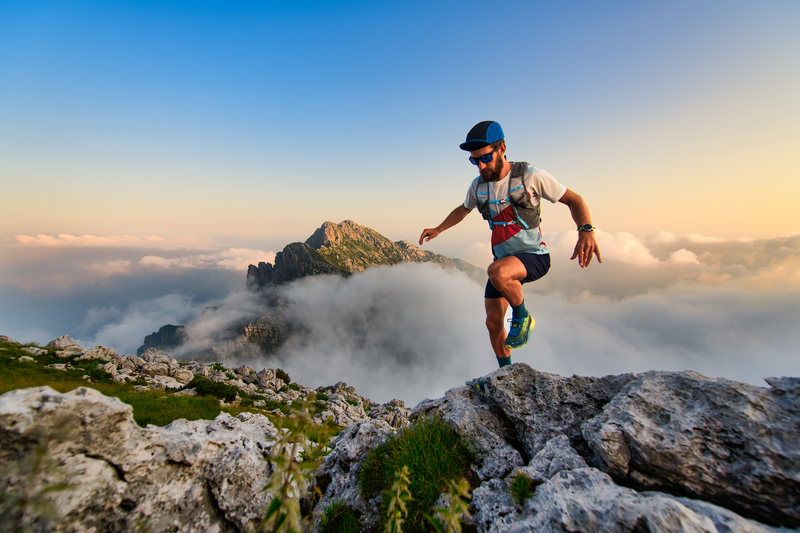
94% of researchers rate our articles as excellent or good
Learn more about the work of our research integrity team to safeguard the quality of each article we publish.
Find out more
ORIGINAL RESEARCH article
Front. Pediatr. , 14 September 2022
Sec. Neonatology
Volume 10 - 2022 | https://doi.org/10.3389/fped.2022.924014
Aim: To construct a prediction model based on the data of premature infants and to apply the data in our study as external validation to the prediction model proposed by Yuejun Huang et al. to evaluate the predictive ability of both models.
Methods: In total, 397 premature infants were randomly divided into the training set (n = 278) and the testing set (n = 119). Univariate and multivariate logistic analyses were applied to identify potential predictors, and the prediction model was constructed based on the predictors. The area under the curve (AUC) value, the receiver operator characteristic (ROC) curves, and the calibration curves were used to evaluate the predictive performances of prediction models. The data in our study were used in the prediction model proposed by Yuejun Huang et al. as external validation.
Results: In the current study, endotracheal intubation [odds ratio (OR) = 10.553, 95% confidence interval (CI): 4.959–22.458], mechanical ventilation (OR = 10.243, 95% CI: 4.811–21.806), asphyxia (OR = 2.614, 95% CI: 1.536–4.447), and antibiotics use (OR = 3.362, 95% CI: 1.454–7.775) were risk factors for late-onset sepsis in preterm infants. The higher birth weight of infants (OR = 0.312, 95% CI: 0.165–0.588) and gestational age were protective factors for late-onset sepsis in preterm infants. The training set was applied for the construction of the models, and the testing set was used to test the diagnostic efficiency of the model. The AUC values of the prediction model were 0.760 in the training set and 0.796 in the testing set.
Conclusion: The prediction model showed a good predictive ability for late-onset sepsis in preterm infants.
Neonatal sepsis is a clinical syndrome caused by pathogenic bacteria, and can invade the blood circulation, affect the growth, and attack the reproduction system of neonates during the 1st month of life, which is characterized by hemodynamic changes and other systemic symptoms of infection (1). Neonatal sepsis may result in organ failure and tissue damage, which is a vital reason for neurocognitive sequelae and neonatal mortality (2, 3). According to the age of onset, neonatal sepsis is divided into early-onset sepsis and late-onset sepsis. Early-onset sepsis reflects transplacental or, more frequently, ascending infections from the maternal genital tract, whereas late-onset sepsis is associated with the postnatal nosocomial or community environment, with the peak incidence reported to be between the 10th and 22nd day of life (4). Patients with late-onset sepsis have a poor prognosis with a prolonged hospital stay, and 18% of mortality in neonates has been caused by late-onset sepsis (5). Early symptoms of neonatal late-onset sepsis are often atypical, and septic shock often occurs due to delayed diagnosis (6). Effective assessment of the risk of sepsis in preterm infants is essential for pediatricians to make early interventions to reduce neonatal mortality.
