- 1Department of Gastroenterology, Children’s Hospital of Nanjing Medical University, Nanjing, China
- 2Medical School, Nanjing University, Nanjing, China
- 3School of Electronic Science and Engineering, Nanjing University, Nanjing, China
- 4College of Medicine, National Taiwan University, Taipei, Taiwan
- 5Department of Quality Management, Children's Hospital of Nanjing Medical University, Nanjing, China
Background: Children with intractable functional constipation (IFC) who are refractory to traditional pharmacological intervention develop severe symptoms that can persist even in adulthood, resulting in a substantial deterioration in their quality of life. In order to better manage IFC patients, efficient subtyping of IFC into its three subtypes, normal transit constipation (NTC), outlet obstruction constipation (OOC), and slow transit constipation (STC), at early stages is crucial. With advancements in technology, machine learning can classify IFC early through the use of validated questionnaires and the different serum concentrations of gastrointestinal motility-related hormones.
Method: A hundred and one children with IFC and 50 controls were enrolled in this study. Three supervised machine-learning methods, support vector machine, random forest, and light gradient boosting machine (LGBM), were used to classify children with IFC into the three subtypes based on their symptom severity, self-efficacy, and quality of life which were quantified using certified questionnaires and their serum concentrations of the gastrointestinal hormones evaluated with enzyme-linked immunosorbent assay. The accuracy of machine learning subtyping was evaluated with respect to radiopaque markers.
Results: Of 101 IFC patients, 37 had NTC, 49 had OOC, and 15 had STC. The variables significant for IFC subtype classification, according to SelectKBest, were stool frequency, the satisfaction domain of the Patient Assessment of Constipation Quality of Life questionnaire (PAC-QOL), the emotional self-efficacy for Functional Constipation questionnaire (SEFCQ), motilin serum concentration, and vasoactive intestinal peptide serum concentration. Among the three models, the LGBM model demonstrated an accuracy of 83.8%, a precision of 84.5%, a recall of 83.6%, a f1-score of 83.4%, and an area under the receiver operating characteristic curve (AUROC) of 0.89 in discriminating IFC subtypes.
Conclusion: Using clinical characteristics measured by certified questionnaires and serum concentrations of the gastrointestinal hormones, machine learning can efficiently classify pediatric IFC into its three subtypes. Of the three models tested, the LGBM model is the most accurate model for the classification of IFC, with an accuracy of 83.8%, demonstrating that machine learning is an efficient tool for the management of IFC in children.
Introduction
Functional constipation (FC) is estimated to affect 7.5% to 12.1% of children worldwide (1). The diagnosis of FC is based on symptoms and is defined according to the Rome IV diagnostic criteria (2, 3). Although the majority of children with FC respond well to conventional treatments, up to one-third of children with IFC respond less favorably (4). Intractable functional constipation (IFC) is defined as the inability of children to pass stool even with the maximum laxative treatment, daily rectal stimulation, behavioral therapy, or toilet training program for more than 3 months (4, 5).
IFC has a negative impact on the quality of life, affecting children and their families; they have poorer scores in physical, emotional, social, and school functioning domains compared to those of healthy populations (6). Children with constipation often present with symptoms like infrequent bowel movements, bloating, abdominal pain, and fecal incontinence (7). If not properly treated, a fourth of these children will continue to experience symptoms as adults (8). Thus, tools for accurately determining IFC subtype are highly essential for more effective management.
Colonic transit time (CTT), which is how long it takes for stool to pass through the colon, is used to classify IFC into three subtypes: normal transit constipation (NTC), outlet obstruction constipation (OOC), and slow transit constipation (STC) (9). The CTT of NTC patients is the same as the CTT for healthy individuals and NTC patients are able to expel stool without problems like healthy individuals do but they still report symptoms of constipation like bloating, abdominal pain, and hard stool (10, 11). OOC patients have a slightly longer than normal CTT due to impaired rectal contraction, paradoxical anal contraction, or inadequate anal relaxation; they are unable to expel stool after it reaches the rectum (12). STC patients have impaired colonic motility and contractility resulting in a prolonged CTT (13).
Because different subtypes have different managements it is important to determine the subtype as early as possible (14–16). For NTC patients, dietary fiber, osmotic laxatives, or prosecretory agents have limited effects. They should instead be referred for psychiatric consultations (4). OOC patients are less likely to respond to laxative therapy and rather need biofeedback therapy, which helps to train pelvic floor muscles involved in defecation (17). STC patients are treated with stimulants or colonic prokinetics and oftentimes require surgical management such as antegrade continence enemas, transcutaneous electrical stimulation, or colectomy (18).
Different pathophysiologic mechanisms have been proposed for different IFC subtypes (19). Numerous studies have demonstrated a substantial relationship between constipation and gastrointestinal hormones which are secreted by enteroendocrine cells dispersed throughout the gastrointestinal tract and act as crucial signaling molecules (20, 21). Some gastrointestinal hormones have been shown to affect colonic motility, including motilin (MTL), vasoactive intestinal peptide (VIP), ghrelin (GHRL), cholecystokinin (CCK) and glucagon-like peptide (GLP-1). MTL is widely distributed in the brain and gut and is known to stimulate gastrointestinal motility and regulate phase III of the migrating motor complex (MMC) (22, 23). VIP is an important inhibitory neurotransmitter in the enteric nervous system that relaxes the smooth muscle of the gastrointestinal tract (24). GHRL can regulate gut motility, adjust intestinal smooth muscle contraction, increase food intake, and secrete gastric acid (25). The motor effects of CCK include postprandial inhibition of gastric emptying and inhibition of colonic transit (26). The physiological role of GLP-1 is to balance energy, enhance glucose-stimulated insulin secretion, and reduce gastric emptying and gastrointestinal motility (27). In an adult study, the gastrointestinal hormone profiles were indicated as a reliable tool to classify IFC into the 3 subtypes; however there has not been a similar study conducted in children (28).
CTT evaluation using the radiopaque markers (ROM) test for subtyping IFC in children (29). This test requires children to swallow radiopaque marker capsules and takes at least 3 days to obtain and interpret the results (9). It is not only time-consuming but also exposes children to high doses of radiation (30). It can also be difficult for younger children to cooperate and swallow the ROMs. Alternative evaluation methods should be explored. Machine learning (ML) has been applied to a wide range of uses in healthcare such as solving diagnostic and prognostic problems (31). ML can identify complex patterns in multiple diverse data sources and increase prediction accuracy by using these uncovered patterns to classify disease phenotypes (32). However, few studies have applied ML to constipation (33, 34) and no study has used ML to analyze clinical and laboratory data to subtype IFC in children.
The aims of the present study are to (1) investigate the differences in clinical symptoms, quality of life, and self-efficacy through the use of constipation questionnaires in children with different subtypes of IFC; (2) evaluate their MTL, GHRL, VIP, CCK, and GLP-1 serum concentrations; (3) use a supervised machine learning model to subtype IFC in children.
Materials and methods
Study design
This was a single-center, cross-sectional study conducted at Children's Hospital of Nanjing Medical University (Nanjing, Jiangsu, China). The participants were recruited from the outpatient clinic of the Department of Gastroenterology. The study was conducted between December 2021 and July 2022. The researchers acquired the approval of the institutional review board (202110090-1) from the hospital where the first author worked and the site where participants were recruited.
Participants
Participants were eligible for the study if they were between the ages of 4–14, had IFC (fulfilling the Rome IV criteria for FC), and responded poorly to 3 or more months of conventional therapy. Children with congenital abnormalities (i.e., cystic fibrosis, Hirschsprung disease, neuronal intestinal dysplasia, and anorectal malformations), anismus, constipation due to irritable bowel syndrome, inflammatory bowel disease, endocrine and metabolic diseases, diseases of myopathy and enteric nervous system, or a history of gastrointestinal surgery were excluded from the study. Patients taking any drug affecting gastrointestinal motility were excluded from the study (Figure 1).
