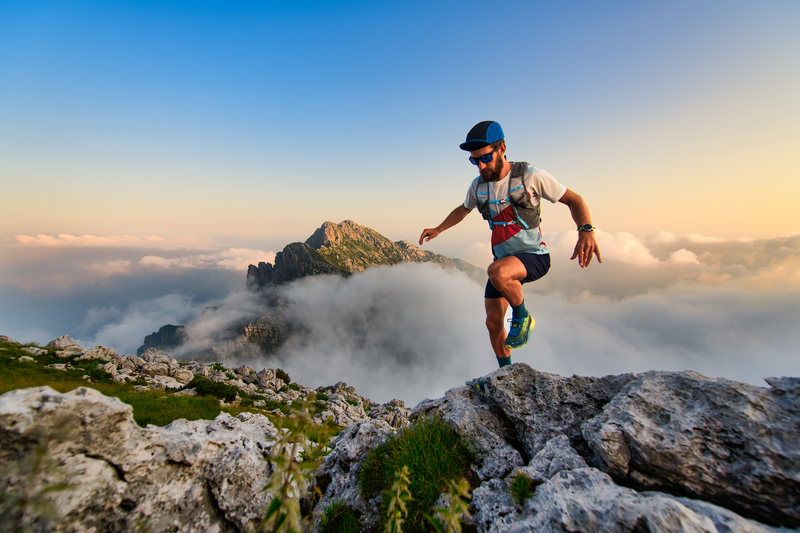
95% of researchers rate our articles as excellent or good
Learn more about the work of our research integrity team to safeguard the quality of each article we publish.
Find out more
ORIGINAL RESEARCH article
Front. Pediatr. , 14 March 2023
Sec. Pediatric Pulmonology
Volume 11 - 2023 | https://doi.org/10.3389/fped.2023.1117142
Backgrounds and Aims: Bronchopulmonary dysplasia (BPD) has serious immediate and long-term sequelae as well as morbidity and mortality. The objective of this study is to develop a predictive model of BPD for premature infants using clinical maternal and neonatal parameters.
Methods: This single-center retrospective study enrolled 237 cases of premature infants with gestational age less than 32 weeks. The research collected demographic, clinical and laboratory parameters. Univariate logistic regression analysis was carried out to screen the potential risk factors of BPD. Multivariate and LASSO logistic regression analysis was performed to further select variables for the establishment of nomogram models. The discrimination of the model was assessed by C-index. The Hosmer-Lemeshow test was used to assess the calibration of the model.
Results: Multivariate analysis identified maternal age, delivery option, neonatal weight and age, invasive ventilation, and hemoglobin as risk predictors. LASSO analysis selected delivery option, neonatal weight and age, invasive ventilation, hemoglobin and albumin as the risk predictors. Both multivariate (AUC = 0.9051; HL P = 0.6920; C-index = 0.910) and LASSO (AUC = 0.8935; HL P = 0.7796; C-index = 0.899) - based nomograms exhibited ideal discrimination and calibration as confirmed by validation dataset.
Conclusions: The probability of BPD in a premature infant could be effectively predicted by the nomogram model based on the clinical maternal and neonatal parameters. However, the model required external validation using larger samples from multiple medical centers.
Bronchopulmonary dysplasia (BPD) is a common chronic lung disease in preterm infants, leading to long-term complications such as cardiopulmonary dysfunction and growth retardation (1, 2). In particular, moderate and severe BPD is a major cause of death and neurodevelopmental disability in preterm infants (3, 4). Although the survival rate of preterm infants has increased significantly with the widespread application of antenatal steroids, exogenous pulmonary surfactant replacement therapy, and the development of neonatal intensive care, the incidence of BPD has been not dramatically decreased (5–7). What's more, BPD has serious immediate and long-term sequelae as well as morbidity and mortality (8–10). Therefore, the prevention of BPD has become a hot topic of clinical concern.
