Technologies, Carlsbad, CA, USA) following manufacturer's instructions (6). The following hemoglobin subunit β-gene mutations were found: IVS-I-I, IVS-I-110 G>A, IVS-I-6, c.118C>T (Cd39 C>T), c.20delA (Cd6-A), c.118C>T (Cd39 C>T), c.316-2A>G (IVS-II-897A>G), IVS-I-110 G>A, c.250delG (Cd82/83-G) ааа(anti 3.7) triplication, c.92 + 1G>A, c.118 C>T, g.34164_37967del3804 [a(-3.7)/aa], g.70676G>T (Cd27 G>T, Hb Knossos), c.93-21G>A (IVS-I-110 G>A), g.63489del [Cd59(-A)], g.70796G>A (IVS-I-110 G>A).
Informed parental consent was obtained in accordance with the Declaration of Helsinki.
2.2 Statistical analysis
The data analyzed were summarized as numbers and percentages. Quantitative data were summarized as the arithmetic mean and standard deviation. Depending on the data distribution, the data was analyzed using either parametric or nonparametric tests. Values of sensitivity, specificity, positive predictive value, negative predictive value, accuracy, Youden index, and area under the curve (AUC with 95% confidence limits) were used to assess the performance of individual formulasand indices. All applied tests were two-way; and p values ≤ 0.05 were considered statistically significant. The AIC (Akaike's Information Criterion) information criterion was used to select variables in the logistic regression model (7). Statistical analysis was performed in MedCalc ver. 19.07, StatSoft Statistica 12.5, and GraphPad Prism 8.4.3.686 (8, 9). Logistic regression analysis was performed in R version 4.1.3 (10). The optimal cut-off value for each formulaand index was based on the value of the area under the ROC curve as the value closest to the value of the area under the ROC curve in which the difference between the sensitivity and specificity was minimal (11).
3 Results
A total of 271 children of both genders were enrolled in the study, with 150 diagnosed with β-thal and 121 with IDA. All children with β-thal had non-transfusion dependant (NTDT) β-thal (β+/β+, β0/β+) or β-thal trait (β/β0, β/β+). There was no significant male-to-female ratio difference between groups (χ2−test p = 0.142). Children with IDA were younger than children with β-thal (median age 18 months for IDA vs. 74 months for β-thal). β-thal children had higher values of RBC, Hb, HCT, MCH, MCHC, and reticulocyte count (RTIC × 1012/L) than children with IDA. In contrast, children with IDA had higher RDW and MCV values than those with β-thal (p < 0.01). Values of MCV and RTIC (per mille) showed no differences between groups (p > 0.05). The results are shown in Table 1.
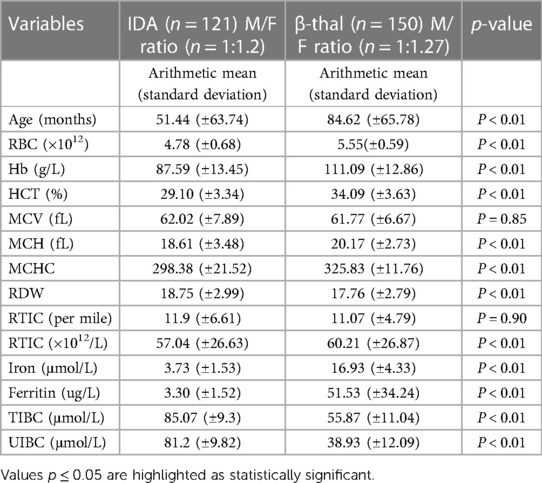
Table 1. Descriptive statistics comparing age, RBC, Hb, HCT, MCV, MCH, MCHC, RDW, RTIC (per mille), and RTIC (×1012 /L) in children with β-thal and IDA (Mann–Whitney U test).
The performance of formulas and indices was tested with already published and new optimal cut-off values generated by ROC analysis.
