- 1Department for Community Health, Faculty of Science and Medicine, University of Fribourg, Fribourg, Switzerland
- 2Department of Paediatrics, Fribourg Hospital, Fribourg, Switzerland
- 3Istanbul Faculty of Medicine, Istanbul University, Istanbul, Türkiye
- 4Department of Paediatrics, The University of Melbourne, Parkville, VIC, Australia
- 5Infectious Diseases Research Group, Murdoch Children’s Research Institute, Parkville, VIC, Australia
- 6Pediatric Infectious Diseases Unit, Geneva University Hospitals and Faculty of Medicine, Geneva, Switzerland
- 7Microbiology Laboratory, Fribourg Hospital, Fribourg, Switzerland
- 8Department of Biology, University of Fribourg and Swiss Institute of Bioinformatics, Fribourg, Switzerland
- 9Infectious Diseases Unit, The Royal Children’s Hospital Melbourne, Parkville, VIC, Australia
Introduction: The intestinal microbiome forms a major reservoir for antibiotic resistance genes (ARGs). Little is known about the neonatal intestinal resistome.
Objective: The objective of this study was to investigate the intestinal resistome and factors that influence the abundance of ARGs in a large cohort of neonates.
Methods: Shotgun metagenomics was used to analyse the resistome in stool samples collected at 1 week of age from 390 healthy, term-born neonates who did not receive antibiotics.
Results: Overall, 913 ARGs belonging to 27 classes were identified. The most abundant ARGs were those conferring resistance to tetracyclines, quaternary ammonium compounds, and macrolide-lincosamide-streptogramin-B. Phylogenetic composition was strongly associated with the resistome composition. Other factors that were associated with the abundance of ARGs were delivery mode, gestational age, birth weight, feeding method, and antibiotics in the last trimester of pregnancy. Sex, ethnicity, probiotic use during pregnancy, and intrapartum antibiotics had little effect on the abundance of ARGs.
Conclusion: Even in the absence of direct antibiotic exposure, the neonatal intestine harbours a high abundance and a variety of ARGs.
Introduction
The intestinal microbiome is a complex ecosystem with a large and diverse community of microbes. This forms a major reservoir for antibiotic resistance genes (ARGs), which is also called the intestinal resistome (1–3). Neonates are rapidly colonised after birth, acquiring their intestinal microbiome from their mothers and other contacts, through milk and from the environment. The most abundant bacterial families in the neonatal intestine are Enterobacteriaceae, Bifidobacteriaceae, Bacteroidaceae, Staphylococcaceae, and Clostridiaceae (4). These bacteria are a major reservoir of clinically relevant ARGs (5–7). Antibiotics influence the composition of intestinal microbiome and resistome (8) but ARGs can also be detected in the absence of antibiotic treatment (9, 10).
Neonates are highly vulnerable to infections, which are often associated with significant morbidity and mortality. Antibiotic-resistant, especially multidrug-resistant, bacteria pose a major threat to neonatal health. It is estimated that, annually, over 200,000 neonatal deaths occur due to infections with antibiotic-resistant pathogens (11). Moreover, infections with antibiotic-resistant pathogens are also associated with higher rates of complications, prolonged hospital stay, increased cost, and worse outcomes. Little is known about the neonatal intestinal resistome.
In this study, we used shotgun metagenomics to investigate the intestinal resistome and the factors that influence the abundance of ARGs in a large cohort of neonates.
Methods
Study design and participants
A subset of neonates from The Melbourne Infant Study: BCG for Allergy and Infection Reduction (MIS BAIR) who did not receive antibiotics during the first week of life were included (12). In the MIS BAIR trial, 1,272 healthy neonates were randomised to Bacille Calmette–Guérin (BCG) immunisation or not to investigate whether the vaccine protects against childhood infection, allergy, and asthma. Inclusion criteria were: gestational age greater than 32 weeks, birth weight greater than 1,500 g, absence of symptoms or signs of illness, and no contraindication to BCG. Data on demographics, pregnancy, and the perinatal period were collected through questionnaires.
Stool collection
On each of the first 10 days of life, stool samples from the first bowel movement of the day were collected into empty sterile tubes by parents (with date and time recorded), who were given detailed oral and written instructions about the collection technique. Samples were stored in domestic freezers (−18°C or below). They were collected from parents’ home by a study team member and kept frozen during transportation to the laboratory, where they were aliquoted for storage at −80°C. One stool sample collected between day 5 and 7 of life was analysed for each neonate.
DNA extraction and metagenomic shotgun sequencing
Aliquots of stools were thawed at room temperature and 100 mg of stool was used for DNA extraction. The FastDNA™ SPIN Kit for Soil (MP Biomedicals, Illkirch-Graffenstaden, France) was used according to manufacturer's instructions. DNA concentrations were measured using Qubit™ dsDNA High Sensitivity Assay kits (Life technologies, California, United States). The mean DNA concentration in samples was 367 ng; the DNA in the negative control was below the detection limit (<0.01 ng/µl) and was therefore not sequenced. Sequencing libraries with a final insert size of approximately 600 bp were prepared with Nextera DNA Flex library preparation kits (Illumina, San Francisco, United States). Illumina PhiX DNA was added to the libraries. Paired sequencing (2 × 149 bp) was done on a NextSeq 550 system (Illumina) with high-output flow cells. Bacterial and fungal even-distributed mock communities (Gut Microbiome Whole cell Mix MSA-2006™ and Mycobiome Whole Cell Mix, respectively, ATCC, Manassas, United States) were sequenced as positive controls.
Bioinformatic analyses
Quality filtering and removal of human-mapping reads
Reads were checked for quality with FastQC v0.11.7 (http://www.bioinformatics.babraham.ac.uk/projects/fastqc/). Filtering of low-quality reads and trimming were done with Trimmomatic v0.39 (13) with the following settings: SLIDINGWINDOW:20:28, MINLEN:100. Sequences mapping to the human genome (vGRCh38.p11) with a confidence score of more than 0.1 were removed with Kraken v2.0.9-beta (14).
Identification of bacteria and bacteriophages
Quality-filtered non-human reads were analysed with Kraken2 and mapped to bacterial and bacteriophagic genomes with confidence score higher than 0.1. Read taxonomic assignment was re-estimated with Bracken v2.6.2 (14, 15) at phylum, family, genus, and species levels.
To calculate the relative abundance of a bacterial taxon in a given sample, the number of reads assigned to the taxon was divided by the total number of reads classified at the same taxonomic level in the sample. The relative abundance of bacteriophages belonging to the Caudovirales order, the most represented taxon in the human intestine (16, 17), was expressed in counts per million (CPM). CPMs were obtained by normalising reads mapping to bacteriophagic genomes with the total number of quality-filtered non-human reads.