The pathogenesis of BPD is complex, with the underlying cause being impaired development of the immature lung in response to inflammation, hyperoxia, and other damaging factors (11, 12). Prenatal and neonatal factors have been associated with BPD (13, 14). The prenatal factors are related to the lack of antenatal steroid therapy, chorioamnionitis, and maternal hypertension (13, 14). Neonatal factors include gestational age, birth weight, and postdelivery resuscitation (13, 14). The independent prenatal risk factors may be oligohydramnios, male gender, and intrauterine growth restriction (14, 15). Postnatal risk factors seem to be the length of exposure to mechanical ventilation, nosocomial pneumonia, and the necessity for FiO2 of more than 0.30 in the delivery room (14, 15). It is important to prevent and control the occurrence of BPD if the risk of BPD development can be identified and assessed early.
The statistical analysis methods commonly used to select the risk variables contain the logistic regression analysis (16). Logistic regression analysis extends the techniques of multiple regression analysis to research situations in which the outcome variable is categorial (17). The least absolute shrinkage and selection operator (LASSO) regression is a shrinkage and variable selection method for regression models, which has been used to determine the variables of ischemic stroke, Alzheimer's disease, COVID-19, and lymph node metastasis (18–22). Nomogram can help present the risk degree of evidence-based outcomes, and the corresponding mathematical equation addresses the impact of risk factors associated with diseases (23, 24). Many studies have identified the risk factors by LASSO regression or multivariate logistic regression and attempted to visualize the incidence and probability of BPD (25, 26). In this study, we sought out to screen risk prediction factors based on these two methods multivariate logistic regression analysis and LASSO logistic regression analysis. The probability of BPD in a premature infant was visualized and compared by evaluating the discrimination performance.
Premature infants with BPD less than 32 weeks of gestational age had been admitted to the Neonatal Intensive Care Unit of Linyi Central Hospital. This study enrolled preterm infants with gestational age less than 32 weeks. At the same time, we collected demographic, clinical and laboratory data. This study enrolled 237 cases of premature infants. This study protocol was reviewed and approved by the Research Ethics Commission of Linyi Central Hospital (No. LCH-LW-202208).
In this study, 36 cases were excluded because of death within 28 days after birth (n = 16), maternal mental retardation (n = 1), maternal psychiatric abnormality (n = 1), aggravation (n = 6), and transfer (n = 12). The number of premature infants who met the inclusion criteria was 237, and the cases were categorized into training set (n = 189) and validation set (n = 48). The training set was used to select potential risk factors by univariate logistic regression analysis followed by multivariable logistic regression analysis and LASSO regression analysis. Nomogram model was generated and validated using data from validation cohort. There were no significant differences in demographic, clinical and laboratory results between training dataset and validation dataset (Table 1).
BPD was defined as a categorical variable: no BPD and BPD (mild, moderate and severe BPD) according to BPD criteria of the National institute of Child Health and Development (NICHD) (27). In this study, premature infants born with gestational age less than 32 weeks were diagnosed with BPD as defined by oxygen support more than 21% of fraction of inspired oxygen (FiO2) at 36-week postmenstrual age for at least 28 days. Mild BPD was defined as not receiving supplemental oxygen; Moderate BPD was receiving oxygen less than 30% of FiO2; Severe BPD was receiving oxygen support more than 30% FiO2 or needing positive-pressure ventilation or nasal continuous positive airway pressure.
In this study, the research collected the potential risk factors, including demographic, clinical, and laboratory information. We removed the variables with the proportion of missing values greater than or equal to 20% in each cohort. The missing data were interpolated using the random forest technique. As for data preprocessing, values of height and weight of puerperal women, prothrombin time, indirect bilirubin, creatine kinase MB, and creatinine were winsorized. The values of white blood cell, activated partial thromboplastin time, glutamic pyruvic transaminase, creatine kinase MB, and blood urea nitrogen were log-transformed; and values of indirect bilirubin and CO2CP were transformed by squared root function. The categorical variables included birth order, gestational age, Apgar scores (1 min and 5 min), duration of ventilation (more than 1 week), neonatal respiratory distress syndrome (NRDS), plateletcrit, and fibrinogen. The data in 2019, 2021, and 2022 were used as the training set, and the data in 2020 was used as the validation set. The sample size in each group meets the ratio requirement of 7:3. Due to the limited sample size, the data set was randomly split, and the data set was divided into training set and validation set by a ratio of 8:2.
