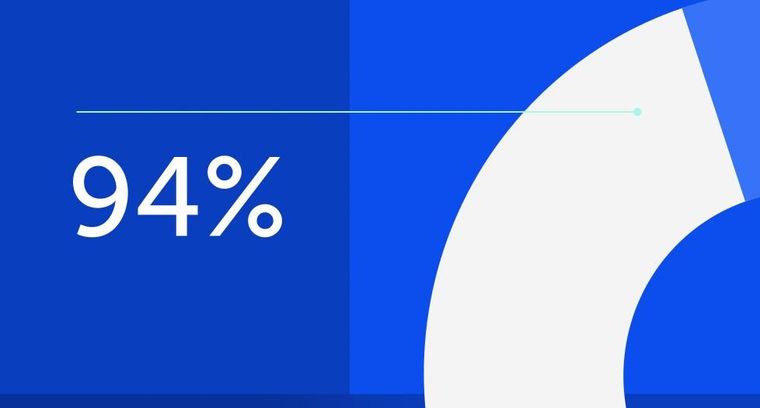
94% of researchers rate our articles as excellent or good
Learn more about the work of our research integrity team to safeguard the quality of each article we publish.
Find out more
MINI REVIEW article
Front. Pediatr., 03 June 2022
Sec. Pediatric Nephrology
Volume 10 - 2022 | https://doi.org/10.3389/fped.2022.893002
This article is part of the Research TopicWomen In Pediatric Nephrology: 2021View all 4 articles
Kidney transplant is the optimal treatment for end-stage kidney disease as it offers significant survival and quality of life advantages over dialysis. While recent advances have significantly improved early graft outcomes, long-term overall graft survival has remained largely unchanged for the last 20 years. Due to the young age at which children receive their first transplant, most children will require multiple transplants during their lifetime. Each subsequent transplant becomes more difficult because of the development of de novo donor specific HLA antibodies (dnDSA), thereby limiting the donor pool and increasing mortality and morbidity due to longer time on dialysis awaiting re-transplantation. Secondary prevention of dnDSA through increased post-transplant immunosuppression in children is constrained by a significant risk for viral and oncologic complications. There are currently no FDA-approved therapies that can meaningfully reduce dnDSA burden or improve long-term allograft outcomes. Therefore, primary prevention strategies aimed at reducing the risk of dnDSA formation would allow for the best possible long-term allograft outcomes without the adverse complications associated with over-immunosuppression. Epitope matching, which provides a more nuanced assessment of immunological compatibility between donor and recipient, offers the potential for improved donor selection. Although epitope matching is promising, it has not yet been readily applied in the clinical setting. Our review will describe current strengths and limitations of epitope matching software, the evidence for and against improved outcomes with epitope matching, discussion of eplet load vs. variable immunogenicity, and conclude with a discussion of the delicate balance of improving matching without disadvantaging certain populations.
• Epitope matching between donor and recipients provides additional benefit over antigen-level matching.
• Numerous software programs are available for comparison but require high-resolution tissue typing for accurate results.
• Enumeration of mismatch does not account for variable immunogenicity of epitopes.
Kidney transplant (KT) is the optimal treatment for end-stage kidney disease (ESKD) as it offers significant mortality and quality of life advantages over dialysis. While advances in immunosuppressive pharmacology and surgical techniques have significantly improved early graft outcomes, long-term graft survival has remained largely unchanged for the last 20 years. Due to the young age at which children receive their first transplant and additional 40–60 year life expectancy, children will require multiple transplants during their lifetime. Development of de novo donor specific antibodies (dnDSA) is associated with shortened graft survival making each subsequent transplant more difficult by limiting the donor pool and increasing mortality due to longer time on dialysis awaiting re-transplantation (1, 2).
Reducing the formation of dnDSA can be achieved through secondary prevention by increasing post-transplant immunosuppression. However, this approach is limited in children by a significantly increased risk for viral and oncologic complications (3–5). Additionally, there is evidence showing improvement in cardiovascular risk factors (blood pressure and lipids) and improved growth, with comparable rates of acute rejection and graft survival, for children on steroid-free and reduced intensity immunosuppression protocols (6–8). There are currently no FDA-approved therapies that have been shown to meaningfully reduce dnDSA burden or improve long-term allograft outcomes (9–11). Primary prevention strategies aimed at reducing the risk of dnDSA formation would allow for the best possible long-term allograft outcomes without the adverse complications from over-immunosuppression. One mechanism by which this can be achieved is by increasing precision of immunological matching at the time of transplant.
Traditional immunological matching has been done with a comparison of whole molecule HLA characterization at 3 loci (A, B, and DR). As the methods of accurate HLA characterization improved, it became clear that different HLA alleles had variable number of amino acid differences ranging from one to many, depending on the compared alleles. Simply enumerating HLA allele mismatches was insufficient to account for structural differences with immunological significance. Eventually, NGS (Next Generation Sequencing), a method that produces accurate genotyping at the molecular level, enabled improved characterization of HLA molecules by identifying the amino acid differences among multiple HLA alleles/molecules. The term “high-resolution tissue typing” or two-field (HR-2F) typing has been used to describe the level of characterization that enables identification of all 11 HLA antigens at the protein/molecular level, and thereby allows an epitope compatibility comparison between the donor and recipient (12). It should be clarified that the term “epitope” is used to describe any amino acid differences between two HLA molecules, whether recognizable by an antibody (Ab) or not, while the term “eplet” was coined by Rene Duquesnoy to indicate the structural elements (clusters of 2-5 polymorphic amino acids), linear or conformational on an HLA molecule recognized by an anti-HLA antibody (Figure 1) (13, 14). Comparison of eplets/epitopes, rather than whole HLA molecules, enables assessment of immunological compatibility with significantly more detail. Previous epitope/eplet mismatch (EMM) studies have reported an association of epitope/eplet load with allograft outcomes, rejection, and the formation of dnDSA (15, 16). Compared to low-resolution HLA matching, EMM predicted an incremental risk of HLA sensitization for increasing number of mismatches and offers additional value in predicting dnDSA formation (17, 18).
