- 1Division of Nephrology and Hypertension, Cincinnati Children's Hospital Medical Center, Cincinnati, OH, United States
- 2Division of Biostatics and Epidemiology, Cincinnati Children's Hospital Medical Center, Cincinnati, OH, United States
- 3School of Medicine, University of Cincinnati, Cincinnati, OH, United States
- 4Division of Rheumatology, Cincinnati Children's Hospital Medical Center, Cincinnati, OH, United States
Background: The Renal Activity Index for Lupus (RAIL) is a composite score of six urinary biomarkers (neutrophil gelatinase–associated lipocalin (NGAL), monocyte chemoattractant protein-1 (MCP-1), kidney injury molecule-1 (KIM-1), ceruloplasmin, adiponectin, and hemopexin) used to monitor lupus nephritis activity in children. We tested stability of RAIL biomarkers prior to meaningful clinical use.
Methods: Urine samples were tested by ELISA under shipping conditions, freeze/thaw, ambient and longer-term storage. Statistical analysis was performed via Deming Regression, Bland-Altman and Spearman Correlation Coefficient.
Results: Biomarker concentration were comparable to freshly collected urine following storage at −80 °C for up to 3 months, and at 4 or 25 °C up to 48 h followed by −80 °C. Neither shipping on dry or wet ice exposure nor addition of two freeze-thaw cycles led to loss of signal, with excellent Spearman Correlation coefficients under all conditions.
Conclusions: RAIL biomarkers are stable following short-term storage at clinically relevant conditions.
Introduction
Lupus nephritis (LN) confers a poor prognosis, as 5–25% of patients with proliferative LN develop kidney disease within 5 years of diagnosis, and about 10% of all patients with LN will develop end-stage kidney disease (1, 2). Disease with onset in childhood or adolescence is termed childhood onset SLE, or cSLE. Outcomes for cSLE are worse than that of adults and children have higher occurrence of LN (3–5). Current laboratory testing is inadequate to diagnose LN activity effectively and evaluate response to therapy, which makes evaluating potential new therapies challenging (6, 7). In fact, traditional laboratory measures will classify patients incorrectly 30–40% of the time when compared to kidney biopsy. As such, complement levels and anti-dsDNA antibody titers are not effective for predicting the course of LN (6, 8, 9). Therefore, research has focused on developing new laboratory tests to non-invasively, accurately, and rapidly diagnose LN and detect its response to treatment.
We have previously described and validated a panel of six urinary biomarkers, neutrophil gelatinase-associated lipocalin (NGAL), monocyte chemoattractant protein-1 (MCP-1/CCL2), kidney injury molecule-1 (KIM-1), ceruloplasmin, adiponectin, and hemopexin. Considering the urinary concentration of all six biomarkers, the pediatric Renal Activity Index for Lupus (RAIL) can be calculated, where a higher score reflects high renal inflammation, and the score is >90% accurate in detecting LN activity histologic activity measures (10–12). The standard operating procedures for collecting and storing urine specimens to be used for RAIL biomarker measurement has yet to be established. We have previously demonstrated the short- and long-term of NGAL and KIM-1 in pediatric AKI patients. We found no significant difference between concentration measurements at baseline (immediate testing within 15 min of aliquoting), 24 h at 4 °C, or 24 h at −80 °C (13). In addition, NGAL and KIM-1 demonstrated long-term stability, without relevant degradation at long term storage, for up to 5 years. To further move the RAIL biomarkers toward clinical utility, we conducted stability testing for the remaining four urinary biomarkers that make up the RAIL score under common clinical and experimental conditions. We hypothesized that the markers would be stable under various clinically relevant conditions including shipping, temperatures, and longer storage.
Materials and methods
Patients and samples
Patients enrolled in the SLE Clinical and Research Database were eligible for the study (IRB CCHMC-2008-0635). Patients were recruited over a 1-month period in September 2021 from Nephrology and Rheumatology clinics. Inclusion criteria were diagnosis of SLE by EULAR/ACR 2019 criteria (14) with or without nephritis. There was no requirement based on disease activity. Exclusion criteria were diagnosis of kidney disease other than Lupus Nephritis, diagnosis of autoimmune disease besides SLE, urine volumes below 10 ml or patient unwilling to provide urine.