The candidate risk factors were initially analyzed by univariate logistic regression analysis. Multivariate logistic regression analysis was performed for risk factor selection based on forward stepwise selection with a significance level alpha of 0.05, and the selected factors were used for model 1 establishment. Least absolute shrinkage and selection operator (LASSO) was carried out for factor selection with a significance level alpha of 0.15, used for model 2 establishment. SPSS Software was used to perform Student's t test, χ2, Z-test, univariate and multivariate logistic regression analysis. The R package “glmnet” was called for LASSO regression analysis. The nomogram models were developed using the R-package “rms”.
The predictive performance of model 1 and model 2 was evaluated using ROC curve and calibration curve using the data from internal validation cohort and external validation cohort. The calibration of the nomogram was accompanied with the Hosmer–Lemeshow test. Harrell's C-index was used to measure the discrimination performance of the model. Model evaluation was carried out using the R-package.
Univariable logistic regression analysis revealed maternal age, caesarean, gestational age, birth weight, 1 min Apgar scores (<8), 5 min Apgar scores (<8), postnatal asphyxia, IV, and duration of ventilation (>1 week), NRDS Grade III-IV, pulmonary surfactant (PS) application, PS + budesonide, hemoglobin, prothrombin time, albumin, and GLBI may be associated with BPD. Multivariable logistic regression confirmed that advanced maternal age, gestational age less than 29 weeks, and duration of ventilation more than 1 week may the causative factors or indicators for BPD (Table 2). Caesarean, high birth weight, and increased hemoglobin level may decrease the risk of BPD in preterm infants. The LASSO logistic regression model was analyzed using the R-package glmnet. The optimal λ value was determined by cross-validation with the number of folds set to 10. The two dotted lines in Figure 1A represent two values, lambda.min and lambda.1se. Lambda.min is defined as the lambda value of the mean value of the smallest target parameter among the lambda values. As for Lambda.1se, it is the lambda value of the most compact model obtained within a variance range of lambda.min. The red dots represent the mean value of the target parameter, and CI was obtained for the target parameter. The curves in Figure 1B are the trajectory of each independent variable coefficient. As the value of lambda increases, the number of independent variables entering the model decreases. When lambda.1se was selected, the variables delivery, weight and age of premature infants, intensive ventilation more than 7 days, hemoglobin, and albumin were included in the establishment of a risk prediction model 2.
Figure 1. LASSO analysis. (A) selection of demographic and clinical characteristics using the LASSO binary logistic regression model; (B) optimal parameter (lambda) selection in the LASSO model. The ordinate indicates the target parameter. The upper abscissa represents the number of non-zero coefficients; the lower abscissa represents the log(λ).
Table 2. Univariate and multivariable logistic regression analysis using the training dataset for the development of BPD.
The prediction model 1 and model 2 were presented in the nomogram (Figures 2A,B). For model 1, formula for calculating the probability of BPD was computed as: Logit(P|BPD) = ln(P/1 − P) = 5.690 + 0.126*MAge − 1.943*Delivery − 3.226*GWeight + 1.223*GAge + 1.308*intensive ventilation − 0.033*hemoglobin; As for model 2, Logit(P|BPD) = ln(P/1 − P) = 11.2260 − 1.5403* Delivery − 3.1594*GWeight + 1.1190*GAge + 1.2411*intensive ventilation − 0.0269*hemoglobin − 0.0701*albumin, where MAge is maternal age, GWeight and GAge are the birth weight and age of premature infants, respectively. The calibration curve of the nomogram model 1 for the probability of BPD demonstrated good agreement between prediction and observation between prediction and observation in the training cohort and validation cohort (Figures 3A,B). The Hosmer–Lemeshow test (HL) yielded a nonsignificant statistic (P = 0.6823) in training cohort and a nonsignificant difference in validation cohort (P = 0.6920). The calibration curve of the nomogram model 2 for the probability of BPD demonstrated good agreement between prediction and observation in the training cohort and validation cohort (Figures 4A,B). The Hosmer–Lemeshow test (HL) yielded a nonsignificant statistic (P = 0.5331) in training cohort and a nonsignificant difference in validation cohort (P = 0.7796).