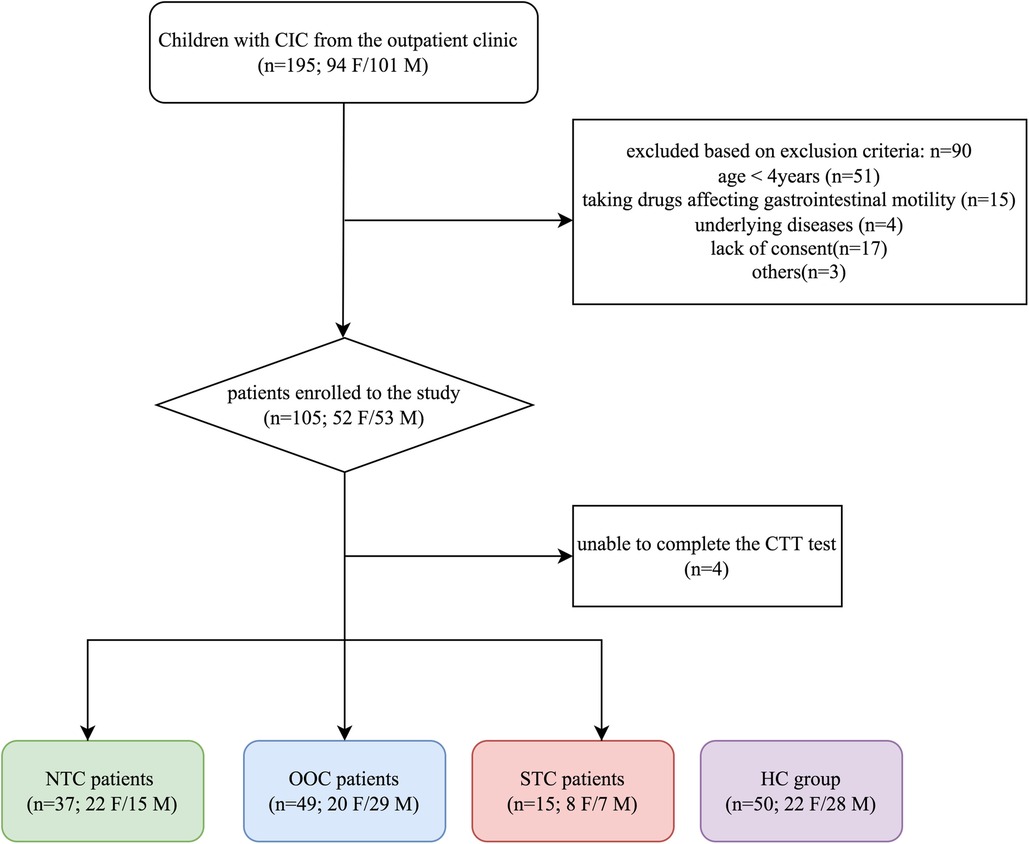
Figure 1. Flow chart of participants throughout the study. IFC, chronic intractable constipation; NTC, normal transit constipation; OOC, outlet obstruction constipation; STC, slow transit constipation; HC, healthy controls; F, female; M, male.
Data collection
During the preliminary visit, participants and their legal guardians were asked about the participant's medical history and were asked to complete the Chinese version of the Patient Assessment of Constipation Symptoms (PAC-SYM) (35), the Self-efficacy for FC questionnaire (SEFCQ) (36), and the Patient Assessment of Constipation Quality of Life (PAC-QOL) (37) for quantification of the participant's clinical symptoms and quality of life. Impartial assistance was provided to anyone who had difficulty understanding. Participants were given a physical examination which included an abdominal examination, an inspection of the perianal region, and an examination of the lumbosacral region. Venous blood samples were obtained for biochemical analysis.
During the second visit (participants were instructed to refrain from using laxatives or enemas 72 h prior to), the participants' CTT's were evaluated using radiopaque markers. The four children who failed to complete the CTT test were excluded from this study.
Children in the healthy control (HC) group were also given physical examinations and were also evaluated with the questionnaires of PAC-SYM, SEFCQ, and PAC-QOL. They were not evaluated for CTT because children without IFC lacked sufficient indications for this test.
Patient assessment of constipation symptoms (PAC-SYM)
PAC-SYM is designed to assess the patient's experience of constipation in the last 2 weeks by quantifying symptoms and symptom severity. It is a self-reported questionnaire consisting of 12 symptoms divided into three domains: abdominal, rectal, and stool; the responses are rated on a 5-point Likert scale. The overall score is a sum ranging from 0 to 48, with higher values indicating more severe symptoms.
Self-efficacy for FC questionnaire (SEFCQ)
SEFCQ is a disease-specific tool for evaluating self-efficacy in children with constipation and has been proven to be a good predictor of self-efficacy in terms of toilet training and treatment outcomes. The Chinese version of the SEFCQ includes 14 items that measure action and emotional self-efficacy. Participants rate each item on a Likert scale of 1 (never) to 4 (always). The total score is the sum of all the items and ranges from 14 to 56, with higher scores indicating greater self-efficacy.
Patient assessment of constipation quality of life (PAC-QOL)
PAC-QOL is a questionnaire that specifically assesses the quality of life of patients diagnosed with constipation. It contains 28 items divided into 4 domains: 4 items in physical discomfort, 8 items in psychosocial discomfort, 11 items in worries and discomfort, and 5 items in treatment satisfaction. The subscale is a Likert scale ranging from 0 (absent) to 4 (very severe). Each domain's score is the sum of the items in that domain with higher scores meaning greater negative effects on quality of life.
Radiopaque marker (ROM) test
In this study, the definitions of NTC, OOC, and STC are based on CTT. The ROM test is widely accessible, non-invasive, inexpensive, and the most common option for assessing CTT (38, 39). In a ROM test, the patient ingests a dissolving capsule containing 24 radiopaque markers and an abdominal radiographic image is taken after 72 h (40). The radiopaque markers are visible on the x-ray and are quantified based on the number and location of the markers remaining in the colon (14). If the number of retained markers is less than 5, indicating a CTT of <72 h, the patient would be classified as NTC. If the number of retained markers is more than 5, indicating a CTT value of >72 h, the patient would be classified as having abnormal transit constipation. Children with abnormal constipation can then be divided into two groups: the OOC group (>50% of the markers retained mainly in the rectosigmoid area and left colon area) and the STC group (retained markers are distributed throughout the whole colon area) (41).
Serum concentration of gastrointestinal motility-related hormones
Venous blood samples were collected after overnight fasting into standard vacuum tubes. Serum samples for hormone concentrations analysis were stored at −80°C. Concentration quantification of serum MTL, GHRL, VIP, CCK, and GLP-1 were performed using an enzyme-linked immunosorbent assay kit by Cusabio.
Statistical analysis
The children in the IFC group and the control group were matched according to age and gender. The Kolmogorov-Smirnov test was used and the data was distributed non-normally (the data was presented with a median and a 25th-75th interquartile range). The chi-square test was used to compare the gender distributions. Mann-Whitney U and Kruskal-Wallis H tests were used for comparing nonnormally distributed variables among the control and IFC subtype groups. For the analysis of the dataset, Spearman correlation analysis was used with the results presented as a heatmap. A univariate analysis was used to identify fifteen variables that contributed to the three IFC subtypes (each with p-values <0.05) and would be suitable for the establishment of ML models.