A random urine sample was collected from each patient and processed within 2 h of collection. Urine was centrifuged for 10 min at 2200x g prior to aliquoting for testing the 10 experimental conditions, as shown in Figure 1. Control condition was freezing at −80 °C and run within 1 week of collection. All thaw times were 45 min.
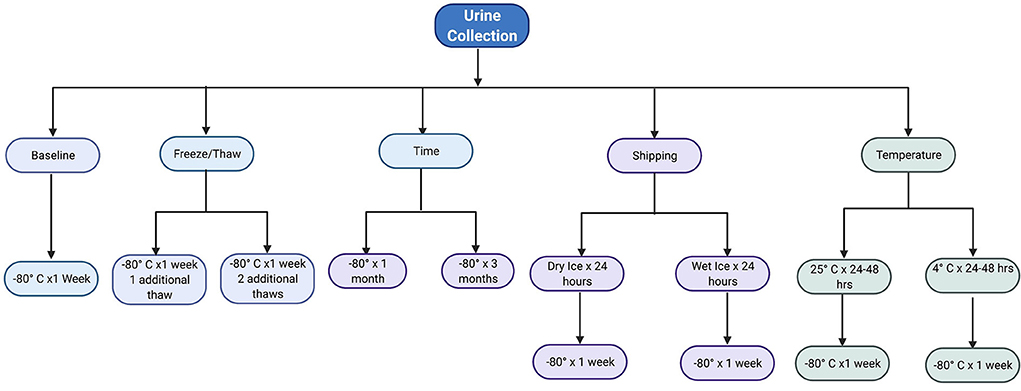
Figure 1. Flowchart of the 10 experimental conditions per urine sample. Image made with BioRender.
Urine biomarker assays
Unless stated otherwise, biomarkers were quantified using commercial ELISA kits as per the manufacturer's instructions. MCP-1 used a four-parameter logistic curve to fit the standard curve. Adiponectin, Ceruloplasmin, and Hemopexin used a log/log curve to fit the standard curve.
Human MCP-1 was measured via ELISA (R&D Systems, Minneapolis, MN, DCP00), diluted 1:1, with a mean minimal detectable dose of 1.7 pg/mL. Intra-assay and inter-assay were 5% and 5.1%, respectively.
Human Adiponectin was measured by commercially available ELISA kit (R&D system, Minneapolis, MN, DRP300), diluted 1:5, with a mean minimal detectable dose of 0.246 ng/ml. Intra-assay and inter-assay coefficients of variation were 3.7 and 6.8%, respectively.
Human Ceruloplasmin was measured by ELISA Kit (Assaypro LLC, St. Charles, MO, EC4201-1), diluted 1:50, with a mean minimal detectable dose of 0.085 ng/ml. Intra-assay and inter-assay were 4.9 and 9.8%, respectively.
Human Hemopexin was measured by commercially available ELISA kit (Assaypro LLC, St. Charles, MO, EH2001-1), diluted 1:20, with a mean minimal detectable dose of 4.2 ng/ml. Intra-assay and inter-assay coefficients of variation were 4.7 and 9.2%, respectively.
Statistical analysis
Natural log-transformation of each biomarker was performed prior to analysis to ensure normal distribution assumptions. Analyte concentrations that were above or below the limits of detection were imputed by 50% of the level of lower limit of detection (LLD) and 50% over the upper limit of detection (ULD), respectively. Means were calculated for each biomarker under each condition. Spearman correlation coefficients were calculated for each experimental condition with the control condition, with of 0.1–0.39 representing weak correlation, 0.4–0.69 representing moderate correlation, 0.7–0.89 representing strong correlation, and >0.9 representing very strong correlation (15). Conditions were then compared to baseline using Deming regression and Bland-Altman bias plots. Since the urinary biomarkers measured under different experimental conditions (including the control condition) may be subject to some degree of measurement variabilities, we took Deming Regression approach, modeling the relationship between each pair of experimental conditions with the control condition. For the Bland-Altman plots, the differences between the experimental vs. control condition for a given urinary biomarker are shown in the y-axis while the corresponding measurement under the control condition is shown in the x-axis. For each urinary biomarker the mean difference, or bias, and the 95% limits of agreement was displayed (16). When the majority of measurements were within the 95% limits of agreement, then a given biomarker was considered stable across the tested experimental conditions. For month 1 and month 3 samples, all urinary biomarkers were repeated four times, so intraclass correlation coefficients (ICC) and coefficients of variation (CV) were calculated. An ICC of <0.5 represents poor reliability, between 0.5 and 0.75 represent moderate reliability and 0.75 to 0.9 indicates good reliability, and >0.9 indicates excellent reliability (17). All statistical analyses were performed using SAS 9.4 (Cary, NC).
