- 1Department of Pediatrics, Qilu Hospital of Shandong University, Jinan, China
- 2Department of Pediatrics, The Fifth People's Hospital of Jinan, Jinan, China
- 3Department of Pediatrics, The People's Hospital of Weifang, Weifang, China
- 4Dana-Farber/Boston Children's Cancer and Blood Disorders Center, Harvard Medical School, Boston, MA, United States
Background: Henoch-Schönlein purpura, now called immunoglobulin A (IgA) vasculitis, is a common autoimmune disease in children, its association with gut microbiota composition remains unknown.
Methods: The collected cases were divided into three groups: G1 group of simple skin type, G2 group with no digestive tract expression, G3 group of mixed digestive tract, and C group of healthy children. The fecal samples of each group of children were collected and the sequencing data was processed and analyzed. The dilution curve reflected the reasonableness of the amount of sequencing data.
Results: The number of species composition sequences in the G1, G2 and G3 groups was lower than that in the C group, especially for the G2 and G3 groups. The four most abundant bacteria were Bacteroidetes, Firmicutes, Proteobacteria and Actinobacteria. The relative abundance of Proteobacteria in the G2 and G3 groups was significantly higher than that in the G1 and C groups, while the relative abundance of Actinobacteria was significantly reduced, and the relative abundance of Actinobacteria in the G1 group was lower than that in the C group. Principal component analysis of the UPGMA clustering tree and each group of samples showed that the microbial community composition of the same group of samples was similar.
Conclusions: The abundance of intestinal microbes in children with IgA vasculitis is lower than in normal children. Bacteroidetes, Firmicutes, Proteobacteria and Actinobacteria are the four most abundant bacteria in the intestinal flora of children. Proteobacteria and Actinobacteria are associated with organ involvement in IgA vasculitis.
Introduction
Immunoglobulin A (IgA) vasculitis, is a systemic vasculitis that is common in pediatrics. It occurs mainly in children between 3 and 10 years of age. Children with mild conditions have only purpura, and some with severe symptoms which could have multiple affected systemic organs, including skin, gastrointestinal tract, joints and kidneys. The incidence of IgA vasculitis with mixed syndromes has been increasing recently (1). The long-term or repeated use of steroids and immune-suppressants, especially for children with gastrointestinal and renal involvement, can easily lead to infection, electrolyte imbalance, and a series of complications that seriously affect their quality of life (2). Therefore, how to effectively prevent and reduce the occurrence of allergic purpura involving vital organs in children is very important.
The development of the metagenomics program has led to the discovery of the interrelationships and effects of the gut with its microbial flora, and providing how different community compositions affect various states of human health at all ages, from infancy to old age (3). We hypothesized that children with IgA vasculitis who have intestinal involvement would have altered fecal microbial flora than the ones without intestinal involvement. Moreover, whether IgA vasculitis patients without intestinal involvement would have intestinal flora that was not significantly different from that of healthy controls is still unknown. To explore the intestinal flora differences of children with IgA vasculitis involving different organs, the study aimed to establish a clearer picture of the differences among children with different types of IgA vasculitis, and identify whether healthy fecal microbiota could potentially be used for the clinical treatment of IgA vasculitis.
Materials and Methods
Patients
IgA Vasculitis Patients With G1-G3 Group
The G1-G3 group was composed of children with IgA vasculitis. They were selected from the hospitalized children at Department of Pediatrics of Qilu Hospital of Shandong University from Octobor 2017 to September 2019.
G1 patients are accord with the criteria as the following: (a) clinical symptoms and physical signs consistent with the diagnostic criteria (4) for IgA vasculitis; (b) no abnormality of the joints, gastrointestinal tract, or urine detecting during examination; (c) in the acute stage of the disease, systemic treatment including the application of corticosteroids has not been started yet; and (d) no antibiotics or microecological preparations have been used in the past month. Fecal samples from children with IgA vasculitis and meeting this enrolment criteria (n = 20) were collected in sterile centrifuge tubes and stored at −78°C. Notably, 15 of the 20 subjects had other symptoms of IgA vasculitis within 6 months after being selected.
G2-G3 patients are cellected according to the following criteria: (a) clinical symptoms and physical signs consistent with the diagnostic criteria for IgA vasculitis; (b) presentation of joint or gastrointestinal symptoms or signs or of abnormal urine; (c) in the acute stage of the disease, systemic treatment has not been started yet; (d) exclusion of other possible factors that can lead to joint, gastrointestinal, or urinary abnormalities; and (e) no antibiotics or microecological preparations have been used in the past month. IgA vasculitis patients meeting this criteria were divided into two groups: those without gastrointestinal symptoms, G2 group (n = 15), and those with gastrointestinal symptoms, G3 group (n = 12). Fecal samples were collected and stored by the methods described above.
Healthy Control Group
The healthy control group (CON) included 15 healthy children who were randomly selected from children's health clinics according to the following criteria: (a) no diarrhea or other gastrointestinal diseases within the previous 4 weeks; (b) no antibiotics or microecological preparations have been used in the past month; and (c) normal results from a routine examination of the stool. Fecal samples were collected and stored by the methods described above.
Methods
The methods are similar to the previously described method (5, 6), and the modification is as following.
Extraction of Genome DNA
The total genomic DNA was extracted from the sample using the CTAB/SDS (7) method. The concentration and purity of DNA were monitored by separation on a 1% agarose geland diluted to a concentration of 1 ng/μl with sterile water.
Amplicon Generation
The diluted genomic DNA was used as a template; specific primers with Barcode were used according to the selection of the sequencing region; Phusion® High-Fidelity PCR Master Mix with GC Buffer was used. The PCR was performed using efficient and high fidelity enzymes to ensure amplification efficiency and accuracy. Primer corresponding area: 18S V4 area-528F-706R; 16S V4 area-515F-806R; ITS1 area-ITS1F-ITS2; ITS2 area-ITS2-3F-ITS2-4R; 18S V9 area-1380F-1510R.
PCR Product Mixing and Purification
The samples were mixed at the same concentration according to the concentration of the PCR product, thoroughly mixed, and the PCR product was detected by 2% agarose gel electrophoresis, and GeneJET gel (Thermo Scientific) was used. The product was recovered.
Library Preparation and Sequencing
The library was constructed using the NEB Next® UltraTM DNA Library Prep Kit for Illumina Library. Qubit quantification and library detection were performed on the constructed library. After passing the test, the MiSeq was used for sequencing on the machine.
Data Analysis
Sequencing Data Processing
The raw data obtained by the Illumina MiSeq/HiSeq sequencing platform has some low quality data that will interfere with the final result. Therefore, it is necessary to preprocess the offline data before further analysis. The specific processing steps are as follows: Data splitting, PE Reads stitching, Tags filter and Tags to chimera sequences. The PE Reads splicing is performed with the application of FLASH (V1.2.7, http://ccb.jhu.edu/software/FLASH/) (8) to split the data for the reading of each sample. Raw Tags are also the stitching sequences obtained.