The significance level for the p-value was set at 0.05. The statistical analyses were carried out using the Statistical Package for Social Science (SPSS 24.0, IBM Corporation, Chicago, IL) and the GraphPad software (Prism 8.0, GraphPad Prism Software Inc., San Diego, CA).
Machine-learning modeling
The resulting dataset, including clinical features and gastrointestinal motility-related hormones, was analyzed with the Python programming language (version 3.9.12), utilizing the Scikit-Learn library (version 1.1.0). For the development of a machine learning model, the children were randomly divided into a training set and a validation set with a 7:3 ratio. The training set was used to develop the ML models, which were then validated with the validation set. Three machine learning methods are utilized to develop prediction models: support vector machine (SVM), random forest (RF), and light gradient boosting machine (LGBM), with 5-fold cross validation for performance evaluation. K-fold cross validation was chosen for its ease of application in selecting the most appropriate model for a given predictive modeling problem and providing skill estimates with low bias. SelectKBest was used to determine the characteristic features. A grid search of the different parameters of each model was performed to determine the best parameters of each model and improve its performance. Accuracy, precision, recall, and f1-score were used as performance indicators. The results were depicted by a confusion matrix.
Results
Participant demographics
There were 105 participants enrolled in the study and 101 of the participants between the ages of 5–9 years old (median age of 6) completed the ROM test and questionnaires. Of 101 IFC patients, 37 were NTC [15 males, between the ages of 5–10 years old (median age of 7)], 49 were OOC [29 males, between the ages of 5–7 years old (median age of 5)] and 15 were STC [7 males, between the ages of 5–11 years old (median age of 6)]. There were 50 participants in the control group [28 males, between the ages of 5–9 years old (median age of 7)]. The BMI of the control group, NTC group, OOC group, and STC group were 16.41 (15.10–18.41), 15.63 (14.61–16.59), 15.88 (14.82–17.00), and 16.53 (13.78–17.60), respectively. The Z-score of the control group, NTC group, OOC group, and STC group were 0.045 (−0.415–1.1), 0.03 (−0.5–0.47), −0.12 (−0.785–1.39), and −0.23 (−2.37–0.69) respectively. The BMI of the control group was generally within the normal BMI range (z-score: −1.0 to +1.0), leaning towards slightly overweight. The time taken per defecation attempt for the control group, NTC, OOC, and STC groups was 10 min (10–15), 10 min (10–20), 15 min (10–25), and 10 min (10–15) respectively. The stool frequency per week were 5 times/week (4–6), 2 times/week (1–2), 2 times/week (1–2) and 1 time/week (1–2), respectively. The Bristol stool consistency were type 4 (3– 4), type 2 (2–3), type 2 (1–2) and type 2 (1–2) respectively. Among IFC groups, the time duration of constipation was 3 years (2–5), 3 years (1.75–4), and 4 years (3–7) for NTC, OOC, and STC groups respectively. Overall, no significant differences were found among the gender, ages, BMIs, and Z-scores of the 4 groups. There were significant differences in stool frequency and Bristol stool scale between the HC and IFC subtype groups (p < 0.001), with no significant difference within each subtype (all p > 0.05). The participant demographics are shown in Table 1.
Clinical characteristics
We compared the PAC-SYM, PAC-QOL, and SEFCQ scores among the four groups. Table 2 shows the medians of the PAC-SYM, PAC-QOL, and SEFCQ scores of the NTC, OOC, STC, and HC groups. There were significant differences in PAC-SYM scores among the four groups (p < 0.001). Pairwise comparison between the HC group and IFC subtype groups also showed significant differences (p < 0.001); however, the NTC, OOC, and STC groups presented similar scores in all the PAC-SYM subscales (abdominal: p = 0.631; rectal: p = 0.762; stool: p = 0.686). There were significant differences (p < 0.001) in SEFCQ scores among the four groups. Pairwise comparison between the HC group and IFC subtype groups significant differences (p < 0.001). Significant differences were also found in PAC-QOL scores among the four groups (p < 0.001). Pairwise comparison between the HC group and IFC subtype groups showed significant differences (p < 0.001). NTC, OOC, and STC groups showed similar PAC-QOL scores in the 3 domains: worries and concerns, psychosocial discomfort, and physical discomfort (p = 0.853, p = 0.953, and p = 0.347 respectively). However, there was a significant difference in the satisfaction domain among the three subtypes (Kruskal-Wallis Test: p = 0.018) for the PAC-QOL subscale score of the satisfaction among the 3 groups. The comparisons of the NTC, OOC, and STC groups are shown in Supplementary Table S1.
Serum concentration of gastrointestinal motility-related hormones
Table 3 shows the serum concentrations of gastrointestinal motility-related hormones (MTL, GHRL, VIP, CCK, and GLP-1) of the NTC, OOC, STC, and HC groups. NTC, OOC, and STC patients had less MTL compared to the patients in the HC group (p < 0.001) and STC patients showed the most reduced MTL level among all groups (STC vs. NTC, p < 0.001; STC vs. OOC, p < 0.001; NTC vs. OOC, p = 0.437). VIP release was significantly higher in STC patients compared to NTC, OOC, and HC patients (STC vs. NTC, p < 0.001; STC vs. OOC, p < 0.001; NTC vs. OOC, p = 0.570). In addition, the HC group's VIP was significantly lower than that of all the IFC subtypes (p < 0.001). Serum CCK and GHRL concentrations were lower in the IFC groups than in the HC group (p < 0.001), though these two hormones did not differ statistically among the NTC, OOC, and STC groups. On the other hand, unlike the other gastrointestinal motility-related hormones, the concentrations of GLP-1 were similar between IFC patients and healthy controls. The pairwise comparisons of the HC, NTC, OOC, and STC groups are shown in Supplementary Table S2.
Machine-learning analysis
In terms of significant variables, stool frequency, PAC-QOL (Satisfaction), SEFCQ (Emotion), MTL, and VIP were chosen by SelectKBest. Figure 2 shows the feature importance of the variables while Figure 3 is a heat map that illustrates the correlation of the 5 variables. We developed three ML algorithms using SVM, RF, and LGBM. These algorithms were trained using the 5 features from the same dataset. We then validated our three ML algorithms using a stratified 5-fold cross validation. Among the three ML models, SVM and RF showed an accuracy of 79.0% and 80.0%, respectively. The optimized LGBM model outperformed the other two with an 83.8% accuracy. The SVM model had a precision of 82.7%, a recall of 81.7%, and a f1-score of 80.3%. The RF model had a precision of 80.4%, a recall of 79.6%, and a f1-score of 79.6%. The LGBM model had a precision of 84.5%, a recall of 83.6%, and a f1-score of 83.4% (Table 4). Figure 4 shows the confusion matrix demonstrating the results of the LGBM model prediction vs. CTT results. The LGBM model was able to accurately classify all those in the HC group, correctly subtype all those in the NTC and STC groups, and manage to correctly subtype 31 out of 36 of those in the OOC group (5 were misclassified as NTC patients). Figure 5 shows the receiver operating characteristic curves for IFC subtype classification of the LGBM model.