Figure 1. Model of HLA binding groove. The yellow shows a single polymorphic amino acid change. The red shows an antibody-verified eplet. The green shows the entire antibody interaction area which can contain the surface area for antibody-epitope interaction.
This review describes the different software tools currently available for comparison of high-resolution tissue typing, their predictive capability and clinical applicability, and discusses the nuances of variable immunogenicity. Practice applications of this technology, limitations and remaining knowledge gaps will also be also addressed.
To support informed clinical decision making, the comparison of donor and recipient HLA types relies on various software applications to identify immunological similarities and differences between pairs. While each has their own approach, the general principle employed by all is that a stronger recipient T/B-cell response is more likely to occur the more different a donor is from the recipient, and therefore the software generates a score to quantify the dissimilarity between the donor and recipient (19). There are currently three commonly internationally used software programs: HLAMatchmaker, single amino acid MM (AMS), and PIRCHE. Each program is described here with emphasis on advantages and disadvantages. The programs are compared in Table 1.
Table 1. Comparison of the three commonly utilized software packages utilized for comparison of donor and recipient HLA types in solid organ transplantation.
HLAMatchmaker, the first developed and most common software, was developed by Rene Duquesnoy in 2002 (20). It is a structurally based matching program based on the understanding that only a portion of the HLA antigen is recognized by HLA antibodies. HLAMatchmaker uses donor/recipient amino acid sequences to determine continuous and discontinuous eplets that are likely part of the area recognized by an antibody's complementary-determining region. HLAMatchmaker focuses only on polymorphic regions and provides information regarding “verified” and “non-verified” by antibody reactivity epitopes. All mismatched eplets are assigned the same value for a sum of eplet mismatch load (19, 21). The number of eplets that are mismatched between donor and recipients are calculated by the software either at each HLA locus by class (I or II), or as a cumulative number. The HLAMatchmaker software has been well validated and has been shown to predict both dnDSA formation, rejection, and graft failure rates (20, 22–24). Given the nature of the software, the “non-verified” epitopes represent mismatched elements that may potentially produce antibodies but have not been proven to do so; therefore, the need for the broader term “epitope” which does not need Ab reactivity verification. Thus, two donor/recipient pairs may have similar absolute numbers of epitope mismatches, but may vary substantially in their impact on antibody production (21). In addition, the HLAMatchmaker site must be continually updated as more epitopes become antibody verified. The last update of the program at the time of this publication was July 2020.
The simplest method is comparison of donor and recipient amino acid sequences, enumerating the number of mismatches generating an amino acid mismatch score (AMS). Akin to eplet mismatch in the HLAMatchmaker program, this method assigns an equal weight to all mismatches but does not make any assumptions about which areas of the molecule may be more relevant for antibody recognition (19). In multivariable models, the odds ratio for developing DSA increased for every 10 amino acid mismatches, with a larger effect size for Class II than Class I DSA, however both were statistically significant (17).
The assessment of various physicochemical parameters may augment the AMS metric, and there have been numerous approaches including Grantham's distance or Epstein coefficient of difference (25, 26). These measures are calculations of amino acid (aa) properties that seek to provide a quantitative measure of the physicochemical change associated with specific aa substitutions in proteins. Even though these are rather simplified models, they are most likely more informative than simple aa mismatch enumeration. More specifically assessing protein to protein differences using physicochemical characteristics have been adopted with some degree of success showing some benefit over simple enumeration (13, 27, 28).
There remains much potential and promising work in methods that seek to incorporate three-dimensional (3D) contextual information into MM scoring systems (29, 30).
The complex immune alloreactivity after solid organ transplantations involves both T and B cells. Indirect T cell activation is proposed as a key player in the humoral response leading to dnDSA formation (31). The PIRCHE algorithm identifies which mismatched HLA epitopes can be recognized by T cells needed to activate the B-cell response. The output from the software includes a score which predicts the risk of DSA formation. Studies have found good correlation between PIRCHE score, DSA formation, and allograft outcome (32–34).
A recent comparative study among KT recipients EMM, AMS, and AMS as augmented by electrostatic mismatch showed that each method was independently associated with DSA formation after adjustment for recipient age, immunosuppression regiment, and non-adherence without evidence of superiority of either approach (35). Meneghini et al. compared the 3 methods of analysis among a cohort of 169 KT recipients and found a strong correlation between the three software scores, however only the PIRCHE score was found to be an independent predictor of donor-specific T-cell response in multivariable analysis, as would be expected (36). Similarly, in a study of 2,787 transplants PIRCHE-II and HLAMatchmaker were both found to independently predict DSA formation in multivariable analysis (controlling for the other method) suggesting that these approaches may complement each other (37). Combining the software approaches and understanding the immune alloreactivity mechanism where both T and B cells are involved, will likely be the most informative approach. Efforts to combine them have already been initiated and presented at the most recent meeting of America Society of Histocompatibility and Immunogenetics (38).
It is important to understand that despite the improved computational analysis software there are limitations. The needed HR-2F typing is available in living donation but not in deceased donors. The NGS technologies until recently did not allow a turn-around time of <7 h for HR-2F, however recent advances have taken a large leap towards ameliorating this limitation (39, 40). To address this problem our community has attempted to impute HR-2F HLA typing utilizing low or even intermediate level typing and an NMDP database called HaploStats or even HLAMatchmaker. Engen et al., studied the accuracy of imputation techniques and showed that only 35.6% of the imputed haplotypes had no mistakes, and HLAMatchmaker was not able to provide high-resolution haplotypes for 45.2% of Caucasian subjects and 63.5% of non-Caucasian subjects (p = 0.002) (41). This study reinforces that bypassing direct and accurate characterization of HLAs at the HR-2F introduces significant bias into the analysis by currently available computational software.