Two formulas/indices with accuracy >80% using published cut-offs were (in descending order): Matos-Carvalho index and England and Fraser (Supplementary Table S1). The most accurate previously published index was the Matos-Carvalho index, both with with its original cut-off value for adults as well as an optimal cut-off value for children. With the previously published cut-off value, this index better distinguishes children with IDA than children with β-thal. With an optimal cut-off value, the accuracy of the Matos-Carvalho index in distinguishing β-thal increased (sensitivity 89.94%, specificity 93.75%). Therefore, the overall proportion of total misclassified patients is reduced and the index has better sensitivity and specificity.
The accuracy of many formulas and indices improved with the new optimal cut off values. Diagnostic accuracy of 10 indices, previously below 80%, increased with new optimal cut-off values above 80%. The accuracy of Pornprasert and RBC indices increased by more than >50% in comparison with published cut-off values. (Supplementary Table S2; Figure S1). Formulas and indices with optimal cut-off and accuracy between <90% and ≥80% are (in descending order): Telmissani (MDHL) formula, England and Fraser formula, Pornprasert (MCHC) index, Sirachainan index, Telmissani (MCHD) formula,CRUISE index, Hameed index,Sargolzaie formula, and Zaghloul II index. They became thereafter acceptable for distinguishing β-thal from IDA (Supplementary Table S2).
As we were not fully satisfied with the overall performance of the aforementined formulas and indices (primarily with sensitivity), we tried to improve diagnostic accuracy by creating a new model(s) suited for children. We applied binary classification using logistic regression (R for Windows 4.1.3). From a series of models generated using R.4.1.3, the most optimal ones were selected using AIC by combined addition and subtraction of variables. The variables used in the CroThalDD-LM1 model are MCH, MCV, and Hb. The model has the following calculation formula:
where exp(y) = ey, y = β0 + β1 × 1 + β2 × 2 + β3 × 3. ×1 = MCH, ×2 = MCV, and ×3 = Hb. β0, β1, β2, β3 are coefficients of the logistic regression model (CroThalDD-LM1 index) shown in Table 2. The number calculated by CroThalDD-LM1 is between 0 and 1. The cut-off value is 0.5, and a number closer to 0 indicates the diagnosis of IDA, while a number closer to 1 indicates β-thal. Three variables built a model more accurate than any previous formulas/indices (composite indices included) with a sensitivity of 94.87%, specificity of 93.51%, accuracy of 94.10%, and AUC of 0.986 (95% CI 0.975–0.997) (Table 2).
The 2nd formula generated via logistic regression uses the same variables as the Matos-Carvalho index (RBC and MCHC) with the addition of reticulocytes. The formula reads: exp(y) = ey, y = β0 + β1 × 1 + β2 × 2 + β3 × 3. ×1 = RBC, ×2 = MCHC, ×3 = RTIC (×1012 /L), β0, β1, β2, β3 are coefficients of the logistic regression model (CroThalDD-LM2 index) shown in Table 3. Three variables built a model with a sensitivity of 95.74%, specificity of 97.56%, accuracy of 96.77%, and AUC of 0.985 (95% CI 0.988–0.999). The addition of RTIC (absolute count) increased the overall accuracy of the model.

Table 3. The CroThalDD-1 formula tested on an independent sample of β-thal from The Republic of North Macedonia.
To simplify the use of the model in everyday clinical practice, we present Microsoft Office Excel and LibreOffice Calc spreadsheets, which use this model (presented in the Supplementary Excel spreadsheet). In this way, the user only needs to enter the values of MCH, MCV, Hb, MCHC RBC, and RTIC (×1012 /L), and the spreadsheets automatically calculate the probability of belonging to each class.
The practitioner can also calculate the sum of the values obtained by the simplified formula y = β0 + β1 × 1 + β2 × 2 + β3 × 3 without additional calculation with the exponential equation.
The simplified CroThalDD-LM1 index calculation formula would be:
The simplified CroThalDD-LM2 index calculation formula would be:
In this case, the cut-off value has changed to 0. Values below 0 belong to IDA and above 0 to β-thal.