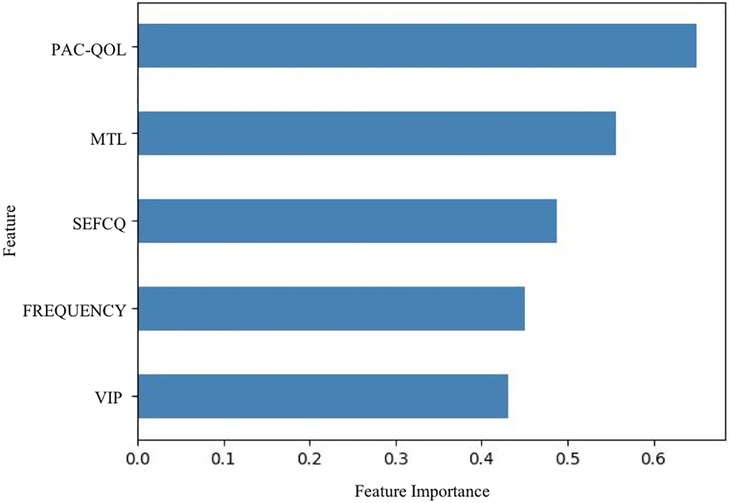
Figure 2. Feature importance of variables estimated by selectKBest. The importance of the features were evaluated by SelectKBest. Of the 15 features that were evaluated, these are the top 5 features used in the prediction models, stool frequency, PAC-QOL, SEFCQ, MTL, VIP. FREQUENCY, stool frequency; PAC-QOL, PAC-QOL (Satisfaction); SEFCQ, SEFCQ (Emotion); MTL, motilin; VIP, vasoactive intestinal peptide.
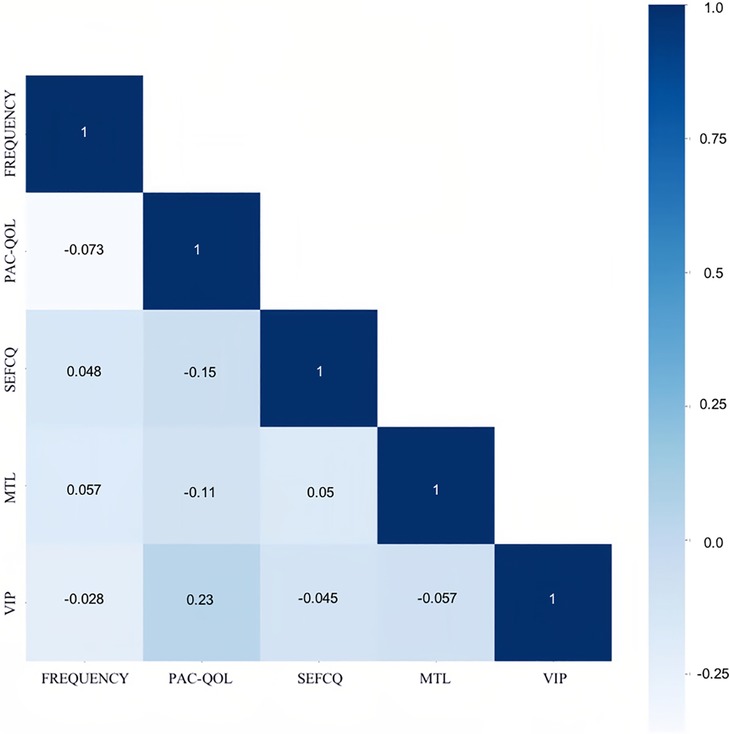
Figure 3. The correlation of the best variables through the heat map. The Spearman correlation was used for this heat map matrix of the top 5 variables as identified by SelectKBest. Each variable on the heat map has a correlation coefficient represented by the color gradient scale. The more positively correlated the features are the darker the shades of blue. FREQUENCY, stool frequency; PAC-QOL, PAC-QOL (Satisfaction); SEFCQ, SEFCQ (Emotion); MTL, motilin; VIP, vasoactive intestinal peptide.
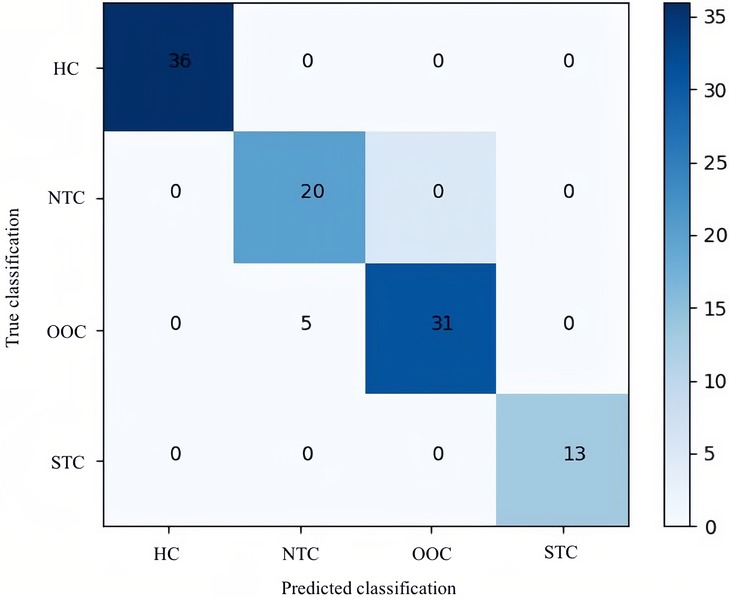
Figure 4. Confusion matrix of the optimal LGBM model. This confusion matrix depicts the accuracy of the LGBM model in IFC subtype classification. The horizontal axis is the predicted label of IFC subtype classification, and the vertical axis is the true label. According to the matrix, of the 36 healthy controls included in the test data, 36 were predicted correctly; of the 25 children with NTC, 20 were predicted correctly; of the 31 children with OOC all were predicted correctly; and of the 13 children with STC, 13 all were predicted correctly by LGBM. HC, healthy controls; NTC, normal transit constipation; OOC, outlet obstruction constipation; STC, slow transit constipation.
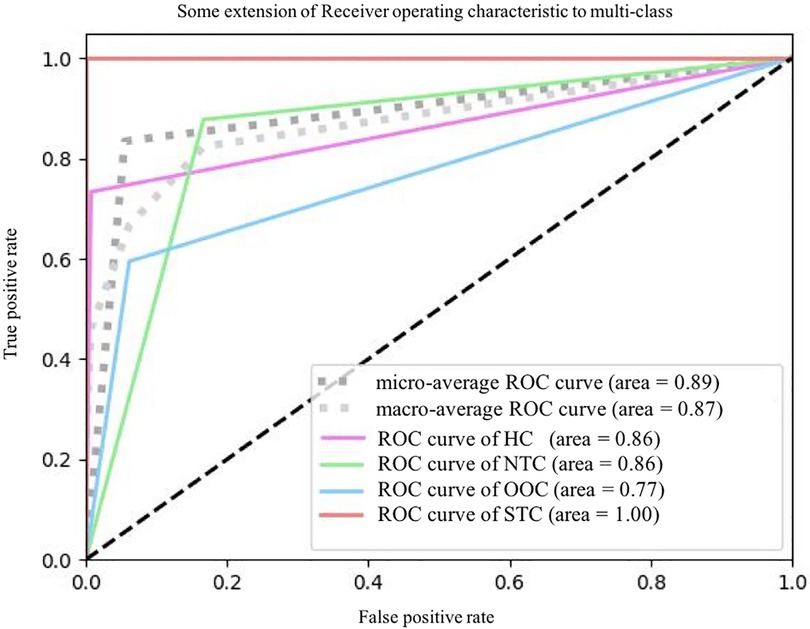
Figure 5. The ROC plots of LGBM classification. ROC, receiver operating characteristic curve; HC, healthy controls; NTC, normal transit constipation; OOC, outlet obstruction constipation; STC, slow transit constipation.