Epitope/eplet based matching has been suggested as a more precise strategy to improve immunologic outcomes with reduced formation of dnDSA compared to antigen-level matching (13, 42–46). It is known that chronic antibody-mediated rejection (ABMR), due to dnDSA is the major cause of late graft loss (15). DSA directed against HLA antigens are central to the development of ABMR. It was also found that formation of dnDSA vary and depend on the number and type of amino acid mismatches between donor and recipient, the electrostatic effect of these changes and the conformational differences in epitopes (47). The important question is what evidence exists correlating using epitope matching to improve clinical outcomes, specifically reducing rejection rates and graft loss.
Philogene et al. demonstrated in a cross-sectional study of 110 racially diverse pediatric KT recipients that an HLA class I eplet load >70 resulted in a greater risk of rejection compared to same race recipient and donor (48). A second retrospective analysis of 151 living donor KT recipients in a European cohort evaluated incidence of ABMR in patients transplanted with low, moderate and high HLA-II epitope mismatch load. ABMR incidence rates were 2, 14, and 13%, respectively, at 96 months (p = 0.036). A sub-analysis including only patients with no preformed DSA (n = 138) demonstrated similar results (49).
Sapir-Pichhadze et al. studied a large American cohort of 118,382 first KT recipients and assessed death censored graft failure based on antibody verified EMM. Antibody-verified eplet mismatches were found to be independent predictors of death-censored graft failure (50). Their team validated the results in an independent Canadian cohort of 52 patients with transplant glomerulopathy and 104 controls, with similar findings. Collectively, these results support donor-recipient matching for specific HLA eplets to reduce the risk of graft loss.
In adult kidney transplantation there is a growing body of literature showing increased risk for DSA formation, ABMR, and allograft failure with increasing eplet/epitope mismatch score (13, 16, 51, 52). In children, there is less published literature, however, most research implementing EMM in pediatric KT has focused on eplet load, an enumeration of eplets mismatched between the donor and recipient (48, 53, 54). Eplet load has been linked to adverse allograft outcomes including acute rejection, allograft loss, and transplant glomerulopathy (50, 51, 55).
Despite the ample evidence for superior ability to risk stratify and predict adverse outcomes by optimizing matching with eplet load testing, perfect immunological matching at the allele or eplet level is impractical. Over-prioritization of HLA matching results in prolonged waitlist time and must be balanced with the morbidity and mortality of advanced CKD and dialysis including increased risk for cardiovascular disease (56, 57). The prolonged waitlist time is unequally burdensome and disadvantages patients from racial and ethnic minority populations who express rare HLA genotypes (58, 59).
Eplet load does not account for the electrostatic and conformational characteristics that result in variable antigen immunogenicity (17, 60). The concept that some eplets are more immunogenic than others was explored in a retrospective study in heart and lung transplant recipients; specific eplet mismatches were found to be disproportionally associated with dnDSA formation and modeling avoidance of these high-risk eplets predicted a significant reduction in dnDSA formation (43). Using imputation to determine HR-2F HLA type in the Scientific Registry of Transplant Recipients (SRTR), a subset of 15 eplet mismatches was linked to a higher hazard of death-censored graft failure among KT recipients, even after accounting for other known risk factors (61, 62). Additionally, a small subset of Ab-verified eplets have been linked to transplant glomerulopathy in an independent Canadian KT cohort (50). These innovative studies are laying the foundation for incorporating variable immunogenicity into the decision framework for donor allocation and acceptance. One major limitation of these studies is the use of imputation to determine HLA type, which is prone to error especially in racial and ethnic minorities (63). The authors appropriately identified this limitation and used sensitivity analysis to compare findings in Caucasian patients with those of other races with similar results.
Just as not all eplets are equally immunogenic, not all DSA are equally pathogenic and as such, eplet load is insufficient to inform risk stratification for DSA most likely to lead to adverse allograft outcomes (13, 16, 64, 65). A novel approach to study the variable immunogenicity of HLA-DQ eplets was designed by Schawalder et al., utilizing pregnancy as the immunological trigger, allowing a study of variable immunogenicity without the need to account for medication non-adherence and other significant post-transplant complications (66). The team identified several DQB1 and DQA1 eplets with higher immunogenicity scores in a primarily Caucasian study cohort, however these findings still need to be confirmed in a broader, more ethnically diverse cohort and then applied to a robust transplant cohort to examine the immunomodulatory impact of immunosuppression on the host response. The Schawalder study also highlighted the challenges of studying variable immunogenicity as the immune response is often complex and assigning antibody specificity to a specific eplet when multiple mismatched eplets on the same HLA molecule are present is fraught with bias. Tambur et al. studied a cohort of KT recipients with 2 DQ mismatches where dnDSA was generated to only 1 of the DQ antigens (2MM1DSA cohort) and showed significant inconsistency with eplet load, affirming that EMM is a significant correlate of rejection at the population level however is not sufficiently informative to serve as a predictor at the individual level (64). A recently described contributor to immunogenicity is the degree of expression of each HLA antigen, adding another level of complexity in assessing immunological risk. In a study of 103 pediatric solid organ transplant donor-recipient pairs, Shieh et al. showed DPB1 expression level was independently associated with DSA formation and EMM lost statistical significance in a multivariable regression model (67).
Implementation of EMM analysis has the potential to greatly improve the precision with which donors and recipients are matched, which can be especially beneficial for highly sensitized patients (68, 69). The principle hurdle to implementation of EMM analysis in deceased donor kidney allocation is the time necessary to perform high resolution tissue typing.