Increasing evidence indicates that multiple factors affect late-onset sepsis in premature infants. According to the National Institute of Child Health and Human Development, the incidence of infection in premature infants increased with a decrease in birth weight (7). Low levels of thyroid function indexes, such as triiodothyronine (T3) and thyroxine (T4), have also been reported to be associated with the occurrence of sepsis in premature infants (8, 9).
Previously, Yuejun Huang et al. established a prediction model for late-onset sepsis in premature infants based on predictors, such as birth weight, endotracheal intubation, time of umbilical venous catheterization (UVC), and thyroid function (10). However, the model was constructed based on the data of newborns from Guangdong province, located in the southern part of China, and whether the prediction model was suitable for predicting late-onset sepsis in newborns from other regions in China was still elusive. In addition, the prediction model lacked external data to validate the results.
In the present study, we constructed a prediction model based on the data of premature infants with or without late-onset sepsis in Affiliated Hangzhou First People's Hospital, Zhejiang University School of Medicine, and the data in our study were applied as external validation to evaluate the predictive performance of the prediction model proposed by Yuejun Huang et al.
The current case-control study collected the data of 397 premature infants recruited from Affiliated Hangzhou First People's Hospital, Zhejiang University School of Medicine between June 2009 and June 2020. The study included infants with a gestational age at birth of <37 weeks who were admitted to the neonatal department within 24 h of birth and stayed in the ward for more than 7 days. Newborns whose mothers had thyroid, liver, kidney, lung, or heart diseases before pregnancy were excluded. Premature infants with congenital malformations or those who were on admission for <7 days were also excluded from the study. All premature infants were randomly divided into the training set (n = 278) and the testing set (n = 119) at a ratio of 7:3. Informed consent was obtained from the parents of the participants, and this study was approved by the Ethics Committee of Affiliated Hangzhou First People's Hospital, Zhejiang University School of Medicine (No. 202102051224000400219).
The data of main variables of the infants were collected, which included the gender of infants, etiological status (no infection or bacterial infection), endotracheal intubation (yes or no), UVC (yes or no), a peripherally inserted central catheter (yes or no), mechanical ventilation (yes or no), dopamine use (yes or no), albumin use (yes or no), asphyxia (yes or no), antibiotic use (yes or no), thyroid hypofunction (yes or no), birth weight (kg), and gestational age (24 to <28 weeks, 28 to <32 weeks, or 32 to <37 weeks). The clinical data of the mothers were also collected, such as age (years), prenatal glucocorticoid use (yes or no), prenatal antibiotic use (yes or no), premature rupture of fetal membranes (yes or no), the method of delivery (cesarean delivery or vaginal delivery), the season of delivery (spring, summer, autumn, or winter), thyroid stimulating hormone (TSH, μIU/ml), free T4 (FT4, pmol/L), free T3 (FT3, pmol/L), T4 (nmol/L), and T3 (nmol/L).
Thyroid function was measured with a chemiluminescence kit (Beckman Coulter, Prague, Czech Republic) using blood samples of preterm infants taken in the morning between 4 and 7 days after birth. High TSH refers to a TSH >10 mIU/L (11). In addition, T3, T4, FT3, and FT4 were corrected using either the 10th percentile or −1 SD as the cut-off value to compensate for the change in TH levels due to gestational age (12, 13). Thyroid hypofunction is defined based on the transient hypothyroxinemia of prematurity (low T4 with normal TSH), congenital hypothyroidism (low T4 with elevated TSH), low serum T3, and high TSH.