Discussion
Children without alarming symptoms of functional constipation, including signs of congenital abnormalities, stunted growth, and symptoms suggestive of sexual abuse, are not routinely recommended to undergo early diagnostic screening (4). However, it is estimated that nearly half of the children with FC do not recover and still depend on laxatives even after 6 to 12 months of treatments (42, 43). Some of these children have poor responses to conservative treatment strategies, with persistent severe symptoms impacting their quality of life (6). Early diagnosis of IFC subtype enables clinicians to find better management strategies and more effective treatments for IFC children rather than using one generalized treatment method. This study was designed to identify the differences in clinical characteristics using specific constipation questionnaires and gastrointestinal motility-related hormones of children with IFC subtypes. Among the variables of the dataset, stool frequency, PAC-QOL (Satisfaction), SEFCQ (Emotion), MTL, VIP are the most distinguishing features of IFC with significant differences between subtypes. To the best of our knowledge, this is the first study that uses ML algorithms to subtype IFC in children. Out of the three ML models investigated, LGBM performed the best, with an accuracy of 83.8%.
One of our aims was to investigate the clinical differences among the HC, NTC, OOC, and STC groups in the pediatric population. We evaluated constipation symptoms, quality of life, and defecation self-efficacy through the use of specific questionnaires (e.g., PAC-SYM, SEFCQ, and PAC-QOL) as well as clinical tools like the Bristol stool scale. Stool frequency, SEFCQ (Emotion), and PAC-QOL (Satisfaction) were selected for model development. Riezzo et al. observed that the frequency of defecation between STC and NTC groups showed a significant difference (28). Jiang et al. reported that OOC patients had more severe constipation symptoms and significantly worse PAC-SYM (Stool) scores than STC and NTC patients in the Chinese chronic constipation adult population (44). Santucci et al. stated that the SEFCQ (Emotion) scale addresses emotions associated with a child's ability to defecate like worry and sadness, which relate to the fear of painful defecation. Low defecation self-efficacy can cause anxiety and may contribute to decreased compliance of behavioral change, which exacerbates constipation symptoms (36). Our PAC-QOL results of the STC group are consistent with those of Melanie et al. who pointed out that children with STC may experience impaired physical and emotional functions (45). The Bristol stool scale ranks stool consistency from type 1 (hard separate lumps) to type 7 (liquid with no solid pieces) and is widely used in clinical practice and research (46). Saad et al. discovered that types 1 and 2 of the Bristol stool scale were predictive of STC in 46 patients with IFC (47).
The pathogenesis of FC is still yet unknown and though some studies have noted the possible role of gastrointestinal motility-related hormones. Thus, the second aim of this study was to assess the serum levels of MTL, GHRL, VIP, CCK, and GLP-1 in children with NTC, OOC, and STC in comparison to those of healthy controls. MTL and VIP were selected, out of all the serum hormone concentrations we evaluated, as two of the features used in our models. In our study, there was a considerable difference in MTL serum levels between the HC group and the 3 IFC subtype groups. Moreover, the MTL serum level of the STC group was lower than that of the OOC and NTC groups. Our findings are consistent with previous research investigating FC in patients with decreased MTL levels in the fasting state (48). Peracchi et al. showed that MTL decreased in the postprandial state in STC patients (49). Additionally, Giuseppe Riezzo et al. demonstrated that MTL level was lower in the STC group than in the control and NTC groups (28). It has also been observed that children with constipation have lower serum MTL levels than healthy children do (50). In our study, the serum VIP levels of IFC subtype groups were significantly increased compared to those in the HC group. In addition, the serum levels of STC groups were much higher than those of the OOC and NTC groups. VIP has been identified as an inhibitory neurotransmitter that relaxes the smooth muscles of the gastrointestinal tract, thereby restraining gastrointestinal motility (51). The levels of neurotransmitters are closely related to the motility of the gastrointestinal tract (34, 52, 53). An in vivo experiment in rats by Zhu et al. has found that an excessively high level of VIP causes intestinal peristalsis to slow down inducing constipation; the serum VIP level decreased after treatment and symptoms were relieved (54). A clinical study by Ling Cheng et al. found that the colon muscle cells of female STC patients have an increased response to inhibitors like VIP that stimulate G protein-coupled receptors (55). Zhizhuwan, a traditional Chinese medicine regulating GI motility, greatly relieved constipation symptoms and decreased VIP level in patients with constipating diabetes mellitus (56).
At present, the only way to subtype IFC in children is with the ROM test to determine the child's CTT (9). However, it is difficult for children to swallow the radiopaque marker capsules and it takes at least 3 days to obtain and interpret the results. Machine learning (ML) can rapidly process high-dimensional data and identify correlations between many variables, making it very efficient when used for disease prediction, diagnosis, and precision (57, 58). Logistic regression, SVM, K Nearest Neighbors, Decision Trees, RF, and Gradient Boosting Machine have been frequently used in pediatric studies (59–62). Specifically, applications of supervised machine learning have been used in studies for disease prediction (63). The majority of artificial intelligence research in gastroenterology focuses on adult diseases but a number of pediatric diseases could benefit from more studies in ML fields (64). Jasbir Dhaliwal et al. used a similarity network fusion and RF in a retrospective study to subtype pediatric inflammatory bowel disease with an accuracy of 97% (65). In a prospective study, De Meij et al. used a logistic ridge regression model to analyze microbiota profiles and discriminate 61 healthy controls from 76 children with chronic functional constipation with an accuracy of 82% (33). With fundamental developments in handling complex and multidimensional datasets, traditional statistical methods used in the past are now being gradually replaced by machine learning-based clinical decision support systems (66).
Herein, we analyzed the dataset including clinical characteristics and gastrointestinal motility-related hormones using ML algorithms to classify patients with IFC. SelectKBest data analysis was used for feature selection. For this study, three models used, SVM and RF are considered more conventional models while LGBM is a more recent model that is being utilized in classification of diseases. To avoid overfitting, k-fold cross validation was used. A forward selection was performed with these three models to optimize the model performance. The LGBM model showed the best performance for classification in our case. Tree-based ML models are known to show good results for classification (67–69). Tsai et al. reported that the LGBM model had a superior performance in discriminating patients with bladder cancer from patients with cystitis based on clinical laboratory data, showing its potential as a faster and more cost-effective diagnostic tool (70). Rudo et al. demonstrated that the LGBM model outperformed KNN, SVM, NB, Bagging, RF, and XGBoost in the prediction of diabetes mellitus in countries with a low concentration of medical experts as a possible means of improving healthcare quality with fewer physicians (71). Peng et al. found that LGBM performed better than other clinical models in early identification of acute kidney injury in congestive heart failure patients (72). This model also helped in treatment planning, determining whether renal replacement therapy was need as well as in assessing the short-term prognosis of the patients. The developed LGBM model is deemed to be very effective for supporting physicians in the early management of a variety of diseases including intractable constipation in children.
The limitations of this study should be noted. We could not compare CTT among all groups and evaluate any possible correlation between CTT and gastrointestinal motility-related hormones for all participants because there were insufficient indications to perform the ROM test on healthy children. Also, we did not analyze the serum levels before and after treatment or the changes in stool consistency and symptom relief. Because all participants of this case-control study were recruited from the children's hospital, hospital control bias was inevitable, though we did our best to mitigate this by recruiting participants with a variety of conditions. For the ML analysis, because the sample size of this single-center design is relatively small, external validation was not available. Further verification through prospective clinical studies with a significantly larger prospective cohort of patients is required to confirm the findings of this study.