A summary of AUC values (with CI) of 41 formulas/indices, 2 composite indices, and novel CroThalDD-LM1 and CroThalDD-LM2 indices are shown in Figure 1. The ten best indices have accuracy above 88% (marked by the dotted line on the x-axis).
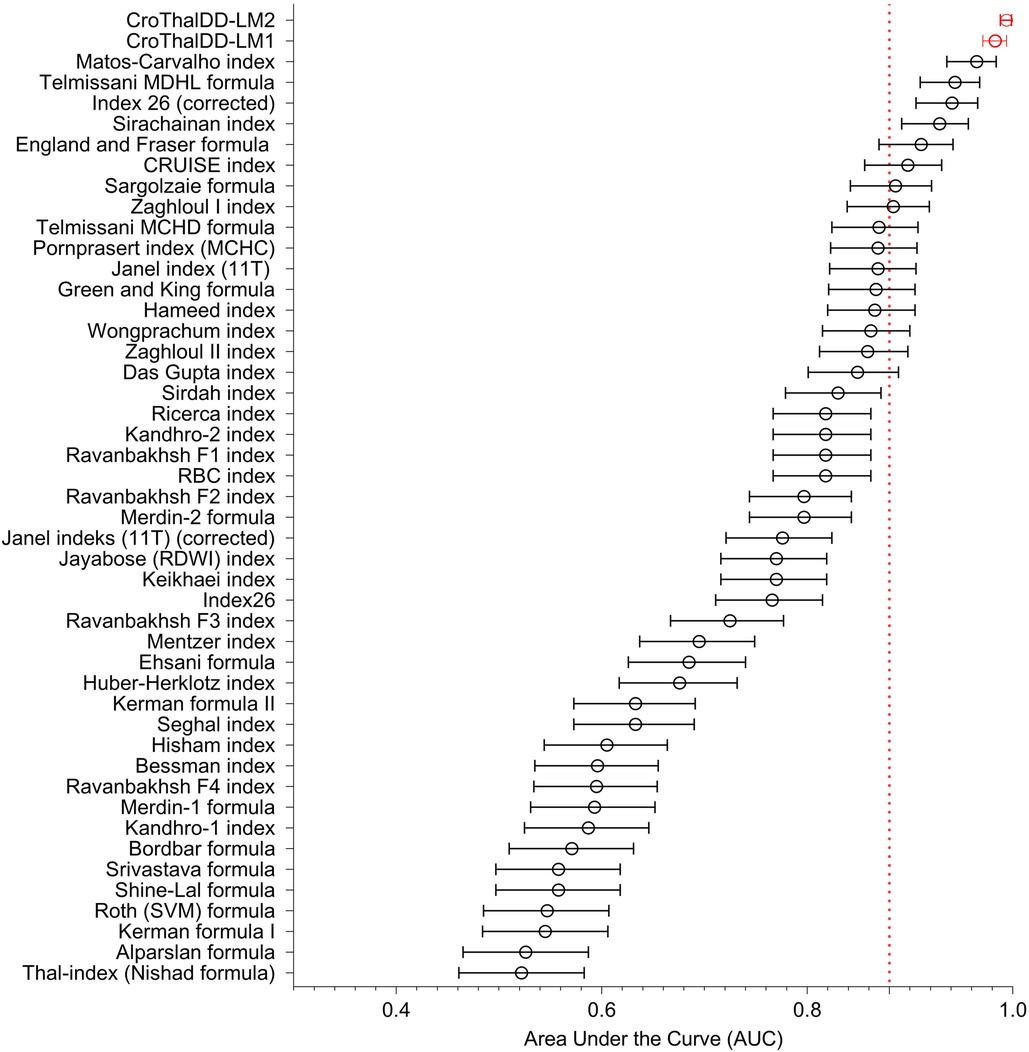
Figure 1. A summary of AUC values (with CI) of 41 formulas/indices, 2 composite indices and novel CroTHalDD-LM1 and CroThalDD-LM2 indices. The ten best indices have accuracy above 88% (marked by the dotted line on the x-axis).
