- 1Geisinger Obesity Institute, Danville, PA, United States
- 2Population Health Sciences, Geisinger, Danville, PA, United States
- 3Nutrition and Weight Management, Geisinger Medical Center, Danville, PA, United States
- 4Nutritional Sciences, Center for Childhood Obesity Research, The Pennsylvania State University, University Park, PA, United States
Patient reported outcome measures (PROM) can engage patients and clinicians to improve health outcomes. Their population health impact may be limited by systematic barriers inhibiting access to completion. In this analysis we evaluated the association between individual parent/child characteristics and clinic factors with parental completion of a locally developed PROM, the Early Healthy Lifestyles (EHL) questionnaire. Participants included parent-child dyads who presented at 14 pediatric clinics for regularly scheduled well-child visits (WCV) prior to age 26 months. EHL items include feeding practices, diet, play time, screen exposure, and sleep. Completion was categorized at patient- (i.e., parent-child dyad) and clinic-levels. Parents completed the 15-item EHL in the patient portal before arrival or in the clinic; ninety-three percent of EHL questionnaires were completed in the clinic vs. 7% in the patient portal. High-completers completed EHL for half of WCVs; low-completers completed at least once; and non-completers never completed. Clinics were classified by EHL adoption level (% high completion): High-adoption: >50%; Moderate-adoption: 10%–50%; and Low-adoption: <10%. Individual-level factors had negligible impact on EHL completion within moderate/low EHL adoption sites; high-adoption sites were used to evaluate infant and maternal factors in association with EHL completion using hierarchical logistic regression. Noncompletion of EHL was significantly associated (p < 0.05) with infant use of public insurance (OR = 1.92 [1.42, 2.59]), >1 clinic site for WCV (OR = 1.83 [1.34, 2.50]), non-White birth mother (OR = 1.78 [1.28, 2.47]), and body weight <2,500 grams or gestational age <34 weeks (OR = 1.74 [1.05, 2.90]). The number of WCVs, a proxy for clinic size, was evaluated but was not associated with completion. Findings indicate potential disparities between populations exposed to, completing, and benefitting from these tools.
Introduction
Patient-reported outcomes (PROs) are any report, direct from the patient without interpretation, of the status of a patient's health condition or health behavior (1). Often, PROs collect self-reported patient data on health-related quality of life; symptoms and symptom burden; and health behaviors (e.g., diet or sleep hygiene) (1). Patient-reported outcome measures (PROM) are the tools by which PROs are measured or collected, and often take the form of a standardize questionnaire delivered to patients in either a condition-specific or generic, routine care setting (2). Although PROM were originally created for use in clinical trials to evaluate treatment efficacy, they are increasingly used to support clinical decision making. PROM encourage the engagement of patients in their healthcare and facilitates patient-provider communication and individualized patient care by healthcare providers (3). PROM have also been successfully implemented in pediatric care settings for disease-specific (4–6) and primary care applications (7). Yet, less is known about the equity of PROM implementation across health systems and patient access to these tools (8). Evidence suggests that barriers to implementation of PROM in clinical settings include technology, stakeholder uncertainty about the, “How” or “Why” PROM would be used, stakeholder concerns about the negative impact of PROM, and competing demands from current clinic workflows (8). However, once PROM are implemented in clinical settings across healthcare systems, it is also unclear whether these barriers to implementation translate to disparities in adoption of PROM and patient completion of the tools.
Social determinants ranging from the structure and systems level (e.g., federal or state regulations), community level (e.g., policies or built environment), institutional level (e.g., schools or healthcare administration), interpersonal level (e.g., support systems), to the individual level (e.g., health behaviors or beliefs) are interconnected and impact the health achievement of individuals (9). Although individual characteristics such as sex, BMI, or age are associated with PROM completion (10, 11), no study has simultaneously evaluated upstream system-level factors and individual-factors associated with PROM completion. In this paper, we performed an observational study with a retrospective chart review to examine individual (parent/child), and clinic factors associated with parental completion of a locally developed PROM, the Early Healthy Lifestyles (EHL) questionnaire, at Geisinger pediatric care clinics between 2016 and 2020. We hypothesize that institutional-level (e.g., clinic size) factors and individual-level factors (e.g., insurance type) will be associated with the completion of PROM.