Conclusion
Children with IFC suffering from severe symptoms (i.e., infrequent defecation, painful bowel movements, and abdominal pain) and responding poorly to the standard conservative treatment will often continue to experience these symptoms in adulthood. The persistence of IFC has a negative impact on their growth, development, and quality of life. With early subtyping of IFC, clinicians can provide management options that target the cause of their constipation whether it is psychiatric therapy for NTC patients, biofeedback therapy to train pelvic muscles for OOC patients, or laxatives and surgery for STC patients. The current method of subtyping IFC using the ROM test to evaluate CTT is time-consuming and involves radiation exposure. Younger children also have more difficulties cooperating with the ROM test. Other methods of subtyping should be considered and further evaluated for more efficient diagnosis of IFC subtypes. Our study indicated the potential of applying ML models to analyze clinical data and gastrointestinal hormone profiles to subtype IFC. This study appears to be the first that uses the LGBM model to subtype IFC in children, shedding new light on the values of features that were not previously recognized.
Data availability statement
The original contributions presented in the study are included in the article/Supplementary Material, further inquiries can be directed to the corresponding author/s.
Ethics statement
The study protocol was reviewed and approved by Institutional Review Board of Children's Hospital of Nanjing Medical University (202110090-1). The legal guardians of each participant provided written informed consents for participation in this study. Written informed consent to participate in this study was provided by the participants’ legal guardian/next of kin.
Author contributions
YH performed the study, collected the data, carried out the initial analyses and drafted the original manuscript. CX performed the analysis and interpretation of the data, trained the machine learning models, and revised the manuscript for the intellectual session. CC revised and edited each iteration of the manuscript. MW and YJ collected and interpreted the data. WL was responsible for data curation and interpretation of the results. YL and ZL contributed to the design of the study, and critically reviewed and revised the manuscript. All authors contributed to the article and approved the submitted version.
Funding
The study was supported by the Special Funds for Scientific and Technological Development of Nanjing, China (ZKX20042); National Natural Science Foundation of China for Distinguished Young Scholars grant (62001241); China Postdoctoral Science Foundation grant (2021M691648).
Conflict of interest
The authors declare that the research was conducted in the absence of any commercial or financial relationships that could be construed as a potential conflict of interest.
Publisher's note
All claims expressed in this article are solely those of the authors and do not necessarily represent those of their affiliated organizations, or those of the publisher, the editors and the reviewers. Any product that may be evaluated in this article, or claim that may be made by its manufacturer, is not guaranteed or endorsed by the publisher.
Supplementary material
The Supplementary Material for this article can be found online at: https://www.frontiersin.org/articles/10.3389/fped.2023.1148753/full#supplementary-material.
References
1. Koppen IJN, Vriesman MH, Saps M, Rajindrajith S, Shi X, van Etten-Jamaludin FS, et al. Prevalence of functional defecation disorders in children: a systematic review and meta-analysis. J Pediatr. (2018) 198:121–30.e6. doi: 10.1016/j.jpeds.2018.02.029
2. Benninga MA, Faure C, Hyman PE, St James Roberts I, Schechter NL, Nurko S. Childhood functional gastrointestinal disorders: neonate/toddler. Gastroenterology. (2016) S0016-5085(16):00182–7. doi: 10.1053/j.gastro.2016.02.016
3. Hyams JS, Di Lorenzo C, Saps M, Shulman RJ, Staiano A, van Tilburg M. Functional disorders: children and adolescents. Gastroenterology. (2016) S0016-5085(16):00181–5. doi: 10.1053/j.gastro.2016.02.015
4. Tabbers MM, DiLorenzo C, Berger MY, Faure C, Langendam MW, Nurko S, et al. Evaluation and treatment of functional constipation in infants and children: evidence-based recommendations from ESPGHAN and NASPGHAN. J Pediatr Gastroenterol Nutr. (2014) 58(2):258–74. doi: 10.1097/MPG.0000000000000266
5. Wilkinson-Smith V, Bharucha AE, Emmanuel A, Knowles C, Yiannakou Y, Corsetti M. When all seems lost: management of refractory constipation-surgery, rectal irrigation, percutaneous endoscopic colostomy, and more. Neurogastroenterol Motil. (2018) 30(5):e13352. doi: 10.1111/nmo.13352
6. Belsey J, Greenfield S, Candy D, Geraint M. Systematic review: impact of constipation on quality of life in adults and children. Aliment Pharmacol Ther. (2010) 31(9):938–49. doi: 10.1111/j.1365-2036.2010.04273.x
7. Wald ER, Di Lorenzo C, Cipriani L, Colborn DK, Burgers R, Wald A. Bowel habits and toilet training in a diverse population of children. J Pediatr Gastroenterol Nutr. (2009) 48(3):294–8. doi: 10.1097/mpg.0b013e31817efbf7
8. Bongers MEJ, van Wijk MP, Reitsma JB, Benninga MA. Long-term prognosis for childhood constipation: clinical outcomes in adulthood. Pediatrics. (2010) 126(1):e156–162. doi: 10.1542/peds.2009-1009
9. Tambucci R, Quitadamo P, Thapar N, Zenzeri L, Caldaro T, Staiano A, et al. Diagnostic tests in pediatric constipation. J Pediatr Gastroenterol Nutr. (2018) 66(4):e89–98. doi: 10.1097/MPG.0000000000001874
10. Eoff JC. Optimal treatment of chronic constipation in managed care: review and roundtable discussion. J Manag Care Pharm. (2008) 14(9 Suppl A):1–15. doi: 10.18553/jmcp.2008.14.S8-A.1
11. Rao SS. Constipation: evaluation and treatment. Gastroenterol Clin North Am. (2003) 32(2):659–83. doi: 10.1016/s0889-8553(03)00026-8
12. Rao SS. Dyssynergic defecation and biofeedback therapy. Gastroenterol Clin North Am. (2008) 37(3):569. doi: 10.1016/j.gtc.2008.06.011
13. Dinning PG, Wiklendt L, Maslen L, Patton V, Lewis H, Arkwright JW, et al. Colonic motor abnormalities in slow transit constipation defined by high resolution, fibre-optic manometry. Neurogastroenterol Motil. (2015) 27(3):379–88. doi: 10.1111/nmo.12502
14. Bautista Casasnovas A, Varela Cives R, Villanueva Jeremias A, Castro-Gago M, Cadranel S, Tojo Sierra R. Measurement of colonic transit time in children. J Pediatr Gastroenterol Nutr. (1991) 13(1):42–5. doi: 10.1097/00005176-199107000-00008
15. Lundin E, Graf W, Garske U, Nilsson S, Maripuu E, Karlbom U. Segmental colonic transit studies: comparison of a radiological and a scintigraphic method. Colorectal Dis. (2007) 9(4):344–51. doi: 10.1111/j.1463-1318.2006.01153.x