Figure 2. Nomogram models estimating the probability of bronchopulmonary dysplasia (BPD) in a premature infant. (A) model 1 incorporates maternal age (MAge), delivery mode, the birth weight and age of premature infants, application of intensive ventilation (IV), and hemoglobin (HGB) level; (B) model 2 includes delivery mode, the birth weight and age of premature infants, application of intensive ventilation (IV), hemoglobin level (HGB), and albumin level (ALBI).
Figure 3. Calibration curves of the nomogram model 1 in the training cohort (A) and validation cohort (B).
Figure 4. Calibration curves of the nomogram model 2 in the training cohort (A) and validation cohort (B).
The C-indexes (AUC) for the prediction nomogram model 1 and model 2 was 0.910 and 0.899 in the training cohort, respectively (Figures 5A,B). The area under the curve (AUC) value of the nomogram model 1 in the validation cohort was 0.9051, and model 2 was 0.8935. LASSO method has a slightly lower AUC comparing to stepwise method. DCA was then applied to compare the clinical usefulness and benefits between model 1 and model 2. The DCA curves of model 1 and model 2 showed indistinct net benefits across a wide range of threshold probability in the training cohort, indicating both model 1 and model 2 possesses clinical usefulness (Figure 6).
Figure 5. Comparison of the predictive sensitivity and specificity of model 1 and model 2 using the training data (A) and validation data (B). Model 1 (red line); model 2 (blue line).
Figure 6. Decision curve analysis (DCA) curves for the model 1 and model 2. The black line is the hypothesis that no patients; the gray line represents the hypothesis that all premature infants have BPD.
Analysis of 189 neonates revealed that 28-day-old neonates were prospectively predicted at the risk of BPD, which may be associated with advanced maternal age, caesarean, gestational age less than 29 weeks, birth weight, 1 min Apgar scores (less than 8), 5 min Apgar scores (less than 8), postnatal asphyxia, intensive ventilation application, duration of ventilation (more than 1 week), NRDS grade III-IV, PS application, PS and budesonide application, decreased hemoglobin, increased prothrombin time, and decreased albumin and GLBI. Risk factors identified by multivariate and LASSO logistic regression analysis were considered early predictors for the development of risk prediction model for BPD. Stepwise and LASSO analysis all selected delivery, birth weight, birth age, intensive ventilation application, and hemoglobin level as predictors. It is clear that LASSO selection method showed better prediction accuracy and interpretability compared to stepwise method.
The maternal parameters were analyzed for selecting risk factors of BPD. The main causes of BPD are advanced maternal age and delivery by caesarean section. There is an ongoing trend in China and developed countries that delayed childbearing shows no signs of diminishing although preterm newborns born to women of increasing maternal age are reported with multiple adverse birth outcomes like BPD (28). For very preterm infants, increasing maternal age is not significantly associated with neonatal mortality or major morbidity (29). Instead, younger maternal age may increase the risk of severe intraventricular hemorrhage in very preterm infants (29). Delivery by caesarean section remained significantly associated with the decreased occurrence of BPD. It is conceivable that these preterm infants born by caesarean section were exposed to postnatal antibiotics, preventing multiple respiratory disorders and lung injury (2).
This study analyzed various antenatal, perinatal, and postnatal factors that may contribute to the development of BPD. Preterm infants born at <29 weeks of gestational age had an increased incidence of BPD. Infants weight was significantly lower in BPD than non-BPD group. Although intensive ventilation and prolonged ventilation can cause lung injury and are risk factors for BPD, lung-protective ventilation is still an important strategy for the current clinical resuscitation of critically ill preterm neonates. Incomplete differentiation of alveolar type 2 cells causes a lack of pulmonary surfactant occurring fairly fate in gestation (30). Pulmonary surfactant replacement therapy and budesonide application were believed to increase the incidence of BPD. However, contrary findings were witnessed with the introduction of the routine application of pulmonary surfactant and budesonide (31–34). It was believed that our study is a retrospective and non-randomized controlled study. Premature infants receiving endotracheal application of pulmonary surfactant and budesonide were mainly with lower body weight and younger gestation age compared to other studies, which may cause a higher incidence of BPD.