Results
Patient urine specimens were obtained in a serial fashion, resulting in 10 urine samples from 10 patients. The average age of the patient population was 19.09 (± 2.51) years. There were 9 females (90%), and the majority were Caucasian (70%), with the remaining African American. Most of the patients (80%) did not have a diagnosis of lupus nephritis, and the two that did have Class II Lupus Nephritis. The urine volumes were sufficient that each collection was able to be run under each experimental collection. MCP-1 had the least number of samples below the lower limit of detection, only 1/220 (0.45%). Adiponectin had 44/220 (20%) and Hemopexin had 25/220 (11.36%) below the lower limit of detection. Ceruloplasmin has samples both above and below the limits of detection, each 44/220 (20%).
Adiponectin
The means of adiponectin under baseline condition was 1.935 ± 1.521, with minimum of −0.486 and maximum of 3.441 from the 10 samples included. As shown in Table 1, the log-transformed means for experimental conditions ranged from 1.626 ± 1.482 (average 15.97% decrease, after 3-month storage) to 2.132 ± 1.476 (under Fridge condition). Spearman correlation coefficients showed duplicates had very good correlation within each sample run, all >0.9, except for room temperature, which was good at 0.888. The Bland-Altman plots (Figure 2) and Deming-Regression (Table 2) show that differences in means for each experimental condition are due to random error. Finally, Table 3 shows ICC for 1 month and 3 months storage conditions, revealing excellent reliability.
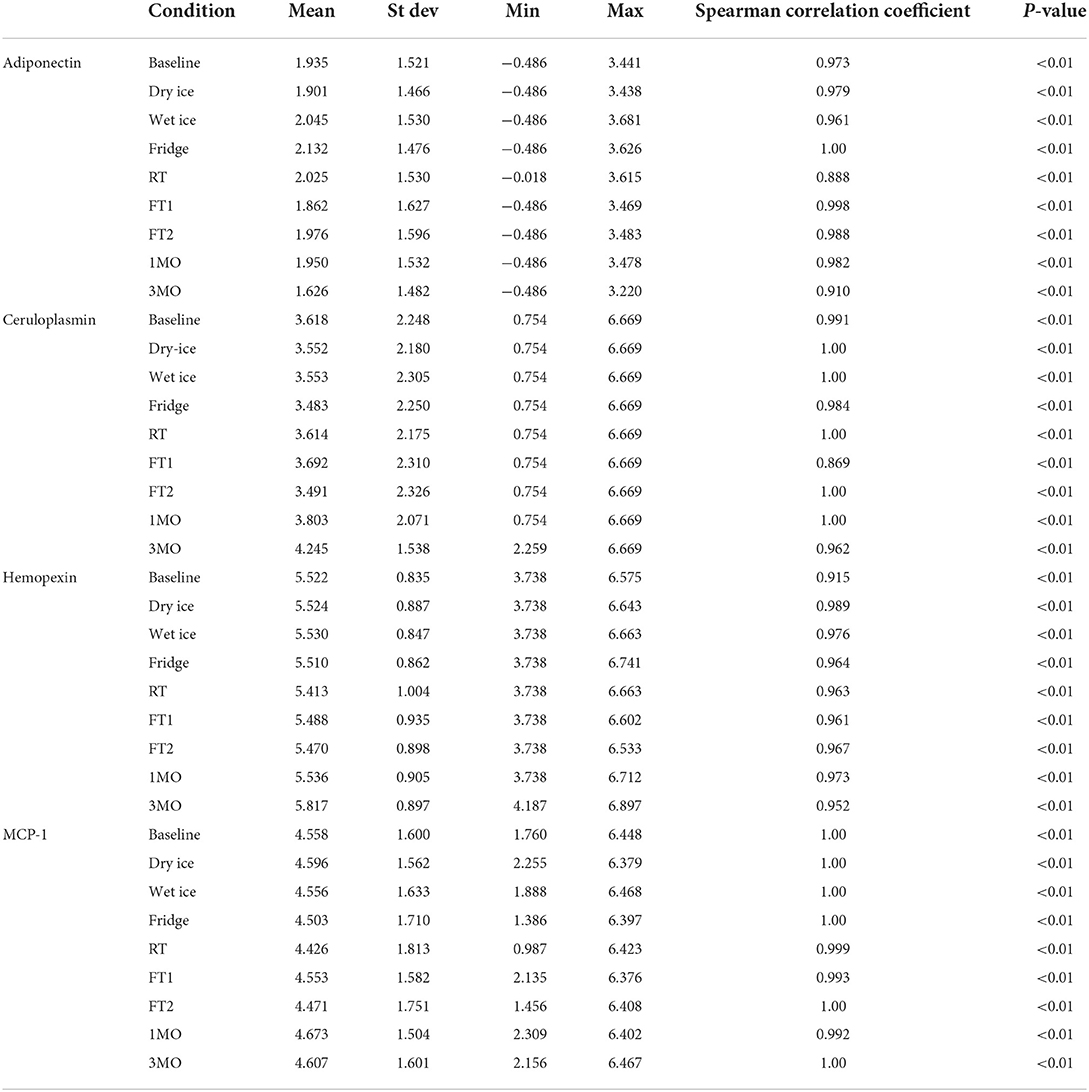
Table 1. Average urinary biomarker concentration under each experimental condition with spearman correlation coefficients.
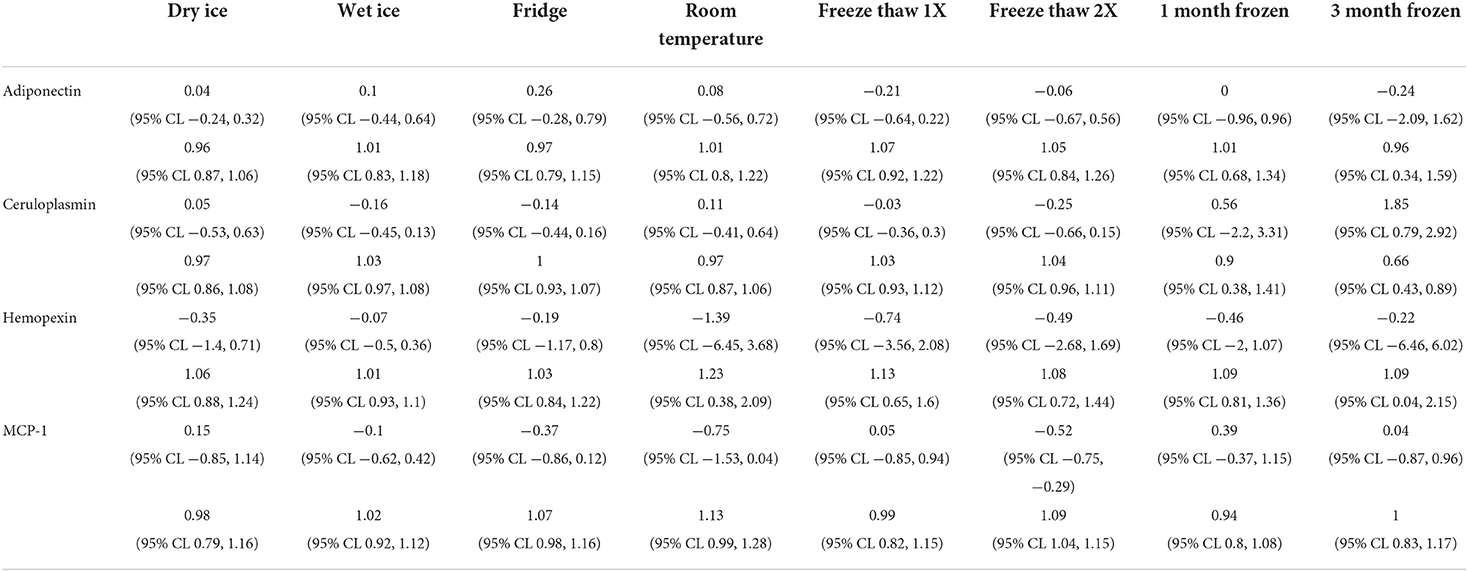
Table 2. Deming-Regression Intercept and beta-coefficient for each biomarker under each experimental condition.