The CroThalDD-1 index was additionally tested on a different population of children from the Republic of North Macedonia unrelated to the Croatian population. These populations are genetically different (12). A slight decrease in sensitivity and specificity was noticed but with still high accuracy. The results are shown in Table 3. Due to the lack of RTIC in the North Macedonian blood samples, the CroThalDD-2 index was not tested.
4 Discussion
Since the appearance of the Mentzer index several decades ago, attempts have been made to find a universal and reliable formula or index to distinguish β-thal from IDA. Sensitivity, specificity, PPV, NPV, accuracy, AUC, and Youden index were analyzed in most discrimination formulas and indices, but their performance differed worldwide. This is especially true for children, for whom the diagnostic accuracy of previously published discrimination formulasand indices showed lower performancethan in adults. Differential diagnosis is especially challenging when both IDA and β-thal are present simultaneously. Elevated erythropoietin levels due to sustanined anemia in β-thal stimulate the release of erythroferrone through erythroblasts (13, 14). This increase in erythroferrone, in turn, supresses hepcidine expression. The resulting increased intestinal iron absorption and with the release of recycled iron from the reticuloendothelial system lead to portal and hepatocyte iron loading, and free circulating iron causes organ damage. Therefore, additional unnecessary long-term iron supplementation leads to iron accumulation and with potential deleterious side effects.
IDA in children becomes clinically apparent at the age of 12 months, and the American Academy of Pediatrics recommends screening infants around 1 year of age due to reduced iron storage. NTDT β-thal typically presents between the ages of 2–4 years. Our results correspond to these age groups (15, 16). A higher RDW value of IDA indicates more significant heterogeneity (anisocytosis) of β-thal, with higher or average RDW values (17). In the case of progressing or long-standing IDA, RBC count and MCV may be decreased due to fewer and smaller erythrocytes being produced in IDA due to depletion of iron stores vs. β-thal.
Most formulasand indices have been generated and tested in adult populations with various discriminating performances in children. Indices with ≥90% accuracy were rated as the most accurate and recommended in a clinical setting for distinguishing β-thal from IDA in Croatian children. Indices with an accuracy between ≥80% and <90% can help to differentiate between these two diseases. Indices with accuracy <80% were not recommended due to many formulasand indices with better performances.
The Matos-Carvalho index has proven to be the most accurate of all formulas and indices in Croatian children, even with the previously published cut-off value described in the literature (18). CBC values on which the initial index was created differ from those in Croatian children, as one depends on the genetic background of patients with β-thal. Due to Croatia's association with the Mediterranean population, high accuracy of the Matos-Carvalho index is expected. A similar performance of the Matos-Carvalho index was observed in the adult Egyptian population (19). This index has not been previously tested in children.
The second-best index with an optimal cut-off value was Telmissani (MDHL) index. The performance rating of our study corresponds to a study of adult patients conducted in Saudi Arabia (21). Both Telmissani indices (MDHL and MCHD) with optimal cut-off values can be recommended for routine tesing in children. They have not been tested in children so far as well. With the published cut-off, the England and Fraser index had the second-best performance rating (22, 23). The original cut-off value of the index showed excellent sensitivity, NPV, and accuracy but insufficient specificity and PPV. After defining the optimal cut-off value and performance improvement, the accuracy of the index increased due to improved specificity and PPV, and it ranked fifth best in terms of performance. England and Fraser formula was previously tested in the population of children in Turkey and had a third-best-ranked performance (below RDWI and RBC indices) (24, 25). This index also proved third best in Chinese children (below RDWI and Green and King indices) (26).