Until recently, most HLA laboratories used probe-based methods including sequence specific primer (SSP) and sequence-specific oligonucleotide prove typing (SSO) which generates low-intermediate resolution typing at the antigen level, and unable to provide the necessary allele-level specificity to allow EMM analysis. Exact genomic sequencing either through Sanger sequence-based typing (SBT) or Next-Generation sequencing (NGS), is gradually becoming the prevalent method for typing as laboratories seek to transition to a single assay for solid organ and bone marrow transplant programs. The major limitation to NGS is the 2–3 days needed for the assay and associated analysis.
In living donor KT, where the time to perform high-resolution typing is not a barrier, there are great possibilities for the application of this technology. The Australian Paired Kidney Exchange employs high-resolution typing and EMM score, utilizing this as a tool to aid in donor selection in addition to other pertinent clinical factors, and has described excellent outcomes in pediatric patients (70). The National Kidney Registry in the United States has started collecting data on EMM between donors and recipients, although no publication is yet available to compare the impact of adding EMM into donor selection considerations.
While the time element remains the main barrier to implementation of this methodology in deceased donor allocation on a large scale, several pilot and small-scale studies have shown benefit for pediatric KT recipients. Starting in January 2014, all patients listed for DDKT in Australia had SSO typing with imputation of alleles entered into the allocation program, and for each patient eplet load thresholds as well as unacceptable matches were pre-determined at the time of listing (53). Utilizing this method, children received DDKT with lower eplet loads compared to the previous allocation scheme, having an exceptionally significant impact on decreasing Class II load [Class II EMM 20.4 (5.4) vs. 63.7 (16.2), p < 0.001], however the study was limited by very small sample size. A pediatric center reported their practice of incorporating Class II EMM prospectively in 19 pediatric patients and showed that the adult reported DR and DQ eplet load thresholds may not be the same for pediatric patients. The paper reported only short-term outcomes and further data is needed to assess the impact on DSA formation and allograft survival (71). Recently, a novel nanopore sequencing technology was reported that can produce reliable, cost-effective, and rapid (<6 h) HR-2F typing (39, 40). While not yet ready for full-time clinical use, the ongoing development of such technologies will enable incorporation of EMM analysis into deceased donor kidney allocation.
Despite the promise of better immunological matching with eplet-based matching, we must be cognizant for the potential to worsen racial and ethnic disparities with over-prioritization of HLA matching. Prior to 2014, HLA matching was prioritized in deceased donor allocation in order to optimize long-term allograft survival. However, as non-White patients express rare HLA types, the likelihood of finding an HLA-matched donor for racial and ethnic minority patients is significantly reduced (58, 59). The 2014 Kidney Allocation System (KAS) deprioritized HLA matching and has narrowed the disparity in waitlist time among Black and Hispanic candidates and improved their transplant rates (72, 73). Unfortunately, the same policy had the unintended consequence of prolonging pediatric waitlist times, highlighting the need for critical appraisal that policy change can have on clinical outcomes (74–76). Because organ allocation is an entangled dynamic system, in which change to one factor can significantly impact the others, prioritization of only one element often fails and highlights the pressing need to develop an allocation model that can optimize immunological matching but that can preserve pediatric priority, as well as minimize racial and geographic disparities (77, 78).
In the United States, the Organ Procurement and Transplantation Network (OPTN) is reviewing allocation policy and strategies to improve equity with a proposal to create a composite allocation score in which candidates would get a summary score of different variables associated with their predicted need and potential for survival benefit, including factors such as age (pediatric priority), geographic proximity to donor, and sensitization. These models are under development and will require close monitoring after implementation. More data are needed to inform approaches to optimize matching for children and one approach might include a focus on class II matching (79).
Epitope/eplet matching holds the promise of better immunological compatibility between donors and recipients thereby reducing risk for DSA formation. Despite many advances, many questions remain including the specific mechanisms of variable immunogenicity, technological barriers to rapid high-resolution molecular tissue typing, and balancing equity in an allocation system. The community however, is eager to use this approach, simply because molecular characterization is more meaningful than an allele name. Nevertheless, there remains considerable ambiguity in how to balance epitope mismatch with clinical factors including medical urgency (dialysis duration or limited access), age, psychosocial factors influencing adherence, and viral infection risk. It is very likely that the appropriate science and tools will soon be available for utilization and application, but additional studies are needed to identify the most efficient and effective approach to optimize outcomes.
OC developed the conceptual overview for the review. OC and DLE wrote the review and participated in critical review and revisions. SA and DM provided critical review and guidance. All authors approved the final version of the manuscript.
This work was supported by (grant number KL2TR003099 to OC) from Johns Hopkins Institute for Clinical and Translational Research (ICTR).
DM is a consultant to and owns options in Omixon. DM receive royalties from Omixon.
The remaining authors declare that the research was conducted in the absence of any commercial or financial relationships that could be construed as a potential conflict of interest.
All claims expressed in this article are solely those of the authors and do not necessarily represent those of their affiliated organizations, or those of the publisher, the editors and the reviewers. Any product that may be evaluated in this article, or claim that may be made by its manufacturer, is not guaranteed or endorsed by the publisher.