16. Maurer AH, Camilleri M, Donohoe K, Knight LC, Madsen JL, Mariani G, et al. The SNMMI and EANM practice guideline for small-bowel and colon transit 1.0. J Nucl Med. (2013) 54(11):2004–13. doi: 10.2967/jnumed.113.129973
17. Rao SS, Seaton K, Miller M, Brown K, Nygaard I, Stumbo P, et al. Randomized controlled trial of biofeedback, sham feedback, and standard therapy for dyssynergic defecation. Clin Gastroenterol Hepatol. (2007) 5(3):331–8. doi: 10.1016/j.cgh.2006.12.023
18. Camilleri M, Brandler J. Refractory constipation: how to evaluate and treat. Gastroenterol Clin North Am. (2020) 49(3):623–42. doi: 10.1016/j.gtc.2020.05.002
19. Sharma A, Rao SSC, Kearns K, Orleck KD, Waldman SA. Review article: diagnosis, management and patient perspectives of the spectrum of constipation disorders. Aliment Pharmacol Ther. (2021) 53(12):1250–67. doi: 10.1111/apt.16369
20. Gribble FM, Reimann F. Enteroendocrine cells: chemosensors in the intestinal epithelium. Annu Rev Physiol. (2016) 78(1):277–99. doi: 10.1146/annurev-physiol-021115-105439
21. Wang JK, Yao SK. Roles of gut Microbiota and metabolites in pathogenesis of functional constipation. Evid Based Complement Alternat Med. (2021) 2021:5560310. doi: 10.1155/2021/5560310
22. Miyano Y, Sakata I, Kuroda K, Aizawa S, Tanaka T, Jogahara T, et al. The role of the vagus nerve in the migrating motor complex and ghrelin- and motilin-induced gastric contraction in suncus. PLoS One. (2013) 8(5):e64777. doi: 10.1371/journal.pone.0064777
23. Wu T, Rayner CK, Young RL, Horowitz M. Gut motility and enteroendocrine secretion. Curr Opin Pharmacol. (2013) 13(6):928–34. doi: 10.1016/j.coph.2013.09.002
24. Umetsu Y, Tenno T, Goda N, Shirakawa M, Ikegami T, Hiroaki H. Structural difference of vasoactive intestinal peptide in two distinct membrane-mimicking environments. Biochim Biophys Acta. (2011) 1814(5):724–30. doi: 10.1016/j.bbapap.2011.03.009
25. Charoenthongtrakul S, Giuliana D, Longo KA, Govek EK, Nolan A, Gagne S, et al. Enhanced gastrointestinal motility with orally active ghrelin receptor agonists. J Pharmacol Exp Ther. (2009) 329(3):1178–86. doi: 10.1124/jpet.108.150193
26. Crawley JN, Corwin RL. Biological actions of cholecystokinin. Peptides. (1994) 15(4):731–55. doi: 10.1016/0196-9781(94)90104-x
27. Hellström PM, Näslund E, Edholm T, Schmidt PT, Kristensen J, Theodorsson E, et al. GLP-1 suppresses gastrointestinal motility and inhibits the migrating motor complex in healthy subjects and patients with irritable bowel syndrome. Neurogastroenterol Motil. (2008) 20(6):649–59. doi: 10.1111/j.1365-2982.2007.01079.x
28. Riezzo G, Chimienti G, Clemente C, D’Attoma B, Orlando A, Mammone Rinaldi C, et al. Colonic transit time and gut peptides in adult patients with slow and normal colonic transit constipation. BioMed Res Int. (2017) 2017:1–10. doi: 10.1155/2017/3178263
29. Rybak A, Martinelli M, Thapar N, Van Wijk MP, Vandenplas Y, Salvatore S, et al. Colonic function investigations in children: review by the ESPGHAN motility working group. J Pediatr Gastroenterol Nutr. (2022) 74(5):681–92. doi: 10.1097/MPG.0000000000003429
30. Rao SS, Kuo B, McCallum RW, Chey WD, DiBaise JK, Hasler WL, et al. Investigation of colonic and whole-gut transit with wireless motility capsule and radiopaque markers in constipation. Clin Gastroenterol Hepatol. (2009) 7(5):537–44. doi: 10.1016/j.cgh.2009.01.017
31. Rajkomar A, Dean J, Kohane I. Machine learning in medicine. N Engl J Med. (2019) 380(14):1347–58. doi: 10.1056/NEJMra1814259
32. Beam AL, Kohane IS. Big data and machine learning in health care. JAMA. (2018) 319(13):1317. doi: 10.1001/jama.2017.18391
33. de Meij TGJ, de Groot EFJ, Eck A, Budding AE, Kneepkens CMF, Benninga MA, et al. Characterization of microbiota in children with chronic functional constipation. PLoS ONE. (2016) 11(10):e0164731. doi: 10.1371/journal.pone.0164731
34. Chen Y, Wu T, Lu W, Yuan W, Pan M, Lee YK, et al. Predicting the role of the human gut microbiome in constipation using machine-learning methods: a meta-analysis. Microorganisms. (2021) 9(10):2149. doi: 10.3390/microorganisms9102149
35. Frank L, Kleinman L, Farup C, Taylor L, Miner P. Psychometric validation of a constipation symptom assessment questionnaire. Scand J Gastroenterol. (1999) 34(9):870–7. doi: 10.1080/003655299750025327
36. Huang Y, Wang Y, Chen Y, Gu X, Yang J, Ma B, et al. Measuring self-efficacy in defecation: validation and utilization of a Chinese version of the self-efficacy for functional constipation questionnaire (SEFCQ) in a pediatric population. Neurogastroenterology Motil. (2022) 34(1). doi: 10.1111/nmo.14255
37. Ruiz-López MC, Coss-Adame E. Quality of life in patients with different constipation subtypes based on the Rome III criteria. Rev Gastroenterol Mex. (2015) 80(1):13–20. doi: 10.1016/j.rgmx.2015.01.003
38. Zaslavsky C, da Silveira TR, Maguilnik I. Total and segmental colonic transit time with radio-opaque markers in adolescents with functional constipation. J Pediatr Gastroenterol Nutr. (1998) 27(2):138–42. doi: 10.1097/00005176-199808000-00002
39. Metcalf AM, Phillips SF, Zinsmeister AR, MacCarty RL, Beart RW, Wolff BG. Simplified assessment of segmental colonic transit. Gastroenterology. (1987) 92(1):40–7. doi: 10.1016/0016-5085(87)90837-7
40. Roberts JP, Newell MS, Deeks JJ, Waldron DW, Garvie NW, Williams NS. Oral [111In] DTPA scintigraphic assessment of colonic transit in constipated subjects. Digest Dis Sci. (1993) 38(6):1032–9. doi: 10.1007/BF01295718
41. Arhan P, Devroede G, Jehannin B, Lanza M, Faverdin C, Dornic C, et al. Segmental colonic transit time. Dis Colon Rectum. (1981) 24(8):625–9. doi: 10.1007/BF02605761
42. Pijpers MA, Bongers MEJ, Benninga MA, Berger MY. Functional constipation in children: a systematic review on prognosis and predictive factors. J Pediatr Gastroenterol Nutr. (2010) 50(3):256–68. doi: 10.1097/MPG.0b013e3181afcdc3
43. Tabbers MM, Boluyt N, Berger MY, Benninga MA. Constipation in children. BMJ Clin Evid. (2010) 2010:0303. PMID: 21718570; PMCID: PMC2907595
44. Jiang Y, Tang Y, Lin L. Clinical characteristics of different primary constipation subtypes in a Chinese population. J Clin Gastroenterol. (2020) 54(7):626–32. doi: 10.1097/MCG.0000000000001269
45. Clarke MCC, Chow CS, Chase JW, Gibb S, Hutson JM, Southwell BR. Quality of life in children with slow transit constipation. J Pediatr Surg. (2008) 43(2):320–4. doi: 10.1016/j.jpedsurg.2007.10.020
46. Lewis SJ, Heaton KW. Stool form scale as a useful guide to intestinal transit time. Scand J Gastroenterol. (1997) 32(9):920–4. doi: 10.3109/00365529709011203
47. Saad RJ, Rao SS, Koch KL, Kuo B, Parkman HP, McCallum RW, et al. Do stool form and frequency correlate with whole-gut and colonic transit? Results from a multicenter study in constipated individuals and healthy controls. Am J Gastroenterol. (2010) 105(2):403–11. doi: 10.1038/ajg.2009.612