OUT Cluster and Species Annotation
All of the Effective Tags sequences of all samples were clustered using Uparse software (Uparse v7.0.1001, http://drive5.com/uparse/) (9), providing clustering with 97% and 95% consensus sequences to become OTUs results, whichpurpose is to study the compositional diversity information of the species of the sample. A sequence in the same OTU is considered to be a sequence derived from one of the same taxon as the hypothetical taxon. When Uparse constructs OTUs, it selects the sequence with the highest frequency according to its algorithm principle, and uses these RDP Classifier and GreenGene database for species annotation analysis to study the phylogenetic relationship between OTUs and uses KRONA for species identification. The results of the annotations are visualized. Based on the species annotation, the number of sequences for each sample at each classification level is calculated, and the sequence of species constitutes a histogram.
To facilitate further study of the phylogenetic relationships of OTUs and the structural differences of major flora between different samples (groups), phylogenetic relationship data for the first 10 genera of OTUs corresponding to the maximum relative abundance were selected and combined with each OTUs. Relative abundance and species annotation confidence for representative sequences, the results of the integration can visualize the diversity of the species composition of the study. According to the type labeling and abundance information of all samples of the genus level, select the top 35 abundance genus and its abundance information in each sample to draw a heat map, and collect clusters from the difference between the classification information and the sample to identify Focus on more species or samples in the study sample. Select the phylogenetic relationship data of the OTUs corresponding to the top 10 relatives of the largest relative abundance and the relative abundance information of their corresponding OTUs to achieve vertical clustering of samples at the OTUs level to examine the differences between different samples or Similarity.
Alpha Diversity
Alpha Diversity is used to analyze community diversity within a sample and includes three indicators: dilution curve, species richness, and community diversity. The sample complexity index was calculated and plotted using Qiime software (Version 1.7.0). The Rarefaction Curve is used to indicate whether the amount of sequencing data of the sample is reasonable and indirectly reflects the richness of the substance in the sample. It is a curve obtained by randomly extracting a certain amount of sequencing data from a sample to calculate the number of species they represent, based on the number of species and the amount of data. In the dilution curve, when the curve tends to be flat, it means that more data will only produce a small amount of new OTU, indicating that the amount of sequencing data is reasonable.
Beta Diversity
Principal Component Analysis (PCA) is a method for dimensionality reduction of multidimensional data and the most important elements and structures in the data by applying variance decomposition (10). It was applied to reduce the dimension of the original variables using the QIIME software package (V1.7.0, http://qiime.org/index.html) (11). It can reflect the difference of multi-dimensional data on the two-dimensional coordinate map, and the method of selecting the two coordinate axes that can reflect the difference between samples is selected from the PCA results. The closer the sample is in the PCA plot, the more similar its community composition is. UPGMA (Unweighted Pair-group Method with Arithmetic Mean) is a commonly used cluster analysis method in environmental biology. It requires a transformation from the distance matrix to a new set of orthogonal axes, where the maximum variation factor is represented by the first principal coordinate, the second maximum is represented by the second primary coordinate, and so on (12). UPGMA clustering is a hierarchical clustering method that uses average links and can be used to interpret the distance matrix (13).
Statistical Processing
The measurement data were expressed as mean ± standard deviation (x ± s), the rank data were analyzed by rank sum test, and analyzed by SPSS 20.0 software. The mean comparison between the two groups was performed by t-test, and the mean of the count data was compared. The Dunnett-t test was used for multi-sample multiple comparison. The Wilcoxon rank sum test was used for grade data. The overall rate was compared by χ2 test. P < 0.05 was considered statistically significant. Differences in individual abundance between the two groups were confirmed using Metastats software (http://metastats.cbcb.umd.edu/) (14, 15). LEfSe (http://huttenhower.sph.harvard.edu/galaxy) was used for quantitative analysis of biomarkers in different groups. The purpose of this method is to analyze data on the number of species much higher than the number of samples to determine the statistical significance, bio-identity and effect size estimates predicted biomarkers (16, 17). In order to determine the difference in microbial communities between the two groups, ANOSIM (18) and MRPP (multi-response permutation procedure) (19) were performed based on the Bray-Curtis distinct distance matrix.
Results
Patient Characteristics
Firstly, 20 children were selected for the G1 group, but 15 of the 20 subjects had other symptoms of IgA vasculitis within 6 months after being selected. Therefore, only 15 samples were included in the statistical analysis of the G1 group. The average ages of the G1 (n = 15), G2 (n = 15), G3 (n = 12), and healthy controls (C group, n = 15) were 5.1 ± 1.3 y, 5.4 ± 0.9 y, 6.3 ± 1.1 y, and 4.9 ± 0.7 y, respectively. The ratio of male to female is 1:1.14, 1:1.50, 1:0.71, and 1:0.88. There were no significant differences in age and gender among the three groups (t = 1.42, p > 0.05).
Intestinal Microbiota Diversity Among Children With Different Types of IgA Vasculitis
We used Illumina high-throughput sequencing to perform multiple pyrosequencing of the V4 hypervariable region of the 16S rRNA gene to characterize the bacterial lineage present in the fecal microbiota of all sample (20). We generated a data set consisting of 1,868,876 filtered 16S rRNA gene sequences, and the mean (±SE) of each sample was 32787.30 ± 3686.54 sequences (Figure 1, Table 1). According to the statistics of the patients' organ involvement (Table 2), children with IgA vasculitis with gastrointestinal damage have a higher rate of kidney damage. They also have fewer Sequence numbers at each classification level.
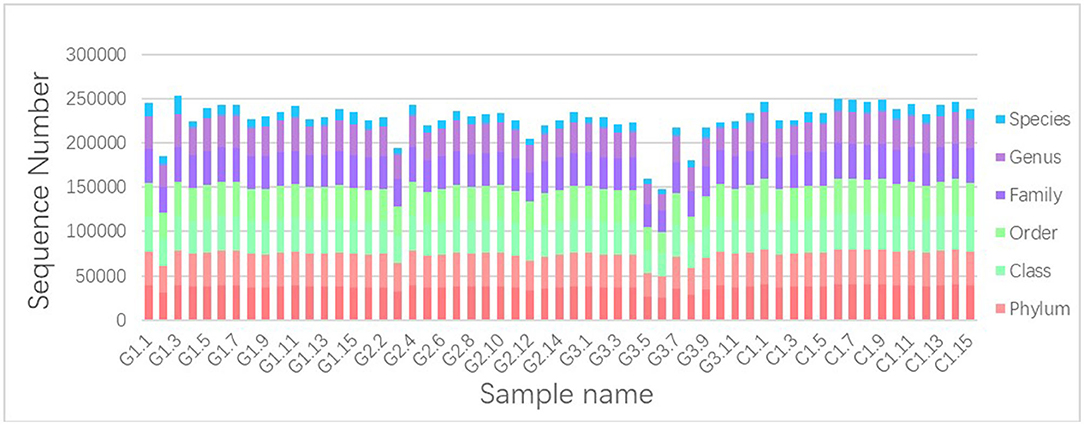
Figure 1. Number of gene sequences in each sample. The abscissa is the sample name; the ordinate indicates the number of sequences that are annotated to that level; the top-down color order of the histogram corresponds to the legend's color order on the right.