Detection of antibiotic resistance genes
ResFinder was used to detect ARGs as the database contains all the major clinically relevant ARGs. Quality-filtered non-human forward (R1) and reverse (R2) FASTQ files were mapped to the ResFinder gene database v4 (18) with bowtie2 v2.3.1 (19) with the following settings: -D 20 -R 3 -N 1 -L 20 -i S,1,0.50 (20). Alignment SAM files were sorted and indexed with SAMtools v1.10 (21). Read counts for a given ResFinder sequence in each sample were obtained with bedtools multicov (BEDTools v2.29.2) (22) with the following settings: -p -q 1.
Read counts were normalised to the total number of reads assigned by Kraken2/Bracken to bacterial species and the length of each ARG sequence (expressed in kb). The ratio was then multiplied by one million. Normalised counts for genes were obtained by adding normalised counts of all the ResFinder sequences of a given ARG. The matching of each ResFinder sequence to a given antibiotic class was obtained from the “phenotype.txt” file contained in the ResFinder database. ARG normalised counts were used for further analyses.
Statistical analysis
Statistics were performed in the R software version 4.2.1. The codes can be found in the Supplementary File 1. The correlation between ARGs and bacterial species composition detected by Kraken2/Bracken was analysed with the Mantel test from the R package vegan v2.6-2 by using 999 permutations. ARG normalised counts and the relative abundance of species were first analysed with the vegdist function by choosing the Horn–Morisita index and obtained distance matrixes were then submitted to the Mantel test.
The bacterial (genus and species) and resistome profiles were analysed by principal coordinate analyses (PCoAs). The relative abundance was square-root-transformed, and a distance matrix was computed based on the Bray–Curtis dissimilarity index with the vegdist function (vegan). The matrix was analysed with the betadisper function (vegan).
The association between different factors and the bacterial (phylum, family, genus, and species) or resistome composition was investigated with permutational multivariate analysis of variance (PERMANOVA) test implemented in the adonis2 function (vegan) by using Bray–Curtis distances and 9,999 permutations.
Ecological indices (richness, Shannon, and Simpson diversity) were computed with the diversity function (vegan). Rarefaction to 35,000 reads was performed for species composition with the rrarefy function (vegan). Differentially abundant taxa were identified by the Wilcoxon rank sum test, and differentially abundant ARGs with DESeq2 v1.36.0 (23). To assess differential abundance of taxa, the median values of relative abundance were used to calculate fold-change, which was then transformed to the log2 scale. This approach enabled comparisons of the abundance of each taxon across multiple samples, while minimising the impact of outliers.
For ARGs, log2-transformed fold changes were provided by DESeq2. Ecological indices were performed on rounded normalised counts of ARGs. DESeq2 was run on pseudo-counts obtained by adding 1 to the rounded normalised counts.
Reference databases
The ResFinder database version 4 consists of 3,139 unique sequences. When adding up the sequences for the same gene (or variants, as for example for blaOXA, blaTEM, etc.), this corresponded to 2,534 ARGs. For Kraken2 analyses, the human genome (vGRCh38.p11), 15,009 bacterial, and 11,833 viral genomes were used. All reference sequences were downloaded from NCBI RefSeq on 3 March 2022.
Ethics
Written informed consent was obtained from parents or guardians of all neonates. The study was approved by the Royal Children's Hospital Human Research Ethics Committee [HREC, authorisation (53543)]. MIS BAIR is registered with the Australian New Zealand Clinical Trials Registry (1051228) and the U.S. National Institutes of Health (NCT01906853).
Results
In total, 390 healthy, term-born neonates who did not receive antibiotics during the first week of life were included. The median gestational age at birth was 39.3 [interquartile range (IQR) 38.4–40.3] weeks and the median birth weight was 3,440 (IQR: 3,122–3,748) grams.
Of these neonates, 33% (129/390) were born by Caesarean section (CS) and 50% (195/390) were female. During the first week of life, 33% (128/390) neonates received formula milk and one neonate received probiotics.
Of the mothers, 18% (71/390) took probiotics during pregnancy and 12% (48/390) received at least one course of antibiotics during the last trimester of pregnancy. Amoxicillin was the most frequently used antibiotic (50%, 30/60), followed by cephalexin (13%, 8/60) and penicillin (7%, 4/60). In total, 23% (90/390) mothers received intrapartum antibiotics. The most frequently used drug was penicillin (52%, 47/90), followed by amoxicillin (33%, 30/90). The main two reasons for intrapartum antibiotic use were anogenital group B Streptococcus carriage (GBS) (58%, 52/90) and prolonged rupture of membranes (34%, 31/90) (Table 1).
Intestinal microbiome composition, richness, and diversity
Delivery mode, gestational age at birth, use of formula milk, and intrapartum antibiotics had a strong effect on the bacterial composition of the intestinal microbiome at all taxonomic levels (PERMANOVA test, p-values <0.05; Table 2 and PCoA plots; Figures S1, S2, Supplementary File S2). Birth weight affected the composition at the family, genus, and species levels (p-values 0.0075, 0.0022, and 0.0012). Probiotic use during pregnancy affected the composition at the phylum level (p-value 0.0413). No association were found between sex, ethnicity, antibiotic use during the last trimester of pregnancy, and the intestinal microbiome composition.
Compared with neonates born by CS, the microbiome of neonates born vaginally had a higher bacterial species richness and diversity (p-values <0.001, 0.006, and 0.015 for richness, Shannon, and Simpson diversity, respectively; Figure S3, Supplementary File S2). Species richness and diversity were also higher in neonates of mothers who did not receive intrapartum antibiotics (p-values 0.002, <0.001, and <0.001 for richness, Shannon, and Simpson diversity, respectively, Figure S3, Supplementary File S2).
There was no difference in the abundance of bacteriophages between neonates born by CS and those born vaginally (median counts per million 1,300, IQR: 407–3,819, vs. 1,890, IQR: 357–5,350, p-value 0.2; Figure S4, Supplementary File S2). A higher abundance of bacteriophages was found in neonates of mothers who received antibiotics during the last trimester of pregnancy (median counts per million 3,021, IQR: 1,073–5,714, vs. 1,444, IQR: 323–4,137, p-value 0.01; Figure S4, Supplementary File S2).
Delivery mode, use of formula milk, probiotic use during pregnancy, antibiotic use during the last trimester of pregnancy, and intrapartum antibiotics were associated with at least 1.5-fold difference in the relative abundance of 47 taxa (4 phyla, 9 families, 13 genera, and 21 species) (p-values <0.05) (Figures S5–S8, Supplementary File S2). The relative abundance of Firmicutes, Bacteroidetes, and Actinobacteria varied according to delivery mode (p-values <0.001; Figure S5, Supplementary File S2). Five of the 390 neonates (one vaginal- and four CS-born) had no reads mapping to Bacteroidetes. In the remaining 385 neonates, the Firmicutes-to-Bacteroidetes ratio was higher in neonates born by CS compared with neonates born vaginally (median 3,324.9, IQR: 1,184.3–14,109.8, vs. 0.6, IQR: 0.1–109.6, p-value <0.001). The relative abundance of Actinobacteria was lower in neonates born by CS (median 0.2, IQR: 0.6–1.7, vs. 4.0, IQR: 0.1–28.1; p-value <0.001, Figure S5, Supplementary File S2). Neonates born by CS had a higher relative abundance of the families Pasteurellaceae, Veillonellaceae, Streptococcaceae, Staphylococcaceae, and Enterococcaceae (p-values <0.01, Figure S6, Supplementary File S2), and the genera Haemophilus, Veillonella, Streptococcus, Staphylococcus, and Enterococcus (p-values <0.01, Figure S7, Supplementary File S2); neonates born vaginally had a higher abundance of Enterobacteriaceae, Tannerellaceae, Bacteroidaceae, and Bifidobacteriaceae (p-values <0.01, Figure S6, Supplementary File S2) and the genera Shigella, Escherichia, Citrobacter, Parabacteroides, Phocaeicola, Bacteroides, and Bifidobacterium (p-values <0.0001, Figure S7, Supplementary File S2). Differently abundant species between neonates born by CS and vaginally are detailed in Figure S8, Supplementary File S2.
