- 1Centre for Heart Lung Innovation, University of British Columbia, Vancouver, BC, Canada
- 2Faculty of Health Sciences, McMaster University, Hamilton, ON, Canada
- 3Department of Epidemiology, Biostatistics, and Occupational Health, McGill University, Montréal, QC, Canada
- 4Faculty of Medicine, University of British Columbia, Vancouver, BC, Canada
Introduction: Metabolomics is an emerging area of research and has the potential to identify clinical biomarkers for predicting or diagnosing cystic fibrosis (CF) pulmonary exacerbations (PEx).
Objective: To identify clinically promising metabolites across different sample sources that can be used to predict or diagnose PEx in CF.
Evidence Review: Searches for original literature were completed through EMBASE, MEDLINE, and all databases on the Web of Science with no restrictions on language or publication date. Gray literature was collected through Google Scholar. Additional studies were obtained by contacting authors and searching reference lists of candidate papers. The patient population included individuals with CF. Studies involving patients who underwent lung transplantation were excluded. The outcome was the prediction or diagnosis of pulmonary exacerbations from metabolites directly measured from biological samples. Search results were downloaded and imported into Covidence and duplicates were removed automatically. Any remaining duplicates were manually tagged and excluded. Two independent reviewers screened each abstract for eligibility and repeated this process for full texts. Risk of bias was conducted using QUADAS-2 by two independent reviewers. A third author resolved any remaining conflicts.
Results: A combined 3974 relevant abstracts were identified and 115 full texts were assessed for eligibility. The final 25 studies underwent data extraction for study design, patient demographics, studied metabolites, concentration values, and diagnostic accuracy values. Included studies differed considerably in methodologies, sample specimen types (exhaled breath condensate [EBC], sputum, saliva, plasma, urine), and disease states. We identified 19 unique metabolites that were measured by two or more studies of which 2 have the potential to predict PEx (EBC 4-hydroxycyclohexylcarboxylic acid [4-HCHC] and lactic acid) and 6 to diagnose PEx (EBC 4-HCHC and lactic acid, sputum lactic acid and nitrate, and plasma arginine and methionine).
Conclusion and Relevance: This systematic review has identified promising metabolites for further study in CF. Certain metabolites may provide clinical potential in predicting or diagnosing PEx, but further validation studies are required. With better tools to aid in the earlier identification of PEx, clinicians can implement preventative measures to mitigate airway damage.
Systematic Review Registration: https://www.crd.york.ac.uk/prospero/
Introduction
Cystic fibrosis (CF) is one of the most common inherited life-shortening conditions that causes chronic progressive lung disease. The disease course is characterized by episodes of acute or subacute clinical worsening referred to as pulmonary exacerbations (PEx) due to increased airway infection and inflammation. PEx symptoms typically include increased productive cough and systemic symptoms such as fatigue, loss of appetite, and weight loss (1). The clinical presentation with PEx can be subtle, especially early in its course, resulting in missed opportunities to intervene with antibiotics to preserve lung function. By using biomarkers to predict imminent exacerbation risk or facilitate earlier diagnosis, patient outcomes can be improved.
Within the “-omics” field, most of the focus has been on examining sputum and blood proteomic and transcriptomic inflammatory markers in CF patients to diagnose PEx (2, 3). However, metabolomic studies are also emerging and involve a range of biospecimens. While most studies have focused on differences in metabolites between CF and non-CF subjects, several studies have also focused on PEx. The present systematic review has synthesized data from the available literature to determine if there are promising metabolites across the various biospecimens to be evaluated further as clinical biomarkers in the prediction and diagnosis of PEx.
Materials and Methods
Study Eligibility
To define the review question, the PICO framework for diagnostic tests was implemented (4). Our population of interest was patients who had a confirmed diagnosis of CF. The investigated test of interest was the analysis of metabolites as a predictive or diagnostic biomarker. A comparator test was not applicable for this review. The outcome was the prediction or diagnosis of a PEx based on various research criteria, such as Fuchs, Rosenfeld, or physician decision to begin antibiotic treatment (5, 6).
Studies that collected biospecimens for the purpose of analyzing metabolites were included in this review. We broadly included studies that used metabolites to predict or diagnose PEx in CF patients. Studies that only examined metabolite changes throughout PEx treatment were excluded. Furthermore, studies were excluded if they solely focused on laboratory samples (e.g., in vitro studies). Studies were also excluded if they used genomic, proteomic, or microbiome approaches without addressing metabolites. A detailed summary of study characteristics is presented in Table 1.
Search Strategy
The search strategy and protocol were registered through PROSPERO [CRD42021269309]. Our study followed the Preferred Reporting Items for Systematic reviews and Meta-Analyses (PRISMA) guidelines for the search strategy, text screening, and data extraction (32). A health sciences librarian advised on the search strategy throughout the piloting process. The primary author (AVN) conducted the last search on August 26, 2021. An example of the search terms used and a completed search can be found in Supplementary Table 1 (Appendix). Alerts for newly published literature were sent to the primary author. Searches for original literature were completed through MEDLINE via Ovid, EMBASE via Ovid, and all databases on Web of Science with no restrictions on language, publication date, or publication status. The search strategy was adapted for each database. Gray literature was collected through Google Scholar. The authors decided to arbitrarily limit citations on Google Scholar to the first 200 results based on recommendations by Haddaway et al. (33). Case reports and case series were excluded from the review.