The outcome was late-onset sepsis in premature infants. The diagnosis of late-onset sepsis in premature infants was based on the Guidelines for the Diagnosis and Treatment of Neonatal Sepsis according to the signs and symptoms as well as laboratory examinations (14). The signs and symptoms, such as unstable temperature, hypotension, poor perfusion with pallor, bradycardia, tachycardia, cyanosis, apnea, lethargy, irritability, seizures, jaundice, abdominal distention, and petechiae, were regarded as suggestive sepsis (15). The blood count, C-reactive protein (CRP), procalcitonin (PCT), and the cultures from blood and other sterile sites were collected to identify bacterial infection. Culture-based diagnostics for late-onset bacterial sepsis was that the infant manifested signs and symptoms of infection after 7 days of age and in line with any of the following: (1) a blood or cerebrospinal fluid (CSF) culture positive for a pathogenic bacterial species, and (2) if the cultures from blood showed conditional pathogenic bacteria (e.g., coagulase-negative staphylococci), the infant was considered to be infected based on the results from other laboratory parameters of infection, such as (1) white blood cell (WBC) count <5 × 109/L or an increased WBC count (age > 3 days, WBC >20 × 109/L); (2) immature neutrophils/total neutrophils ≥0.16; (3) platelet count <100 × 109/L; and (4) C-reactive protein levels ≥8 mg/L (15).
The continuous variables with normal distribution were displayed as mean ± standard deviation (mean ± SD) and comparisons between groups were subjected to the t-test. The continuous variables with non-normal distribution were described by M (Q1, Q3), and the differences between groups were compared by the Wilcoxon rank-sum test. The categorical variables were expressed as n (%), and a chi-square test was used for comparing the differences between the groups. Logistic analyses were applied to identify potential predictors, and the prediction model was constructed based on the predictors. After collinearity diagnosis by multivariate logistic regression, predictors with strong collinearity [variance inflation factor (VIF) refers to a relative measure of variance increase caused by the collinearity of independent variables in the estimator of the regression coefficient. VIF > 10 indicates that there is a strong collinearity problem] (16) were excluded in the model. Herein, 70% of the samples were involved as the training set for constructing the model, and 30% of the samples were used as the testing set to test the diagnostic efficiency of the model. The data in our study were applied into the prediction model proposed by Huang et al. (10) as external validation to evaluate its predictive ability. The receiver operator characteristic (ROC) curves and the calibration curves were used to evaluate the differentiation and consistency of the prediction models. The baseline data were analyzed via SAS 9.4 statistical analysis software and the ROC curves and the calibration curves were plotted by Python. The value of p < 0.05 was considered statistically significant.
This study recruited 397 premature infants who were randomly divided into the training set (n = 278) and the testing set (n = 119). The detailed characteristics of the patients in the training set and the testing set are exhibited in Table 1. In the training set, 138 premature infants were diagnosed to have late-onset sepsis, accounting for 49.64%. In this study, 140 (50.36%) premature infants were boys and 138 (49.64%) were girls. Furthermore, two hundred and eleven infants did not receive endotracheal intubation, accounting for 75.90% of the participants, and 67 received endotracheal intubation, accounting for 24.10% of the participants. Additionally, 188 patients received UVC, accounting for 66.19% of the participants. In total, 103 (37.05%) infants underwent peripheral central catheter insertion. There were 66 (23.74%) patients who received mechanical ventilation. Dopamine was used in 7 (2.52%) patients and albumin was used in 61 (21.94%) patients. Neonatal asphyxia occurred in 85 infants, accounting for 30.58%. In addition, two hundred and forty-six (88.49%) infants received antibiotics. The mean weight of the infants was 1.54 ± 0.41 kg. As for the baseline data of the pregnant mother, the mean age was 31.27 ± 4.86 years old (Table 2).
Table 2. Comparisons of characteristics between the control group and the case group in the training set.
As exhibited in Table 3, compared with patients without endotracheal intubation, the risk of late-onset sepsis in premature infants was 10.553-fold higher in patients with endotracheal intubation [odds ratio (OR) = 10.553, 95% confidence interval (CI): 4.959–22.458]. Mechanical ventilation treatment increased the risk of late-onset sepsis in premature infants (OR = 10.243, 95% CI: 4.811–21.806). Asphyxia increased the risk of late-onset sepsis by 2.614-fold when compared with infants without asphyxia (OR = 2.614, 95% CI: 1.536–4.447). Relative to infants without antibiotics use, the risk of late-onset sepsis was increased by 3.362-fold in those with antibiotic use (OR = 3.362, 95% CI: 1.454–7.775). Increased birth weight of infants was associated with a decreased risk of late-onset sepsis (OR = 0.312, 95% CI: 0.165–0.588). Compared with infants delivered at a gestational age of 24 to <28 weeks, the risk of late-onset sepsis decreased by 0.409-fold in infants delivered at a gestational age of 28 to <32 weeks (OR) and further by 0.282-fold in infants delivered at a gestational age of 32 to <37 weeks (OR = 0.282, 95% CI: 0.123–0.649).