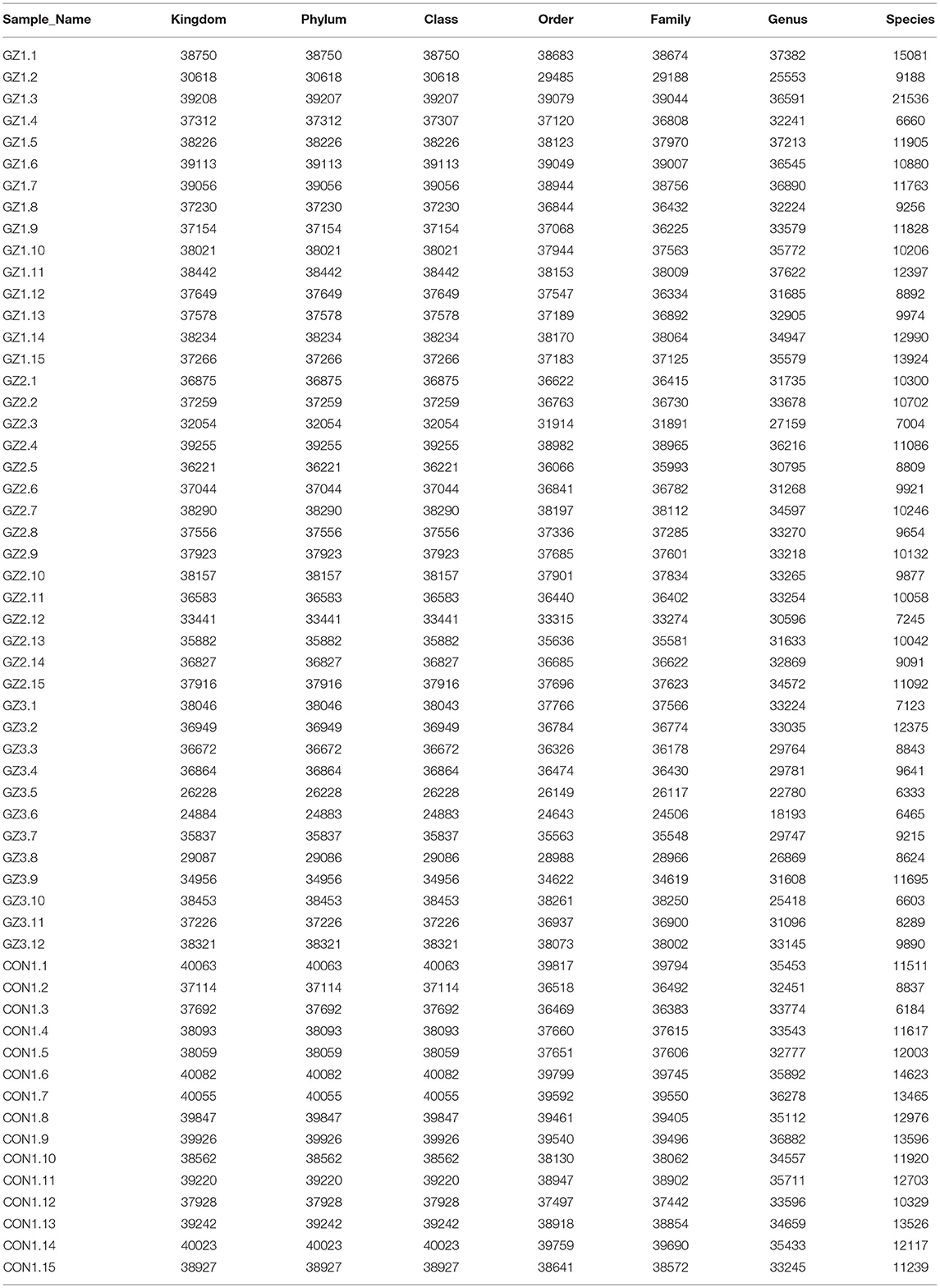
Table 1. Number of gene sequences in each sample (Figure 1 data supplement form).
More than 10 bacterial phyla were detected in all samples, and more than 92.6% of all samples were found to belong to the four most-populated bacterial phyla. They were Bacteroidetes, Firmicutes, Proteobacteria and Actinobacteria (Figure 2, Table 1). The Relative abundance of predominant intestinal flora in children with IgA vasculitis and healthy controls has been counted (Table 3). Bacteroidetes and Firmicutes were the two most abundant bacteria in all samples. The relative abundances of Firmicutes did not significantly differ in G1, G2, G3 and C groups (43.5, 36.3, 41.3, and 45.3%, respectively, Figure 3A). Similarly, the relative abundances of Bacteroidetes also did not significantly differ by study groups (47.2, 49.5, 45.3, and 38.7%, respectively, Figure 3D). In addition to Bacteroidetes and Firmicutes, the microbial population with a high proportion of abundance in the study population is Proteobacteria and Actinobacteria. The proportion of Actinobacteria in G1, G2, G3 and C groups were 4.8, 1.0, 1.0, and 9.8% (Figure 3B). And the proportion of Proteobacteria were 3.8 and 12.8%, 11.5 and 4.3% (Figure 3C), respectively. The relative abundance of Proteobacteria in the G2 and G3 groups was much higher than that in the G1 and C groups (P < 0.001), while the relative abundance of Actinobacteria was significantly lower than that in the G1 and C groups (P < 0.001). In addition, the relative abundances of Actinobacteria in G1 and C groups were also significantly different. The relative abundance Actinobacteria in G1 group was lower than that in C group (P < 0.001) (Table 1).
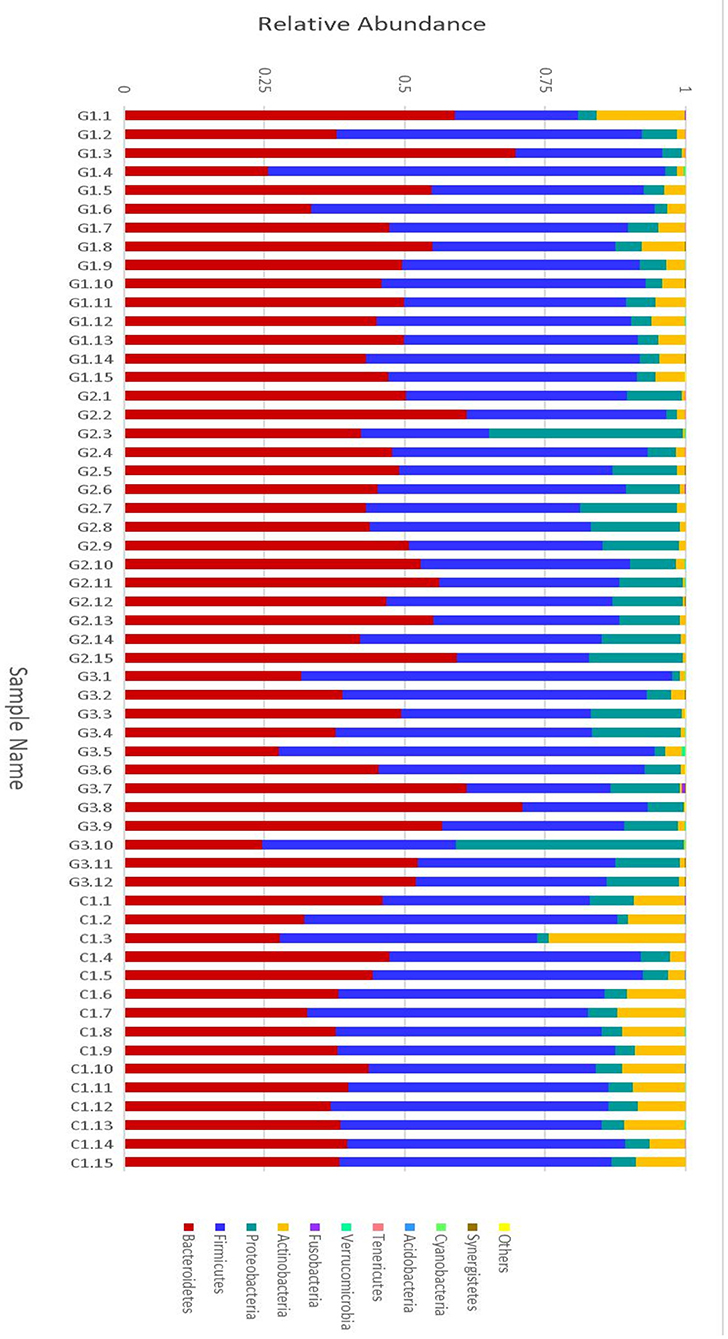
Figure 2. Relative abundance of the intestinal flora at the family level in children. The abscissa is the sample name; the ordinate indicates the relative abundance; “Others” represents the sum of the relative abundance of all the orders of the 10 largest phyla with the highest relative abundance (The maximum relative abundance of a phylum in all samples).