Neonates who received formula milk during the first week of life had a higher abundance of Enterobacteriaceae, Veillonellaceae, Streptococcaceae, and Enterococcaceae and a lower abundance of Staphylococcaceae and Bacteroidaceae (p-values 0.001, 0.022, 0.095, 0.017, <0.001, and <0.001, respectively; Figure S6, Supplementary File S2). At the genera level, Klebsiella, Citrobacter, Veillonella, and Enterococcus were more abundant in neonates exposed to formula milk, while Staphylococcus, Parabacteroides, Phocaeicola, and Bacteroides were less abundant (p-values 0.001, <0.001, 0.012, 0.029, <0.001, <0.0001, <0.001, and <0.001, respectively; Figure S7, Supplementary File S2). Differently abundant species between neonates who did and did not receive formula milk in the first 7 days of life are detailed in Figure S8, Supplementary File S2.
Neonates of mothers who took probiotics during pregnancy had a lower abundance of Pasteurellaceae and Streptococcaceae, but a higher abundance of Bacteroidaceae (p-values 0.031, 0.014, and 0.035; Figure S6, Supplementary File S7). These neonates also had a lower abundance of Haemophilus and Streptococcus (p-values 0.045 and 0.011; Figure S7, Supplementary File S2) [Streptococcus vestibularis and Streptococcus thermophilus (p-values 0.032 and 0.028; Figure S8, Supplementary File S2)]. Neonates of mothers who received antibiotics during the last trimester of pregnancy had a higher abundance of Enterococcaceae (p-value 0.043) [Enterococcus faecalis (p-value 0.017, respectively; Figure S6 and Figure S8, Supplementary File S2)] and a higher abundance of Streptococcus parasanguinis (p-value 0.015, Figure S8, Supplementary File S2). Neonates of mothers who received intrapartum antibiotics had a lower abundance of Actinobacteria (p-value <0.0001; Figure S5, Supplementary File S2). These neonates had a higher abundance of Pasteurellaceae (Haemophilus) and a lower abundance of Bifidobacteriaceae (Bifidobacterium) (p-values 0.002, 0.001, <0.0001, and <0.0001, respectively; Figures S6, S7, Supplementary File S2). Furthermore, a higher abundance of Haemophilus parainfluenzae (p-values <0.001; Figure S8, Supplementary File S2) was found in neonates whose mothers received antibiotics during delivery.
Antibiotic resistance genes
Overall, 913 ARGs belonging to 27 classes were identified. The most abundant ARGs were tetQ, tetM, tetW, tetK (tetracycline), qacD, qacC, qacA (quaternary ammonium compound), sitABCD (peroxide), msrD, msrA (macrolide-streptogramin-B), ermF, ermB, ermC (macrolide-lincosamide-streptogramin-B), mdfA (macrolide-aminoglycoside-tetracycline-quinolone-amphenicol-rifamycin), mefA (macrolide), lsaA (lincosamide-streptogramin-A), fosB (fosfomycin), sul2 (folate pathway antagonist), blaZ; and mecA (beta-lactam).
The abundance of ARGs correlated with the bacterial species composition (Mantel test, statistic r 0.46, p-value 0.001). Spearman correlation analyses between the relative abundance of bacterial genera and species and ARGs can be found in Figures S9, S10, Supplementary File S2).
There was an association between gestational age, birth weight, mode of delivery, formula milk and the overall abundance of ARGs (PERMANOVA test, p-values <0.05; Table 2). There was no association between sex, ethnicity, probiotic use during pregnancy, antibiotic use during the last trimester of pregnancy, intrapartum antibiotic use, and the overall abundance of ARGs. A summary of the clinical factors associated with differences in the intestinal resistome can be found in Table 3.
The effect of delivery mode on the abundance of ARGs
In the PCoAs, resistome profiles of neonates born by CS and those born vaginally clustered apart (Figure 1). There was no difference in ARGs richness or diversity (Figure S11, Supplementary File S2) or overall abundance of ARGs (median of total normalised ARG counts, 531, IQR: 315–1,025 vs. 636, 413–1,011, p-value 0.28).
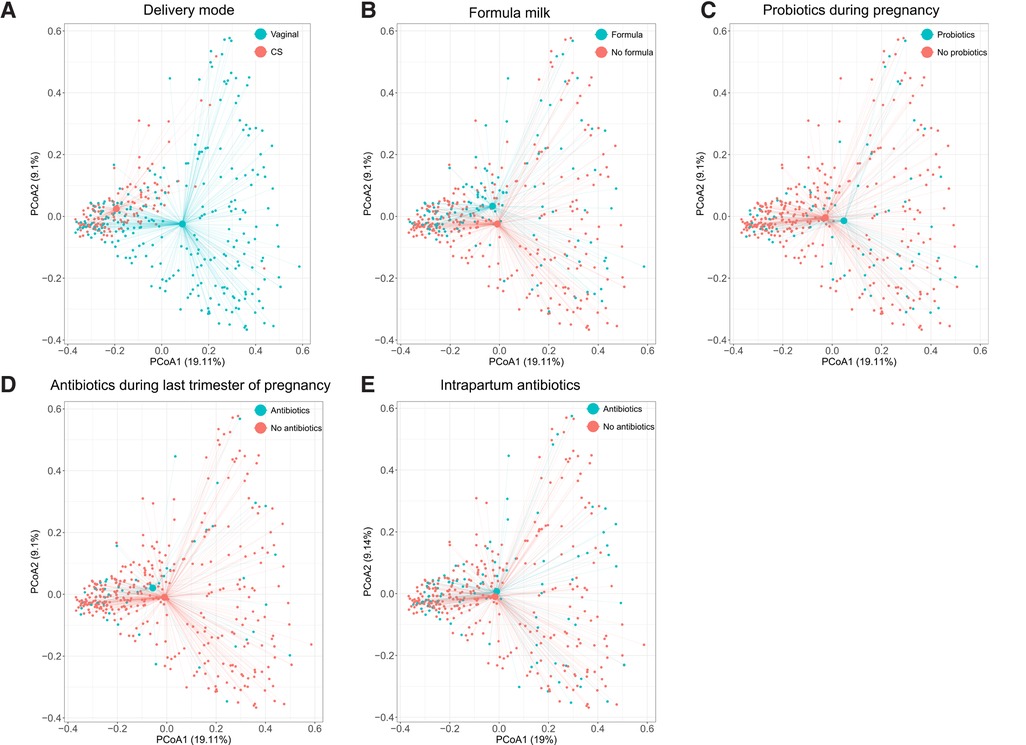
Figure 1. Principal coordinate analyses of resistome profiles done on the normalised read counts of 913 ARGs detected in 390 infants. Axes indicate the percentage of total variance. Colours indicate delivery mode (A), formula milk during the first 7 days of life (B), probiotics use during pregnancy (any trimester) (C), antibiotics use during pregnancy (last trimester) (D), and intrapartum antibiotic use (E). The centroids indicate average resistome composition. Lines connect centroids to corresponding data points. ARGs, antibiotic resistance genes; CS, Caesarean section.