1. Foster BJ, Dahhou M, Zhang X, Platt RW, Smith JM, Hanley JA. Impact of HLA mismatch at first kidney transplant on lifetime with graft function in young recipients. Am J Transplant. (2014) 14:876–85. doi: 10.1111/ajt.12643
2. Loupy A, Hill GS, Jordan SC. The impact of donor-specific anti-HLA antibodies on late kidney allograft failure. Nat Rev Nephrol. (2012) 8:348–57. doi: 10.1038/nrneph.2012.81
3. Smith JM, Dharnidharka VR. Viral surveillance and subclinical viral infection in pediatric kidney transplantation. Pediatr Nephrol. (2015) 30:741–8. doi: 10.1007/s00467-014-2866-8
4. Hogan J, Pietrement C, Sellier-Leclerc AL, Louillet F, Salomon R, Macher MA, et al. Infection-related hospitalizations after kidney transplantation in children: incidence, risk factors, and cost. Pediatr Nephrol. (2017) 32:2331–41. doi: 10.1007/s00467-017-3737-x
5. Ploos van Amstel S, Vogelzang JL, Starink MV, Jager KJ, Groothoff JW. (2015). Long-term risk of cancer in survivors of pediatric ESRD. Clin J Am Soc Nephrol. 10:2198–204. doi: 10.2215/CJN.03630415
6. Supe-Markovina K, Melquist JJ, Connolly D, DiCarlo HN, Waltzer WC, Fine RN, et al. Alemtuzumab with corticosteroid minimization for pediatric deceased donor renal transplantation: a seven-yr experience. Pediatr Transplant. (2014) 18:363–8. doi: 10.1111/petr.12253
7. Sarwal MM, Ettenger RB, Dharnidharka V, Benfield M, Mathias R, et al. Complete steroid avoidance is effective and safe in children with renal transplants: a multicenter randomized trial with three-year follow-up. Am J Transplant. (2012) 12:2719–29. doi: 10.1111/j.1600-6143.2012.04145.x
8. Wojciechowski D, Chandran S, Yang JYC, Sarwal MM, Vincenti F. (2017). Retrospective evaluation of the efficacy and safety of belatacept with thymoglobulin induction and maintenance everolimus: a single-center clinical experience. Clin Transplant. 31:13042. doi: 10.1111/ctr.13042
9. Cioni M, Nocera A, Tagliamacco A, Basso S, Innocente A, Fontana I, et al. Failure to remove de novo donor-specific HLA antibodies is influenced by antibody properties and identifies kidney recipients with late antibody-mediated rejection destined to graft loss - a retrospective study. Transpl Int. (2019) 32:38–48. doi: 10.1111/tri.13325
10. Charnaya O, Tuchman S, Moudgil A. Results of early treatment for de novo donor-specific antibodies in pediatric kidney transplant recipients in a cross-sectional and longitudinal cohort. Pediatr Transplant. (2018) 22:13108. doi: 10.1111/petr.13108
11. Kizilbash S, Claes D, Ashoor I, Chen A, Jandeska S, Matar RB, et al. Bortezomib in the treatment of antibody-mediated rejection in pediatric kidney transplant recipients: a multicenter Midwest Pediatric Nephrology Consortium study. Pediatr Transplant. (2017) 21:12873. doi: 10.1111/petr.12873
12. Huang Y, Dinh A, Heron S, Gasiewski A, Kneib C, Mehler H, et al. Assessing the utilization of high-resolution 2-field HLA typing in solid organ transplantation. Am J Transplant. (2019) 19:1955–63. doi: 10.1111/ajt.15258
13. Wiebe C, Kosmoliaptsis V, Pochinco D, Gibson IW, Ho J, Birk PE, et al. HLA-DR/DQ molecular mismatch: a prognostic biomarker for primary alloimmunity. Am J Transplant. (2019) 19:1708–19. doi: 10.1111/ajt.15177
14. Tambur AR. HLA-epitope matching or eplet risk stratification: the devil is in the details. Front Immunol. (2018) 9:e02010. doi: 10.3389/fimmu.2018.02010
15. Wiebe C, Pochinco D, Blydt-Hansen TD, Ho J, Birk PE, Karpinski M, et al. Class II HLA epitope matching-A strategy to minimize de novo donor-specific antibody development and improve outcomes. Am J Transplant. (2013) 13:3114–22. doi: 10.1111/ajt.12478
16. Senev A, Coemans M, Lerut E, Van Sandt V, Kerkhofs J, Daniëls L, et al. Eplet mismatch load and de novo occurrence of donor-specific anti-hla antibodies, rejection, and graft failure after kidney transplantation: an observational cohort study. J Am Soc Nephrol. (2020) 31:2193–204. doi: 10.1681/ASN.2020010019
17. Kosmoliaptsis V, Mallon DH, Chen Y, Bolton EM, Bradley JA, Taylor CJ. Alloantibody responses after renal transplant failure can be better predicted by donor-recipient HLA amino acid sequence and physicochemical disparities than conventional HLA matching. Am J Transplant. (2016) 16:2139–47. doi: 10.1111/ajt.13707
18. Tafulo S, Malheiro J, Santos S, Dias L, Almeida M, Martins LS, et al. HLA class II eplet mismatch load improves prediction of dnDSA development after living donor kidney transplantation. Int J Immunogenet. (2021) 48:1–7. doi: 10.1111/iji.12519
19. Tambur AR, Campbell P, Chong AS, Feng S, Ford ML, Gebel H, et al. Sensitization in transplantation: assessment of risk (STAR) 2019 working group meeting report. Am J Transplant. (2020) 20:2652–68. doi: 10.1111/ajt.15937
20. Duquesnoy RJ. HLAMatchmaker: a molecularly based algorithm for histocompatibility determination. I Description of the algorithm. Hum Immunol. (2002) 63:339–52. doi: 10.1016/S0198-8859(02)00382-8