Ceruloplasmin
Likewise, mean for ceruloplasmin under baseline condition was 3.618 ± 2.248, with minimum of 0.754 and maximum of 6.669 from the 10 samples. As shown in Table 1, the log-transformed means for experimental conditions ranged from 3.483 ± 2.25 (average 3.73% decrease, under Fridge condition) to 4.245 ± 1.538 (after 3-month storage). Spearman correlation coefficients showed duplicates had very good correlation within each sample run, all >0.9 apart from the first freeze thaw experiment. The Bland-Altman plots and Deming-Regression are shown in Figure 3 and Table 2 respectively. There was some variability within the lower limits of detection but overall, differences in means for each experimental condition are due to random error. Finally, Table 3 shows ICC for 1 month and 3 months storage conditions, revealing excellent reliability.
Hemopexin
Mean for hemopexin under baseline condition was 5.522 ± 0.835, with minimum of 3.738 and maximum of 6.575 from the 10 samples. As shown in Table 1, the log-transformed means for experimental conditions ranged from 5.413 ± 1.004 (average 1.8% decrease, under Room Temperature) to 5.817 ± 0.897 (after 3-month storage). Spearman correlation coefficients showed duplicates had very good correlation within each sample run, all >0.9. The Bland-Altman plots (Figure 4) and Deming-Regression (Table 2) show that differences in means for each experimental condition are due to random error. Finally, Table 3 shows ICC for 1 month and 3 months storage conditions, revealing excellent reliability.
MCP-1
Mean of MCP-1 was 4.558 ± 1.600, with minimum of 1.760 and maximum of 6.448 from the 10 samples at baseline condition. As shown in Table 1, the log-transformed means for experimental conditions ranged from 4.426 ± 1.813 (average 2.90% decrease, under Room Temperature) to 4.673 ± 1.504 (after 1-month storage). Spearman correlation coefficients showed duplicates had very good correlation within each sample run, all >0.9. The Bland-Altman plots (Figure 5) and Deming-Regression (Table 2) show that differences in means for each experimental condition are due to random error. Finally, Table 3 shows ICC for 1 month and 3 months storage conditions, revealing excellent reliability.
Discussion
In this study, we meaningfully add to the growing literature on using urinary biomarkers for LN evaluation. Exploration of stability is important in anticipation to move the RAIL into the clinical realm and consider use of the RAIL biomarkers in clinical trials.
We have previously shown the stability of NGAL and KIM-1 under different temperatures, freeze/thaw conditions and long-term storage (13). We now add four additional biomarkers of importance to lupus nephritis research: MCP-1, Adiponectin, Hemopexin and Ceruloplasmin. We tested effect of temperature, freeze/thaw, shipping, and storage for months, without significant differences between baseline and the experimental conditions. Importantly, we provide new data on the stability of urinary adiponectin, hemopexin, and ceruloplasmin that has not previously been reported.
We showed that the four other biomarkers included in the RAIL are stable at room temperature and 4 °C for up to 48 h. This has been replicated for other biomarkers as well. Wang et al. (18) evaluated effect of various temperature (4 °C, 22 °C, 40 °C) and short-term storage (24 h, 48 h) on the metabolite signature in urine. The control used was −20 °C. As in this study, they also found stability of the metabolite signature at 4 °C for 24 and 48 h, as well 22 °C for 24 h, however not 48 h. There was no integrity of the metabolite signature at 40 °C for any length of time. The effect of temperature on urinary KIM-1 and NGAL have been studied in various other studies (19, 20). Both proteins have been shown to be stable at 4 °C at 48 h as well as 25 °C at 48 h. NGAL has further been shown to be stable for up to 7 days at room temperature, 4 °C and −20 °C, which is not the case with KIM-1 (21, 22). Chang et al. (20), besides studying KIM-1 and NGAL, as well as other biomarkers, also evaluated MCP-1, and has shown it to be stable after 48 h at 4 °C, 48 h at 25 °C, no centrifugation but immediate aliquot and storage at −80 °C.