Methods
Early healthy lifestyles (EHL) tool
The EHL PROM is a risk assessment tool comprised of 15-items (e.g., questions) that allow parents or caregivers to self-assess obesogenic behaviors that may increase their child's risk for obesity. The EHL tool was originally created and implemented as part of the WEE Baby Care study—a pragmatic, randomized clinical trial that compared standard pediatric clinical care to a responsive parenting intervention (12). The EHL tool's theory was informed by the Centers for Disease Control and Prevention's Infant Feeding Practices Study II (13, 14), the Intervention Nurses Start Infants Growing on Healthy Trajectories (INSIGHT) trial (15) and Healthy Active Living for Families (HALF) materials (16). The EHL tool assesses the domains of infant feeding and parenting practices: food and beverage intake, sleep hygiene, physical activity, television/media exposure, and soothing practices. As such, the EHL identifies signals across several domains that are aligned with anticipatory guidance to support regulation of eating, sleep, emotion, and activity for healthy development. The EHL is currently available only in English and requires less than five minutes to complete. Standard of care at Geisinger is for the EHL tool to fire two weeks prior to each scheduled well-child visit (WCV) that occurs at a Geisinger clinic for children between birth and 26 months of age. The tool can be completed in the patient portal (MyGeisinger, i.e., MyChart in EPIC® Electronic Health Record) prior to arrival, on a clinic iPad in the waiting room, or in the exam room via staff interview. All procedures were approved by the Geisinger Clinic Institution Review Board (IRB).
Inclusion / exclusion criteria
For this study WCV encounters were limited to those WCVs that occurred at a clinic that was offering the EHL; limited to corresponding time windows of 2016–2020; and the parent or caregiver responded to EHL Q1 with either, “I live with this child and care for him/her regularly” or “I do not live with this child but care for him/her regularly.” Criteria for available pediatric patient data included a baseline measurement whereby the child attended a qualifying WCV at age <3 months / weight and length (or height) were measured; a qualifying WCV while aged 6–15 months with weight and length (or height) measured; and a qualifying WCV while aged 18–26 months with weight and length (or height) measured. All patients (i.e., parent-child dyads) must have had at least 3 WCVs at study clinics.
Completer vs. non-completer definitions were established to ensure equal average dose or opportunity for completion of the EHL tool at qualifying WCVs. An EHL questionnaire was only considered complete if 100% of the questions were answered. Three EHL completion groups were established. A Non-completer completed the EHL zero times at their qualifying WCVs. A Completer was split into low and high categories: Low: completed EHL at 1%–49% of their qualifying WCVs and High: completed EHL at ≥50% of their qualifying WCVs (17). These criteria resulted in the identification of N = 4,960 eligible patients. When available, maternal electronic health record (EHR) data was linked with infant EHR using the Births / Deliveries ID's using International Classification of Diseases, 9th Revision (ICD-9-CM) from mother and infant EHR.
Exclusion criteria included: patient visited Geisinger clinics at which EHL was not being administered; attended a WCV outside the time window of 2016–2020; and/or parent/caregiver responded to EHL Q1 with “I do not live with this child regularly and I do not care for him/her regularly.”
Clinic sites
Clinic sites were conveniently selected for EHL administration, principally because these sites aligned with the WEE Baby Care Study (12) implementation or were identified by clinical leadership as viable implementation sites. All clinic staff are instructed to and evaluated on their performance to close care gaps as a quality initiative. Incomplete PROM are flagged as care gaps to alert check-in staff of the opportunity to improve quality. Thus, if EHL were incomplete when the parent presents at the clinic, then staff were instructed to ask the parent to complete the EHL on an iPad, or if the workflow demands, room the patient and collect the data by interview with staff entry into the EHR. The clinics selected for EHL administration are predominantly clustered in two counties across the Central PA region: Luzerne and Lackawanna Counties and encompass a 40–50-mile radius. For the analysis presented in this paper, which covered the years 2016–2020, N = 14 clinics were represented. Some patients (19%) had WCV at >1 clinic location, therefore, a “primary clinic site” was chosen for each patient based on the clinic site at which the most WCVs were completed. The distribution of EHL completion group was reviewed and each primary clinic site was categorized into one of three EHL adoption groups, based on the distribution of EHL completion across all sites: High EHL adoption: sites with >50% high completers; Moderate EHL adoption: sites with 10%–50% high completers; Low EHL adoption: sites with <10% high completers. EHL adoption category was the primary clinic factor under consideration. This clinic factor was used to evaluate which sites had sufficient variability to further explore patient level characteristics.