BPD severity is greatly associated with Apgar scores at 1 min and 5 min (35, 36). Premature infants diagnosed with BPD were registered with lower 5 min Apgar scores compared with non-BPD. Postnatal asphyxia was linked to the development of BPD as evidenced by our analysis and other studies (37–40). In our preterm infants, NRDS, especially NRDS grade III–IV, is an important cause of BPD. Laboratory examination revealed that premature infants with BPD showed increased prothrombin time and decreased levels of hemoglobin, albumin and globulin. Fetal hemoglobin decreases early, indicating the reduction of endogenous blood component, which has been proposed as a predictive value for BPD development (41). The levels of albumin and globulin have been considered biomarkers associated with BPD in other studies (42).
Multivariate and LASSO logistic regression analysis was carried out to select the predictors for BPD. It is worth noting that LASSO differently selected albumin as the predictors instead of maternal age compared to stepwise method. Both stepwise and LASSO-based nomogram models exhibited good discrimination. Model 1 by stepwise method had a favorable discrimination performance with an AUC of 0.9051, compared to 0.8935 for LASSO logistic regression model 2, analyzed with the validation dataset. A retrospective analysis included risk factors birth weight, gestational age, gender, et al. for generating risk scoring system, of which a sensitivity was 65%–90.3% and a specificity was 77.8%–88% (36, 43). The strengths of our models included a more favorable discrimination performance, comparison of two predictor selection methods stepwise and LASSO logistic regression analysis, and external validation of the risk factors. The established prediction models could be used to predict the probability of BPD for premature infants by using the scoring formulae that were proposed based on maternal age, delivery mode, the birth weight and age of premature infants, application of intensive ventilation, hemoglobin level, and albumin level. The established models may help the clinicians to early diagnose the disease, design therapy project and estimate prognosis. However, our study was limited in selection bias and a relatively small sample of BPD from a single medical center.
This study of risk scoring for BPD supports that the probability of BPD could be predicted for premature infants by maternal age, delivery options, birth weight, birth age, invasive ventilation, hemoglobin and albumin identified by stepwise and LASSO logistic regression analysis. The developed nomogram model 1 and model 2 provided risk predictors for BPD, and explain the potential detriments for premature infants. However, further larger samples from multiple medical centers are required for external validation.
The original contributions presented in the study are included in the article/Supplementary Material, further inquiries can be directed to the corresponding author.
The studies involving human participants were reviewed and approved by the Research Ethics Commission of Linyi Central Hospital. Written informed consent from the participants’ legal guardian/next of kin was not required to participate in this study in accordance with the national legislation and the institutional requirements.
Conception and design: DL. Collection of the data: YGa, YGu and MC. Analysis and interpretation of the data: YGa. Drafting of the paper: YGa. Writing—review & editing: DL, YGu and MC. All authors contributed to the article and approved the submitted version.
The authors declare that the research was conducted in the absence of any commercial or financial relationships that could be construed as a potential conflict of interest.
All claims expressed in this article are solely those of the authors and do not necessarily represent those of their affiliated organizations, or those of the publisher, the editors and the reviewers. Any product that may be evaluated in this article, or claim that may be made by its manufacturer, is not guaranteed or endorsed by the publisher.