21. Kramer CSM, Koster J, Haasnoot GW, Roelen DL, Claas FHJ, Heidt S. HLA-EMMA: a user-friendly tool to analyse HLA class I and class II compatibility on the amino acid level. Hla. (2020) 96:43–51. doi: 10.1111/tan.13883
22. Duquesnoy RJ, Askar M. HLAMatchmaker: a molecularly based algorithm for histocompatibility determination. V. Eplet matching for HLA-DR, HLA-DQ, and HLA-DP. Hum Immunol. (2007) 68:12–25. doi: 10.1016/j.humimm.2006.10.003
23. Duquesnoy RJ. A structurally based approach to determine HLA compatibility at the humoral immune level. Hum Immunol. (2006) 67:847–62. doi: 10.1016/j.humimm.2006.08.001
24. Duquesnoy RJ, Witvliet M, Doxiadis II, de Fijter H, Claas FH. HLAMatchmaker-based strategy to identify acceptable HLA class I mismatches for highly sensitized kidney transplant candidates. Transpl Int. (2004) 17:22–30. doi: 10.1111/j.1432-2277.2004.tb00379.x
25. Grantham R. Amino acid difference formula to help explain protein evolution. Science. (1974) 185:862–4. doi: 10.1126/science.185.4154.862
26. Epstein CJ. Non-randomness of ammo-acid changes in the evolution of homologous proteins. Nature. (1967) 215:355–9. doi: 10.1038/215355a0
27. Kosmoliaptsis V, Chaudhry AN, Sharples LD, Halsall DJ, Dafforn TR, Bradley JA, et al. Predicting HLA class I alloantigen immunogenicity from the number and physiochemical properties of amino acid polymorphisms. Transplantation. (2009) 88:791–8. doi: 10.1097/TP.0b013e3181b4a9ff
28. Kosmoliaptsis V, Sharples LD, Chaudhry AN, Halsall DJ, Bradley JA, Taylor CJ. Predicting HLA class II alloantigen immunogenicity from the number and physiochemical properties of amino acid polymorphisms. Transplantation. (2011) 91:183–90. doi: 10.1097/TP.0b013e3181ffff99
29. Ponomarenko J, Bui H-H, Li W, Fusseder N, Bourne PE, Sette A, et al. ElliPro: a new structure-based tool for the prediction of antibody epitopes. BMC Bioinformatics. (2008) 9:514. doi: 10.1186/1471-2105-9-514
30. Mallon DH, Kling C, Robb M, Ellinghaus E, Bradley JA, Taylor CJ, et al. Predicting humoral alloimmunity from differences in donor and recipient HLA surface electrostatic potential. J Immunol. (2018) 201:3780–92. doi: 10.4049/jimmunol.1800683
31. Geneugelijk K, Spierings E. PIRCHE-II: an algorithm to predict indirectly recognizable HLA epitopes in solid organ transplantation. Immunogenetics. (2020) 72:119–29. doi: 10.1007/s00251-019-01140-x
32. Geneugelijk K, Thus KA, Spierings E. Predicting alloreactivity in transplantation. J Immunol Res. (2014) 2014:159479. doi: 10.1155/2014/159479
33. PIRCHE. Available online at: https://www.pirche.com/pirche/#/ (accessed February 15, 2022).
34. Geneugelijk K, Niemann M, Drylewicz J, van Zuilen AD, Joosten I, Allebes WA, et al. PIRCHE-II is related to graft failure after kidney transplantation. Front Immunol. (2018) 9:321. doi: 10.3389/fimmu.2018.00321
35. Wiebe C, Kosmoliaptsis V, Pochinco D, Taylor CJ, Nickerson P. A comparison of HLA molecular mismatch methods to determine HLA immunogenicity. Transplantation. (2018) 102:1338–43. doi: 10.1097/TP.0000000000002117
36. Meneghini M, Crespo E, Niemann M, Torija A, Lloberas N, Pernin V, et al. Donor/recipient HLA molecular mismatch scores predict primary humoral and cellular alloimmunity in kidney transplantation. Front Immunol. (2020) 11:623276. doi: 10.3389/fimmu.2020.623276
37. Lachmann N, Niemann M, Reinke P, Budde K, Schmidt D, Halleck F, et al. Donor-recipient matching based on predicted indirectly recognizable HLA epitopes independently predicts the incidence of de novo donor-specific HLA antibodies following renal transplantation. Am J Transplant. (2017) 17:3076–86. doi: 10.1111/ajt.14393
38. Mangiola M, Ellison MA, Marrari M, Bentlejewski C, Sadowski J, Zern D, et al. Oral abstracts (OR): improved immunological risk stratification of pediatric heart trasnplant patients by combining PIRCHE-II with HLAMatchmaker or HLA-EMMA. Hum Immunol. (2021) 82:7–45. doi: 10.1016/j.healun.2022.03.015
39. Mosbruger TL, Dinou A, Duke JL, Ferriola D, Mehler H, Pagkrati I, et al. Utilizing nanopore sequencing technology for the rapid and comprehensive characterization of eleven HLA loci; addressing the need for deceased donor expedited HLA typing. Hum Immunol. (2020) 81:413–22. doi: 10.1016/j.humimm.2020.06.004
40. De Santis D, Truong L, Martinez P, D'Orsogna L. Rapid high-resolution HLA genotyping by MinION Oxford nanopore sequencing for deceased donor organ allocation. HLA. (2020) 96:141–62. doi: 10.1111/tan.13901
41. Engen RM, Jedraszko AM, Conciatori MA, Tambur AR. Substituting imputation of HLA antigens for high-resolution HLA typing: EVALUATION of a multiethnic population and implications for clinical decision making in transplantation. Am J Transplant. (2021) 21:344–52. doi: 10.1111/ajt.16070
42. Duquesnoy RJ. Are we ready for epitope-based HLA matching in clinical organ transplantation? Transplantation. (2017) 101:1755–65. doi: 10.1097/TP.0000000000001667