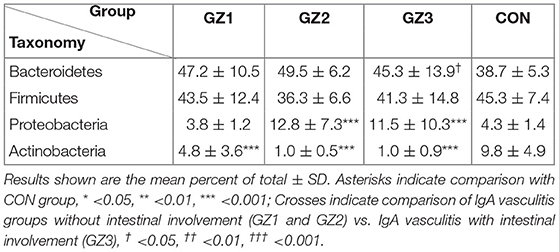
Table 3. Relative abundance of predominant intestinal flora in children with IgA vasculitis and healthy controls.
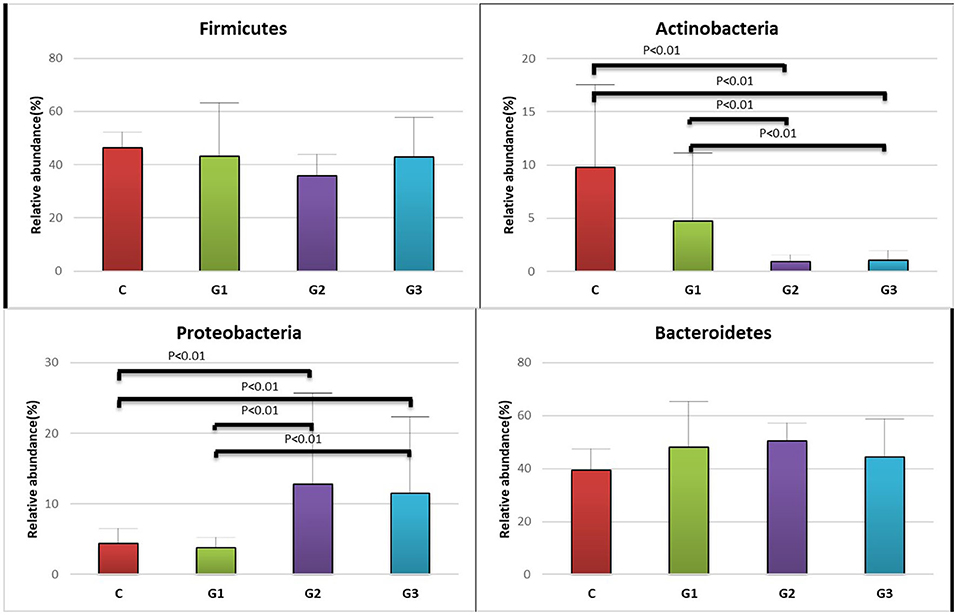
Figure 3. Relative abundance of the intestinal flora at the family level in the four groups of children (Firmicutes, Actinobacteria, Proteobacteria, Bacteroidetes). The abscissa is the group name; the ordinate indicates the percentage of abundance of a particular intestinal flora; the column color corresponds to the legend color on the right. (A) Relative abundance (%) of Firmicutes in the intestinal flora of the four groups of children. (B) Relative abundance (%) of Actinobacteria in the intestinal flora of the four groups of children. (C) Relative abundance (%) of Proteobacteria, in the intestinal flora of the four groups of children. (D) Relative abundance (%) of Bacteroidetes, in the intestinal flora of the four groups of children.
16S rRNA Genetic Survey Distinguishes Children With Different Types of IgA Vasculitis
Complete linkage hierarchical clustering divides most of the samples in the G1, G2, G3, and C groups into different clusters (Figures 4, 5). Of course, some of these samples are separated in other groups. However, this individual phenomenon has no significant impact on the analysis and judgment of the resulting trend. Notably, the samples were divided into several clusters. The resemblance between these clusters was low, which indicates that the intestinal microbiotas in these groups are different from one another. We have found a wealth of Bacteroides (Figure 2), in which the Enterobacteriaceae family has the highest relative abundance, in all four groups. We also observed abundant Firmicutes; it was found in all subjects (Figure 2) and this phylum was previously thought to be strictly related to children with other autoimmune diseases (21). The results showed a clear association between different clinical symptoms of IgA vasculitis and gut microbial composition.