1. Hwang JS, Rehan VK. Recent advances in bronchopulmonary dysplasia: pathophysiology, prevention, and treatment. Lung. (2018) 196:129–38. doi: 10.1007/s00408-018-0084-z
2. Thébaud B, Goss KN, Laughon M, Whitsett JA, Abman SH, Steinhorn RH, et al. Bronchopulmonary dysplasia. Nat Rev Dis Primers. (2019) 5:78. doi: 10.1038/s41572-019-0127-7
3. Werthammer J, Brown ER, Neff RK, Taeusch HW Jr. Sudden infant death syndrome in infants with bronchopulmonary dysplasia. Pediatrics. (1982) 69:301–4. doi: 10.1542/peds.69.3.301
4. Gray PH, Burns YR, Mohay HA, O’Callaghan MJ, Tudehope DI. Neurodevelopmental outcome of preterm infants with bronchopulmonary dysplasia. Arch Dis Child Fetal Neonatal Ed. (1995) 73:F128–34. doi: 10.1136/fn.73.3.F128
5. Gilfillan M, Bhandari A, Bhandari V. Diagnosis and management of bronchopulmonary dysplasia. Br Med J. (2021) 375:n1974. doi: 10.1136/bmj.n1974
6. Olaloko O, Mohammed R, Ojha U. Evaluating the use of corticosteroids in preventing and treating bronchopulmonary dysplasia in preterm neonates. Int J Gen Med. (2018) 11:265–74. doi: 10.2147/ijgm.S158184
7. Zhong YY, Li JC, Liu YL, Zhao XB, Male M, Song DK, et al. Early intratracheal administration of corticosteroid and pulmonary surfactant for preventing bronchopulmonary dysplasia in preterm infants with neonatal respiratory distress syndrome: a meta-analysis. Curr Med Sci. (2019) 39:493–9. doi: 10.1007/s11596-019-2064-9
8. Sillers L, Alexiou S, Jensen EA. Lifelong pulmonary sequelae of bronchopulmonary dysplasia. Curr Opin Pediatr. (2020) 32:252–60. doi: 10.1097/MOP.0000000000000884
9. Haggie S, Robinson P, Selvadurai H, Fitzgerald DA. Bronchopulmonary dysplasia: a review of the pulmonary sequelae in the post-surfactant era. J Paediatr Child Health. (2020) 56:680–9. doi: 10.1111/jpc.14878
10. Dassios T, Greenough A. Long-term sequelae of bronchopulmonary dysplasia. Respiratory diseases of the newborn infant sheffield. Eur Respir Soc. (2021). p. 68–78.
11. Pasha AB, Chen XQ, Zhou GP. Bronchopulmonary dysplasia: pathogenesis and treatment. Exp Ther Med. (2018) 16:4315–21. doi: 10.3892/etm.2018.6780
12. Holzfurtner L, Shahzad T, Dong Y, Rekers L, Selting A, Staude B, et al. When inflammation meets lung development—an update on the pathogenesis of bronchopulmonary dysplasia. Mol Cell Pediatr. (2022) 9:1–12. doi: 10.1186/s40348-022-00137-z
13. Klinger G, Sokolover N, Boyko V, Sirota L, Lerner-Geva L, Reichman B. Perinatal risk factors for bronchopulmonary dysplasia in a national cohort of very-low-birthweight infants. Am J Obstet Gynecol. (2013) 208:115.e1–e9. doi: 10.1016/j.ajog.2012.11.026
14. Ramos-Navarro C, Maderuelo-Rodríguez E, Concheiro-Guisán A, Pérez-Tarazona S, Rueda-Esteban S, Sánchez-Torres A, et al. Risk factors and bronchopulmonary dysplasia severity: data from the Spanish bronchopulmonary dysplasia research network. Eur J Pediatr. (2022) 181:789–99. doi: 10.1007/s00431-021-04248-z
15. Shima Y, Kumasaka S, Migita M. Perinatal risk factors for adverse long-term pulmonary outcome in premature infants: comparison of different definitions of bronchopulmonary dysplasia/chronic lung disease. Pediatr Int. (2013) 55:578–81. doi: 10.1111/ped.12151
16. Bursac Z, Gauss CH, Williams DK, Hosmer DW. Purposeful selection of variables in logistic regression. Source Code Biol Med. (2008) 3:1–8. doi: 10.1186/1751-0473-3-17
17. Boateng EY, Abaye DA. A review of the logistic regression model with emphasis on medical research. J Data Anal Inform Process. (2019) 7:190–207. doi: 10.4236/jdaip.2019.74012