43. McCaughan JA, Battle RK, Singh SKS, Tikkanen JM, Moayedi Y, Ross HJ, et al. Identification of risk epitope mismatches associated with de novo donor-specific HLA antibody development in cardiothoracic transplantation. Am J Transplant. (2018) 18:2924–33. doi: 10.1111/ajt.14951
44. Wiebe C, Nickerson P. Strategic use of epitope matching to improve outcomes. Transplantation. (2016) 100:2048–52. doi: 10.1097/TP.0000000000001284
45. Everly MJ, Rebellato LM, Haisch CE, Ozawa M, Parker K, Briley KP, et al. Incidence and impact of de novo donor-specific alloantibody in primary renal allografts. Transplantation. (2013) 95:410–7. doi: 10.1097/TP.0b013e31827d62e3
46. Wiebe C, Nickerson PW. Human leukocyte antigen molecular mismatch to risk stratify kidney transplant recipients. Curr Opin Organ Transplant. (2020) 25:8–14. doi: 10.1097/MOT.0000000000000714
47. Sellarés J, de Freitas DG, Mengel M, Reeve J, Einecke G, Sis B, et al. Understanding the causes of kidney transplant failure: the dominant role of antibody-mediated rejection and nonadherence. Am J Transplant. (2012) 12:388–99. doi: 10.1111/j.1600-6143.2011.03840.x
48. Philogene MC, Amin A, Zhou S, Charnaya O, Vega R, Desai N, et al. Eplet mismatch analysis and allograft outcome across racially diverse groups in a pediatric transplant cohort: a single-center analysis. Pediatr Nephrol. (2020) 35:83–94. doi: 10.1007/s00467-019-04344-1
49. Tafulo S, Malheiro J, Santos S, Dias L, Almeida M, Martins S, et al. Degree of HLA class II eplet mismatch load improves prediction of antibody-mediated rejection in living donor kidney transplantation. Hum Immunol. (2019) 80:966–75. doi: 10.1016/j.humimm.2019.09.010
50. Sapir-Pichhadze R, Zhang X, Ferradji A, Madbouly A, Tinckam KJ, Gebel HM, et al. Epitopes as characterized by antibody-verified eplet mismatches determine risk of kidney transplant loss. Kidney Int. (2020) 97:778–85. doi: 10.1016/j.kint.2019.10.028
51. Sapir-Pichhadze R, Tinckam K, Quach K, Logan AG, Laupacis A, John R, et al. HLA-DR and -DQ eplet mismatches and transplant glomerulopathy: a nested case-control study. Am J Transplant. (2015) 15:137–48. doi: 10.1111/ajt.12968
52. Sakamoto S, Iwasaki K, Tomosugi T, Niemann M, Spierings E, Miwa Y, et al. Analysis of T and B cell epitopes to predict the risk of de novo donor-specific antibody (DSA) production after kidney transplantation: a two-center retrospective cohort study. Front Immunol. (2020) 11:2000. doi: 10.3389/fimmu.2020.02000
53. Kausman JY, Walker AM, Cantwell LS, Quinlan C, Sypek MP, Ierino FL. Application of an epitope-based allocation system in pediatric kidney transplantation. Pediatr Transplant. (2016) 20:931–8. doi: 10.1111/petr.12815
54. Sypek MP, Hiho S, Cantwell L, Clayton P, Hughes P, Le Page AK, et al. Human leukocyte antigen eplet mismatches and long-term clinical outcomes in pediatric renal transplantation: a pragmatic, registry-based study. Pediatr Transplant. (2020) 24:e13705. doi: 10.1111/petr.13705
55. Sharma A, Taverniti A, Graf N, Teixeira-Pinto A, Lewis JR, Lim WH, et al. The association between human leukocyte antigen eplet mismatches, de novo donor-specific antibodies, and the risk of acute rejection in pediatric kidney transplant recipients. Pediatr Nephrol. (2020) 35:1061–8. doi: 10.1007/s00467-020-04474-x
56. Vu LT, Baxter-Lowe LA, Garcia J, McEnhill M, Summers P, Hirose R, et al. HLA-DR matching in organ allocation: balance between waiting time and rejection in pediatric kidney transplantation. Arch Surg. (2011) 146:824–9. doi: 10.1001/archsurg.2011.147
57. Amaral S, Sayed BA, Kutner N, Patzer RE. Preemptive kidney transplantation is associated with survival benefits among pediatric patients with end-stage renal disease. Kidney Int. (2016) 90:1100–8. doi: 10.1016/j.kint.2016.07.028
58. Roberts JP, Wolfe RA, Bragg-Gresham JL, Rush SH, Wynn JJ, Distant DA, et al. Effect of changing the priority for HLA matching on the rates and outcomes of kidney transplantation in minority groups. N Engl J Med. (2004) 350:545–51. doi: 10.1056/NEJMoa025056
59. Wolfe RA, Ashby VB, Milford EL, Bloembergen WE, Agodoa LYC, Held PJ, et al. Differences in access to cadaveric renal transplantation in the United States. Am J Kidney Dis. (2000) 36:1025–33. doi: 10.1053/ajkd.2000.19106
60. Abbott RK, Crotty S. Factors in B cell competition and immunodominance. Immunol Rev. (2020) 296:120–31. doi: 10.1111/imr.12861
61. Mohammadhassanzadeh H, Oualkacha K, Zhang W, Klement W, Bourdiec A, Lamsatfi J, et al. On path to informing hierarchy of eplet mismatches as determinants of kidney transplant loss. Kidney Int Rep. (2021) 6:1567–79. doi: 10.1016/j.ekir.2021.03.877
62. Zahran S, Bourdiec A, Zhang X, Sapir-Pichhadze R. Not all eplet mismatches are created equal - A cohort study illustrating implications to long-term graft outcomes. Hum Immunol. (2022) 83:225–32. doi: 10.1016/j.humimm.2021.11.007
63. Engen RM, Jedraszko AM, Conciatori MA, Tambur AR, Substituting imputation of HLA antigens for high-resolution HLA typing: evaluation of a multiethnic population and implications for clinical decision making in transplantation. Am J Transplant.