Data Collection Process
A data collection form was created and modified based on recommendations from the PRISMA guidelines and included study details such as study design, patient demographics, metabolite processing methodology, statistical analysis methodology, sample source, identifiable metabolite nomenclature, metabolite concentration data, diagnostic accuracy data, association to inflammation, and risk of bias using Quality Assessment of Diagnostic Accuracy Studies (QUADAS-2) (34). The data extraction form was piloted on five studies and adjusted accordingly. Two independent reviewers (AVN and DH) extracted data from the eligible full-texts and proceeded to reach consensus through discussion and input from a third reviewer (BSQ).
Synthesis of Results
Due to the heterogeneity of the included studies, a meta-analysis was not feasible. Details of the included studies are demonstrated in Table 1, including study designs and patient characteristics. A tabulated summary of studied metabolites that were classified based on their chemical taxonomy is presented in Table 2. The biospecimen types and analytical platforms used to measure the metabolites are detailed in Table 3. Furthermore, we included information on whether these unique metabolites were used to predict or diagnose PEx, the sample source, the direction of change in concentrations between disease states, and any statistical significance in comparisons between disease states. Metabolites that appeared in two or more studies are summarized in Table 4. Metabolite ratios and broad pathways implicated in PEx that were identified within studies were not included in our summary. A summary of the risk of bias using QUADAS-2 can be accessed in Supplementary Table 2 (Appendix).
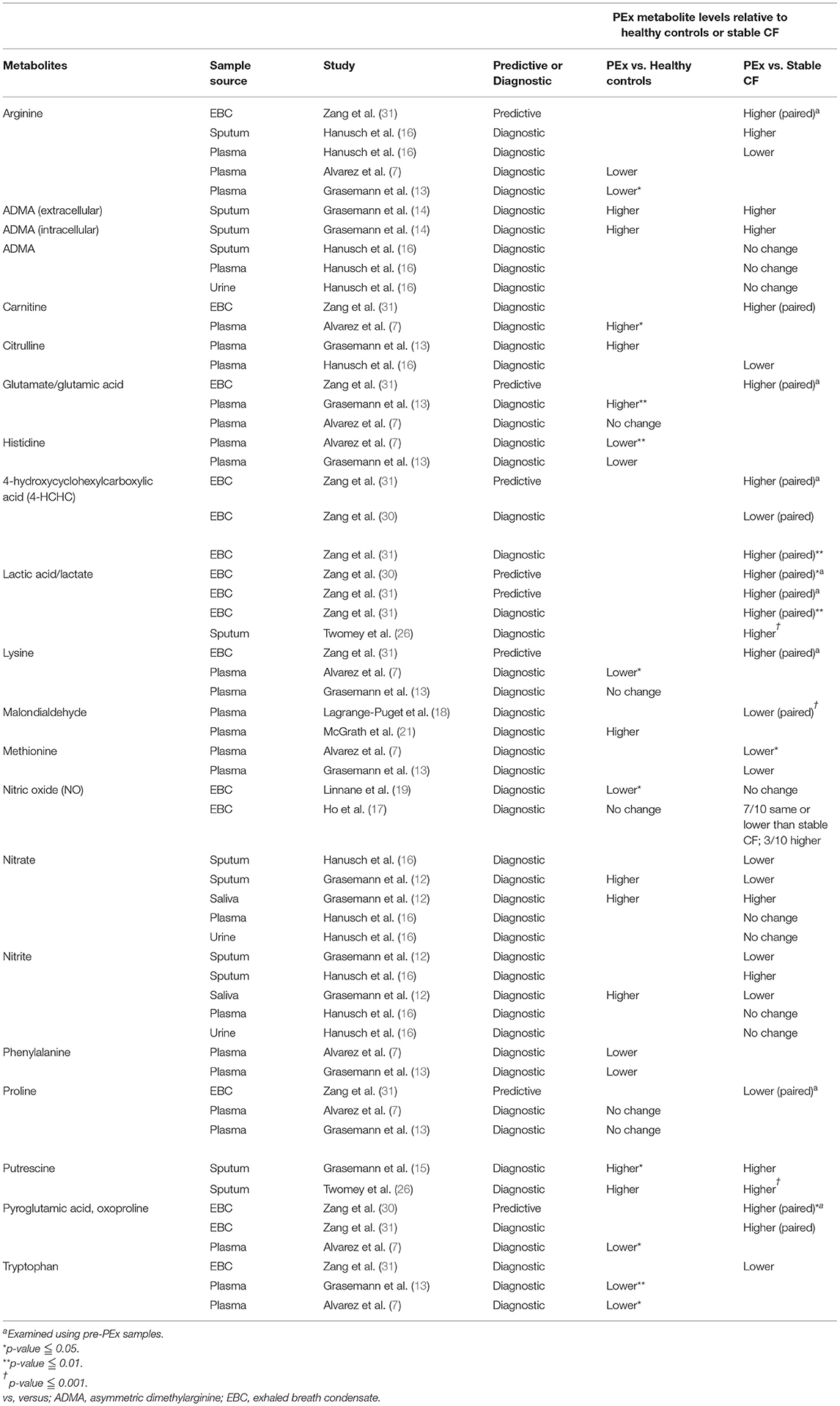
Table 4. Metabolites analyzed in two or more studies from various biospecimens to predict or diagnose PEx.
Results
Study Selection
Initially, 9055 search results were uploaded onto Covidence for screening. A combined 3974 relevant abstracts were identified after duplicates were automatically removed. Authors were contacted for full-texts or additional information. 115 full texts were assessed for eligibility prior to data extraction. Of these 115, one was added through an updated search alert. The final 25 studies underwent full data extraction and risk of bias assessment. Three studies were deemed to be predictive and 22 studies were diagnostic. The study selection process is illustrated in Figure 1 through a PRISMA diagram.