The prediction model for late-onset sepsis in premature infants was constructed based on the predictors identified via univariate analysis. The results of multicollinearity analysis revealed that endotracheal intubation (VIF = 54.49320) and mechanical ventilation (VIF = 53.36468) indicated that they had strong collinearity with other variables (Supplementary Table 1). Finally, the prediction model was ln ()= 4.927 + 0.346 (Male) – 0.299 (28w-32w gestational age) – 0.871 (32w−37w gestational age) + 0.974 (antibiotic use) + 0.698 (asphyxia) – 1.894 (birth weight) + 0.266 (albumin use) – 1.586 (prenatal glucocorticoid use) – 1.217 (umbilical vein catheterization) + 0.135 (premature rupture of fetal membranes) – 0.410 (peripherally inserted central catheter) – 0.027 (age of mother) + 0.368 (dopamine use) + 0.356 Summer + 0.524 Spring + 0.978 Winter (Table 4).
As seen in Figure 1, in the training set, the AUC was 0.760 (95% CI: 0.704–0.816), the accuracy was 0.719 (0.663–0.771), the sensitivity was 0.645 (0.565–0.725), the specificity was 0.793 (0.726–0.860), the negative predictive value (NPV) was 0.694 (0.622–0.765), and the positive predictive value (PPV) was 0.754 (0.677–0.832) (Table 5). In the testing set, the AUC was 0.796 (95% CI: 0.715–0.877) (Figure 2). The cutoff value was 0.539. The accuracy was 0.714 (95% CI: 0.624–0.793), the sensitivity was 0.678 (95% CI: 0.559–0.793), the specificity was 0.750 (95% CI: 0.640–0.860), the NPV was 0.703 (95% CI: 0.591–0.815), and the PPV was 0.727 (95% CI: 0.610–0.845) (Table 5). The nomogram was plotted based on the prediction model (Figure 3). A sample was randomly selected from the training set to validate the performance of the prediction model. The baseline data of the sample were a male infant with a gestational age at 24 to <28 weeks, the use of albumin, and asphyxia. The birth weight of the infant was 1.9 kg. The infant did not use UVC, peripheral catheterization into the central vein, or dopamine and was born in summer. The mother was 33 years old and used prenatal glucocorticoid. The total score of this infant was 862, and the possibility of late-onset sepsis was 0.5908 (Figure 4). The predicted result was similar to the actual result, and the infant had late-onset sepsis, indicating that the predictive ability of the model was good.
Figure 1. The receiver operator characteristic (ROC) curve showed the predictive ability of the prediction model in the training set in our model and Yuejun Huang et al.'s model.
Figure 2. The ROC curve showed the predictive ability of the prediction model in the testing set in our model and Yuejun Huang et al.'s model.
Figure 4. A sample was randomly selected from the training set to validate the performance of the prediction model.