48. Aydin S, Donder E, Akin OK, Sahpaz F, Kendir Y, Alnema MM. Fat-free milk as a therapeutic approach for constipation and the effect on serum motilin and ghrelin levels. Nutrition. (2010) 26(10):981–5. doi: 10.1016/j.nut.2009.11.023
49. Peracchi M, Basilisco G, Tagliabue R, Terrani C, Locati A, Bianchi PA, et al. Postprandial gut peptide plasma levels in women with idiopathic slow-transit constipation. Scand J Gastroenterol. (1999) 34(1):25–8. doi: 10.1080/00365529950172781
50. Ulusoy E, Arslan N, Küme T, Ülgenalp A, Çirali C, Bozkaya Ö, et al. Serum motilin levels and motilin gene polymorphisms in children with functional constipation. Minerva Pediatr. (2021) 73(5):420–5. doi: 10.23736/S2724-5276.16.04369-X
51. Iwasaki M, Akiba Y, Kaunitz JD. Recent advances in vasoactive intestinal peptide physiology and pathophysiology: focus on the gastrointestinal system. F1000Res. (2019) 8:1629. doi: 10.12688/f1000research.18039.1
52. Long L, Wang J, Chen N, Zheng S, Shi L, Xu Y, et al. Curcumin ameliorates reserpine-induced gastrointestinal mucosal lesions through inhibiting IκB-α/NF-κB pathway and regulating expression of vasoactive intestinal peptide and gastrin in rats. J Med Food. (2016) 19(6):528–34. doi: 10.1089/jmf.2015.3570
53. Hu TG, Wen P, Fu HZ, Lin GY, Liao ST, Zou YX. Protective effect of mulberry (morus atropurpurea) fruit against diphenoxylate-induced constipation in mice through the modulation of gut microbiota. Food Funct. (2019) 10(3):1513–28. doi: 10.1039/c9fo00132h
54. Zhu J, Tong H, Ye X, Zhang J, Huang Y, Yang M, et al. The effects of low-dose and high-dose decoctions of fructus aurantii in a rat model of functional dyspepsia. Med Sci Monit. (2020) 26:e919815. doi: 10.12659/MSM.919815
55. Cheng L, Biancani P, Behar J. Progesterone receptor a mediates VIP inhibition of contraction. Am J Physiol-Gastrointes Liver Physiol. (2009) 298(3):G433–9. doi: 10.1152/ajpgi.00346.2009
56. Liu Y, Zhao XR, Wang R, Qiu GQ, Zhang M. Effect of Zhizhuwan on gastrointestinal peptide concentrations in plasma of diabetic gastroenteropathy with constipation patients. Zhongguo Zhong Yao Za Zhi. (2008) 33(24):2966–8. PMID: 19294863
57. Yu MK, Ma J, Fisher J, Kreisberg JF, Raphael BJ, Ideker T. Visible machine learning for biomedicine. Cell. (2018) 173(7):1562–5. doi: 10.1016/j.cell.2018.05.056
58. Esteva A, Robicquet A, Ramsundar B, Kuleshov V, DePristo M, Chou K, et al. A guide to deep learning in healthcare. Nat Med. (2019) 25(1):24–9. doi: 10.1038/s41591-018-0316-z
59. Oke I, VanderVeen D. Machine learning applications in pediatric ophthalmology. Semin Ophthalmol. (2021) 36(4):210–7. doi: 10.1080/08820538.2021.1890151
60. Patel D, Hall GL, Broadhurst D, Smith A, Schultz A, Foong RE. Does machine learning have a role in the prediction of asthma in children? Paediatr Respir Rev. (2022) 41:51–60. doi: 10.1016/j.prrv.2021.06.002
61. Goodlich BI, Armstrong EL, Horan SA, Baque E, Carty CP, Ahmadi MN, et al. Machine learning to quantify habitual physical activity in children with cerebral palsy. Dev Med Child Neurol. (2020) 62(9):1054–60. doi: 10.1111/dmcn.14560
62. Brons A, de Schipper A, Mironcika S, Toussaint H, Schouten B, Bakkes S, et al. Assessing children’s fine motor skills with sensor-augmented toys: machine learning approach. J Med Internet Res. (2021) 23(4):e24237. doi: 10.2196/24237
63. Bi Q, Goodman KE, Kaminsky J, Lessler J. What is machine learning? A primer for the epidemiologist. Am J Epidemiol. (2019) 188(12):2222–39. doi: 10.1093/aje/kwz189
64. Patel V, Khan MN, Shrivastava A, Sadiq K, Ali SA, Moore SR, et al. Artificial intelligence applied to gastrointestinal diagnostics: a review. J Pediatr Gastroenterol Nutr. (2020) 70(1):4–11. doi: 10.1097/MPG.0000000000002507
65. Dhaliwal J, Erdman L, Drysdale E, Rinawi F, Muir J, Walters TD, et al. Accurate classification of pediatric colonic inflammatory bowel disease subtype using a random forest machine learning classifier. J Pediatr Gastroenterol Nutr. (2021) 72(2):262–9. doi: 10.1097/MPG.0000000000002956
66. Tjoa E, Guan C. A survey on explainable artificial intelligence (XAI): toward medical XAI. IEEE Trans Neural Netw Learn Syst. (2021) 32(11):4793–813. doi: 10.1109/TNNLS.2020.3027314
67. Rhodes NJ, O’Donnell JN, Lizza BD, McLaughlin MM, Esterly JS, Scheetz MH. Tree-based models for predicting mortality in gram-negative bacteremia: avoid putting the CART before the horse. Antimicrob Agents Chemother. (2016) 60(2):838–44. doi: 10.1128/AAC.01564-15
68. Freeman C, Kuli D, Basir O. Feature-selected tree-based classification. IEEE Trans Cybern. (2013) 43(6):1990–2004. doi: 10.1109/TSMCB.2012.2237394
69. Parikh SA, Gomez R, Thirugnanasambandam M, Chauhan SS, De Oliveira V, Muluk SC, et al. Correction to: decision tree based classification of abdominal aortic aneurysms using geometry quantification measures. Ann Biomed Eng. (2019) 47(1):332. doi: 10.1007/s10439-018-02131-x
70. Tsai IJ, Shen WC, Lee CL, Wang HD, Lin CY. Machine learning in prediction of bladder cancer on clinical laboratory data. Diagnostics (Basel). (2022) 12(1):203. doi: 10.3390/diagnostics12010203
71. Rufo DD, Debelee TG, Ibenthal A, Negera WG. Diagnosis of diabetes Mellitus using gradient boosting machine (LightGBM). Diagnostics (Basel). (2021) 11(9):1714. doi: 10.3390/diagnostics11091714
Keywords: intractable constipation, constipation subtypes, children, machine learning, quality of life
Citation: Huang Y-H, Xie C, Chou C-Y, Jin Y, Li W, Wang M, Lu Y and Liu Z (2023) Subtyping intractable functional constipation in children using clinical and laboratory data in a classification model. Front. Pediatr. 11:1148753. doi: 10.3389/fped.2023.1148753
Received: 20 January 2023; Accepted: 3 April 2023;
Published: 24 April 2023.
Edited by:
Daniele Zama, Sant'Orsola-Malpighi Polyclinic, ItalyReviewed by:
David Avelar Rodriguez, University of Toronto, CanadaStephen Walker, Wake Forest University, United States
Kip Zimmerman, Wake Forest University, United States
© 2023 Huang, Xie, Chou, Jin, Li, Wang, Lu and Liu. This is an open-access article distributed under the terms of the Creative Commons Attribution License (CC BY). The use, distribution or reproduction in other forums is permitted, provided the original author(s) and the copyright owner(s) are credited and that the original publication in this journal is cited, in accordance with accepted academic practice. No use, distribution or reproduction is permitted which does not comply with these terms.
*Correspondence: Yan Lu bHV5YW5fY3B1QDE2My5jb20= Zhifeng Liu emZsaXVAbmptdS5lZHUuY24=
†These authors have contributed equally to this work and share first authorship
Specialty Section: This article was submitted to Pediatric Gastroenterology, Hepatology and Nutrition, a section of the journal Frontiers in Pediatrics