The data on longer-term storage are somewhat conflicting. Pennemans et al. (23) evaluated KIM-1 and showed good recovery at −80 °C for up to 1.5 years, but a bigger decline in biomarker concentration with storage at −20 °C. De Vrie et al. (22) evaluated KIM-1 and NGAL at −80 °C for 6 months, with both markers showing stability. Herrington et al. (24) showed urine albumin preservation for up to 6 months at −80 °C or −40 °C, but not −20 °C. In contrast, several authors have shown more variability in biomarker stability, even at −80 °C. Nauta et al. (25) evaluated multiple markers, including KIM-1, NGAL at 1 year in −20 °C or −80 °C. All markers except urine cystatin C showed a gradual decline in concentration, which seemed to stabilize after 6 months. Liu et al. (26) compared storage at −70 °C. KIM-1, NGAL, IL-18, liver-type fatty acid-binding protein (L-FABP) in hospitalized and non-hospitalized patients, where processing could be up to 6 h. Median duration of storage was 17.8 months for inpatient, and 14.6 months for outpatient samples. For NGAL, IL-18, L-FABP, storage time was not significantly associated with biomarker levels. KIM-1 levels were lower with longer storage times in the outpatient group, but this was finding was not found with the inpatient group.
The effect of freeze thaw cycles was studied for urine NGAL and KIM-1 previously. We newly evaluated the effects of freeze thaws on hemopexin, adiponectin, MCP-1 and ceruloplasmin concentrations. Hogan et al. (21) showed that NGAL was stable for up to 3 freeze/thaw cycles at −20 °C. Further, Herrington et al. (24) showed that urine albumin is preserved after 3 freeze/thaw cycles. These reports are similar to this study, with storage temperature of −80 °C. Pennemans et al. (23) performed a study in KIM-1, comparing the difference between 3 h and 24-h thaw period compared to 1 h, with optimal thawing time being 1 h.
Our study is the first to show the stability of biomarkers under study shipping conditions, both under dry ice and with wet ice. This has important implications for the measurement of these biomarkers by select clinical laboratories in stored urine samples from patients and from previous clinical trials.
One limitation with this study may be the small sample size, including of patients with lupus nephritis, which may reduce its generalizability. While most of the biomarkers were within the detectable range of the assay kits, ceruloplasmin had percentages of samples both above and below outside range of detection on assay. However, the amount below the detectable limit was reasonable, and the amount above detection that did not show large amount of decay.
In conclusion, our study demonstrates stability of hemopexin, ceruloplasmin, MCP-1 and adiponectin under different conditions that are important for clinical and experimental use, including up 48 h at room temperature, 4 °C, as well as up to 3 months at −80 °C storage. Importantly, we provide new data that these biomarkers are stable under storage with both wet and dry ice. With rising use of biomarkers for both clinical use and research, these results are reassuring that shipping, as well as long term storage and multiple freeze/thaw cycles can be employed prior to batch measurement.
Data availability statement
The original contributions presented in the study are included in the article/supplementary material, further inquiries can be directed to the corresponding author.
Ethics statement
The studies involving human participants were reviewed and approved by Cincinnati Children's Hospital IRB (IRB CCHMC-2008-0635). Written informed consent to participate in this study was provided by the participants' legal guardian/next of kin.
Author contributions
Research idea and study design: EC, HB, and PD. Data acquisition: JR. Data analysis and interpretation: BH, EC, HB, and PD. Statistical analysis: BH and TQ. Supervision or mentorship: HB and PD. All authors contributed to the article and approved the submitted version.
Funding
This study was supported by the Lupus Foundation of America, Falk Foundation, and Portico (P30AR076316). PD was supported by a grant from the NIH (P50DK096418).