The prediction model from Huang et al. (10) was applied in our data. The prediction model of Yuejun Huang et al. was ln () = −1.995 (birth weight) + 0.297 (umbilical vein catheterization) + 1.648 (endotracheal intubation) + 1.407 (thyroid hypofunction) + 0.024 (age of mother) + 0.029 (delivery mode) - 0.143 (gestational age) + 0.053 asphyxia – 0.033 (peripherally inserted central catheter) + 0.037 (mechanical ventilation). In the training set, the AUC was 0.707 (95% CI: 0.646–0.768) (Figure 1), the accuracy was 0.680 (95% CI: 0.622–0.734), the sensitivity was 0.471 (0.386–0.558), the specificity was 0.886 (95% CI: 0.821–0.933), the NPV was 0.629 (95% CI: 0.558–0.697), and the PPV was 0.802 (95% CI: 0.699–0.883). In the testing set, the AUC was 0.612 (95% CI: 0.510–0.715) (Figure 2), the accuracy was 0.580 (95% CI: 0.486–0.670), the sensitivity was 0.441 (95% CI: 0.312–0.576), the specificity was 0.717 (95% CI: 0.586–0.825), the NPV was 0.566 (95% CI: 0.447–0.679), and the PPV was 0.605 (95% CI: 0.444–0.750) in the testing set (Table 5). The AUC in the testing set of our model was statistically higher than the AUC in the testing set using the model by Huang et al. (10) (p = 0.006). The calibration curves of the training set and the testing set were respectively plotted, and it revealed that our prediction model had a better predictive value (Figure 5).
In the present study, the data of 397 premature infants were analyzed to identify the predictors of late-onset sepsis in those patients and established a prediction model for late-onset sepsis in premature infants. The results delineated that endotracheal intubation, mechanical ventilation treatment, neonatal asphyxia, antibiotics use, birth weight, and gestational age were predictors associated with the risk of late-onset sepsis in premature infants. The prediction model was constructed based on predictors, and the predictive performance was good in the training set and the testing set. Additionally, our data were applied to the prediction model from Huang et al. (10), and the results depicted that the predictive ability of our prediction model might be better than the prediction model from Yuejun Huang et al. (10).
Endotracheal intubation is a vital intervention for the stabilization of critically ill infants (17). As an invasive medical operation, endotracheal intubation can cause damage to the upper respiratory mucosa and destroy the natural immune barrier of the body (18). Prolonged endotracheal intubation can also change the bacterial colonization of the respiratory tract, which might increase the incidence of sepsis (19). This supported the results of our study, which revealed that the use of endotracheal intubation was an independent risk factor for late-onset sepsis in preterm infants. According to the study by Manandhar S et al., each additional day of mechanical ventilation application increased the risk of sepsis by 0.086 times for patients in a neonatal intensive care unit (20). The association between mechanical ventilation use and the risk of late-onset sepsis was also identified by Yapicioglu et al. (21). These studies gave support to the findings in the current study. Infants with asphyxia also had a higher risk of late-onset sepsis in the current study. Perinatal asphyxia is one of the main causes of neonatal deaths all through the world (22). Perinatal asphyxia might result in the disorder of thyroid function and might lead to low levels of thyroid hormones in newborns, and thyroid hormones are essential participants in regulating the innate immune system (9, 23). Low thyroid hormones levels were reported to increase the risk of bacterial sepsis and associated with the poor prognosis of newborns and young children (24, 25). Antibiotics are the most commonly applied treatment for suspected sepsis in neonatal intensive care units because of the diagnostic challenges of sepsis and the relative immunosuppression of newborns (26). The use of antimicrobial agents, such as antibiotics, is reported to be a risk factor for multidrug-resistant bacterial infections, which may lead to sepsis (27). This provided evidence to the results of this study, showing that the use of antibiotics was a risk factor for late-onset sepsis in preterm infants. This serves to remind the pediatricians to use antibiotics with caution in preterm infants. According to a report from the Turkish Nosocomial Infections Study Group, the frequency of sepsis was increased by 22% in infants with a birth weight of <1,500 g, 6% in infants with a birth weight of 1,500–2,500 g, and 3% in infants with a birth weight of ≥2,500 g, demonstrating that the risk of sepsis was increased with the decrease in birth weight of the newborns (28). This provided evidence to the findings in the present study, uncovering that increased birth weight of infants was associated with a decreased risk of late-onset sepsis. A systematic review and meta-analysis conducted in India analyzed risk factors for neonatal sepsis and found that neonates with a gestational age of <37 weeks have a higher risk of sepsis (29). Similarly, we found that there might be a negative association between the risk of late-onset sepsis and the gestation age in preterm infants. This suggests that preterm infants with low birth weight and small for gestation age should receive timely interventions to prevent the occurrence of late-onset sepsis.