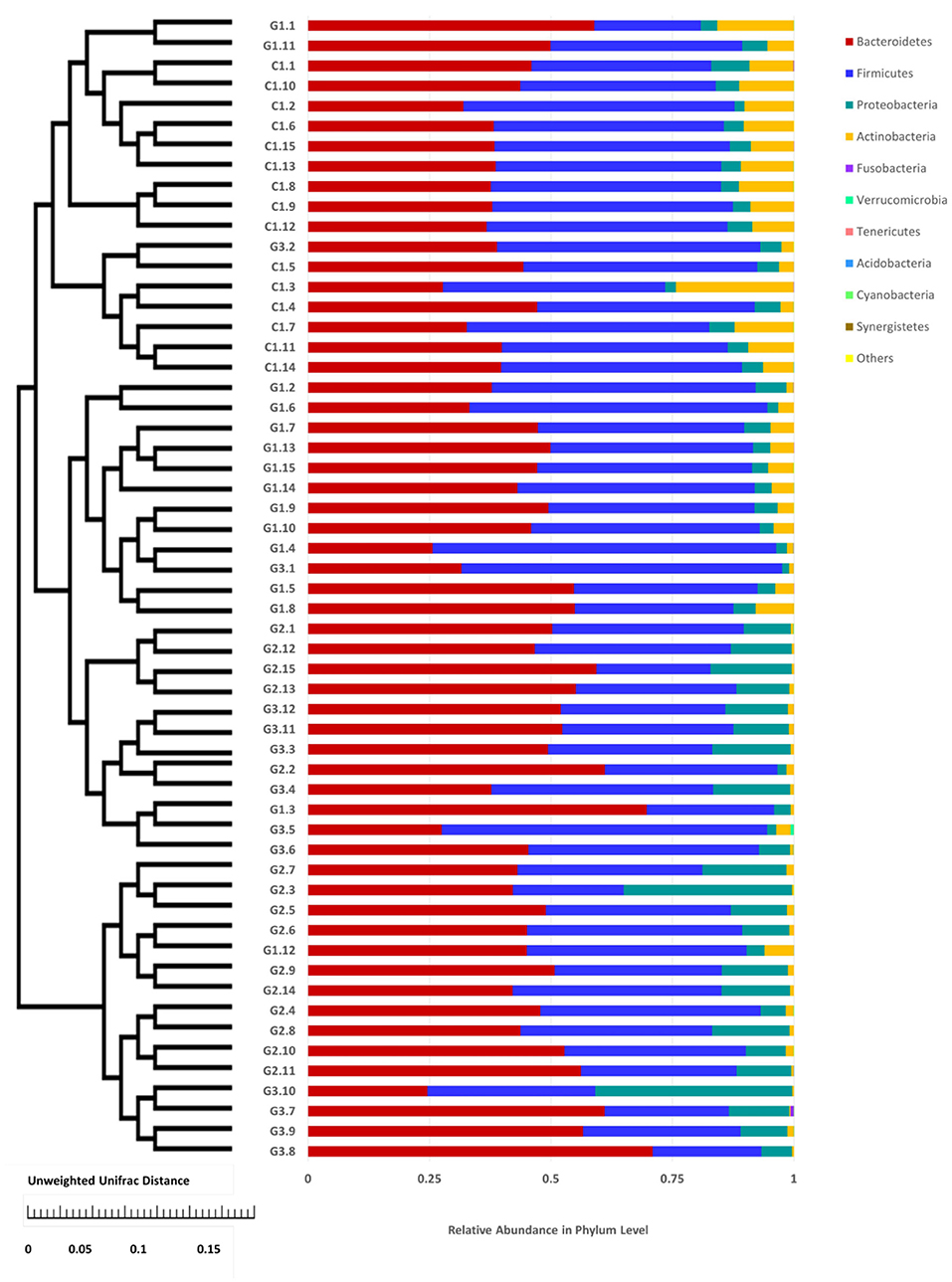
Figure 4. Cluster of relative abundance of the intestinal flora at the phylum level in each sample. The left side is the UPGMA cluster tree structure, on the right is the sample at the phylum level of the relative abundance of specie distribution map.
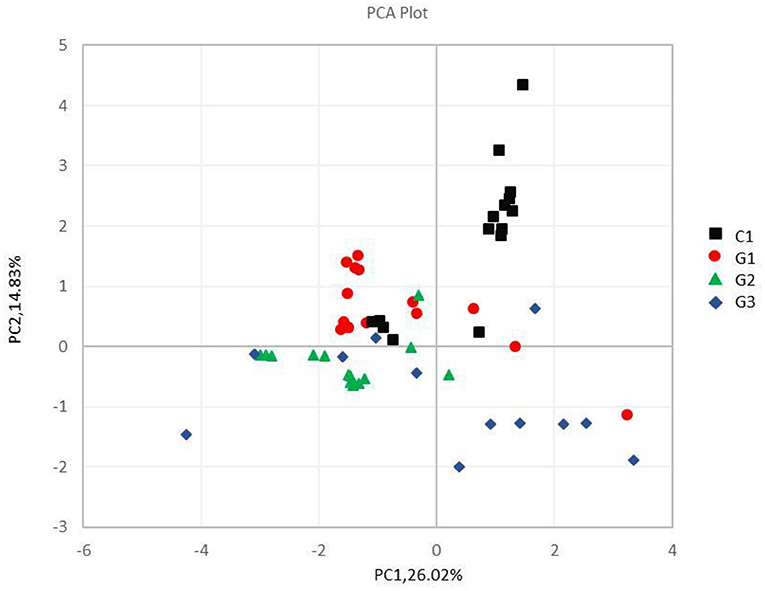
Figure 5. Principal component analysis of different samples at the phylum level. The abscissa and the ordinate represent two principal components, respectively, and the percentage indicates the contribution of the principal component to the sample difference; the same group of samples is represented by the same color dot.
Microbial Richness and Biodiversity
Rarefaction curves (Figure 6) showed a plateau in the number of new observed OTUs when the number of sequences evaluated for each sample was >10,000. Thus, evaluation of >24,000 sequences for each sample ensured that the sequencing data volume was sufficient to reflect the information of most microorganisms present in each sample.
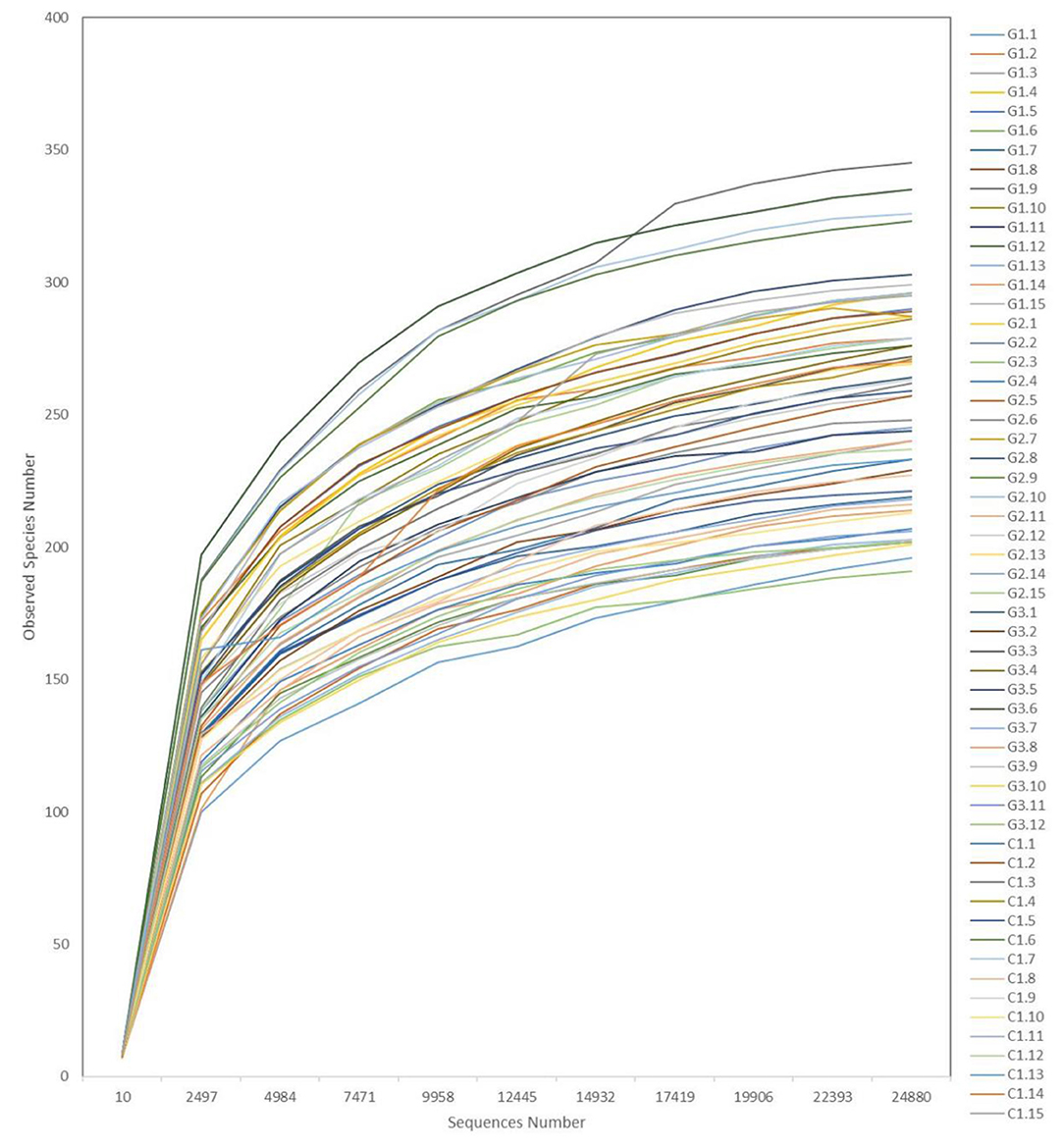
Figure 6. Representative rarefaction curves. The abscissa is the number of valid sequences per sample, the ordinate is the number of operational taxonomic units (OTUs) species observed. Each curve represents a different sample.