Statistical analysis
SAS version 9.4 was used for statistical analysis. To evaluate the association between birth characteristics of the child and maternal characteristics with EHL completion, data from labor and delivery (LD) were used to identify children that had available delivery data and to link the mother to the infant. Of the 4,960 infants described above, there were a total of 2,991 (60%) that were in the LD database including n = 1,087 from high adoption sites, n = 1,363 from moderate EHL adoption sites, and n = 541 from low EHL adoption sites. Chi-square and t-tests were performed to compare completer vs. non-completer across demographics and Primary Clinic EHL adoption.
Hierarchical logistic regression (HLR) was used to evaluate the infant and maternal characteristics that were associated with completion of EHL. This model used a random intercept term within PROC GLIMMIX in SAS to account for multilevel correlation introduced by clinic site (18). Individual level factors had negligible impact on EHL completion within moderate/low EHL adoption sites, as such the subset of high-adoption sites were used to evaluate whether infant characteristics, birth characteristics, and maternal characteristics were associated with EHL completion in the HLR.
We first selected items for consideration in the HLR model by using clinic size, infant, birth, and maternal characteristics with p-values < 0.20 in unadjusted analysis (19). The items were then re-evaluated with an HLR model that accounted for primary clinic site and items that continued to have p-value < 0.20 were retained. Models of infant characteristics were then compared using the cohort of patients that attended high-adoption sites (n = 1708) vs. a subset of the cohort that attended high-adoption sites and had complete delivery information (n = 1087) in addition to birth characteristics and maternal characteristics. Given that these two models were consistent with one another, we chose to continue with the sub-cohort that had complete delivery data (n = 1087) to avoid issue with missing data. Lastly, model building was completed using a forward stepwise approach (after considering both strength of association and scientific plausibility) with the goal of identifying a minimal set of clinical variables that independently predict EHL completion.
Results
Table 1 presents the distribution of number of completed EHL questionnaires by number of WCVs attended per completer group. The median number of WCV was 7 for non-completers and 8 for both low and high completers. Table 2 describes primary clinic sites by EHL adoption category and the respective proportion of individuals in each completer group. There was an unequal distribution of high completers across primary clinic site, with 5 sites categorized as high EHL adoption (comprised of >50% high completers), 2 sites categorized as moderate EHL adoption (10%–49% high completers), and 9 sites categorized as low EHL adoption (<10% high completers). The number of WCVs over a multi-year period varied across the three categories of adoption although high EHL adopters had the smallest variance (n = 241) compared to moderate (n = 531) and low (n = 827) adopters. The ratio of male to female infants was nearly equal (50.7% male overall and not associated with EHL adoption level, p = 0.271) (Table 3). Race/ethnicity was different based on EHL adoption level (p < 0.0001). Most participants identified as non-Hispanic/Latino White (73% in High adoption sites, 86% in Moderate adoption sites, 74% in low adoption sites), followed by Hispanic/Latino (15%, 7.5%, 18.3%) and African American (10%, 4.4%, 6.5%). The number of WCV attended also differed by clinic adoption level, whereby nine or more WCV were completed by 27.9% of participants in High adoption sites and 19.5% and 25.9% in Moderate and Low adoption sites respectively. Lastly, use of public insurance also differed by clinic adoption level with the greatest percentage of participants (33.9%) at High adoption sites (Table 3).
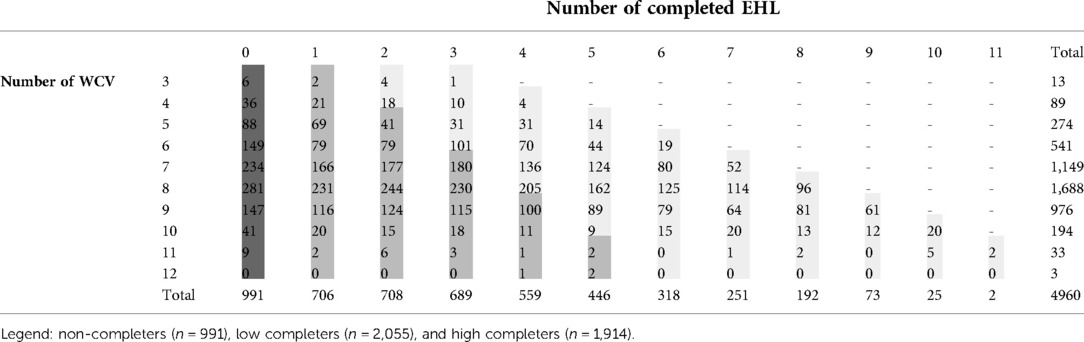
Table 1. The distribution of number of completed EHL questionnaires by number of WCVs attended per completer group.