The Pornprasert index uses only MCHC as a discrimination value (27). After defining the optimal cut-off value, its accuracy increased by 65% due to an increase in sensitivity and specificity. The index was created for Thai school children; therefore, a favorable result of the discriminatory performance of this index among Croatian children for the two diseases is expected. Since our study population encompasses toddlers and preschool children, an MCHC cut-off value >309 corresponds with the diagnosis of β-thal in the entire population of children. Thai and Croatian populations have different median ages for β-thal, so the difference in cut-off value is understandable. The Sirachainan index was also generated on a population of healthy school children, and excellent initial performance of this index was also expected (28). After defining the optimal cut-off value, its accuracy improved substantially by 13.92%, and the index proved valuable in distinguishing children with β-thal from children with IDA.
The last four indices which improved accuracy after defining optimal cut-off values >80% were CRUISE, Hameed, Sargolzaie, and Zaghloul II. After defining the optimal cut-off value, the CRUISE index outperformed the formulas/indices initially developed for the adult Iranian population (29). Hameed index with the new cut-off value showed balanced sensitivity and specificity with acceptable accuracy (19). After defining the optimal cut-off value, the Sargolzaie index also significantly increased its accuracy (30). Defining the cut-off values of the Zaghloul II index in children (regardless of gender), the index showed a significant improvement in accuracy mainly due to its increase in specificity and PPV (31). All of these indices have not been tested in children so far.
Using linear regression components embedded in the logit scale, logistic regression iteratively identifies the combination of variables with the most significant probability of discriminating between β-thal and IDA. Logistic regression analysis generated two new discriminations indices distinguishing children with β-from IDA in children with the highest sensitivity, specificity, and accuracy of all formulas/indices, as mentioned earlier.
The RTIC count (per mille and absolute reticulocyte number) is high in the case of active β-thal but also severe IDA (20) due to erythropoietin stimulation. A significanlty higher reticulocyte count was already observed in NTDT thalassemia patients, in comparison with IDA (32). Since the Matos-Carvalho index shows the best results in our children, we put to the test the performance of the variables of this index (RBC, MCHC) with the addition of RTIC. The result is the new CroThalDD-LM2 index. Adding RTIC usually improves the performance of some of the already published formulas/indices.
The results of our study are logistic model-generated indices, the first discrimination indices designed precisely for the pediatric population, including preschool children. To make the calculation with the exponential equation more accessible, we created Excel and Libre Office spreadsheets. Even though CroThalDD-LM2 has better accuracy, sensitivity, and CI, we suggest using both novel formulas/indices to see which has the advantage in practical application. Although these indices are derived from Croatian children database, we encourage their use in other children populations, even adults.
We tested all available formulas/indices of β-thal of native adult origin and adopted them for use in our children population. We hope that our results will help practitioners already accustomed to native formulas/indices to improve their reliability of β-thal diagnostics in children. All modified cut-offs of such native formulas/indices are listed in the Supplementary Files S1. A comparison between such native formulas/indices and our novel-generated indices is very welcome (33–35).
4.1 Limitations of the study
The indices using the average reticulocyte cell volume, the average reticulocyte hemoglobin content, % Micro-R, or mean platelet volume to separate β-thal from IDA were not analyzed as they are not routine in routine analysis in Croatia or North Macedonia (36, 37). We encountered only four children with α-thalassemia trait, and therefore, we cannot support the usability of these indicies in α-thalassemia trait. Further research is required.
5 Conclusions
All tested formulas/indices which are generated worldwide were tested and modified on the children population. The Matos-Carvalho index shows the best diagnostic performance for distinguishing β-thal from IDA in children, We recommend the use of formulas/indices with modified cut-offs with a performance of >90% accuracy. We generated two novel indices from the children in order to reflect the peculiarities in β-thal diagnostics in such populations, which were observed by previous authors by using formulas/indices generated from the adults. A comparison of both native and our indices is encouraged.
Data availability statement
The raw data supporting the conclusions of this article will be made available by the authors, without undue reservation.
Ethics statement
The studies involving humans were approved by University Hospital Centre Zagreb. The studies were conducted in accordance with the local legislation and institutional requirements. Written informed consent for participation in this study was provided by the participants’ legal guardians/next of kin.