19. Meng Z, Wang M, Guo S, Zhou Y, Zheng M, Liu M, et al. Development and validation of a LASSO prediction model for better identification of ischemic stroke: a case-control study in China. Front Aging Neurosci. (2021) 13:630437. doi: 10.3389/fnagi.2021.630437
20. Wang L, Li P, Hou M, Zhang X, Cao X, Li H. Construction of a risk prediction model for Alzheimer's Disease in the elderly population. BMC Neurol. (2021) 21:1–10. doi: 10.1186/s12883-020-02014-6
21. Gao Y, Cai G-Y, Fang W, Li H-Y, Wang S-Y, Chen L, et al. Machine learning based early warning system enables accurate mortality risk prediction for COVID-19. Nat Commun. (2020) 11:1–10. doi: 10.1038/s41467-019-13993-7
22. Kang J, Choi YJ, Kim I-k, Lee HS, Kim H, Baik SH, et al. LASSO-based machine learning algorithm for prediction of lymph node metastasis in T1 colorectal cancer. Cancer Res Treat. (2021) 53:773–83. doi: 10.4143/crt.2020.974
23. Gong J, Ou J, Qiu X, Jie Y, Chen Y, Yuan L, et al. A tool for early prediction of severe coronavirus disease 2019 (COVID-19): a multicenter study using the risk nomogram in Wuhan and Guangdong, China. Clin Infect Dis. (2020) 71:833–40. doi: 10.1093/cid/ciaa443
24. Wang H, Zhang L, Liu Z, Wang X, Geng S, Li J, et al. Predicting medication nonadherence risk in a Chinese inflammatory rheumatic disease population: development and assessment of a new predictive nomogram. Patient Prefer Adherence. (2018) 12:1757. doi: 10.2147/PPA.S159293
25. Zhang J, Luo C, Lei M, Shi Z, Cheng X, Wang L, et al. Development and validation of a nomogram for predicting bronchopulmonary dysplasia in very-low-birth-weight infants. Front Pediatr. (2021) 9:648828. doi: 10.3389/fped.2021.648828
26. Song M, Lei M, Luo C, Shi Z, Cheng X, Ding W, et al. Development of a nomogram for moderate-to-severe bronchopulmonary dysplasia or death: role of N-terminal pro-brain natriuretic peptide as a biomarker. Front Pediatr. (2021) 9:727362. doi: 10.3389/fped.2021.727362
27. Jobe AH, Bancalari E. Bronchopulmonary dysplasia. Am J Respir Crit Care Med. (2001) 163:1723–9. doi: 10.1164/ajrccm.163.7.2011060
28. Kanungo J, James A, McMillan D, Lodha A, Faucher D, Lee SK, et al. Advanced maternal age and the outcomes of preterm neonates: a social paradox? Obstet Gynecol. (2011) 118:872–7. doi: 10.1097/AOG.0b013e31822add60
29. Qi Z, Wang Y, Lin G, Ma H, Li Y, Zhang W, et al. Impact of maternal age on neonatal outcomes among very preterm infants admitted to Chinese neonatal intensive care units: a multi-center cohort study. Transl Pediatr. (2022) 11:1130–9. doi: 10.21037/tp-22-1
30. Whitsett JA, Wert SE, Weaver TE. Diseases of pulmonary surfactant homeostasis. Annu Rev Pathol. (2015) 10:371–93. doi: 10.1146/annurev-pathol-012513-104644
31. Muehlbacher T, Bassler D, Bryant MB. Evidence for the management of bronchopulmonary dysplasia in very preterm infants. Children. (2021) 8:298. doi: 10.3390/children8040298
32. Gharehbaghi MM, Ganji S, Mahallei M. A randomized clinical trial of intratracheal administration of surfactant and budesonide combination in comparison to surfactant for prevention of bronchopulmonary dysplasia. Oman Med J. (2021) 36:e289. doi: 10.5001/omj.2021.84
33. Ng EH, Shah V. Guidelines for surfactant replacement therapy in neonates. Paediatr Child Health. (2021) 26:35–49. doi: 10.1093/pch/pxaa116
34. Banerjee S, Fernandez R, Fox GF, Goss KCW, Mactier H, Reynolds P, et al. Surfactant replacement therapy for respiratory distress syndrome in preterm infants: United Kingdom national consensus. Pediatr Res. (2019) 86:12–4. doi: 10.1038/s41390-019-0344-5