Discussion
The major findings of this study are (1) The abundance of intestinal microbes in children with IgA vasculitis is lower than in healthy children, (2) children with IgA vasculitis who present with different organ involvement show distinct patterns of intestinal microbiota, (3) Proteobacteria are increased and Actinobacteria are decreased in patients with IgA vasculitis who present with joint or intestinal symptoms compared to patients with only skin symptoms, (4) similar to other autoimmune diseases, Bacteroidetes, Firmicutes, Proteobacteria and Actinobacteria are the four most abundant bacteria in the intestinal flora of children with IgA vasculitis.
Human intestinal flora are typically composed of about 30 genera and more than 500 kinds of bacteria, which together play an important role in promoting food digestion and synthesizing protein, vitamins, and other nutrients, as well as in resisting the invasion of foreign pathogens and enhancing the immune system (22). The types, numbers, and distribution of normal flora vary due to differences in factors, such as age, race, dietary structure, and lifestyle. And both the structure and number of intestinal flora can be changed by a variety of diseases (23). Cumulatively, human gut bacteria contain more than three million genes, meaning 100 times more than human hosts (24). They have a variety of metabolic functions such as dietary component metabolism, drug detoxification, vitamins and essential amino acids synthesis (25). Different types of bacteria have different abilities in performing a certain metabolic function, that is, a specific microbiota is more effective or less effective in performing certain functions than other microbial groups (26). In recent years, increasingly more studies on perinatal and neonatal intestinal microbes have found that gestational age, delivery mode, and feeding methods each affect the composition of neonatal intestinal flora (27), and probiotics can effectively prevent the occurrence of enterocolitis (28). A variety of autoimmune diseases, such as multiple sclerosis (29) and autoimmune encephalitis (30), have also been confirmed as being impacted by intestinal microbial regulation.
The association of gut microbiota composition with IgA vasculitis and different clinical presentations of IgA vasculitis has not previously been studied. Our study used Illumina sequencing technology to study the composition of the intestinal flora of children with different clinical symptoms of IgA vasculitis and found that the microbial diversity in the feces of patients was high. The results show that more than 92.3% of genes in all samples belong to Bacteroidetes, Firmicutes, Proteobacteria, or Actinobacteria, which is in agreement with a previous study describing these phyla as composing the majority of gut microbiota found in children with other autoimmune diseases. The previous research also reported that the dominant human intestinal flora mainly belonged to the phyla Bacteroidetes and Firmicutes (31, 32), and our study found the same results.
Our study showed that the relative abundance of the gut microbiota in the CON group was higher than that in the IgA vasculitis group. This is consistent with the related research of other researchers (33). By comparing the microbial richness, we found that the abundance of intestinal bacteria was significantly lower in children with IgA vasculitis than in healthy children, and it was higher in children with other clinical manifestations of IgA vasculitis than in those with only purpura. This difference is more pronounced in children with IgA vasculitis who are involved in the gastrointestinal tract, especially in children with IgA vasculitis who have both gastrointestinal and renal damage. Previous work has applied metabolomics to querying the function of gut microbe communities in other autoimmune diseases. Studies of intestinal flora in patients with rheumatoid arthritis have shown that ion diversity in feces in patients with early rheumatoid arthritis (ERA) is significantly lower than in healthy controls (34). At the same time, similar findings are also reflected in other connective tissue diseases. Compared with healthy controls, patients with Sjögren's syndrome have lower metabolic diversity reported in saliva samples (35), and patients with psoriatic arthritis (36) and inflammatory bowel disease (IBD) (37) have lower intestinal microbiota diversity. In addition, studies of dextran sulfate-induced colitis mice have also shown that fecal insulin autoantibodies (IAA) levels are low (38).
Ather et al. reported that segmented Filamentous bacteria can promote the differentiation of Th17, resulting in an increased immune response (39). In our study, although Firmicutes tended to be represented slightly more in the intestinal flora of healthy children than in that of children with IgA vasculitis, these differences were not significant. However, we found significant differences in the abundance of Proteobacteria between healthy children and those with IgA vasculitis. Proteobacteria was not only more represented in the IgA vasculitis groups than in the CON group, but it was also found more frequently in children with IgA vasculitis who had mixed clinical syndromes than in those who had only skin injury. These results suggest that Proteobacteria may be another important group of bacteria that has an important effect on the regulation of immune disorders and on the remission of the associated disease. In contrast, the abundance of Actinobacteria was significantly lower in children with IgA vasculitis than in healthy controls, especially in those with mixed clinical syndromes. However, Bacteroides fragilis, which has been previously implicated in inducing the development of Tregs to suppress immune response (40), did not show the same trends. Therefore, further research is needed to determine whether or not Actinobacteria can affect the activity of immune cells and effectors.
In summary, the dominant human intestinal flora mainly belonged to the phyla Bacteroidetes and Firmicutes. As with several other autoimmune diseases, the incidence and progression of IgA vasculitis is also affected by the composition of gut microbiota. The number and abundance of intestinal microbes in children with IgA vasculitis are low, especially in children with multiple organs involvement including both gastrointestinal and renal damage. Proteobacteria and Actinobacteria may play an important role in the pathogenesis and prognosis of IgA vasculitis. The relative abundance of Actinobacteria was significantly reduced in all affected groups and was lower in children with multiple organ damage. Therefore, Actinobacteria may also be associated with the severity of children with IgA vasculitis. Treatment with active supplementation of this flora in the early stages of the disease may reduce organ damage and reduce the severity of IgA vasculitis. This study also has certain limitations such as small sample size. And no flora intervention therapy had been given because there was currently no suitable targeted flora. Further relevant supplements will be made in the follow-up research. In general, this research provided a new way to describe the pathogenesis of IgA vasculitis and may aid in the future development of microorganism treatments.