64. Tambur AR, McDowell H, Hod-Dvorai R, Abundis MAC, Pinelli DF. The quest to decipher HLA immunogenicity: telling friend from foe. Am J Transplant. (2019) 19:2910–25. doi: 10.1111/ajt.15489
65. Wen J, Basu A, Bentall A, Henderson N, Dukek B, Gandhi M, et al. Is the level of HLA eplet mismatch a risk factor for graft loss among kidney transplant recipients who have already formed de novo donor specific antibody? Hum Immunol. (2021) 82:240–6. doi: 10.1016/j.humimm.2021.02.004
66. Schawalder L, Hönger G, Kleiser M, van Heck MR, van de Pasch LAL, Vendelbosch S, et al. Development of an immunogenicity score for HLA-DQ eplets: a conceptual study. HLA. (2021) 97:30–43. doi: 10.1111/tan.14110
67. Shieh M, Hayeck TJ, Dinh A, Duke JL, Chitnis N, Mosbruger T, et al. Complex linkage disequilibrium effects in HLA-DPB1 expression and molecular mismatch analyses of transplantation outcomes. Transplantation. (2021) 105:637–47. doi: 10.1097/TP.0000000000003272
68. Duquesnoy RJ, Howe J, Takemoto S. HLAmatchmaker: a molecularly based algorithm for histocompatibility determination. IV An alternative strategy to increase the number of compatible donors for highly sensitized patients. Transplantation. (2003) 75:889–97. doi: 10.1097/01.TP.0000055097.58209.83
69. Heidt S, Haasnoot GW, Witvliet MD, van der Linden-van Oevelen MJH, Kamburova EG, Wisse BW, et al. Allocation to highly sensitized patients based on acceptable mismatches results in low rejection rates comparable to nonsensitized patients. Am J Transplant. (2019) 19:2926–33. doi: 10.1111/ajt.15486
70. Sypek MP, Alexander SI, Cantwell L, Ierino FL, Ferrari P, Walker AM, et al. Optimizing outcomes in pediatric renal transplantation through the australian paired kidney exchange program. Am J Transplant. (2017) 17:534–41. doi: 10.1111/ajt.14041
71. Bryan CF, Chadha V, Warady BA. Donor selection in pediatric kidney transplantation using DR and DQ eplet mismatching: a new histocompatibility paradigm. Pediatr Transplant. (2016) 20:926–30. doi: 10.1111/petr.12762
72. Zhang X, Melanson TA, Plantinga LC, Basu M, Pastan SO, Mohan S, et al. Racial/ethnic disparities in waitlisting for deceased donor kidney transplantation 1 year after implementation of the new national kidney allocation system. Am J Transplant. (2018) 18:1936–46. doi: 10.1111/ajt.14748
73. Melanson TA, Hockenberry JM, Plantinga L, Basu M, Pastan S, Mohan S, et al. New kidney allocation system associated with increased rates of transplants among black and hispanic patients. Health Affairs (Project Hope). (2017) 36:1078–85. doi: 10.1377/hlthaff.2016.1625
74. Stewart DE, Kucheryavaya AY, Klassen DK, Turgeon NA, Formica RN, Aeder MI. Changes in deceased donor kidney transplantation one year after KAS implementation. Am J Transplant. (2016) 16:1834–47. doi: 10.1111/ajt.13770
75. Nazarian SM, Peng AW, Duggirala B, Gupta M, Bittermann T, Amaral S, et al. The kidney allocation system does not appropriately stratify risk of pediatric donor kidneys: implications for pediatric recipients. Am J Transplant. (2018) 18:574–9. doi: 10.1111/ajt.14462
76. Krissberg J, Kaufmann M, Gupta A, Bendavid E, Stedman M, Cheng X, et al. Racial disparities in pediatric kidney transplantation under the new kidney allocation system in the United States. Clin J Am Soc Nephrol. (2021) 16:1862–71. doi: 10.2215/CJN.06740521
77. Wood NL, Mogul DB, Perito ER, VanDerwerken D, Mazariegos GV, Hsu EK, et al. Liver simulated allocation model does not effectively predict organ offer decisions for pediatric liver transplant candidates. Am J Transplant. (2021) 21:3157–62. doi: 10.1111/ajt.16621
78. Karami F, Kernodle AB, Ishaque T, Segev DL, Gentry SE. Allocating kidneys in optimized heterogeneous circles. Am J Transplant. (2021) 21:1179–85. doi: 10.1111/ajt.16274
Keywords: kidney transplant, epitope, eplet, pediatric, donor specific antibodies
Citation: Charnaya O, Levy Erez D, Amaral S and Monos DS (2022) Pediatric Kidney Transplantation—Can We Do Better? The Promise and Limitations of Epitope/Eplet Matching. Front. Pediatr. 10:893002. doi: 10.3389/fped.2022.893002
Received: 09 March 2022; Accepted: 16 May 2022;
Published: 03 June 2022.
Edited by:
Katherine Twombley, Medical University of South Carolina, United StatesReviewed by:
Nicole Hayde, Children's Hospital at Montefiore, United StatesCopyright © 2022 Charnaya, Levy Erez, Amaral and Monos. This is an open-access article distributed under the terms of the Creative Commons Attribution License (CC BY). The use, distribution or reproduction in other forums is permitted, provided the original author(s) and the copyright owner(s) are credited and that the original publication in this journal is cited, in accordance with accepted academic practice. No use, distribution or reproduction is permitted which does not comply with these terms.
*Correspondence: Olga Charnaya, b2NoYXJuYTFAamhtaS5lZHU=
Disclaimer: All claims expressed in this article are solely those of the authors and do not necessarily represent those of their affiliated organizations, or those of the publisher, the editors and the reviewers. Any product that may be evaluated in this article or claim that may be made by its manufacturer is not guaranteed or endorsed by the publisher.
Research integrity at Frontiers
Learn more about the work of our research integrity team to safeguard the quality of each article we publish.