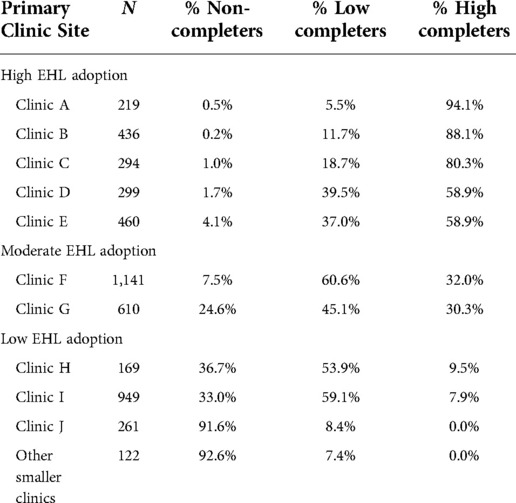
Table 2. Primary clinic sites by EHL adoption category with the proportion of individuals in each completer group.
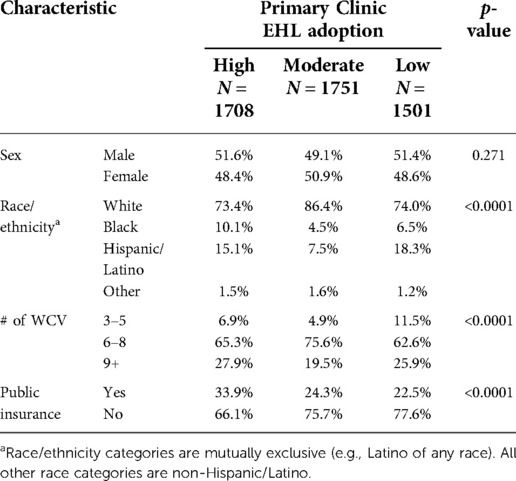
Table 3. Comparison of selected characteristics of patients by level of EHL adoption at their primary clinic site.
Providing that a patient's opportunity for EHL exposure and completion depended upon clinic adoption level, Low EHL Adoption sites were removed from further analysis. Choosing to only evaluate High and Moderate adoption sites, where patient opportunity to complete the EHL was more consistent, allowed for evaluation of our primary interest in individual characteristics associated with EHL completion. Clinic size was not associated with high completion (p = 0.795) when evaluating all sites (regardless of adoption level). Furthermore, when limited to high adoption sites, clinic size remained not associated with high completion (p = 0.407). Individual characteristics significantly associated (p < 0.0001) with High Completion of EHL at High Adoption sites included infant race/ethnicity, utilizing one primary WCV clinic, and use of private insurance (Table 4). Infant birth weight (p = 0.015), maternal parity (p = 0.03), maternal age (p = 0.0049), maternal race/ethnicity (p < 0.0001), and maternal use of private insurance (p = 0.0003) were also associated with High Completion of EHL (Table 4).
Table 5 presents the final model results of the hierarchical logistic regression and identifies items significantly associated with the odds of noncompletion of the EHL. These items included infant use of public insurance (OR = 1.92 [1.42, 2.59]), greater than one clinic site used for WCV (OR = 1.83 [1.34,2.50]), non-White birth mother (OR = 1.78 [1.28,2.47]), and birthweight <2,500 grams or gestational age <34weeks (OR = 1.74 [1.05, 2.90]).
Discussion
The goal of this study was to investigate the factors associated with the completion or non-completion of the Early Healthy Lifestyles (EHL) PROM. The integration of the EHL into standard pediatric care and the electronic health record (EHR) across several clinical sites in the Geisinger network allowed for both the compilation of a large cohort of individuals that had the opportunity to complete the EHL and for the linkage of health data for infants and mothers.
Our evaluation of the factors associated with completion and non-completion of the EHL tool was important from an implementation science perspective and from a patient-centered healthcare perspective. PROM are a standardized way to collect information directly from patients on their experience, perception, or beliefs relative to a disease or health status (20). With the advancement of health information technology (HIT), PROM can be implemented in easy-to-use formats for patients, often via an online patient portal or on a tablet in the waiting room of the clinic. Responses can be integrated into the EHR, allowing for quick scoring and review by providers and implementation into patient-centered care (21).