In this study, factors associated with the risk of late-onset sepsis in preterm patients were assessed, and a prediction model was constructed based on predictors in the training set. The validation was conducted in the testing set. ROC curves were drawn to present the diagnostic ability of the prediction model. The AUC was 0.760 in the training set and 0.796 in the testing set, implying the good predictive performance of the model. A nomogram for predicting the risk of late-onset sepsis in preterm patients was also plotted, which allowed us to easily calculate the score directly from the graph and obtain the possibility of late-onset sepsis in preterm patients. An actual case in the training set was randomly chosen to validate the predictive ability of our model, and the predicted results were similar to the actual outcome of the case. Moreover, the external validation of the previous prediction model for late-onset sepsis in preterm patients proposed by Huang et al. (10) was performed using our data, and it showed that the AUC was 0.707 in the training set and 0.612 in the testing set. All these findings indicated that the prediction model in our study had a good predictive value for late-onset sepsis in preterm patients, which might help identify patients who have a high risk of late-onset sepsis and provide timely intervention to prevent the occurrence of late-onset sepsis in those patients. The nomogram was platted, which we believe is easy for pediatricians to use to calculate the possibility of infants having late-onset sepsis. The predictors were common information and easy to be collected clinically. The prediction model can guide evaluation and treatment decisions.
Several limitations exist in the current study. First, the sample size was small, which might have decreased the statistical power of our results. Second, the prediction model lacks external validation in other data. Third, important variables, such as the status of inflammation in the chorionic plate, were not included in this study. In the future, more well-designed studies with larger sample sizes or/and different populations are required to verify the findings of our study.
The predictors of late-onset sepsis in preterm infants were analyzed, and a prediction model was established based on the predictors, which presented a good predictive ability. The findings of our study might offer a reference to identify preterm infants with a high risk of late-onset sepsis and provide timely treatments to reduce the occurrence of late-onset sepsis in those patients.
The raw data supporting the conclusions of this article will be made available by the authors, without undue reservation.
The studies involving human participants were reviewed and approved by Affiliated Hangzhou First People's Hospital, Zhejiang University School of Medicine (No. 202102051224000400219). Written informed consent to participate in this study was provided by the participants' legal guardian/next of kin.
XS: designed the study and wrote the manuscript. XS, XL, and YW: collected, analyzed, and interpreted the data. XL and YW: critically reviewed, edited, and approved the manuscript. All authors read and approved the final manuscript.
The authors declare that the research was conducted in the absence of any commercial or financial relationships that could be construed as a potential conflict of interest.
All claims expressed in this article are solely those of the authors and do not necessarily represent those of their affiliated organizations, or those of the publisher, the editors and the reviewers. Any product that may be evaluated in this article, or claim that may be made by its manufacturer, is not guaranteed or endorsed by the publisher.
The Supplementary Material for this article can be found online at: https://www.frontiersin.org/articles/10.3389/fped.2022.924014/full#supplementary-material
1. Balayan S, Chauhan N, Chandra R, Kuchhal NK, Jain U. Recent advances in developing biosensing based platforms for neonatal sepsis. Biosens Bioelectron. (2020) 169:112552. doi: 10.1016/j.bios.2020.112552
2. Hentges CR, Silveira RC, Procianoy RS, Carvalho CG, Filipouski GR, Fuentefria RN, et al. Association of late-onset neonatal sepsis with late neurodevelopment in the first two years of life of preterm infants with very low birth weight. J Pediatr. (2013) 90:50–7. doi: 10.1016/j.jped.2013.10.002
3. Liang LD, Kotadia N, English L, Kissoon N, Ansermino JM, Kabakyenga J, et al. Predictors of mortality in neonates and infants hospitalized with sepsis or serious infections in developing countries: a systematic review. Front Pediatr. (2018) 6:277. doi: 10.3389/fped.2018.00277
4. Dong Y, Speer CP. Late-onset neonatal sepsis: recent developments. Arch Dis Child Fetal Neonatal Ed. (2015) 100:F257–263. doi: 10.1136/archdischild-2014-306213
5. Greenberg RG, Kandefer S, Do BT, Smith PB, Stoll BJ, Bell EF, et al. Late-onset sepsis in extremely premature infants: 2000–2011. Pediatr Infect Dis J. (2017) 36:774–9. doi: 10.1097/inf.0000000000001570