In total, 119 ARGs were differentially abundant between the two groups (DESeq2, FDR-corrected p-value <0.05): msrD, qacA, lsaA, tetM, and mecA were more than three-fold more abundant in neonates born by CS; mdfA, cfxA5, cfxA, sitABCD, and tetQ were more than three-fold more abundant in neonates born vaginally (Figure 2).
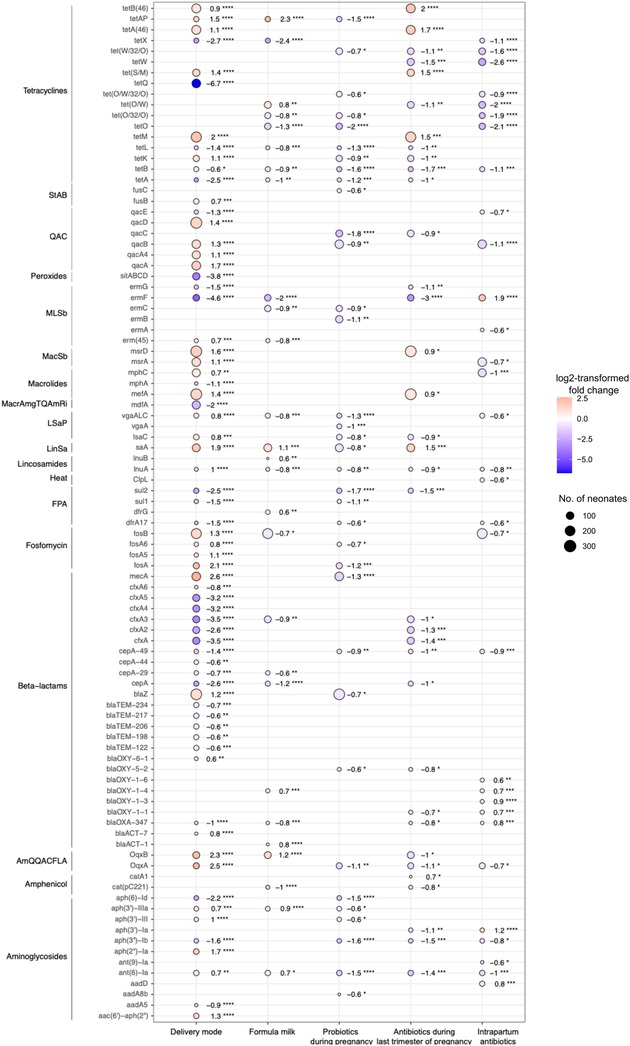
Figure 2. Differentially abundant antibiotic resistance genes. Dots depict genes associated with an FDR-corrected p-value of less than 0.05 and linear fold-change of at least 1.5 in the DESeq2 analyses. Colours of the dots represent the log2FC and size of the dots the number of neonates in whom ARGs were detected. Red colour and positive values of log2FC indicate the gene is more abundant in neonates born by Caesarean section, who received formula milk in the first 7 days of life, or whose mothers took probiotics (any trimester) or antibiotics during pregnancy (last trimester) or received intrapartum antibiotics. Blue colour and negative value of log2FC indicate that the gene is more abundant in neonates born vaginally, who were exclusively breastfed in the first 7 days of life or whose mothers did not take probiotics (any trimester) or antibiotics during pregnancy (last trimester) or did not receive intrapartum antibiotics. Wilcoxon rank sum test: *p < 0.05; **p < 0.01; ***p < 0.001; ****p < 0.0001. log2FC, log2-transformed fold-change; StAB, steroid antibacterial; QAC, quaternary ammonium compounds; MLSb, macrolide, lincosamide, streptogramin-B; MacSb, macrolide, streptogramin-B; MacrAmgTQAmRi, macrolide, aminoglycoside, tetracycline, quinolone, amphenicol, rifamycin; LSaP, lincosamide, streptogramin-A, pleuromutilin; LinSa, lincosamide, streptogramin-A; FPA, folate pathway antagonist; AmQQACFLA, amphenicol, quinolone, quaternary ammonium compounds, folate pathway antagonist.
The effect of formula milk on the abundance of ARGs
Resistome profiles clustered according to the feeding method in the first week of life (Figure 1). There was no difference in richness or diversity of ARGs (Figure S11, Supplementary File S2) or in the overall abundance of ARG between neonates who did or did not receive formula milk during the first week of life (median of total normalised ARG counts 665, IQR: 422–1,026, vs. 575, IQR: 346–1,009, p-value 0.3).
Forty-three ARGs were differentially abundant between the two groups (DESeq2, FDR-corrected p-value <0.05); two of them were detected in more than 100 neonates. LsaA (lincosamide-streptogramin-A) was more abundant in neonates who received formula milk, and fosB (fosfomycin) was more abundant in neonates who were exclusively breastfed (Figure 2).
The effect of probiotic use during pregnancy on the abundance of ARGs
Resistome profiles did not cluster according to probiotic use in pregnancy (Figure 1), and there was no difference in ARG richness and diversity between neonates of mothers who took probiotics during pregnancy and those who did not (Figure S11, Supplementary File S2) or in the overall abundance of ARGs (medians of total normalised ARG counts 609, IQR: 375–992, vs. 685, IQR: 325–1,030, respectively; p-value 0.7).
Thirty-five ARGs were differentially abundant between the two groups (DESeq2, FDR-corrected p-value <0.05). Five ARGs were detected in more than 100 neonates. BlaZ, mecA (beta-lactam), qacB (quaternary ammonium compounds), ermB (macrolide-lincosamide-streptogramin-B), and lsaA (lincosamide-streptogramin-A) were less abundant in neonates of mothers who took probiotics during pregnancy (Figure 2).
The effect of antibiotic use during the last trimester of pregnancy on the abundance of ARGs
Resistome profiles did not cluster according to antibiotic use in the last trimester of pregnancy (Figure 1). There was no difference in ARG richness and diversity between neonates of mothers who received antibiotics in the last trimester of pregnancy and these who did not (Figure S11, Supplementary File S2). However, the overall abundance of ARGs was higher in neonates whose mothers took antibiotics (median 833, IQR: 530–1,269 vs. 584, 361–934, p-value 0.018).