Conclusions
The abundance of intestinal microbes in children with IgA vasculitis is lower than in normal children. Bacteroidetes, Firmicutes, Proteobacteria and Actinobacteria are the four most abundant bacteria in the intestinal flora of children. Proteobacteria and Actinobacteria are associated with organ involvement in IgA vasculitis.
Data Availability Statement
The datasets presented in these studies can be found in online repositories. The names of the repository and accession number can be found below: NCBI Sequence Read Archive, accession SAMN22814273.
Ethics Statement
The studies involving human participants were reviewed and approved by Ethics Committee on Scientific Research of Shandong University Qilu Hospital. Written informed consent was obtained from the individual(s), and minor(s)' legal guardian/next of kin, for the publication of any potentially identifiable images or data included in this article.
Author Contributions
ML: writing—original draft preparation. XW: validation and data analysis. XB and RJ: conceptualization and methodology. AZ and AF: writing—review and editing. AZ: project administration. All authors contributed to the article and approved the submitted version.
Funding
This work was supported by Key Research and Development Program of Shandong Province (2019GSF108060), Shandong Provincial Natural Science Foundation (ZR202010220039).
Conflict of Interest
The authors declare that the research was conducted in the absence of any commercial or financial relationships that could be construed as a potential conflict of interest.
Publisher's Note
All claims expressed in this article are solely those of the authors and do not necessarily represent those of their affiliated organizations, or those of the publisher, the editors and the reviewers. Any product that may be evaluated in this article, or claim that may be made by its manufacturer, is not guaranteed or endorsed by the publisher.
References
1. Kaya Akca U, Batu ED, Serin O, Ipek OF, Aydin O, Teksam O, et al. Penile involvement of immunoglobulin a vasculitis/Henoch-Schönlein purpura. J Pediatr Urol. (2021) 17:409.e1–409.e8. doi: 10.1016/j.jpurol.2021.01.012
2. Borovitz Y, Alfandary H, Haskin O, Levi S, Kaz S, Davidovits M, et al. Lower prednisone dosing for steroid-sensitive nephrotic syndrome relapse: a prospective randomized pilot study. Eur J Pediatr. (2020) 179:279–83. doi: 10.1007/s00431-019-03506-5
3. Boutin RCT, Sbihi H, Dsouza M, Malhotra R, Petersen C, Dai D, et al. Mining the infant gut microbiota for therapeutic targets against atopic disease. Allergy. (2020) 75:2065–8. doi: 10.1111/all.14244
4. Leung AKC, Barankin B, Leong KF. Henoch-Schonlein purpura in children: an updated review. Curr Pediatr Rev. (2020) 16:265–76. doi: 10.2174/1573396316666200508104708
5. Dong G, Zhang J, Yang Z, Feng X, Li J, Li D, et al. The association of gut microbiota with idiopathic central precocious puberty in girls. Front Endocrinol. (2019) 10:941. doi: 10.3389/fendo.2019.00941
6. Zambrana LE, McKeen S, Ibrahim H, Zarei I, Borresen EC, Doumbia L, et al. Rice bran supplementation modulates growth, microbiota and metabolome in weaning infants: a clinical trial in Nicaragua and Mali. Sci Rep. (2019) 9:13919. doi: 10.1038/s41598-019-50344-4
7. Hao B, Wang K, Zhou Y, Sui C, Wang L, Bai R, et al. Label-free detecting of the compaction and decompaction of ctDNA Molecules induced by surfactants with SERS based on a nanoPAA-ZnCl2-AuLs solid substrate. ACS Omega. (2020) 5:1109–19. doi: 10.1021/acsomega.9b03294
8. Magoc T, Salzberg SL. FLASH: fast length adjustment of short reads to improve genome assemblies. Bioinformatics. (2011) 27:2957–63. doi: 10.1093/bioinformatics/btr507
9. Edgar RC. UPARSE: highly accurate OTU sequences from microbial amplicon reads. Nat Methods. (2013) 10:996–8. doi: 10.1038/nmeth.2604
10. Liu W, Zhang R, Shu R, Yu J, Li H, Long H, et al. Study of the relationship between microbiome and colorectal cancer susceptibility using 16SrRNA sequencing. Biomed Res Int. (2020) 2020:7828392. doi: 10.1155/2020/7828392
11. Caporaso JG, Bittinger K, Bushman FD, DeSantis TZ, Andersen GL, Knight R. PyNAST: a flexible tool for aligning sequences to a template alignment. Bioinformatics. (2010) 26:266–7. doi: 10.1093/bioinformatics/btp636
12. Son HJ, Kim N, Song CH, Nam RH, Choi SI, Kim JS, et al. Sex-related alterations of gut microbiota in the C57BL/6 mouse model of inflammatory bowel disease. J Cancer Prev. (2019) 24:173–82. doi: 10.15430/JCP.2019.24.3.173
13. Geng H, Shu S, Dong J, Li H, Xu C, Han Y, et al. Association study of gut flora in Wilson's disease through high-throughput sequencing. Medicine. (2018) 97:e11743. doi: 10.1097/MD.0000000000011743
14. Zhao MM, Du SS, Li QH, Chen T, Qiu H, Wu Q, et al. High throughput 16SrRNA gene sequencing reveals the correlation between Propionibacterium acnes and sarcoidosis. Respir Res. (2017) 18:28. doi: 10.1186/s12931-017-0515-z
15. White JR, Nagarajan N, Pop M. Statistical methods for detecting differentially abundant features in clinical metagenomic samples. PLoS Comput Biol. (2009) 5:e1000352. doi: 10.1371/journal.pcbi.1000352
16. Moreno-Arrones OM, Serrano-Villar S, Perez-Brocal V, Saceda-Corralo D, Morales-Raya C, Rodrigues-Barata R, et al. Analysis of the gut microbiota in alopecia areata: identification of bacterial biomarkers. J Eur Acad Dermatol Venereol. (2020) 34:400–5. doi: 10.1111/jdv.15885
17. Quagliariello A, Del Chierico F, Russo A, Reddel S, Conte G, Lopetuso LR, et al. Gut microbiota profiling and gut-brain crosstalk in children affected by pediatric acute-onset neuropsychiatric syndrome and pediatric autoimmune neuropsychiatric disorders associated with streptococcal infections. Front Microbiol. (2018) 9:675. doi: 10.3389/fmicb.2018.00675
18. Garza-Gonzalez E, Mendoza-Olazaran S, Morfin-Otero R, Ramirez-Fontes A, Rodriguez-Zulueta P, Flores-Trevino S, et al. Intestinal microbiome changes in fecal microbiota transplant (FMT) vs FMT enriched with Lactobacillus in the treatment of recurrent clostridioides difficile Infection. Can J Gastroenterol Hepatol. (2019) 2019:4549298. doi: 10.1155/2019/4549298