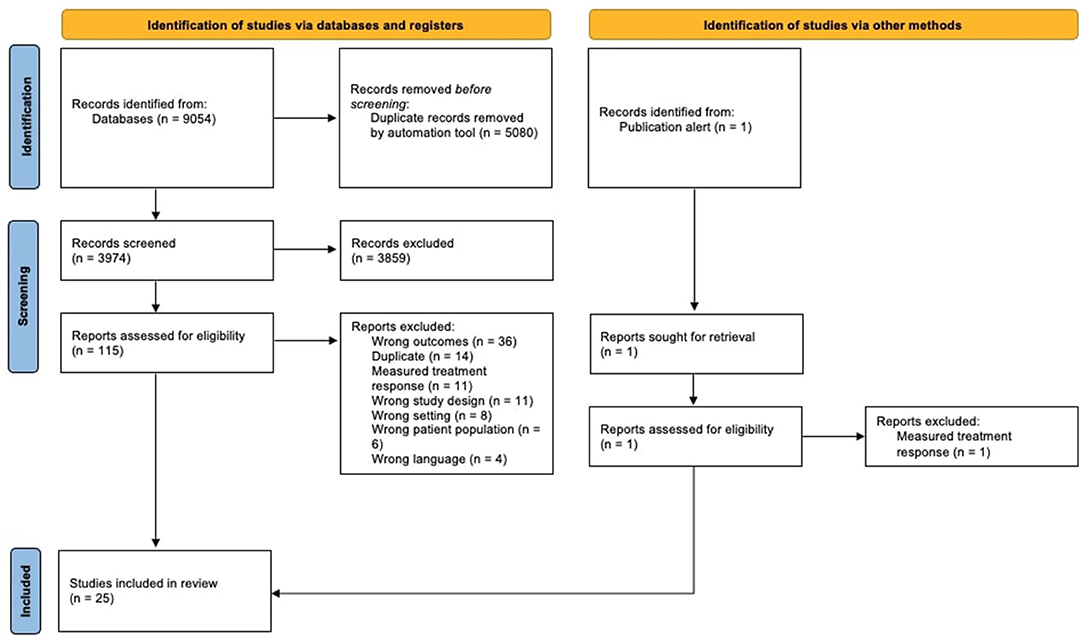
Figure 1. PRISMA (Preferred Reporting Items for Systematic Review and Meta-Analysis) flow diagram detailing study selection process.
Study Designs
Details of eligible studies are shown in Table 1. 13/25 (52%) studies were conducted in North America and the remainder were completed in Europe. 13/25 (52%) of the studies had <50 total participants. 14/25 (56%) studies followed the same patients during stable and PEx visits (paired samples). Two studies only used non-CF healthy controls instead of stable CF patients. The remaining studies collected data in both non-CF healthy individuals and stable CF patients. 8/25 (32%) of the eligible studies defined PEx diagnosis using previously published criteria (e.g., Fuchs, Rosenfeld, etc.) while the remaining studies used a variety of criteria such as physician-defined criteria, the decision to use intravenous antibiotics, or specific symptoms or tests. Most of the studies (22/25, 88%) in this present review examined metabolites for the diagnosis of PEx, as detailed in Table 1. Diagnostic validity concepts such as sensitivity and specificity of metabolites were only analyzed in three eligible studies.
Biospecimen Types and Metabolite Methodologies
Biospecimen types and methods of metabolite identification and analysis varied among studies (Table 3). Out of the 25 included studies, 11/25 (44%) analyzed sputum, 10/25 (40%) plasma and/or serum, 5/25 (20%) exhaled breath condensate (EBC), and 2/25 (8%) urine samples. Within certain studies, multiple analytical platforms were used for various metabolites. For at least one included metabolite within a study, 4/25 (16%) used liquid chromatography with tandem mass spectrometry (LC-MS-MS), 3/25 (12%) used LC-MS, 4/25 (16%) used gas chromatography in some capacity with either MS or another apparatus, 6/25 (24%) used high-performance liquid chromatography (HPLC), and 4/25 (16%) used ultra-performance liquid chromatography (UPLC). Other analytical platforms were variable. A list of metabolites from the eligible studies categorized according to class as outlined by the Human Metabolome Database (HMDB) (35) is detailed in Table 2. Only named metabolites were included in the table, whereas compounds with only molecular formulas were not.
Patient Characteristics From Included Studies
Patient characteristics of the included studies are detailed in Table 3. Seven studies focused on pediatric patients (<18 years old), nine studies included solely adult patients, and 8 studies included both populations studies included both populations. Few of the studies reported metabolites found in adults and pediatric patients separately. FEV1 values for both stable CF and PEx visits were not reported in every study. In Table 3, the FEV1 values represent only the PEx visits, which ranged from 32 to 82% across all studies.
Risk of Bias Assessment
Using QUADAS-2, the risk of bias of eligible studies was determined by two independent reviewers (AVN and DH). Summary of the risk of bias and applicability concerns can be found in Supplementary Table 2 (Appendix). 10/25 (40%) of the studies had two or more domains within the risk of bias assessment rated as either “High risk” or “Unclear” and most studies demonstrated high risk of bias for the index test as the index test assessor, typically whoever was retrieving or analyzing the sample, was not blinded. Most included studies (18/25; 72%) did not pose any applicability concerns in any of the domains. Three studies (23–25) were deemed as high risk for applicability in the patient selection domain and only one (24) was deemed as high risk within the index test applicability domain. Since most of the studies were not explicitly designed to be diagnostic accuracy studies, some QUADAS-2 signaling questions were not applicable. In this case, the reviewers indicated the prompt as “Unclear.” Depending on the answers of the other domain-specific signaling questions, the reviewers would determine if there was a risk of bias despite the “Unclear” response.