Thirty-six ARGs were differentially abundant between the two groups (DESeq2, FDR-corrected p-value <0.05); nine ARGs were present in more than 100 neonates. In neonates of mothers who did received antibiotics, msrD (macrolide-streptogramin-B), ermB (macrolide-lincosamide-streptogramin-B), mefA (macrolide), lsaA (lincosamide-streptogramin-A), and tet(S/M), tet(M), tetA(46), tetB(46) (tetracycline) were more abundant. In neonates whose mothers did not receive antibiotics cfxA (beta-lactam) and tetW (tetracycline) were more abundant (Figure 2).
The effect of intrapartum antibiotic use on the abundance of ARGs
Resistome profiles did not cluster according to intrapartum antibiotic use (Figure 1), and intrapartum antibiotic use did not influence ARGs’ richness and diversity (Figure S11, Supplementary File S2). There was also no difference in the overall number of ARGs between neonates of mothers who received intrapartum antibiotics and those who did not (medians of total normalised counts 642, IQR: 378–1,043, vs. IQR: 609, 370–984, p-value 0.7).
Thirty-seven ARGs were differentially abundant between neonates whose mothers did or did not receive intrapartum antibiotics (DESeq2, FDR-corrected p-value <0.05). Five of these ARGs were present in more than 100 neonates. The abundance of tetW (tetracycline), qacB, qacA4 (quaternary ammonium compounds), mphC (macrolide), msrA (macrolide-streptogramin-B), and fosB (fosfomycin) was higher in neonates whose mothers did not receive intrapartum antibiotics (Figure 2).
Discussion
In this study, the largest to date to use shotgun metagenomic sequencing to investigate the neonatal resistome, the neonatal intestine was found to harbour a high abundance and variety of ARGs, even in the absence of direct antibiotic exposure. Our findings are consistent with previous studies, which also found that the neonatal intestine harbours a high abundance of ARGs and that the most commonly found ARGs in neonates confer resistance to aminoglycosides, beta-lactams, macrolides, tetracyclines, or multidrug resistance (25). Similar to other studies, we found a strong association between the bacterial species composition of the intestinal microbiome and the composition of the resistome (20, 26–30). Previously, ARGs in neonates have been most frequently associated with the orders Bacteroidales and Enterobacteriales and the genera Bacteroides, Clostridioides, Escherichia, and Staphylococcus (26–30). An inverse correlation between the abundance of Bifidobacterium and the abundance of ARGs has been reported (26, 31). As in previous studies (32), in our cohort, neonates born by CS and neonates of mothers who received intrapartum antibiotics were found to have a significantly lower abundance of Bifidobacterium. Bifidobacteria are not only associated with a lower abundance of ARGs but also other health benefits, such as lower rates of allergic sensitisation, eczema, or asthma (33) and higher responses to vaccines (34).
A further factor that influenced the abundance and type of ARGs in the neonatal intestine was delivery mode. This is likely because delivery mode has a strong effect on the phylogenetic composition of the intestinal microbiome. Vertical transmission of ARGs from mothers to neonates occurs through contact, either during delivery with the genital tract or later through contact with the nasopharyngeal, oral, and skin microbiome (26, 35–37). Neonates born vaginally are, therefore, likely colonised by ARGs from the maternal vaginal and intestinal microbiome, whereas infants born by CS predominantly acquire ARGs from the maternal skin microbiome (4). As an example, in our cohort and in previous studies, a higher abundance of mecA was found in neonates born by CS (38, 39), which is likely attributable to colonisation by Streptococcus and Staphylococcus from the skin microbiome (4). Previous studies have reported a higher overall abundance of ARGs and a higher abundance of multidrug resistance-conferring genes bcr, mls_abc, mls_hdr, macAB, ykk, and mdr; the tetracycline resistance-conferring gene tet_efflux; the chloramphenicol resistance-conferring gene blt; and the vancomycin resistance-conferring gene vanA in the stool of neonates born by CS (39–41). A higher abundance of the beta-lactam resistance-conferring gene blaA, tetracycline resistance-conferring gene tet_RPP, and multidrug resistance-conferring genes mexEF and mexHI has been found in stool of vaginal-born neonates (41). Delivery mode has been reported to affect the composition of the intestinal microbiome for up to 6–12 months (42–46), for Clostridia spp. even up to seven years of age (47). Therefore, the association between delivery mode and differences in the composition of intestinal resistome might be clinically relevant, as the first year of life is the period with the highest risk for bacterial infection in children. However, longitudinal studies are needed to investigate how long these differences in the resistome persist. Some previous studies did not find an influence of delivery mode on the abundance of ARGs in neonatal stool, but this might have been because they were underpowered (29, 48–50). Furthermore, previous studies that used shotgun metagenomic sequencing to investigate the intestinal resistome in neonates often included neonates who were treated with antibiotics or for whom antibiotic exposure was not specified (26–27, 29, 35, 41, 48–54).
Consistent with our findings, it has previous been reported that breastfeeding influences the abundance of ARGs in the neonatal intestine (49). Also, similar to our findings, infant sex has previously been reported to have little effect on the abundance of ARGs in neonates (25). None of the previous studies investigated the effect of gestational age, birth weight, ethnicity, and probiotic use during pregnancy on the neonatal resistome. There have only been two previous studies that investigated whether maternal antibiotic use during pregnancy influences the neonatal intestinal resistome. One study did not find an association (54), and the other reported a higher abundance of blacepA and blacfxA in stool of neonates whose mothers were exposed to antibiotics (48). As in our study, it has been reported that intrapartum antibiotics does not affect the abundance of ARGs in neonatal stool (29, 49). However, some studies did find a higher abundance of ARGs in the neonatal intestinal microbiome (conferring resistance to beta-lactams, aminoglycosides, and fosfomycin) (26, 54, 55).
While the abundance of ARGs between neonates and their mothers was not compared in this study, previous studies have reported a higher abundance of ARGs in the intestine of neonates compared with their mothers (26, 35, 36, 39, 40). Again, this is likely explained by the bacterial phylogenetic composition. Neonates have a high abundance of Gammaproteobacteria, including Enterobacteriaceae, in their intestinal microbiome, which carry high numbers of ARGs (2, 26, 56–58).
The strengths of this study include the use of shotgun metagenomic sequencing that allowed simultaneous investigation of bacterial and resistome composition, the large sample size, and the absence of direct antibiotic exposure. A limitation of metagenomic sequencing is that it does not allow differentiation between genotypic and phenotypic resistance. It is, therefore, not possible to determine if the identified ARGs are expressed. Other limitations of this study include the absence of a longitudinal follow-up, the use of only one database for ARGs identification, the lack of detailed investigation of bacteriophages due to the absence of enrichment for viruses prior to extraction, and non-performance of RNA sequencing. Furthermore, although it has previously been reported that in neonates direct antibiotic use is associated with a higher abundance of ARGs and probiotic use with a lower abundance (25), this was not investigated in this study due to low numbers.