6. Liu Z, Feng J, Shi L. Clinical analysis and examination of neonatal sepsis. J Pak Med Assoc. (2020) 70:120–4.
7. Escalante MJ, Ceriani-Cernadas JM, D'Apremont I, Bancalari A, Webb V, Genes L, et al. Late onset sepsis in very low birth weight infants in the South American NEOCOSUR network. Pediatr Infect Dis J. (2018) 37:1022–7. doi: 10.1097/inf.0000000000001958
8. Dilli D, Dilmen U. The role of interleukin-6 and C-reactive protein in non-thyroidal illness in premature infants followed in neonatal intensive care unit. J Clin Res Pediatr Endocrinol. (2012) 4:66–71. doi: 10.4274/jcrpe.625
9. Opazo MC, Haensgen H, Bohmwald K, Venegas LF, Boudin H, Elorza AA, et al. Imprinting of maternal thyroid hormones in the offspring. Int Rev Immunol. (2017) 36:240–55. doi: 10.1080/08830185.2016.1277216
10. Huang Y, Yu X, Li W, Li Y, Yang J, Hu Z, et al. Development and validation of a nomogram for predicting late-onset sepsis in preterm infants on the basis of thyroid function and other risk factors: Mixed retrospective and prospective cohort study. J Adv Res. (2020) 24:43–51. doi: 10.1016/j.jare.2020.02.005
11. Corbetta C, Weber G, Cortinovis F, Calebiro D, Passoni A, Vigone MC, et al. A 7-year experience with low blood TSH cutoff levels for neonatal screening reveals an unsuspected frequency of congenital hypothyroidism (CH). Clin Endocrinol. (2009) 71:739–745. doi: 10.1111/j.1365-2265.2009.03568.x
12. Williams FL, Mires GJ, Barnett C, Ogston SA, van Toor H, Visser TJ, et al. Transient hypothyroxinemia in preterm infants: the role of cord sera thyroid hormone levels adjusted for prenatal and intrapartum factors. J Clin Endocrinol Metab. (2005) 90:4599–4606. doi: 10.1210/jc.2005-0214
13. Miao KF, Duan W, Qian Y, Chen DQ. Analysis of influencing factors of transient hypothyroxinemia and low T3 syndrome in premature infants. Zhonghua Er Ke Za Zhi. (2013) 51:607–11. doi: 10.3760/cma.j.issn.0578-1310.2013.08.011
14. Cai N, Fan W, Tao M, Liao W. A significant decrease in hemoglobin concentrations may predict occurrence of necrotizing enterocolitis in preterm infants with late-onset sepsis. J Int Med Res. (2020) 48:300060520952275. doi: 10.1177/0300060520952275
15. Shane AL, Sánchez PJ, Stoll BJ. Neonatal sepsis. Lancet. (2017) 390:1770–80. doi: 10.1016/S0140-6736(17)31002-4
16. Cheng J, Sun J, Yao K, Xu M, Cao Y. A variable selection method based on mutual information and variance inflation factor. Spectrochim Acta A Mol Biomol Spectrosc. (2022) 268:120652. doi: 10.1016/j.saa.2021.120652
17. Sanders RC Jr, Giuliano JS Jr, Sullivan JE, Brown CA III, Walls RM, Nadkarni V, et al. Level of trainee and tracheal intubation outcomes. Pediatrics. (2013) 131:e821–8. doi: 10.1542/peds.2012-2127
18. Olson JC, Karvellas CJ. Critical care management of the patient with cirrhosis awaiting liver transplant in the intensive care unit. Liver Transpl. (2017) 23:1465–76. doi: 10.1002/lt.24815
19. Bozaykut A, Ipek IO, Kilic BD. Predicting neonatal sepsis in ventilated neonates. Indian J Pediatr. (2008) 75:39–42. doi: 10.1007/s12098-008-0004-y
20. Manandhar S, Amatya P, Ansari I, Joshi N, Maharjan N, Dongol S, et al. Risk factors for the development of neonatal sepsis in a neonatal intensive care unit of a tertiary care hospital of Nepal. BMC Infect Dis. (2021) 21:546. doi: 10.1186/s12879-021-06261-x