Author contributions
DT: Conceptualization, Data curation, Formal Analysis, Investigation, Methodology, Resources, Software, Validation, Visualization, Writing – original draft, Writing – review & editing. JV: Conceptualization, Data curation, Formal Analysis, Investigation, Methodology, Resources, Software, Validation, Visualization, Writing – original draft, Writing – review & editing. SK: Data curation, Resources, Validation, Writing – review & editing. DM: Conceptualization, Formal Analysis, Investigation, Supervision, Writing – original draft, Writing – review & editing. EB: Conceptualization, Data curation, Formal Analysis, Resources, Supervision, Writing – original draft, Writing – review & editing.
Funding
The author(s) declare financial support was received for the research, authorship, and/or publication of this article.
The authors have received funding from The Croatian cooperative group for hematologic diseases (KROHEM) for the funding of this article.
Acknowledgments
The article was written with the support of the Croatian Cooperative Group for Hematological Diseases (KROHEM).
Conflict of interest
The authors declare that the research was conducted in the absence of any commercial or financial relationships that could be construed as a potential conflict of interest.
Publisher's note
All claims expressed in this article are solely those of the authors and do not necessarily represent those of their affiliated organizations, or those of the publisher, the editors and the reviewers. Any product that may be evaluated in this article, or claim that may be made by its manufacturer, is not guaranteed or endorsed by the publisher.
Supplementary material
The Supplementary Material for this article can be found online at: https://www.frontiersin.org/articles/10.3389/fped.2023.1258054/full#supplementary-material
References
1. Kranjčec I, Matijašić Stjepović N, Buljan D, Ružman L, Malić Tudor K, Jović Arambašić M, et al. Management of childhood iron deficiency anemia in a developed country—a multi-center experience from Croatia. Diagnostics. (2023) 13:3607. doi: 10.3390/diagnostics13243607
2. Ferih K, Elsayed B, Elshoeibi AM, Elsabagh AA, Elhadary M, Soliman A, et al. Applications of artificial intelligence in thalassemia: a comprehensive review. Diagnostics (Basel). (2023) 13(9):1551. doi: 10.3390/diagnostics13091551
3. Higgins V, Tahmasebi H, Bohn MK, Hall A, Adeli K. CALIPER hematology reference standards (II). Am J Clin Pathol. (2020) 154(3):342–52. doi: 10.1093/ajcp/aqaa057
4. Brancaleoni V, Di Pierro E, Motta I, Cappellini MD. Laboratory diagnosis of thalassemia. Int J Lab Hematol. (2016) 38(Suppl 1):32. doi: 10.1111/ijlh.12527
5. Harthoorn-Lasthuizen EJ, Lindemans J, Langenhuijsen MM. Influence of iron deficiency anaemia on haemoglobin A2 levels: possible consequences for beta-thalassaemia screening. Scand J Clin Lab Invest. (1999) 59(1):65–70. doi: 10.1080/00365519950186011
6. Atanasovska B, Bozhinovski G, Chakalova L, Kocheva S, Karanfilski O, Plaseska-Karanfiska D. Molecular diagnostics of β-thalassemia. Balkan J Med Genet. (2012) 15(Suppl):61–5. doi: 10.2478/v10034-012-0021-z
7. Jessop A. Let the Evidence Speak: Using Bayesian Thinking in Law, Medicine, Ecology and other Areas. Cham: Springer (2018).
9. TIBCO Statistica, v. 12.5.0, TIBCO Software Inc, Palo Alto, CA, USA. (2017). Available at: https://www.tibco.com/products/tibco-statistica (Accessed May 07, 2023).
10. R Core Team. R: A language and environment for statistical computing. R Foundation for Statistical Computing, Vienna, Austria. (2020). Available at: https://www.R-project.org/ (Accessed May 07, 2023).