PROM like the EHL are beneficial to population health when exposure to and utilization of the tools is high. Hence, a main aim of this study was to examine the implementation patterns of the EHL across the clinics at which it was active in the system; benefit from patient and provider use of PROM is highly dependent upon exposure or adoption. Initial analyses revealed that clinic site was significantly correlated with completion of the EHL, whereby clinic “adoption” of the tool varied highly across clinics with specific sites more likely to have non-completers over high completers. We also found that primary utilization of more than one clinic site for WVC was associated with EHL noncompletion. Given the spatial context of clinics in this study, it is possible that dyads might attend more than one clinic for convenience; whether some are closer to home while others are closer to work or childcare (22).
Increasing evidence suggests that healthcare system-level factors including characteristics of providers and clinic staff can influence the implementation of PROM (23). Additional factors impacting adoption of collecting of PRO or barriers to PROM are large clinic size [high burden of implementation with the number of patients seen] (24); clinic leadership biases and belief in value of PROM tool (24); and language barriers—most PROM are written in English and this creates a significant barrier for non-English speakers (3, 25). The two clinics with the highest volume of WCVs, a proxy for clinic size, were in the moderate and low EHL adoption categories, whereas the clinics with high adoption had the smallest volume and variance in WCVs, potentially supporting the premise that large clinic size is a barrier. However, total visits, including a distribution of acute and preventive care visits, the number of staff and type, and operating hours would provide a more comprehensive assessment of whether clinic size affects completion. Prior research has identified associations between staff and clinic factors and the receipt of preventive care (26). Future directions include evaluating the features of high, medium, and low adoption sites that might be contributing to completion. It is important to note, however, that we found no association between clinic size and completion that could have been limited to how we defined clinic size as the number of WCVs before age 2 years.
Alternatively, our data reveal that 6.6% of EHL were completed via the MyGeisinger patient portal. Increasingly, studies show that electronic patient portals are underutilized, and this is especially true among low-income and minoritized populations (27). Given that patient portals can be a main or important implementation method for PROM, it is relevant to consider how low rates of registration and broader system-level barriers may incite disparities in PROM completion among these communities. Other factors affecting patient portal use and the low utilization rate include lack of patient education about the portal and inconsistent provider facilitation of portal use (27).
In addition to evaluating system-level factors, as encompassed by EHL adoption level, that were associated with the completion of EHL, we sought to elucidate the individual-level factors that might influence completion. We found that the odds of an individual being a non-completer were highest for: infant utilization of public insurance [a surrogate for low-income households (28)], utilizing more than one clinic site for WCV, non-White birthmothers, and infant birthweight of <2,500 grams or gestational age <34 weeks. Some items simultaneously align highly with social determinants of health factors related to preventive care utilization, continuity of care, and attendance of well-child visits. Hardy et al., demonstrated that in rural communities, preventive care receipt is lower, due in part to provider type, poverty, unemployment, and education levels (29). Wolf et al., also detected that poor attendance at preventive care was associated with mothers and children who were publicly insured and children whose mothers had younger age, greater number of pregnancies and transportation (30). Alternatively, it has also been observed that premature and low-birth weight infants display varied health care use patterns. One study revealed that of infants born at ≤35 weeks gestation, 43% completed the full course of preventive care visits in the first 18 months (31). Furthermore, varied patterns in attendance or completion of health supervision visits among premature (32) and low birth weight (33, 34) infants is associated with under vaccination. Lastly, evidence also suggests that continuity of care (e.g., utilizing the same clinic or providers for care and minimal interruptions in the schedule of care) is linked with higher well-visit attendance and improved receipt of preventive screening (35, 36).
Given that the EHL is administered at WCV, and this population is a rural population, it is relevant to consider how these factors may interact. Previous studies conducted in this region and population have detected high utilization rates of public insurance—in 2018, among WCVs across 55 departments at Geisinger, 33.3% of the children with a mean age of 3.3 years received Medical Assistance. The population included in this study displayed a similar rate of public insurance, of around 34%, across a wider window of time. Ultimately, these findings and concurrent parallels with factors associated with receiving care, suggest a larger issue of inequitable access to PROM. Since individual level factors had negligible impact on EHL completion within moderate/low EHL adoption sites, the subset of high-adoption sites was used in the hierarchical logistic regression to evaluate whether infant and maternal factors were associated with EHL completion. In other words, despite attending WCV at High Adoption sites, individual-level factors had a significant effect on determining completion. Additional research is needed to elucidate the context around individuals making it to care but not completing EHL or other PROM either because it is not offered to them specifically or because individuals chose not to complete the tool for personal, health and/or technology literacy, or other reasons.