Biomarkers for Predicting and Diagnosing PEx
We identified 19 unique metabolites that were measured by two or more studies (Table 4). Metabolites were deemed to have potential for either prediction or diagnosis of PEx if: (1) the changes had consistent directionality in at least two studies within the same sample type (regardless of statistical significance) and at least one of the two studies compared PEx to stable CF samples as opposed to healthy controls as the latter comparison is more likely to be confounded by other factors; or (2) there was statistical significance within at least one study when comparing PEx to stable CF samples.
Biomarkers to Predict PEx
Just three studies evaluated metabolites to predict PEx in CF and two were performed by the same research group (30, 31). In both studies, EBC lactic acid levels were higher in pre-PEx compared to stable CF samples. Zang et al. (30) also found statistical significantly higher levels of EBC pyroglutamic acid in pre-PEx compared to paired stable samples. Zang et al. (31) evaluated other metabolites from EBC including arginine, lysine, proline, glutamic acid, and 4-HCHC, but these were not found to be significantly different in pre-PEx compared to paired stable CF samples.
Biomarkers to Diagnose PEx
Several metabolites from plasma and airway samples (sputum, EBC) were deemed to have potential as biomarkers to diagnose PEx. Plasma methionine was found to be lower in PEx compared to stable CF samples in two studies (7, 13), with one comparison being statistically significant (7). Three studies (7, 13, 16) found lower plasma arginine levels in PEx compared to healthy control or stable CF samples, but only the comparison to healthy controls was statistically significant (13). Plasma histidine, phenylalanine, and tryptophan levels were lower in PEx compared to healthy control samples in two separate studies (7, 13) but these were not compared to stable CF samples. Plasma carnitine (7, 31) and glutamate (7, 13) were significantly higher and plasma lysine and pyroglutamic acid levels were significantly lower in PEx compared to healthy control samples in one study (7) each but once again these were not compared to stable CF samples.
In terms of EBC, 4-HCHC and lactic acid levels were significantly higher in PEx compared to paired stable CF samples and both were evaluated in the same study (31). EBC NO levels were significantly lower in PEx compared to healthy control samples in one study (19) but was not compared to stable CF samples. For sputum, putrescine was consistently higher in PEx compared to healthly control and stable CF samples (15, 26) but only the comparison to healthy control samples was statistically significant. Sputum nitrate was consistently lower in PEx compared to stable CF samples in two studies, but the differences were not statistically significant (12, 16).
Discussion
By applying metabolomic biomarkers to track disease activity, there is the potential to diagnose PEx earlier in its course. Thus, PEx management can be enacted earlier to prevent long-term and irreversible lung damage. While individual studies have evaluated metabolites to either predict or diagnose PEx in CF, this systematic review fills a gap in the literature by synthesizing all of the published studies to date to identify promising metabolites in need for further study.
Heterogeneity and Bias in Study Designs
Despite some metabolites being evaluated in two or more studies, heterogeneity in study designs posed a challenge in synthesizing the results. While some studies used paired samples from individual patients during different disease states (i.e., stable vs. PEx), other studies used independent groups of healthy or CF patients to serve as stable controls. Use of independent groups is more vulnerable to confounding by factors other than disease state that might be driving the group differences in metabolite profiles.
Other considerations must also be made when studying the volatile nature of metabolites. One concern is the lack of a fasting protocol in most studies. The associated vitamins, minerals and macronutrients contained in food can impact the accuracy of test samples, especially when these same metabolites were the primary analyte of interest (36). The inconsistency across studies may impact the concentration of metabolites. Another consideration is the timeframe between sample collection and treatment. Antibiotics are routinely used for PEx treatment and may impact the metabolome during the initial treatment period. Thus, studies should ensure that samples are taken prior to any intervention and with uniform fasting protocols.
Another aspect to be considered is the reporting of blinding during index and reference testing. While it may be difficult to blind research coordinators collecting samples from CF or non-CF patients, those who process and analyze the samples should be blinded to the patient's disease state. One randomized pilot study did blind researchers during sample anaylsis (7).
Furthermore, existing studies have been conducted using a wide variety of sample sources and analytical methods to identify and quantify the metabolites. While there are advantages in collecting samples such as urine and blood due to ease of collection across most age groups and translation into clinical practice (37), sensitivity and specificity are potential concerns when monitoring lung disease status as detected metabolites could arise from other organs. Due to the wide array of metabolites and dynamic range of concentrations, no single analytical tool is capable of measuring all metabolites which is reflected in the diversity of platforms examined. As such, several of the included studies used two or more complementary analytical platforms. The most widely used analytical approaches were LC- and GC-MS, as well as ultra-performance LC (UPLC) and high-performance LC (HPLC), the latter separation techniques sometimes used in combination with MS to improve sensitivity and analyte resolution (38, 39). An advantage of LC-MS is that it does not require chemical pre-processing and can detect a broad range of metabolites which makes it ideal for untargeted discovery analysis. While GC-MS is more quantitative with excellent separation reproducibility and lower running costs than LC-MS, it is limited to the measurement of thermally stable and volatile compounds (40). Sample preparation and metabolite extraction differences across these platforms can pose a challenge in making comparisons across studies (41). As such, future studies must continue to explore the differences and similarities in metabolites across the various biological compartments and factor in the analytical platform used.