21. Yapicioglu H, Ozcan K, Sertdemir Y, Mutlu B, Satar M, Narli N, et al. Healthcare-associated infections in a neonatal intensive care unit in Turkey in 2008: incidence and risk factors, a prospective study. J Trop Pediatr. (2011) 57:157–64. doi: 10.1093/tropej/fmq060
22. Audu LI, Otuneye AT, Mairami AB, Mukhtar-Yola M, Mshelia LJ. Determination of neonatal case-specific fatality rates in a tertiary health institution in North Central Nigeria. BMC Pediatr. (2021) 21:302doi: 10.1186/s12887-021-02778-x
23. Pirrone A, Panzani S, Govoni N, Castagnetti C, Veronesi MC. Thyroid hormone concentrations in foals affected by perinatal asphyxia syndrome. Theriogenology. (2013) 80:624–9. doi: 10.1016/j.theriogenology.2013.06.003
24. Yildizdaş D, Onenli-Mungan N, Yapicioglu H, Topaloglu AK, Sertdemir Y, Yüksel B, et al. Thyroid hormone levels and their relationship to survival in children with bacterial sepsis and septic shock. J Pediatr Endocrinol Metab. (2004) 17:1435–42. doi: 10.1515/JPEM.2004.17.10.1435
25. Grondman I, Nooijer de, Antonakos AH, Janssen N, Mouktaroudi NAF, Leventogiannis M, et al. The Association of TSH and Thyroid Hormones With Lymphopenia in Bacterial Sepsis and COVID-19. J Clin Endocrinol Metab. (2021) 106:1994–2009. doi: 10.1210/clinem/dgab148
26. Korang SK, Safi S, Nava C, Greisen G, Gupta M, Lausten-Thomsen U, et al. Antibiotic regimens for late-onset neonatal sepsis. Cochrane Database Syst Rev. (2021) 5:Cd013836. doi: 10.1002/14651858.CD013836.pub2
27. Silva ACB, Anchieta LM, Lopes MFP, Romanelli RMC. Inadequate use of antibiotics and increase in neonatal sepsis caused by resistant bacteria related to health care assistance: a systematic review. Braz J Infect Dis. (2018) 22:328–37. doi: 10.1016/j.bjid.2018.07.009
28. Turkish Neonatal Society and Nosocomial Infections Study group. Nosocomial infections in neonatal units in Turkey: epidemiology, problems, unit policies and opinions of healthcare workers. Turk J Pediatr. (2010) 52:50–57.
Keywords: sepsis, late-onset, prediction, preterm infants, East China
Citation: Shuai X, Li X and Wu Y (2022) Prediction for late-onset sepsis in preterm infants based on data from East China. Front. Pediatr. 10:924014. doi: 10.3389/fped.2022.924014
Received: 20 April 2022; Accepted: 03 August 2022;
Published: 14 September 2022.
Edited by:
Ming-Chou Chiang, Linkou Chang Gung Memorial Hospital, TaiwanReviewed by:
Roberto Murgas Torrazza, Tecnología e Innovación, PanamaCopyright © 2022 Shuai, Li and Wu. This is an open-access article distributed under the terms of the Creative Commons Attribution License (CC BY). The use, distribution or reproduction in other forums is permitted, provided the original author(s) and the copyright owner(s) are credited and that the original publication in this journal is cited, in accordance with accepted academic practice. No use, distribution or reproduction is permitted which does not comply with these terms.
*Correspondence: Xianghua Shuai, c2h1YWl4aWFuZ2h1YUBvdXRsb29rLmNvbQ==
Disclaimer: All claims expressed in this article are solely those of the authors and do not necessarily represent those of their affiliated organizations, or those of the publisher, the editors and the reviewers. Any product that may be evaluated in this article or claim that may be made by its manufacturer is not guaranteed or endorsed by the publisher.
Research integrity at Frontiers
Learn more about the work of our research integrity team to safeguard the quality of each article we publish.