11. Unal I. Defining an optimal cut-point value in ROC analysis: an alternative approach. Comput Math Methods Med. (2017) 2017:3762651. doi: 10.1155/2017/3762651
12. Dimovski A, Efremov DG, Jankovic L, Juricic D, Zisovski N, Stojanovski N, et al. Beta-thalassemia in Yugoslavia. Hemoglobin. (1990) 14(1):15–24. doi: 10.3109/03630269009002251
13. Coffey R, Ganz T. Erythroferrone: an erythroid regulator of hepcidin and iron metabolism. Hemasphere. (2018) 2(2):e35. doi: 10.1097/HS9.0000000000000035
14. Shash H. Non-transfusion-dependent thalassemia: a panoramic review. Medicina (Kaunas). (2022) 58(10):1496. doi: 10.3390/medicina58101496
15. Subramaniam G, Girish M. Iron deficiency anemia in children. Indian J Pediatr. (2015) 82(6):558–64. doi: 10.1007/s12098-014-1643-9
16. Rachmilewitz EA, Giardina PJ. How I treat thalassemia. Blood. (2011) 118(13):3479–88. doi: 10.1182/blood-2010-08-300335
17. Flynn MM, Reppun TS, Bhagavan NV. Limitations of red blood cell distribution width (RDW) in evaluation of microcytosis. Am J Clin Pathol. (1986) 85(4):445–9. doi: 10.1093/ajcp/85.4.445
18. Matos JF, Dusse LM, Borges KB, de Castro RL, Coura-Vital W, Carvalho M. A new index to discriminate between iron deficiency anemia and thalassemia trait. Rev BrasHematolHemoter. (2016) 38(3):214–9. doi: 10.1016/j.bjhh.2016.05.011
19. Hamed EO, El-Deen AF. Simple red cell indices in screening an discrimination of iron deficiency anemia and beta thalassemia trait in Egyptian patients. OJBD. (2019) 9:9–19. doi: 10.4236/ojbd.2019.91002
20. Hoffmann JJML, Urrechaga E. Verification of 20 mathematical formulas for discriminating between iron deficiency anemia and thalassemia trait in microcytic anemia. Lab Med. (2020) 51(6):628–34. doi: 10.1093/labmed/lmaa030
21. Telmissani OA, Khalil S, Roberts GT. Mean density of hemoglobin per liter of blood: a new hematologic parameter with an inherent discriminant function. Lab Hematol. (1999) 5:149–52.
22. England JM, Fraser PM. Differentiation of iron deficiency from thalassaemia trait by routine blood-count. Lancet. (1973) 1(7801):449–52. doi: 10.1016/s0140-6736(73)91878-3
23. England JM, Fraser P. Discrimination between iron-deficiency and heterozygous-thalassaemia syndromes in differential diagnosis of microcytosis. Lancet. (1979) 1(8108):145–8. doi: 10.1016/s0140-6736(79)90532-4
24. Demir A, Yarali N, Fisgin T, Duru F, Kara A. Most reliable indices in differentiation between thalassemia trait and iron deficiency anemia. Pediatr Int. (2002) 44(6):612–6. doi: 10.1046/j.1442-200x.2002.01636.x
25. Düzenli Kar Y, Özdemir ZC, Emir B, Bör Ö. Erythrocyte indices as differential diagnostic biomarkers of iron deficiency anemia and thalassemia. J Pediatr Hematol Oncol. (2020) 42(3):208–13. doi: 10.1097/MPH.0000000000001597
26. Shen C, Jiang YM, Shi H, Liu JH, Zhou WJ, Dai QK, et al. Evaluation of indices in differentiation between iron deficiency anemia and beta-thalassemia trait for Chinese children. J Pediatr Hematol Oncol. (2010) 32(6):e218–22. doi: 10.1097/MPH.0b013e3181e5e26e
27. Pornprasert S, Panya A, Punyamung M, Yanola J, Kongpan C. Red cell indices and formulas used in differentiation of β-thalassemia trait from iron deficiency in Thai school children. Hemoglobin. (2014) 38(4):258–61. doi: 10.3109/03630269.2014.930044
28. Sirachainan N, Iamsirirak P, Charoenkwan P, Kadegasem P, Wongwerawattanakoon P, Sasanakul W, et al. New mathematical formula for differentiating thalassemia trait and iron deficiency anemia in thalassemia prevalent area: a study in healthy school-age children. Southeast Asian J Trop Med Public Health. (2014) 45(1):174–82.24964667