In this study we were limited by the completeness of infant and maternal EHR for our investigation of individual-level characteristics. We were unable to evaluate food insecurity because of the high rate of missingness on this pre-visit screener. Furthermore, because not all individuals who receive care at Geisinger have delivered at Geisinger, we were unable to acquire Labor / Delivery information on each mother-infant dyad. Despite these limitations, we were able to compile a sizable cohort of n = 1,087 mother-infant dyads. It is also relevant to consider the possible impact that the written language of the EHL had on completion. Some of the clinics we evaluated receive a higher percentage of patients for whom English is a second or non-preferred language—we were unable to determine the extent to which this impacted completion. We plan to elucidate language barriers to PROM completion in future investigations.
By using hierarchical logistic regression to model the association between individual-level factors and EHL completion while controlling for clinic location, this study was able to provide valuable insights into the possible reasons for variable completion of PROM in patient care. These results underscore both individual- and system-level factors that influence completion of PROM and illuminate potential disparities between populations being exposed to, completing, and benefitting from these tools.
Data availability statement
The raw data supporting the conclusions of this article will be made available by the authors, without undue reservation.
Ethics statement
The study was reviewed and approved by The Geisinger IRB, approval #2012-0363. Written informed consent to participate in this study was provided by the participants’ legal guardian/next of kin.
Author contributions
CFM: Conceptualization, methodology, investigation, writing—original draft, writing—review / editing. GCW: Methodology, data curation, formal analysis, writing—review / editing. JFH: Methodology, writing—review / editing. WJC: Methodology, project administration, funding acquisition, writing—review / editing. JSS: Methodology, writing—review / editing. LBD: Conceptualization, methodology, resources, project administration, funding acquisition, writing—review / editing. All authors contributed to the article and approved the submitted version.
Funding
The authors declare that no funds, grants, or other support were received during the preparation of this manuscript.
Conflict of interest
The authors declare that the research was conducted in the absence of any commercial or financial relationships that could be construed as a potential conflict of interest.
Publisher's note
All claims expressed in this article are solely those of the authors and do not necessarily represent those of their affiliated organizations, or those of the publisher, the editors and the reviewers. Any product that may be evaluated in this article, or claim that may be made by its manufacturer, is not guaranteed or endorsed by the publisher.
References
1. Centers for medicare and medicaid services. Patient reported outcome measures. Centers for medicare and medicaid services 2021.
2. Nguyen H, Butow P, Dhillon H, Sundaresan P. A review of the barriers to using patient-reported outcomes (PROs) and patient-reported outcome Measures (PROMs) in routine cancer care. J Med Radiat Sci. (2021) 68(2):186–95. doi: 10.1002/jmrs.421
3. Hughes S, Aiyegbusi OL, Lasserson D, Collis P, Glasby J, Calvert M. Patient-reported outcome measurement: a bridge between health and social care? J R Soc Med. (2021) 114(8):381–8. doi: 10.1177/01410768211014048
4. Anderson LM, Papadakis JL, Vesco AT, Shapiro JB, Feldman MA, Evans MA, et al. Patient-reported and parent proxy-reported outcomes in pediatric medical specialty clinical settings: a systematic review of implementation. J Pediatr Psychol. (2020) 45(3):247–65. doi: 10.1093/jpepsy/jsz082
5. Lunt LE, Shoop-Worrall S, Smith N, Cleary G, McDonagh J, Smith AD, et al. Validation of novel patient-centred juvenile idiopathic arthritis-specific patient-reported outcome and experience measures (PROMs/PREMs). Pediatr Rheumatol Online J. (2020) 18(1):91. doi: 10.1186/s12969-020-00481-2
