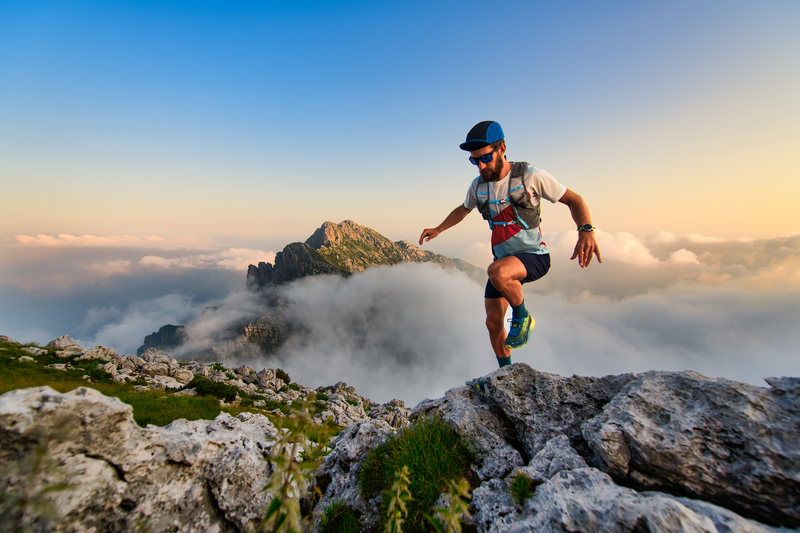
94% of researchers rate our articles as excellent or good
Learn more about the work of our research integrity team to safeguard the quality of each article we publish.
Find out more
REVIEW article
Front. Pediatr. , 20 October 2021
Sec. Child and Adolescent Psychiatry
Volume 9 - 2021 | https://doi.org/10.3389/fped.2021.685660
This article is part of the Research Topic Obsessive-Compulsive Disorder (OCD) Across the Lifespan: Current Diagnostic Challenges and the Search for Personalized Treatment View all 26 articles
Pathogenesis of obsessive-compulsive disorder (OCD) mainly involves dysregulation of serotonergic neurotransmission, but a number of other factors are involved. Genetic underprints of OCD fall under the category of “common disease common variant hypothesis,” that suggests that if a disease that is heritable is common in the population (a prevalence >1–5%), then the genetic contributors—specific variations in the genetic code—will also be common in the population. Therefore, the genetic contribution in OCD is believed to come from multiple genes simultaneously and it is considered a polygenic disorder. Genomics offers a number of advanced tools to determine causal relationship between the exposure and the outcome of interest. Particularly, methods such as polygenic risk score (PRS) or Mendelian Randomization (MR) enable investigation of new pathways involved in OCD pathogenesis. This premise is also facilitated by the existence of publicly available databases that include vast study samples. Examples include population-based studies such as UK Biobank, China Kadoorie Biobank, Qatar Biobank, All of US Program sponsored by National Institute of Health or Generations launched by Yale University, as well as disease-specific databases, that include patients with OCD and co-existing pathologies, with the following examples: Psychiatric Genomics Consortium (PGC), ENIGMA OCD, The International OCD Foundation Genetics Collaborative (IOCDF-GC) or OCD Collaborative Genetic Association Study. The aim of this review is to present a comprehensive overview of the available Big Data resources for the study of OCD pathogenesis in the context of genomics and demonstrate that OCD should be considered a disorder which requires the approaches offered by personalized medicine.
Obsessive-compulsive disorder (OCD) is a common disorder with a population prevalence of 2–3% (1). Moreover, up to 13% of adults experience some kind of obsessive-compulsive behaviors (OCB) during their lifetime (1). Obsessive-compulsive disorder has chronic course with child onset in 50–70% of cases, typically associated with significant impairment and comorbidity. The spectrum of obsessive-compulsive (OC) symptoms varies from non-bothersome intrusive thoughts and compulsive behaviors (OCB) to full-blown OCD. Irrespective of where someone falls along the spectrum, a primary contributing factor to this OC spectrum is dysregulation of neurotransmission, mainly the serotonergic system (2–4). Nevertheless, other factors have also been found to influence the occurrence of OCD (5, 6), in particular, brain injury (7), toxicity (8), infection, and autoimmunity, especially in context of pediatric autoimmune neuropsychiatric disorders associated with streptococcal infections (PANDAS) and pediatric acute-onset neuropsychiatric syndrome (PANS) (9, 10) and genetics (11). A number of candidate genes have been proven to play a role in pathogenesis of OCD, mainly related to serotonergic, glutamatergic, and dopaminergic pathways (12), but recent studies have demonstrated that OCD occurrence is multifactorial and probably is a consequence of gene/environment interactions (13, 14). Moreover, although it has been assumed that serotonergic mechanisms are important for OCD, this still needs to be empirically proven by unbiased genome wide association studies (GWAS).