29. Jahangiri M, Rahim F, Malehi AS. Diagnostic performance of hematological discrimination indices to discriminate between βeta thalassemia trait and iron deficiency anemia and using cluster analysis: introducing two new indices tested in Iranian population. Sci Rep. (2019) 9(1):18610. doi: 10.1038/s41598-019-54575-3
30. Sargolzaie N, Miri-Moghaddam E. A local equation for differential diagnosis of β-thalassemia trait and iron deficiency anemia by logistic regression analysis in Southeast Iran. Hemoglobin. (2014) 38(5):355–8. doi: 10.3109/03630269.2014.948187
31. Zaghloul A, Al-Bukhari TA, Bajuaifer N, Shalaby M, Al-Pakistani HA, Halawani SH, et al. Introduction of new formulas and evaluation of the previous red blood cell indices and formulas in the differentiation between beta thalassemia trait and iron deficiency anemia in the Makkah Region. Hematology. (2016) 21(6):351–8. doi: 10.1080/10245332.2015.1133753
32. Noronha JF, Grotto HZ. Measurement of reticulocyte and red blood cell indices in patients with iron deficiency anemia and beta-thalassemia minor. Clin Chem Lab Med. (2005) 43(2):195–7. doi: 10.1515/CCLM.2005.033
33. Urrechaga E, Borque L, Escanero JF. Erythrocyte and reticulocyte parameters in iron deficiency and thalassemia. J Clin Lab Anal. (2011) 25(3):223–8. doi: 10.1002/jcla.20462
34. Miri-Moghaddam E, Sargolzaie N. Cut off determination of discrimination indices in differential diagnosis between iron deficiency anemia and β-thalassemia minor. Int J Hematol Oncol Stem Cell Res. (2014) 8(2):27–32.24800036
35. Bordbar E, Taghipour M, Zucconi BE. Reliability of different RBC indices and formulas in discriminating between β-thalassemia minor and other microcytic hypochromic cases. Mediterr J Hematol Infect Dis. (2015) 7:e2015022. doi: 10.4084/MJHID.2015.022
36. Paokanta P, Harnpornchai N. Risk analysis of thalassemia using knowledge representation model: diagnostic Bayesian networks. 2012 IEEE-EMBS international conference on biomedical and health informatics (BHI) (2012). p. 155–8
Keywords: β-thalassemia, iron deficiency anemia, discrimination index, classification, generalized linear models (GLM), children
Citation: Turudic D, Vucak J, Kocheva S, Milosevic D and Bilic E (2024) Differentinating between non-transfusion dependant β-thalassemia and iron deficinecy anemia in children using ROC and logistic regression analysis: two novel discrimination indices designed for pediatric patients. Front. Pediatr. 11:1258054. doi: 10.3389/fped.2023.1258054
Received: 13 July 2023; Accepted: 21 December 2023;
Published: 16 January 2024.
Edited by:
Giulia Ceglie, Bambino Gesù Pediatric Hospital (IRCCS), ItalyReviewed by:
Joseph Louis Lasky, Cure 4 The Kids, United StatesMarcelo Gerardin Poirot Land, Federal University of Rio de Janeiro, Brazil
© 2024 Turudic, Vucak, Kocheva, Milosevic and Bilic. This is an open-access article distributed under the terms of the Creative Commons Attribution License (CC BY). The use, distribution or reproduction in other forums is permitted, provided the original author(s) and the copyright owner(s) are credited and that the original publication in this journal is cited, in accordance with accepted academic practice. No use, distribution or reproduction is permitted which does not comply with these terms.
*Correspondence: Daniel Turudic ZGFuaWVsdHVydWRpY0BnbWFpbC5jb20=
†These authors have contributed equally to this work and share first authorship