19. Citronberg JS, Curtis KR, White E, Newcomb PA, Newton K, Atkinson C, et al. Association of gut microbial communities with plasma lipopolysaccharide-binding protein (LBP) in premenopausal women. ISME J. (2018) 12:1631–41. doi: 10.1038/s41396-018-0064-6
20. Bai H, Jin W, Guo J, Ding Y, Chang C, Guo X, et al. lncRNA expression reveals the potential regulatory roles in hepatocyte proliferation during rat liver regeneration. Biomed Res Int. (2019) 2019:8597953. doi: 10.1155/2019/8597953
21. Tremlett H, Fadrosh DW, Faruqi AA, Hart J, Roalstad S, Graves J, et al. Associations between the gut microbiota and host immune markers in pediatric multiple sclerosis and controls. BMC Neurol. (2016) 16:182. doi: 10.1186/s12883-016-0703-3
22. Postler TS, Ghosh S. Understanding the holobiont: how microbial metabolites affect human health and shape the immune system. Cell Metab. (2017) 26:110–30. doi: 10.1016/j.cmet.2017.05.008
23. Wang X, Zhang L, Wang Y, Liu X, Zhang H, Liu Y, et al. Gut microbiota dysbiosis is associated with henoch-schonlein purpura in children. Int Immunopharmacol. (2018) 58:1–8. doi: 10.1016/j.intimp.2018.03.003
24. Holman DB, Bearson BL, Allen HK, Shippy DC, Loving CL, Kerr BJ, et al. Chlortetracycline enhances tonsil colonization and fecal shedding of multidrug-resistant Salmonella enterica serovar typhimurium DT104 without major alterations to the porcine tonsillar and intestinal microbiota. Appl Environ Microbiol. (2019) 85:e02354–18. doi: 10.1128/AEM.02354-18
25. Sakkas H, Bozidis P, Touzios C, Kolios D, Athanasiou G, Athanasopoulou E, et al. Nutritional status and the influence of the vegan diet on the gut microbiota and human health. Medicina. (2020) 56:88. doi: 10.3390/medicina56020088
26. Zhang Y, Xia G, Nie X, Zeng Y, Chen Y, Qian Y, et al. Differences in manifestations and gut microbiota composition between patients with different henoch-schonlein purpura phenotypes. Front Cell Infect Microbiol. (2021) 11:641997. doi: 10.3389/fcimb.2021.641997
27. Collado MC, Cernada M, Neu J, Perez-Martinez G, Gormaz M, Vento M. Factors influencing gastrointestinal tract and microbiota immune interaction in preterm infants. Pediatr Res. (2015) 77:726–31. doi: 10.1038/pr.2015.54
28. Chang HY, Chen JH, Chang JH, Lin HC, Lin CY, Peng CC. Multiple strains probiotics appear to be the most effective probiotics in the prevention of necrotizing enterocolitis and mortality: An updated meta-analysis. PLoS ONE. (2017) 12:e0171579. doi: 10.1371/journal.pone.0171579
29. Buscarinu MC, Fornasiero A, Romano S, Ferraldeschi M, Mechelli R, Renie R, et al. The Contribution of gut barrier changes to multiple sclerosis pathophysiology. Front Immunol. (2019) 10:1916. doi: 10.3389/fimmu.2019.01916
30. Weissert R. Adaptive immunity is the key to the understanding of autoimmune and paraneoplastic inflammatory central nervous system disorders. Front Immunol. (2017) 8:336. doi: 10.3389/fimmu.2017.00336
31. Mushtaq N, Hussain S, Zhang S, Yuan L, Li H, Ullah S, et al. Molecular characterization of alterations in the intestinal microbiota of patients with grade 3 hypertension. Int J Mol Med. (2019) 44:513–22. doi: 10.3892/ijmm.2019.4235
32. Bradley PH, Pollard KS. Proteobacteria explain significant functional variability in the human gut microbiome. Microbiome. (2017) 5:36. doi: 10.1186/s40168-017-0244-z
33. Cao J, Wu C, Wang K, Hu H, Duan J, Zhao B, et al. Metagenomic profiling reveals dominance of gram-positive bacteria in the gut microbiome shifts associated with immunoglobulin A vasculitis (Henoch-Schonlein Purpura). Clin Transl Immunology. (2021) 10:e1342. doi: 10.1002/cti2.1342
34. Stoll ML, Kumar R, Lefkowitz EJ, Cron RQ, Morrow CD, Barnes S. Fecal metabolomics in pediatric spondyloarthritis implicate decreased metabolic diversity and altered tryptophan metabolism as pathogenic factors. Genes Immun. (2016) 17:400–5. doi: 10.1038/gene.2016.38
35. Kageyama G, Saegusa J, Irino Y, Tanaka S, Tsuda K, Takahashi S, et al. Metabolomics analysis of saliva from patients with primary Sjogren's syndrome. Clin Exp Immunol. (2015) 182:149–53. doi: 10.1111/cei.12683
36. Boer CG, Radjabzadeh D, Medina-Gomez C, Garmaeva S, Schiphof D, Arp P, et al. Intestinal microbiome composition and its relation to joint pain and inflammation. Nat Commun. (2019) 10:4881. doi: 10.1038/s41467-019-12873-4
37. Lavelle A, Sokol H. Gut microbiota-derived metabolites as key actors in inflammatory bowel disease. Nat Rev Gastroenterol Hepatol. (2020) 17:223–37. doi: 10.1038/s41575-019-0258-z
38. Shiomi Y, Nishiumi S, Ooi M, Hatano N, Shinohara M, Yoshie T, et al. GCMS-based metabolomic study in mice with colitis induced by dextran sulfate sodium. Inflamm Bowel Dis. (2011) 17:2261–74. doi: 10.1002/ibd.21616
39. Yi J, Jung J, Han D, Surh CD, Lee YJ. Segmented filamentous bacteria induce divergent populations of antigen-specific CD4 T cells in the small intestine. Mol Cells. (2019) 42:228–36. doi: 10.14348/molcells.2018.0424
Keywords: children, gut microbiota, sequencing, immune disease, IgA vasculitis
Citation: Li M, Wang X, Lin X, Bian X, Jing R, Frelinger A and Zhang A (2022) Comparison and Analysis of Gut Microbiota in Children With IgA Vasculitis With Different Clinical Symptoms. Front. Pediatr. 9:800677. doi: 10.3389/fped.2021.800677
Received: 27 October 2021; Accepted: 18 November 2021;
Published: 07 January 2022.
Edited by:
Ozgur Kasapcopur, Istanbul University-Cerrahpasa, TurkeyReviewed by:
Rabia Miray Kisla Ekinci, Ministry of Health, TurkeySezgin Sahin, Istanbul University-Cerrahpasa, Turkey
Copyright © 2022 Li, Wang, Lin, Bian, Jing, Frelinger and Zhang. This is an open-access article distributed under the terms of the Creative Commons Attribution License (CC BY). The use, distribution or reproduction in other forums is permitted, provided the original author(s) and the copyright owner(s) are credited and that the original publication in this journal is cited, in accordance with accepted academic practice. No use, distribution or reproduction is permitted which does not comply with these terms.
*Correspondence: Andrew Frelinger, QW5kcmV3LkZyZWxpbmdlckBjaGlsZHJlbnMuaGFydmFyZC5lZHU=; Aijun Zhang, emhhbmdhaWp1bkBzZHUuZXVkLmNu
†These authors have contributed equally to this work