35. Sinkin RA, Cox C, Phelps DL. Predicting risk for bronchopulmonary dysplasia: selection criteria for clinical trials. Pediatrics. (1990) 86:728–36. doi: 10.1542/peds.86.5.728
36. Sharma A, Xin Y, Chen X, Sood BG. Early prediction of moderate to severe bronchopulmonary dysplasia in extremely premature infants. Pediatr Neonatol. (2020) 61:290–9. doi: 10.1016/j.pedneo.2019.12.001
37. Chen ZL, Liu J, Feng ZC. Experts’ consensus on the criteria for the diagnosis and grading of neonatal asphyxia in China. Transl Pediatr. (2013) 2:64–5. doi: 10.3978/j.issn.2224-4336.2013.04.03
38. Doyle LW, Cheong JL, Ehrenkranz RA, Halliday HL. Early (<8 days) systemic postnatal corticosteroids for prevention of bronchopulmonary dysplasia in preterm infants. Cochrane Database Syst Rev. (2017) 10:Cd001146. doi: 10.1002/14651858.CD001146.pub5
39. Bruce GNJC. Bronchopulmonary dysplasia: chronic pulmonary disease following neonatal respiratory failure. Chest. (1985) 87:528–35. doi: 10.1378/chest.87.4.528
40. Roberts D, Brown J, Medley N, Dalziel SR. Antenatal corticosteroids for accelerating fetal lung maturation for women at risk of preterm birth. Cochrane Database Syst Rev. (2017) 3:Cd004454. doi: 10.1002/14651858.CD004454.pub3
41. Hellström W, Martinsson T, Hellstrom A, Morsing E, Ley D. Fetal haemoglobin and bronchopulmonary dysplasia in neonates: an observational study. Arch Dis Child Fetal Neonatal Ed. (2021) 106:88–92. doi: 10.1136/archdischild-2020-319181
42. Balena-Borneman J, Ambalavanan N, Tiwari HK, Griffin RL, Halloran B, Askenazi D. Biomarkers associated with bronchopulmonary dysplasia/mortality in premature infants. Pediatr Res. (2017) 81:519–25. doi: 10.1038/pr.2016.259
Keywords: BPD, logistic regression analysis, risk prediction, preterm infants, nomogram model
Citation: Gao Y, Liu D, Guo Y and Cao M (2023) Risk prediction of bronchopulmonary dysplasia in preterm infants by the nomogram model. Front. Pediatr. 11:1117142. doi: 10.3389/fped.2023.1117142
Received: 6 December 2022; Accepted: 16 February 2023;
Published: 14 March 2023.
Edited by:
Salvatore Cazzato, Azienda Ospedaliero Universitaria Ospedali Riuniti, ItalyReviewed by:
Scott Guthrie, Vanderbilt University Medical Center, United States© 2023 Gao, Liu, Guo and Cao. This is an open-access article distributed under the terms of the Creative Commons Attribution License (CC BY). The use, distribution or reproduction in other forums is permitted, provided the original author(s) and the copyright owner(s) are credited and that the original publication in this journal is cited, in accordance with accepted academic practice. No use, distribution or reproduction is permitted which does not comply with these terms.
*Correspondence: Dongyun Liu TGl1ZG9uZ3l1bjAwN0AxNjMuY29t
Specialty Section: This article was submitted to Pediatric Pulmonology, a section of the journal Frontiers in Pediatrics
Disclaimer: All claims expressed in this article are solely those of the authors and do not necessarily represent those of their affiliated organizations, or those of the publisher, the editors and the reviewers. Any product that may be evaluated in this article or claim that may be made by its manufacturer is not guaranteed or endorsed by the publisher.
Research integrity at Frontiers
Learn more about the work of our research integrity team to safeguard the quality of each article we publish.