Acknowledgments
We thank Catherine Robben, Megan Quinlan-Waters and Rebecca Hopkins for CRC and lab support.
Conflict of interest
Author PD is a co-inventor on submitted patents for the use of NGAL as a biomarker of kidney disease.
The remaining authors declare that the research was conducted in the absence of any commercial or financial relationships that could be construed as a potential conflict of interest.
Publisher's note
All claims expressed in this article are solely those of the authors and do not necessarily represent those of their affiliated organizations, or those of the publisher, the editors and the reviewers. Any product that may be evaluated in this article, or claim that may be made by its manufacturer, is not guaranteed or endorsed by the publisher.
References
1. Parikh SV, Almaani S, Brodsky S, Rovin BH. Update on lupus nephritis: core curriculum 2020. Am J Kidney Dis. (2020) 76:265–81. doi: 10.1053/j.ajkd.2019.10.017
2. Almaani S, Meara A, Rovin BH. Update on lupus nephritis. Clin J Am Soc Nephrol. (2017) 12:825–35. doi: 10.2215/CJN.05780616
3. Silva CA, Avcin T, Brunner HI. Taxonomy for systemic lupus erythematosus with onset before adulthood. Arthritis Care Res. (2012) 64:1787–93. doi: 10.1002/acr.21757
4. Aggarwal A, Srivastava P. Childhood onset systemic lupus erythematosus: how is it different from adult SLE? Int J Rheum Dis. (2015) 18:182–91. doi: 10.1111/1756-185X.12419
5. Borgia RE, Silverman ED. Childhood-onset systemic lupus erythematosus: an update. Curr Opin Rheumatol. (2015) 27:483–92. doi: 10.1097/BOR.0000000000000208
6. Malvar A, Pirruccio P, Alberton V, Lococo B, Recalde C, Fazini B, et al. Histologic vs. clinical remission in proliferative lupus nephritis. Nephrol Dial Transplant. (2017) 32:1338–44. doi: 10.1093/ndt/gfv296
7. Chen L, Tan M, Huang J, Wen S, Cheng C, Liu Y, et al. Effects of alternative remission criteria on outcome of pediatric proliferative lupus nephritis: a multi-center retrospective study of pediatric proliferative lupus nephritis. Am J Transl Res. (2021) 13:4510–20.
8. Rovin BH, Zhang X. Biomarkers for lupus nephritis: the quest continues. Clin J Am Soc Nephrol. (2009) 4:1858–65. doi: 10.2215/CJN.03530509
9. Mina R, Abulaban K, Klein-Gitelman MS, Eberhard BA, Ardoin SP, Singer N, et al. Validation of the lupus nephritis clinical indices in childhood-onset systemic lupus erythematosus. Arthritis Care Res. (2016) 68:195–202. doi: 10.1002/acr.22651
10. Brunner HI, Bennett MR, Gulati G, Abulaban K, Klein-Gitelman MS, Ardoin SP, et al. Urine biomarkers to predict response to lupus nephritis therapy in children and young adults. J Rheumatol. (2017) 44:1239–48. doi: 10.3899/jrheum.161128
11. Brunner HI, Bennett MR, Abulaban K, Klein-Gitelman MS, O'Neil KM, Tucker L, et al. Development of a novel renal activity index of lupus nephritis in children and young adults. Arthritis Care Res. (2016) 68:1003–11. doi: 10.1002/acr.22762
12. Gulati G, Bennett MR, Abulaban K, Song H, Zhang X, Ma Q, et al. Prospective validation of a novel renal activity index of lupus nephritis. Lupus. (2017) 26:927–36. doi: 10.1177/0961203316684212
13. Schuh MP, Nehus E, Ma Q, Haffner C, Bennett M, Krawczeski CD, et al. Long-term stability of urinary biomarkers of acute kidney injury in children. Am J Kidney Dis. (2016) 67:56–61. doi: 10.1053/j.ajkd.2015.04.040
14. Aringer M. EULAR/ACR classification criteria for SLE. Semin Arthritis Rheum. (2019) 49:S14–7. doi: 10.1016/j.semarthrit.2019.09.009