Conclusion
In this study, phylogenetic composition was strongly associated with the resistome composition. Other factors that were associated with the abundance of ARGs were delivery mode, gestational age, birth weight, feeding method, and antibiotics in the last trimester of pregnancy. Sex, ethnicity, probiotic use during pregnancy, and intrapartum antibiotics had little effect on the abundance of ARGs.
The neonatal intestine has a low colonisation resistance, which potentially makes it easier for antibiotic-resistant populations to establish themselves. In addition, neonates have a high abundance of Gammaproteobacteria, which are associated with high rates of horizontal gene transfer (59). Future studies will help identify the clinical relevance of the factors that are associated with differences in the intestinal resistome and in the development of evidence-based interventions to modulate the abundance of ARGs in neonates, for example, by the use of prebiotics, probiotics, and bacteriophages.
Data availability statement
Quality-filtered non-human read FASTQ files were deposited in the European Nucleotide Archive under the project PRJEB55714. Codes used for the pre-processing of sequencing reads (quality-filtering, removal of human DNA, etc.) are available on Github (https://github.com/miraclelab-unifr/Eczema).
Ethics statement
The studies involving human participants were reviewed and approved by the Royal Children's Hospital Human Research Ethics Committee. Written informed consent to participate in this study was provided by the participants’ legal guardian/next of kin.
Author contributions
PZ and NC conceptualised and designed the study that was led and supervised by PZ. NC is the chief principal investigator of the MIS BAIR trial. WJ performed the sequencing. LFP and NLM helped with sample handling. SL, OFC, PZ, and LF analysed the data. SL and PZ wrote the initial draft of the manuscript. All authors contributed to the article and approved the submitted version.
Funding
This work was supported by the Australian National Health and Medical Research Council (NHMRC) (grant no. GNT1051228 and GNT1099680) and the Royal Children’s Hospital Foundation (grant no. RCHF 2019-1140). PZ is supported by grants from the Swiss National Foundation (grant no. PZPGP3_193140) and the University of Fribourg and the Fribourg Hospital, Switzerland. NC is supported by a National Health and Medical Research Council (NHMRC) Investigator Grant (grant no. GNT1197117). None of the funders had a role in designing the study or in the study conduct.
Acknowledgements
We thank Susie Germano, Rhian Bonnici, Suzanna Vidmar, Tamblyn Thomason, and Samuel Forster for their help with data entry and management and/or the processing of samples, and Kaya Gardiner for project co-ordination.
Conflict of interest
The authors declare that the research was conducted in the absence of any commercial or financial relationships that could be construed as a potential conflict of interest.
Publisher's note
All claims expressed in this article are solely those of the authors and do not necessarily represent those of their affiliated organizations, or those of the publisher, the editors and the reviewers. Any product that may be evaluated in this article, or claim that may be made by its manufacturer, is not guaranteed or endorsed by the publisher.
Supplementary material
The Supplementary Material for this article can be found online at: https://www.frontiersin.org/articles/10.3389/fped.2023.1169651/full#supplementary-material
Supplementary File 1
R code
Supplementary File 2
Supplementary figures and tables
Supplementary File 3
Distribution of log2-transformed normalised reads of ARGs
Supplementary Table 1
Excel table containing results of DESeq2 analyses
Supplementary Table 2
Excel table containing results of Wilcoxon tests on phyla
Supplementary Table 3
Excel table containing results of Wilcoxon tests on families
Supplementary Table 4
Excel table containing results of Wilcoxon tests on genera
Supplementary Table 5
Excel table containing results of Wilcoxon tests on species
Supplementary Table 6
Bacteria and viruses identified with Bracken v2.6.2
Supplementary Table 7
ARGs identified with ResFinder gene database v4
Supplementary Table 8
Metadata
References
1. Salyers AA, Gupta A, Wang Y. Human intestinal bacteria as reservoirs for antibiotic resistance genes. Trends Microbiol. (2004) 12(9):412–6. doi: 10.1016/j.tim.2004.07.004
2. Hu Y, Yang X, Qin J, Lu N, Cheng G, Wu N, et al. Metagenome-wide analysis of antibiotic resistance genes in a large cohort of human gut microbiota. Nat Commun. (2013) 4:2151. doi: 10.1038/ncomms3151
3. Penders J, Stobberingh EE, Savelkoul PH, Wolffs PF. The human microbiome as a reservoir of antimicrobial resistance. Front Microbiol. (2013) 4:87. doi: 10.3389/fmicb.2013.00087
4. Zimmermann P, Curtis N. Factors influencing the intestinal microbiome during the first year of life. Pediatr Infect Dis J. (2018) 37(12):e315–35. doi: 10.1097/INF.0000000000002103
5. Diallo OO, Baron SA, Abat C, Colson P, Chaudet H, Rolain JM. Antibiotic resistance surveillance systems: a review. J Glob Antimicrob Resist. (2020) 23:430–8. doi: 10.1016/j.jgar.2020.10.009
6. Duranti S, Lugli GA, Mancabelli L, Turroni F, Milani C, Mangifesta M, et al. Prevalence of antibiotic resistance genes among human gut-derived bifidobacteria. Appl Environ Microbiol. (2017) 83(3):e02894-16. doi: 10.1128/AEM.02894-16
7. Logan LK. Carbapenem-resistant enterobacteriaceae: an emerging problem in children. Clin Infect Dis. (2012) 55(6):852–9. doi: 10.1093/cid/cis543
8. Zimmermann P, Curtis N. The effect of antibiotics on the composition of the intestinal microbiota—a systematic review. J Infect. (2019) 79(6):471–89. doi: 10.1016/j.jinf.2019.10.008
9. Lebeaux RM, Karalis DB, Lee J, Whitehouse HC, Madan JC, Karagas MR, et al. The association between early life antibiotic exposure and the gut resistome of young children: a systematic review. Gut Microbes. (2022) 14(1):2120743. doi: 10.1080/19490976.2022.2120743