Synthesis of Metabolite Results
Metabolites from the nitric oxide pathway have been the most extensively studied to date. Based on available data, it remains unclear if exhaled NO is lower during PEx compared to stable state as the studies performed to date have been relatively small and the findings have been inconsistent (17, 19). Metabolites of NO, including nitrate and nitrite, do not appear to be consistently higher or lower during PEx when sampled from saliva, plasma or urine but nitrates were lower in sputum in two studies but the differences were not statistically significant when compared to stable CF subjects (12, 16). Arginine, an amino acid that acts as a substrate to form NO, was not significantly higher in sputum during PEx (16) but another study demonstrated its potential to predict PEx when elevated in EBC (31). Contrary to the increase in airway sampling, plasma arginine was lower in CF PEx compared to healthy controls (13) but was not significantly lower than stable CF samples (16).
Carboxylic acids and derivatives have also been investigated in the context of PEx. EBC pyroglutamic acid levels were higher in pre-PEx compared to stable CF samples demonstrating the potential to predict PEx (30, 31). Plasma methionine levels were lower in PEx compared to stable CF samples and has the potential to diagnose PEx (7, 13). Plasma histidine, lysine, phenylalanine, pyroglutamic acid, and tryptophan levels were found to be lower and glutamic acid levels higher in CF PEx compared to healthy control samples but levels were not compared to stable CF (7, 13). This may reflect intrinsic differences between CF and healthy controls as opposed to differences related to PEx specifically as malnutrition in CF can lead to changes in the plasma amino acid profile compared to healthy subjects (42).
Within the organonitrogen compound class, sputum putrescine shows promise as being a diagnostic biomarker of PEx as levels were found to be higher in PEx compared to both healthy control and stable CF samples in two separate studies although the comparisons were not statistically significant due to the small sample sizes of the studies (15, 26). The potential role of ornithine-derived putrescine in PEx is unclear but it may play a role in smooth muscle regulation and anti-inflammatory processes (43). Within the organooxygen class, 4-hydroxycyclohexylcarboxylic acid (4-HCHC) from EBC was higher in PEx compared to stable CF samples. In terms of its potential role in CF pathophysiology, 4-HCHC is a rare organic acid involved in gut microbial and mammalian metabolism and its appearance in EBC is suggestive of gut-lung crosstalk with increased inflammation in both compartments (44).
Lactic acid, or lactate, has demonstrated both predictive and diagnostic potential in our review. When analyzed predictively, two studies from the same research group have found EBC lactic acid to be higher during pre-PEx compared to stable CF samples. Diagnostically, EBC and sputum lactic acid levels were higher in PEx compared to stable CF samples (26, 31). Lactic acid is a fermentation metabolite that might originate from anaerobic bacteria within the hypoxic airway environment. Anaerobic bacteria have been implicated in the pathophysiology of PEx (45).
Limitations
Within this current review, there are several limitations that must be considered. Firstly, studies with statistically significant findings are more likely to be published which poses concern for publication bias similar to most systematic reviews. Furthermore, most conference abstracts were excluded during full-text review due to a lack of patient details, data presentation, and inability to assess risk of bias. Secondly, due to the heterogeneity of the study designs and objectives, we were unable to synthesize the results in a formal meta-analysis. Thus, we were limited to providing a tabulated summary of the studied metabolites and their respective directionality of change and the statistical significance of the change.
Future Directions
With the evolving landscape of metabolomic research in CF, future studies must consider better standardization in study design, timing and method of metabolite collection and analysis, and reporting. Future studies that aim to test the diagnostic or predictive value of metabolite biomarkers in PEx should also ensure that data is collected during clearly defined disease states. The distinction between stable CF, pre-PEx, PEx, and pre-treatment and post-treatment states can be challenging but should be clearly outlined in future study designs. As well, consistency in fasting protocols across all sample types may be warranted.
Moreover, based on this review's search results, there were only two studies from the same research group that examined metabolites to help predict PEx (30, 31). This result highlights the need for more studies to consider prediction alongside diagnosis when characterizing biomarkers. While predicting PEx is understandably a more challenging clinical setting to apply biomarkers, this is the setting that will provide the most clinical utility as demonstrating differences in metabolites in settings in which there is a clear difference in clinical presentation (stable vs. PEx) is less useful but a reasonable starting point. Furthermore, comparison of metabolite levels to healthy control samples is less useful as differences may reflect differences between CF disease vs. healthy as opposed to disease activity related to PEx whereby a comparison to stable CF samples is much more relevant. Furthermore, metabolomic biomarkers for disease monitoring must also be re-evaluated in the context of CFTR modulator use as promising biomarkers, such as FeNO, can be influenced by CFTR modulators (46).
Lastly, it is important to consider how these studies can be translated into clinical practice in the long-term. For any promising metabolites to be used, they must undergo rigorous testing and validation as mandated by the Institute of Medicine to determine its appropriateness in clinical practice (47). Within this systematic review, we were unable to identify any metabolomic biomarkers that have undergone this process.
Conclusion
Overall, this systematic review creates a foundation for future metabolomic biomarker research in PEx. While there are promising metabolites for predicting and diagnosing PEx that have been identified in multiple studies, further validation and exploration is needed. These studies should aim to standardize study design, metabolite collection, analysis, and reporting before clinical validation can be considered.
Data Availability Statement
The original contributions presented in the study are included in the article/Supplementary Material, further inquiries can be directed to the corresponding author.