15. Schober P, Boer C, Schwarte LA. Correlation coefficients: appropriate use and interpretation. Anesth Analg. (2018) 126:1763–8. doi: 10.1213/ANE.0000000000002864
16. Chhapola V, Kanwal SK, Brar R. Reporting standards for Bland-Altman agreement analysis in laboratory research: a cross-sectional survey of current practice. Ann Clin Biochem. (2015) 52:382–6. doi: 10.1177/0004563214553438
17. Terry K, Koo MYL. A guideline of selecting and reporting intraclass correlation coefficients for reliability research. J Chiropr Med. (2016) 15:155–63. doi: 10.1016/j.jcm.2016.02.012
18. Wang X, Gu H, Palma-Duran SA, Fierro A, Jasbi P, Shi X, et al. Influence of storage conditions and preservatives on metabolite fingerprints in urine. Metabolites. (2019) 9:203. doi: 10.3390/metabo9100203
19. Parikh CR, Butrymowicz I, Yu A, Chinchilli VM, Park M, Hsu CY, et al. Urine stability studies for novel biomarkers of acute kidney injury. Am J Kidney Dis. (2014) 63:567–72. doi: 10.1053/j.ajkd.2013.09.013
20. Chang C, Obeid W, Thiessen-Philbrook H, Parikh CR. Sample processing and stability for urine biomarker studies. J Appl Lab Med. (2021) 6:1628–34. doi: 10.1093/jalm/jfab082
21. Hogan MC, Lieske JC, Lienczewski CC, Nesbitt LL, Wickman LT, Heyer CM, et al. Strategy and rationale for urine collection protocols employed in the NEPTUNE study. BMC Nephrol. (2015) 16:190. doi: 10.1186/s12882-015-0185-3
22. van de Vrie M, Deegens JK, van der Vlag J, Hilbrands LB. Effect of long-term storage of urine samples on measurement of kidney injury molecule 1 (KIM-1) and neutrophil gelatinase-associated lipocalin (NGAL). Am J Kidney Dis. (2014) 63:573–6. doi: 10.1053/j.ajkd.2013.10.010
23. Pennemans V, Rigo JM, Penders J, Swennen Q. Collection and storage requirements for urinary kidney injury molecule-1 (KIM-1) measurements in humans. Clin Chem Lab Med. (2011) 50:539–43. doi: 10.1515/cclm.2011.796
24. Herrington W, Illingworth N, Staplin N, Kumar A, Storey B, Hrusecka R, et al. Effect of processing delay and storage conditions on urine albumin-to-creatinine ratio. Clin J Am Soc Nephrol. (2016) 11:1794–801. doi: 10.2215/CJN.13341215
25. Nauta FL, Bakker SJ, Lambers Heerspink H, de Zeeuw D, van Oeveren W, Bilo H, et al. Effect of frozen storage on urinary concentration of kidney damage markers. Am J Kidney Dis. (2012) 59:586–9. doi: 10.1053/j.ajkd.2011.11.025
Keywords: lupus nephritis, urine, biomarker, stability, SLE
Citation: Cody EM, Rose JE, Huang B, Qiu T, Brunner HI and Devarajan P (2022) Stability of novel urinary biomarkers used for lupus nephritis. Front. Pediatr. 10:974049. doi: 10.3389/fped.2022.974049
Received: 20 June 2022; Accepted: 11 July 2022;
Published: 29 July 2022.
Edited by:
David Selewski, Medical University of South Carolina, United StatesReviewed by:
Aftab S. Chishti, University of Kentucky, United StatesBrendan Crawford, University of Arkansas for Medical Sciences, United States
Copyright © 2022 Cody, Rose, Huang, Qiu, Brunner and Devarajan. This is an open-access article distributed under the terms of the Creative Commons Attribution License (CC BY). The use, distribution or reproduction in other forums is permitted, provided the original author(s) and the copyright owner(s) are credited and that the original publication in this journal is cited, in accordance with accepted academic practice. No use, distribution or reproduction is permitted which does not comply with these terms.
*Correspondence: Ellen M. Cody, ZW1jb2R5MTMmI3gwMDA0MDtnbWFpbC5jb20=