10. Leo S, Curtis N, Zimmermann P. The neonatal intestinal resistome and factors that influence it—a systematic review. Clin Microbiol Infect. (2022) 28(12):1539–46. doi: 10.1016/j.cmi.2022.07.014
11. Laxminarayan R, Matsoso P, Pant S, Brower C, Røttingen JA, Klugman K, et al. Access to effective antimicrobials: a worldwide challenge. Lancet. (2016) 387(10014):168–75. doi: 10.1016/S0140-6736(15)00474-2
12. Messina NL, Gardiner K, Donath S, Flanagan K, Ponsonby AL, Shann F, et al. Study protocol for the Melbourne infant study: BCG for allergy and infection reduction (MIS BAIR), a randomised controlled trial to determine the non-specific effects of neonatal BCG vaccination in a low-mortality setting. BMJ Open. (2019) 9(12):e032844. doi: 10.1136/bmjopen-2019-032844
13. Bolger AM, Lohse M, Usadel B. Trimmomatic: a flexible trimmer for Illumina sequence data. Bioinformatics. (2014) 30(15):2114–20. doi: 10.1093/bioinformatics/btu170
14. Wood DE, Lu J, Langmead B. Improved metagenomic analysis with Kraken 2. Genome Biol. (2019) 20(1):257. doi: 10.1186/s13059-019-1891-0
15. Lu J, Salzberg SL. Ultrafast and accurate 16S rRNA microbial community analysis using Kraken 2. Microbiome. (2020) 8(1):124. doi: 10.1186/s40168-020-00900-2
16. Shkoporov AN, Clooney AG, Sutton TDS, Ryan FJ, Daly KM, Nolan JA, et al. The human gut virome is highly diverse, stable, and individual specific. Cell Host Microbe. (2019) 26(4):527–41.e5. doi: 10.1016/j.chom.2019.09.009
17. Hoyles L, McCartney AL, Neve H, Gibson GR, Sanderson JD, Heller KJ, et al. Characterization of virus-like particles associated with the human faecal and caecal microbiota. Res Microbiol. (2014) 165(10):803–12. doi: 10.1016/j.resmic.2014.10.006
18. Zankari E, Hasman H, Cosentino S, Vestergaard M, Rasmussen S, Lund O, et al. Identification of acquired antimicrobial resistance genes. J Antimicrob Chemother. (2012) 67(11):2640–4. doi: 10.1093/jac/dks261
19. Langmead B, Salzberg SL. Fast gapped-read alignment with bowtie 2. Nat Methods. (2012) 9(4):357–9. doi: 10.1038/nmeth.1923
20. Pärnänen K, Karkman A, Hultman J, Lyra C, Bengtsson-Palme J, Larsson DGJ, et al. Maternal gut and breast milk microbiota affect infant gut antibiotic resistome and mobile genetic elements. Nat Commun. (2018) 9(1):3891. doi: 10.1038/s41467-018-06393-w
21. Li H, Handsaker B, Wysoker A, Fennell T, Ruan J, Homer N, et al. The sequence alignment/map format and SAMtools. Bioinformatics. (2009) 25(16):2078–9. doi: 10.1093/bioinformatics/btp352
22. Quinlan AR, Hall IM. BEDTools: a flexible suite of utilities for comparing genomic features. Bioinformatics. (2010) 26(6):841–2. doi: 10.1093/bioinformatics/btq033
23. Love MI, Huber W, Anders S. Moderated estimation of fold change and dispersion for RNA-seq data with DESeq2. Genome Biol. (2014) 15(12):550. doi: 10.1186/s13059-014-0550-8
24. Anderson MJ. A new method for non-parametric multivariate analysis of variance. Austral Ecol. (2001) 26(1):32–46. 10.1111j.1442-9993.2001.01070.pp.x
25. Leo S, Curtis N, Zimmermann P. The neonatal intestinal resistome and factors that influence it—a systematic review. Clin Microbiol Infect. (2022) 28(12):1539–46. doi: 10.1016/j.cmi.2022.07.014
26. Guernier-Cambert V, Chamings A, Collier F, Alexandersen S. Diverse bacterial resistance genes detected in fecal samples from clinically healthy women and infants in Australia—a descriptive pilot study. Front Microbiol. (2021) 12:596984. doi: 10.3389/fmicb.2021.596984
27. Tapiainen T, Koivusaari P, Brinkac L, Lorenzi HA, Salo J, Renko M, et al. Impact of intrapartum and postnatal antibiotics on the gut microbiome and emergence of antimicrobial resistance in infants. Sci Rep. (2019) 9(1):10635. doi: 10.1038/s41598-019-46964-5
28. Li W, Tapiainen T, Brinkac L, Lorenzi HA, Moncera K, Tejesvi M, et al. Vertical transmission of gut microbiome and antimicrobial resistance genes in infants exposed to antibiotics at birth. J Infect Dis. (2020) 224(7):1236–46. doi: 10.1093/infdis/jiaa155
29. Casaburi G, Duar RM, Vance DP, Mitchell R, Contreras L, Frese SA, et al. Early-life gut microbiome modulation reduces the abundance of antibiotic-resistant bacteria. Antimicrob Resist Infect Control. (2019) 8:131. doi: 10.1186/s13756-019-0583-6
30. Zhang L, Kinkelaar D, Huang Y, Li Y, Li X, Wang HH. Acquired antibiotic resistance: are we born with it? Appl Environ Microbiol. (2011) 77(20):7134–41. doi: 10.1128/AEM.05087-11
31. Taft DH, Liu J, Maldonado-Gomez MX, Akre S, Huda MN, Ahmad SM, et al. Bifidobacterial dominance of the gut in early life and acquisition of antimicrobial resistance. mSphere. (2018) 3(5):e00441–18. doi: 10.1128/mSphere.00441-18
32. Zimmermann P, Curtis N. Effect of intrapartum antibiotics on the intestinal microbiota of infants: a systematic review. Arch Dis Child Fetal Neonatal Ed. (2020) 105(2):201–8. doi: 10.1136/archdischild-2018-316659
33. Zimmermann P, et al. Association between the intestinal microbiota and allergic sensitization, eczema, and asthma: a systematic review. J Allergy Clin Immunol. (2019) 143(2):467–85. doi: 10.1016/j.jaci.2018.09.025
34. Zimmermann P, Curtis N. The influence of the intestinal microbiome on vaccine responses. Vaccine. (2018) 36(30):4433–9. doi: 10.1016/j.vaccine.2018.04.066
35. Yassour M, et al. Strain-level analysis of mother-to-child bacterial transmission during the first few months of life. Cell Host Microbe. (2018) 24(1):146–54.e4. doi: 10.1016/j.chom.2018.06.007
36. Ravi A, Avershina E, Foley SL, Ludvigsen J, Storrø O, Øien T, et al. The commensal infant gut meta-mobilome as a potential reservoir for persistent multidrug resistance integrons. Sci Rep. (2015) 5:15317. doi: 10.1038/srep15317
37. Zhang K, Jin M, Yang D, Shen Z, Liu W, Yin J, et al. Antibiotic resistance genes in gut of breast-fed neonates born by caesarean section originate from breast milk and hospital ward air. BMC Microbiol. (2022) 22(1):36. doi: 10.1186/s12866-022-02447-8
38. Jiménez E, Delgado S, Maldonado A, Arroyo R, Albújar M, García N, et al. Staphylococcus epidermidis: a differential trait of the fecal microbiota of breast-fed infants. BMC Microbiol. (2008) 8(1):143. doi: 10.1186/1471-2180-8-143