Author Contributions
AVN and BQ conceptualized the research question, designed the study protocol, contributed to study screening, drafted the manuscript, revised for scientific content, and provided critical revisions. AVN, MB, and DH contributed to study screening and data extraction. AVN and DH completed risk of bias assessment. AVN, BQ, MB, and DH contributed to table design and formatting. BQ supervised the study and provided support. All authors reviewed and approved the final draft.
Funding
BQ was supported by a Michael Smith Foundation for Health Research Scholar Award (#16414).
Conflict of Interest
The authors declare that the research was conducted in the absence of any commercial or financial relationships that could be construed as a potential conflict of interest.
Publisher's Note
All claims expressed in this article are solely those of the authors and do not necessarily represent those of their affiliated organizations, or those of the publisher, the editors and the reviewers. Any product that may be evaluated in this article, or claim that may be made by its manufacturer, is not guaranteed or endorsed by the publisher.
Acknowledgments
Our team thanks Mr. Dean Giuistini, MLIS for his consultation on the search strategy. We also thank our volunteer, Ms. Dina Babiker, for her contributions during abstract screening.
Supplementary Material
The Supplementary Material for this article can be found online at: https://www.frontiersin.org/articles/10.3389/fped.2022.896439/full#supplementary-material
References
1. Ferkol T, Rosenfeld M, Milla CE. Cystic fibrosis pulmonary exacerbations. J Pediatr. (2006) 148:259–64. doi: 10.1016/j.jpeds.2005.10.019
2. Sagel SD, Chmiel JF, Konstan MW. Sputum biomarkers of inflammation in cystic fibrosis lung disease. Proc Am Thorac Soc. (2007) 4:406–17. doi: 10.1513/pats.200703-044BR
3. Shoki AH, Mayer-Hamblett N, Wilcox PG, Sin DD, Quon BS. Systematic review of blood biomarkers in cystic fibrosis pulmonary exacerbations. Chest. (2013) 144:1659–70. doi: 10.1378/chest.13-0693
4. Luijendijk HJ. How to create PICO questions about diagnostic tests. BMJ Evid-Based Med. (2021) 26:155–7. doi: 10.1136/bmjebm-2021-111676
5. Fuchs HJ, Borowitz DS, Christiansen DH, Morris EM, Nash ML, Ramsey BW, et al. Effect of aerosolized recombinant human DNase on exacerbations of respiratory symptoms and on pulmonary function in patients with cystic fibrosis. The pulmozyme study group. N Engl J Med. (1994) 331:637–42. doi: 10.1056/NEJM199409083311003
6. Sanders DB, Bittner RCL, Rosenfeld M, Hoffman LR, Redding GJ, Goss CH. Failure to recover to baseline pulmonary function after cystic fibrosis pulmonary exacerbation. Am J Respir Crit Care Med. (2010) S182:627–32. doi: 10.1164/rccm.200909-1421OC
7. Alvarez JA, Chong EY, Walker DI, Chandler JD, Michalski ES, Grossmann RE, et al. Plasma metabolomics in adults with cystic fibrosis during a pulmonary exacerbation: a pilot randomized study of high-dose vitamin D3 administration. Metabolism. (2017) 70:31–41. doi: 10.1016/j.metabol.2017.02.006
8. Barr HL, Halliday N, Cámara M, Barrett DA, Williams P, Forrester DL, et al. Pseudomonas aeruginosa quorum sensing molecules correlate with clinical status in cystic fibrosis. Eur Respir J. (2015) 46:1046–54. doi: 10.1183/09031936.00225214
9. Cantin AM, Bilodeau G, Larivée P, Richter MV. Plasma biomarkers and cystic fibrosis lung disease. Clin Investig Med Med Clin Exp. (2012) 35:E173–181. doi: 10.25011/cim.v35i4.17145
10. Felton E, Burrell A, Chaney H, Sami I, Koumbourlis AC, Freishtat RJ, et al. Inflammation in children with cystic fibrosis: contribution of bacterial production of long-chain fatty acids. Pediatr Res. (2021) 90:99–108. doi: 10.1038/s41390-021-01419-4
11. Ghorbani P, Santhakumar P, Hu Q, Djiadeu P, Wolever TMS, Palaniyar N, et al. Short-chain fatty acids affect cystic fibrosis airway inflammation and bacterial growth. Eur Respir J. (2015) 46:1033–45. doi: 10.1183/09031936.00143614
12. Grasemann H, Ioannidis I, Tomkiewicz RP, Groot H de, Rubin BK, Ratjen F. Nitric oxide metabolites in cystic fibrosis lung disease. Arch Dis Child. (1998) 78:49–53. doi: 10.1136/adc.78.1.49
13. Grasemann H, Schwiertz R, Grasemann C, Vester U, Racké K, Ratjen F. Decreased systemic bioavailability of L-arginine in patients with cystic fibrosis. Respir Res. (2006) 7:87. doi: 10.1186/1465-9921-7-87
14. Grasemann H, Al-Saleh S, Scott JA, Shehnaz D, Mehl A, Amin R, et al. Asymmetric dimethylarginine contributes to airway nitric oxide deficiency in patients with cystic fibrosis. Am J Respir Crit Care Med. (2011) 183:1363–8. doi: 10.1164/rccm.201012-1995OC