6. Crudgington H, Collingwood A, Bray L, Lyle S, Martin R, Gringras P, et al. Mapping epilepsy-specific patient-reported outcome measures for children to a proposed core outcome set for childhood epilepsy. Epilepsy Behav. (2020) 112:107372. doi: 10.1016/j.yebeh.2020.107372
7. Jetelina KK, Woodson TT, Gunn R, Muller B, Clark KD, DeVoe JE, et al. Evaluation of an electronic health record (EHR) tool for integrated behavioral health in primary care. J Am Board Fam Med. (2018) 31(5):712–23. doi: 10.3122/jabfm.2018.05.180041
8. Stover AM, Haverman L, van Oers HA, Greenhalgh J, Potter CM, Ahmed S, et al. Using an implementation science approach to implement and evaluate patient-reported outcome measures (PROM) initiatives in routine care settings. Qual Life Res. (2020) 30(11):3015–33. doi: 10.1007/s11136-020-02564-9
9. Braveman P, Egerter S, Williams DR. The social determinants of health: coming of age. Annu Rev Public Health. (2011) 32(1):381–98. doi: 10.1146/annurev-publhealth-031210-101218
10. Cotter EJ, Hannon CP, Locker P, Davey A, Wang KC, Verma NN, et al. Male sex, decreased activity level, and higher BMI associated with lower completion of patient-reported outcome measures following ACL reconstruction. Orthop J Sports Med. (2018) 6(3). doi: 10.1177/2325967118758602
11. Schamber EM, Takemoto SK, Chenok KE, Bozic KJ. Barriers to completion of patient reported outcome measures. J Arthroplasty. (2013) 28(9):1449–53. doi: 10.1016/j.arth.2013.06.025
12. Savage JS, Kling SMR, Cook A, Hess L, Lutcher S, Marini M, et al. A patient-centered, coordinated care approach delivered by community and pediatric primary care providers to promote responsive parenting: pragmatic randomized clinical trial rationale and protocol. BMC Pediatr. (2018) 18(1):293. doi: 10.1186/s12887-018-1263-z
13. Ihmels MA, Welk GJ, Eisenmann JC, Nusser SM, Myers EF. Prediction of BMI change in young children with the family nutrition and physical activity (FNPA) screening tool. Ann Behav Med. (2009) 38(1):60–8. doi: 10.1007/s12160-009-9126-3
14. Ihmels MA, Welk GJ, Eisenmann JC, Nusser SM. Development and preliminary validation of a family nutrition and physical activity (FNPA) screening tool. Int J Behav Nutr Phys Act. (2009) 6:14–14. doi: 10.1186/1479-5868-6-14
15. Paul IM, Williams JS, Anzman-Frasca S, Beiler JS, Makova KD, Marini ME, et al. The intervention nurses start infants growing on healthy trajectories (INSIGHT) study. BMC Pediatr. (2014) 14:184–184. doi: 10.1186/1471-2431-14-184
16. Encouraging healthy active living for families: a report of the healthy active living for families project.
17. Kling SM, Harris HA, Marini M, Cook A, Hess LB, Lutcher S, et al. Advanced health information technologies to engage parents, clinicians, and community nutritionists in coordinating responsive parenting care: descriptive case series of the women, infants, and children enhancements to early healthy lifestyles for baby (WEE Baby) care randomized controlled trial. JMIR Pediatr Parent. (2020) 3(2):e22121. doi: 10.2196/22121
18. Dai J, Li Z, Rocke D. Hierarchical logistic regression modeling with SAS GLIMMIX. SAS Conference Proceedings: Western Users of SAS Software. 2006.
19. Vittinghoff E, Glidden DV, Shiboski SC, McCulloch CE. Regression methods in biostatistics. 2nd ed. New York: Springer Science + Business Media (2012).
20. Austin E, LeRouge C, Hartzler AL, Segal C, Lavallee DC. Capturing the patient voice: implementing patient-reported outcomes across the health system. Qual Life Res. (2019) 29(2):347–55. doi: 10.1007/s11136-019-02320-8
21. Jensen R, Rothrock N, DeWitt E, Spiegel B, Tucker C, Crane H, et al. The role of technical advances in the adoption and integration of patient-reported outcomes in clinical care. Med Care. (2015) 53(2):153–9. doi: 10.1097/MLR.0000000000000289
22. Matthews SA, Yang T. Spatial polygamy and contextual exposures (SPACEs): promoting activity space approaches in research on place and health. Am Behav Sci. (2013) 57(8):1057–81. doi: 10.1177/0002764213487345
23. Wolff AC, Dresselhuis A, Hejazi S, Dixon D, Gibson D, Howard AF, et al. Healthcare provider characteristics that influence the implementation of individual-level patient-centered outcome measure (PROM) and patient-reported experience measure (PREM) data across practice settings: a protocol for a mixed methods systematic review with a narrative synthesis. Syst Rev. (2021) 10(1):1–169. doi: 10.1186/s13643-021-01725-2