39. Gosalbes MJ, Vallès Y, Jiménez-Hernández N, Balle C, Riva P, Miravet-Verde S, et al. High frequencies of antibiotic resistance genes in infants’ meconium and early fecal samples. J Dev Orig Health Dis. (2016) 7(1):35–44. doi: 10.1017/S2040174415001506
40. Bäckhed F, Roswall J, Peng Y, Feng Q, Jia H, Kovatcheva-Datchary P, et al. Dynamics and stabilization of the human gut microbiome during the first year of life. Cell Host Microbe. (2015) 17(6):852. doi: 10.1016/j.chom.2015.05.012
41. Shi YC, Guo H, Chen J, Sun G, Ren RR, Guo MZ, et al. Initial meconium microbiome in Chinese neonates delivered naturally or by cesarean section. Sci Rep. (2018) 8(1):3255. doi: 10.1038/s41598-018-21657-7
42. Adlerberth I, Strachan DP, Matricardi PM, Ahrne S, Orfei L, Aberg N, et al. Gut microbiota and development of atopic eczema in 3 European birth cohorts. J Allergy Clin Immunol. (2007) 120(2):343–50. doi: 10.1016/j.jaci.2007.05.018
43. Jakobsson HE, Abrahamsson TR, Jenmalm MC, Harris K, Quince C, Jernberg C, et al. Decreased gut microbiota diversity, delayed bacteroidetes colonisation and reduced Th1 responses in infants delivered by caesarean section. Gut. (2014) 63(4):559–66. doi: 10.1136/gutjnl-2012-303249
44. Gronlund MM, Lehtonen OP, Eerola E, Kero P Fecal microflora in healthy infants born by different methods of delivery: permanent changes in intestinal flora after cesarean delivery. J Pediatr Gastroenterol Nutr. (1999) 28(1):19–25. doi: 10.1097/00005176-199901000-00007
45. Wampach L, Heintz-Buschart A, Hogan A, Muller EEL, Narayanasamy S, Laczny CC, et al. Colonization and succession within the human gut microbiome by archaea, bacteria, and microeukaryotes during the first year of life. Front Microbiol. (2017) 8:738. doi: 10.3389/fmicb.2017.00738
46. Martin R, Makino H, Cetinyurek Yavuz A, Ben-Amor K, Roelofs M, Ishikawa E, et al. Early-life events, including mode of delivery and type of feeding, siblings and gender, shape the developing gut microbiota. PLoS One. (2016) 11(6):e0158498. doi: 10.1371/journal.pone.0158498
47. Salminen S, Gibson GR, McCartney AL, Isolauri E. Influence of mode of delivery on gut microbiota composition in seven year old children. Gut. (2004) 53(9):1388–9. doi: 10.1136/gut.2004.041640
48. Loo EXL, Zain A, Yap GC, Purbojati RW, Drautz-Moses DI, Koh YQ, et al. Longitudinal assessment of antibiotic resistance gene profiles in gut microbiomes of infants at risk of eczema. BMC Infect Dis. (2020) 20(1):312. doi: 10.1186/s12879-020-05000-y
49. Rahman SF, Olm MR, Morowitz MJ, Banfield JF. Machine learning leveraging genomes from metagenomes identifies influential antibiotic resistance genes in the infant gut microbiome. mSystems. (2018) 3(1):e00123-17. doi: 10.1128/mSystems.00123-17
50. Dierikx T, Berkhout D, Eck A, Tims S, van Limbergen J, Visser D, et al. Influence of timing of maternal antibiotic administration during caesarean section on infant microbial colonisation: a randomised controlled trial. Gut. (2021) 71(9):1803–11. doi: 10.1136/gutjnl-2021-324767
51. Reyman M, van Houten MA, Watson RL, Chu M, Arp K, de Waal WJ, et al. Effects of early-life antibiotics on the developing infant gut microbiome and resistome: a randomized trial. Nat Commun. (2022) 13(1):893. doi: 10.1038/s41467-022-28525-z
52. Esaiassen E, Hjerde E, Cavanagh JP, Pedersen T, Andresen JH, Rettedal SI, et al. Effects of probiotic supplementation on the gut Microbiota and antibiotic resistome development in preterm infants. Front Pediatr. (2018) 6:347. doi: 10.3389/fped.2018.00347
53. Hourigan SK, Subramanian P, Hasan NA, Ta A, Klein E, Chettout N, et al. Comparison of infant gut and skin microbiota, resistome and virulome between neonatal intensive care unit (NICU) environments. Front Microbiol. (2018) 9:1361. doi: 10.3389/fmicb.2018.01361
54. Ravi A, Avershina E, Angell IL, Ludvigsen J, Manohar P, Padmanaban S, et al. Comparison of reduced metagenome and 16S rRNA gene sequencing for determination of genetic diversity and mother-child overlap of the gut associated microbiota. J Microbiol Methods. (2018) 149:44–52. doi: 10.1016/j.mimet.2018.02.016
55. Nogacka A, Salazar N, Suárez M, Milani C, Arboleya S, Solís G, et al. Impact of intrapartum antimicrobial prophylaxis upon the intestinal microbiota and the prevalence of antibiotic resistance genes in vaginally delivered full-term neonates. Microbiome. (2017) 5(1):93. doi: 10.1186/s40168-017-0313-3
56. Bäckhed F, Roswall J, Peng Y, Feng Q, Jia H, Kovatcheva-Datchary P, et al. Dynamics and stabilization of the human gut microbiome during the first year of life. Cell Host Microbe. (2015) 17(5):690–703. doi: 10.1016/j.chom.2015.04.004
57. Moore AM, Patel S, Forsberg KJ, Wang B, Bentley G, Razia Y, et al. Pediatric fecal microbiota harbor diverse and novel antibiotic resistance genes. PLoS One. (2013) 8(11):e78822. doi: 10.1371/journal.pone.0078822
58. Li J, Jia H, Cai X, Zhong H, Feng Q, Sunagawa S, et al. An integrated catalog of reference genes in the human gut microbiome. Nat Biotechnol. (2014) 32(8):834–41. doi: 10.1038/nbt.2942
Keywords: antibiotic resistance, mobilome, stool, microbiome, microbiota, gut, infant
Citation: Leo S, Cetiner OF, Pittet LF, Messina NL, Jakob W, Falquet L, Curtis N and Zimmermann P (2023) Metagenomics analysis of the neonatal intestinal resistome. Front. Pediatr. 11:1169651. doi: 10.3389/fped.2023.1169651
Received: 19 February 2023; Accepted: 25 April 2023;
Published: 16 June 2023.
Edited by:
Marcus de Goffau, University of Cambridge, United KingdomReviewed by:
Lu-Quan Li, Chongqing Medical University, ChinaChristian R. Kahlert, Children's Hospital of Eastern Switzerland, Switzerland
© 2023 Leo, Cetiner, Pittet, Messina, Jakob, Falquet, Curtis and Zimmermann. This is an open-access article distributed under the terms of the Creative Commons Attribution License (CC BY). The use, distribution or reproduction in other forums is permitted, provided the original author(s) and the copyright owner(s) are credited and that the original publication in this journal is cited, in accordance with accepted academic practice. No use, distribution or reproduction is permitted which does not comply with these terms.
*Correspondence: Petra Zimmermann cGV0cmEuemltbWVybWFubkB1bmlmci5jaA==