15. Grasemann H, Shehnaz D, Enomoto M, Leadley M, Belik J, Ratjen F. L-ornithine derived polyamines in cystic fibrosis airways. PLoS ONE. (2012) 7:e46618. doi: 10.1371/journal.pone.0046618
16. Hanusch B, Brinkmann F, Mayorandan S, Chobanyan-Jürgens K, Wiemers A, Jansen K, et al. Local and systemic alterations of the L-arginine/nitric oxide pathway in sputum, blood, and urine of pediatric cystic fibrosis patients and effects of antibiotic treatment. J Clin Med. (2020) 9:3802. doi: 10.3390/jcm9123802
17. Ho LP, Innes JA, Greening AP. Exhaled nitric oxide is not elevated in the inflammatory airways diseases of cystic fibrosis and bronchiectasis. Eur Respir J. (1998) 12:1290–4. doi: 10.1183/09031936.98.12061290
18. Lagrange-Puget M, Durieu I, Ecochard R, Abbas-Chorfa F, Drai J, Steghens JP, et al. Longitudinal study of oxidative status in 312 cystic fibrosis patients in stable state and during bronchial exacerbation. Pediatr Pulmonol. (2004) 38:43–9. doi: 10.1002/ppul.20041
19. Linnane SJ, Keatings VM, Costello CM, Moynihan JB, O'Connor CM, Fitzgerald MX, et al. Total sputum nitrate plus nitrite is raised during acute pulmonary infection in cystic fibrosis. Am J Respir Crit Care Med. (1998) 158:207–12. doi: 10.1164/ajrccm.158.1.9707096
20. Lucca F, Da Dalt L, Ros M, Gucciardi A, Pirillo P, Naturale M, et al. Asymmetric dimethylarginine and related metabolites in exhaled breath condensate of children with cystic fibrosis. Clin Respir J. (2018) 12:140–8. doi: 10.1111/crj.12502
21. McGrath LT, Mallon P, Dowey L, Silke B, McClean E, McDonnell M, et al. Oxidative stress during acute respiratory exacerbations in cystic fibrosis. Thorax. (1999) 54:518–23. doi: 10.1136/thx.54.6.518
22. Montuschi P, Paris D, Melck D, Lucidi V, Ciabattoni G, Raia V, et al. NMR spectroscopy metabolomic profiling of exhaled breath condensate in patients with stable and unstable cystic fibrosis. Thorax. (2012) 67:222–8. doi: 10.1136/thoraxjnl-2011-200072
23. Quinn RA, Lim YW, Mak TD, Whiteson K, Furlan M, Conrad D, et al. Metabolomics of pulmonary exacerbations reveals the personalized nature of cystic fibrosis disease. PeerJ. (2016) 4:e2174. doi: 10.7717/peerj.2174
24. Raghuvanshi R, Vasco K, Vázquez-Baeza Y, Jiang L, Morton JT, Li D, et al. High-resolution longitudinal dynamics of the cystic fibrosis sputum microbiome and metabolome through antibiotic therapy. mSystems. (2020) 5:e00292-20. doi: 10.1128/mSystems.00292-20
25. Topçu D, Tugcu G, Ozcan F, Aslan M, Yalcinkaya A, Polat SE, et al. Plasma ceramides and sphingomyelins of pediatric patients increase in primary ciliary dyskinesia but decrease in cystic fibrosis. Lipids. (2020) 55:213–23. doi: 10.1002/lipd.12230
26. Twomey KB, Alston M, An SQ, O'Connell OJ, McCarthy Y, Swarbreck D, et al. Microbiota and metabolite profiling reveal specific alterations in bacterial community structure and environment in the cystic fibrosis airway during exacerbation. PLoS ONE. (2013) 8:e82432. doi: 10.1371/journal.pone.0082432
27. van Horck M, Alonso A, Wesseling G, de Winter-de Groot K, van Aalderen W, Hendriks H., et al. (2016). Biomarkers in exhaled breath condensate are not predictive for pulmonary exacerbations in children with cystic fibrosis: results of a one-year observational study. PLOS ONE 11:e0152156. doi: 10.1371/journal.pone.0152156
28. Vazquez J, Betancourt M, Forseen C, Manavathu E. A surrogate marker for exacerbations and an analysis of indoleamine 2, 3-dioxygenase activity in cystic fibrosis patients. In: Pediatric Pulmonology. Orlando, FL; Orange County Convention Center (2016). p. S194–485. Available online at: https://www.ncbi.nlm.nih.gov/pmc/articles/PMC7159391/ (accessed March 1, 2022)
29. Wojewodka G, Sanctis JBD, Bernier J, Bérubé J, Ahlgren HG, Gruber J, et al. Candidate markers associated with the probability of future pulmonary exacerbations in cystic fibrosis patients. PLoS ONE. (2014) 9:e88567. doi: 10.1371/journal.pone.0088567
30. Zang X, Monge ME, McCarty NA, Stecenko AA, Fernández FM. Feasibility of early detection of cystic fibrosis acute pulmonary exacerbations by exhaled breath condensate metabolomics: a pilot study. J Proteome Res. (2017) 16:550–8. doi: 10.1021/acs.jproteome.6b00675
31. Zang X, Monge ME, Gaul DA, McCarty NA, Stecenko A, Fernández FM. Early detection of cystic fibrosis acute pulmonary exacerbations by exhaled breath condensate metabolomics. J Proteome Res. (2020) 19:144–52. doi: 10.1021/acs.jproteome.9b00443
32. Page MJ, McKenzie JE, Bossuyt PM, Boutron I, Hoffmann TC, Mulrow CD, et al. The PRISMA 2020 statement: an updated guideline for reporting systematic reviews. PLoS Med. (2021) 18:e1003583. doi: 10.1371/journal.pmed.1003583