24. Hsiao C, Dymek C, Kim B, Russell B. Advancing the use of patient-reported outcomes in practice: understanding challenges, opportunities, and the potential of health information technology. Qual Life Res. (2019) 28(6):1575–83. doi: 10.1007/s11136-019-02112-0
25. Jahagirdar D, Kroll T, Ritchie K, Wyke S. Using patient reported outcome measures in health services: a qualitative study on including people with low literacy skills and learning disabilities. BMC Health Serv Res. (2012) 12(1):431. doi: 10.1186/1472-6963-12-431
26. Dietz VJ, Baughman AL, Dini EF, Stevenson JM, Pierce BK, Hersey JC. Vaccination practices, policies, and management factors associated with high vaccination coverage levels in Georgia public clinics. Arch Pediatr Adolesc Med. (2000) 154(2):184–9. doi: 10.1001/archpedi.154.2.184
27. Kim J, Mathews H, Cortright LM, Zeng X, Newton E. Factors affecting patient portal use among low-income pregnant women: mixed-methods pilot study. JMIR Form Res. (2018) 2(1):e6. doi: 10.2196/formative.5322
28. Schwartz BS, Bailey-Davis L, Bandeen-Roche K, Pollak J, Hirsch AG, Nau C, et al. Attention deficit disorder, stimulant use, and childhood body mass index trajectory. Pediatrics. (2014) 133(4):668–76. doi: 10.1542/peds.2013-3427
29. Hardy RY, Liu GC, Kelleher K. Contribution of social determinant of health factors to rural-urban preventive care differences among medicaid enrollees. Acad Pediatr. (2021) 21(1):93–100. doi: 10.1016/j.acap.2020.08.022
30. Wolf ER, Donahue E, Sabo RT, Nelson BB, Krist AH. Barriers to attendance of prenatal and well-child visits. Acad Pediatr. (2021) 21(6):955–960. doi: 10.1016/j.acap.2020.11.025
31. D'Agostino JA, Passarella M, Saynisch P, Martin AE, Macheras M, Lorch SA. Preterm infant attendance at health supervision visits. Pediatrics (Evanston). (2015) 136(4):e794–802. doi: 10.1542/peds.2015-0745
32. Hofstetter AM, Jacobson EN, deHart MP, Englund JA. Early childhood vaccination status of preterm infants. Pediatrics (Evanston). (2019) 144(3):1. doi: 10.1542/peds.2018-3520
33. Bary-Weisberg D, Stein-Zamir C. Vaccination timeliness and completeness among preterm and low birthweight infants: a national cohort study. Hum Vaccin Immunother. (2021) 17(6):1666–74. doi: 10.1080/21645515.2020.1840255
34. Batra JS, Eriksen EM, Zangwill KM, Lee M, Marcy SM, Ward JI, et al. Evaluation of vaccine coverage for low birth weight infants during the first year of life in a large managed care population. Pediatrics (Evanston). (2009) 123(3):951–8. doi: 10.1542/peds.2008-0231
Keywords: patient-reported outcome measure (PROM), pediatric care settings, social determinansts of health, healthcare factors, individual-level factors
Citation: McCabe CF, Wood GC, Franceschelli-Hosterman J, Cochran WJ, Savage JS and Bailey-Davis L (2022) Patient-reported outcome measures can advance population health, but is access to instruments and use equitable?. Front. Pediatr. 10:892947. doi: 10.3389/fped.2022.892947
Received: 9 March 2022; Accepted: 28 September 2022;
Published: 18 October 2022.
Edited by:
Nakiya Naomi Showell, Johns Hopkins Medicine, United StatesReviewed by:
Lisa DeCamp, University of Colorado, United StatesBrandon Smith, Johns Hopkins Medicine, United States
© 2022 McCabe, Wood, Franceschelli-Hosterman, Cochran, Savage and Bailey-Davis. This is an open-access article distributed under the terms of the Creative Commons Attribution License (CC BY). The use, distribution or reproduction in other forums is permitted, provided the original author(s) and the copyright owner(s) are credited and that the original publication in this journal is cited, in accordance with accepted academic practice. No use, distribution or reproduction is permitted which does not comply with these terms.
* Correspondence: Carolyn F. McCabe Y21jY2FiZTFAZ2Vpc2luZ2VyLmVkdQ==
† ORCID Carolyn F. McCabe orcid.org/0000-0002-1321-621X G. Craig Wood orcid.org/0000-0002-6015-9692 Jennifer S. Savage orcid.org/0000-0002-2912-8687 Lisa Bailey-Davis orcid.org/0000-0002-8781-1521
Specialty Section: This article was submitted to Children and Health, a section of the journal Frontiers in Pediatrics