33. Haddaway NR, Collins AM, Coughlin D, Kirk S. The role of google scholar in evidence reviews and its applicability to grey literature searching. PLoS ONE. (2015) 10:e0138237. doi: 10.1371/journal.pone.0138237
34. Whiting PF, Rutjes AWS, Westwood ME, Mallett S, Deeks JJ, Reitsma JB, et al. QUADAS-2: a revised tool for the quality assessment of diagnostic accuracy studies. Ann Intern Med. (2011) 155:529–36. doi: 10.7326/0003-4819-155-8-201110180-00009
35. Wishart DS, Guo A, Oler E, Wang F, Anjum A, Peters H, et al. HMDB 5.0: the human metabolome database for 2022. Nucleic Acids Res. (2022) 50:D622–31. doi: 10.1093/nar/gkab1062
36. Smith L, Villaret-Cazadamont J, Claus SP, Canlet C, Guillou H, Cabaton NJ, et al. Important considerations for sample collection in metabolomics studies with a special focus on applications to liver functions. Metabolites. (2020) 10:E104. doi: 10.3390/metabo10030104
37. Muhlebach MS, Sha W. Lessons learned from metabolomics in cystic fibrosis. Mol Cell Pediatr. (2015) 2:9. doi: 10.1186/s40348-015-0020-8
38. Xiao JF, Zhou B, Ressom HW. Metabolite identification and quantitation in LC-MS/MS-based metabolomics. Trends Anal Chem TRAC. (2012) 32:1–14. doi: 10.1016/j.trac.2011.08.009
39. Churchwell MI, Twaddle NC, Meeker LR, Doerge DR. Improving LC–MS sensitivity through increases in chromatographic performance: comparisons of UPLC–ES/MS/MS to HPLC–ES/MS/MS. J Chromatogr B. (2005) 825:134–43. doi: 10.1016/j.jchromb.2005.05.037
40. Marchev AS, Vasileva LV, Amirova KM, Savova MS, Balcheva-Sivenova ZP, Georgiev MI. Metabolomics and health: from nutritional crops and plant-based pharmaceuticals to profiling of human biofluids. Cell Mol Life Sci. (2021) 78:6487–503. doi: 10.1007/s00018-021-03918-3
41. Gowda GAN, Djukovic D. Overview of mass spectrometry-based metabolomics: opportunities and challenges. Methods Mol Biol Clifton NJ. (2014) 1198:3–12. doi: 10.1007/978-1-4939-1258-2_1
42. Hloch O, Fila L, Havlin J, Palova S, Charvat J. The changes of plasma amino acids and lipid profiles in patients with cystic fibrosis and malnutrition induced by immunonutrition. J Nutr Disord Ther. (2018) 8:239. doi: 10.4172/2161-0509.1000239
43. Chideckel EW, Fedan JS, Mike P. Polyamines and putreanine relax respiratory tract smooth muscle in the guinea-pig. Eur J Pharmacol. (1985) 116:187–90. doi: 10.1016/0014-2999(85)90203-1
44. Zheng X, Xie G, Zhao A, Zhao L, Yao C, Chiu NHL, et al. The footprints of gut microbial–mammalian co-metabolism. J Proteome Res. (2011) 10:5512–22. doi: 10.1021/pr2007945
45. Carmody LA, Caverly LJ, Foster BK, Rogers MAM, Kalikin LM, Simon RH, et al. Fluctuations in airway bacterial communities associated with clinical states and disease stages in cystic fibrosis. PLoS ONE. (2018) 13:e0194060. doi: 10.1371/journal.pone.0194060
46. Grasemann H, Klingel M, Avolio J, Prentice C, Gonska T, Tullis E, et al. Long-term effect of CFTR modulator therapy on airway nitric oxide. Eur Respir J. (2020) 55:1901113. doi: 10.1183/13993003.01113-2019
47. Committee Committee on the Review of Omics-Based Tests for Predicting Patient Outcomes in Clinical Trials Board Board on Health Care Services Board Board on Health Sciences Policy Institute Institute of Medicine. Evolution of Translational Omics: Lessons Learned and the Path Forward. Micheel CM, Nass SJ, Omenn GS, editors. Washington, DC: National Academies Press (2012). Available online at: http://www.ncbi.nlm.nih.gov/books/NBK202168/ (accessed March 14, 2022).
Keywords: metabolomics, biomarkers, systematic review, respirology, cystic fibrosis
Citation: Nguyen A-LV, Haas D, Bouchard M and Quon BS (2022) Metabolomic Biomarkers to Predict and Diagnose Cystic Fibrosis Pulmonary Exacerbations: A Systematic Review. Front. Pediatr. 10:896439. doi: 10.3389/fped.2022.896439
Received: 15 March 2022; Accepted: 12 May 2022;
Published: 31 May 2022.
Edited by:
Kristin Skogstrand, Statens Serum Institute, DenmarkReviewed by:
Arturo Solis-Moya, Dr. Carlos Sáenz Herrera National Children's Hospital, Costa RicaAndrea Coverstone, Washington University in St. Louis, United States
Samin Hamidi, Tabriz University of Medical Sciences, Iran
Copyright © 2022 Nguyen, Haas, Bouchard and Quon. This is an open-access article distributed under the terms of the Creative Commons Attribution License (CC BY). The use, distribution or reproduction in other forums is permitted, provided the original author(s) and the copyright owner(s) are credited and that the original publication in this journal is cited, in accordance with accepted academic practice. No use, distribution or reproduction is permitted which does not comply with these terms.
*Correspondence: Bradley S. Quon, QnJhZGxleS5RdW9uJiN4MDAwNDA7aGxpLnViYy5jYQ==