A surge of new genetic technologies, such as GWAS, has enabled much more precise analysis of the genetic underprints of diseases. Genome wide association studies is an observational study of a genome-wide set of genetic variants in different individuals to determine if any variant is associated with a trait of interest (15). Genome wide association studies typically focuses on associations between single nucleotide polymorphisms (SNPs) and the outcome of interest. Consequently, SNPs are a substitution of a single nucleotide at a specific position in the genome that is present in a sufficiently large fraction of the population (16). Furthermore, blooming of advanced statistical and mathematical methods facilitate even more precise discoveries in the area of genetics. In particular, a method called Mendelian Randomization (MR) (17) enables establishment of casual relationship between genetically determined risk factors and the phenotype of interest, in this case, OCD. Moreover, by using external GWAS results with a list of SNPs that have reached genome wide significance and genotyping a particular individual it is possible to estimate genetic risk related to an outcome of interest. This risk is described as a numeric value denominated polygenic risk score (PRS) (18). Genome wide association studies, MR, PRS, and SNPs are the basic terms used in population genetics, the field of genetics that derives from epidemiological studies. Creation of research consortia that enable agglomeration of more and more data is a key mechanism that facilitates research in this area. At the same time, scientists are facing the problem of too many data that, due to its huge volume, are denominated Big Data. The concept of Big Data falls under the umbrella of the acronym “3 V” model: volume, velocity and variety. Rapid development of all aforementioned areas also has repercussions on the discoveries regarding pathophysiology of OCD.
The aim of this review is to present an overview of the available consortium and Big Data resources gathering OCD-related data and how these, and other resources are used to unravel genetics of OCD. Finally, we would like to demonstrate how tools provided by population genetics and genomics enable personalized diagnosis and treatment of OCD.
Population genetics offers powerful possibilities to overcome limitations of observational studies and demonstrate causal inference. As mutations are randomly distributed during meiosis, mutation-disease associations are not influenced by confounding post-natal factors. Population genetics uses a number of techniques and analytical methods that enable determination of casual link between the exposure and the outcome. Candidate gene studies were the main method to test associations between genes and diseases before the development of more advanced genotyping technologies (19). This approach is hypothesis-driven and is supported by the specific supposition in which specific biological pathway is related to the final phenotype. Genome wide association studies, on the other hand, are not precluded by the hypothesis-driven approach as they enable investigation of millions of SNPs across the genome for association with a particular disease. In this case, it is standard to use an adjusted threshold for statistical significance of p < 5 × 10e−8 to account for the approximately 1 million independent loci found across the human genome. Genome wide association studies technique enabled creation of another important statistical tool, PRS. This allows creation of scores that summarize the load of mutations related to a specific trait. Polygenic risk score is a sum of risk alleles for a given person, which is often more powerful predictor of disease occurrence than an individual SNP. Finally, MR is a statistical method aimed at determining and quantifying causal relationships between genetically-determined exposures and outcomes of interest (20). Importantly, in contrast to randomized clinical trials, the most frequently used tool to evaluate causality, MR can be performed using already available open-access data from different sources, allowing the evaluation of larger numbers of possible mechanisms and accelerating the speed of the translational cycle.
The growing amount of data and information in field of medicine is offering a number of new opportunities, but is also a major challenge, both in terms of data storage as well as analysis. Expansive development and use of new technologies, adopted from the fields of bioinformatics, statistics, and mathematics, help scientists analyze these data in a proper manner, and interpret the results. Moreover, the new research philosophy, based on the construction of large international and intercontinental consortia, permits researchers to overcome previous methodological limitations, mainly related to small sample size. Importantly, in accordance with the inclusive nature of research, more and more data are publicly available. As vast majority of common diseases, such as cardiovascular conditions or mental disorders, are multifactorial; they are result of the complex interactions of genes and environment (21). These disorders also fall under the category of the common disease, common variant hypothesis (21), which argues that genetic variations with appreciable frequency in the population at large, but with relatively low penetrance, are the major contributors to genetic susceptibility to common diseases. This means that large samples are required to study associations between these exposures and disease, and to identify targets for treatment and prevention.
In recent years, several population-based initiatives were initiated in order to collect demographic, epidemiological, clinical, neuroimaging, biomarkers, and genetic data. The most relevant examples include such projects as UK Biobank, All of US Program sponsored by National Institute of Health, Generations by the Yale University, China Kadoorie Biobank or Qatar Biobank. All of these databases contain data about psychiatric health and symptoms and could be used to investigate a number of questions related to OCD pathophysiology. The most robust and well-described study is UK Biobank (www.ukbiobank.ac.uk). UK Biobank is a population-based cohort and biobank investigating contributions of genetic predisposition and environmental exposure to the development of disease. The study was initiated in 2006, included over half a million people aged 40–69 years at onset, and proposed long-term follow-ups. Recruitment was finalized in 2010 and the resource is constantly growing. In 2017, genotyping of all participants was completed (22), in 2019 a wide range of biomarkers was released, and multimodal neuroimaging for almost 100,000 participants is gradually being published (23). The design of the UK Biobank study facilitates exploration of an extensive range of diverse risk factors and outcomes and provides tools to detect small effects in a large study sample. Importantly, UK Biobank also provides baseline and follow-up data on mental disorders, including OCD (24).
Several population-based studies were launched in the US. National Institute of Health initiated All Of US (https://allofus.nih.gov/) program which aims to enroll 1 million adults across the US. This study was initiated in 2015 under the government of Barack Obama and is a reflection of the efforts aiming to popularize precision medicine. As indicated in Carrosco-Ramiro et al. (25), precision or personalized medicine derives from the advances in genetic/genomic techniques and the completion of the Human Genome Project (HGP). Precision medicine incorporates information from genome sequencing and clinical data which enables therapy adjustment according to patient's own genome and environmental factors. Importantly, precision medicine is executed in line with the following premises: predictive, preventive, personalized, and participatory (P4). All Of US is destinated to facilitate the implementation of the P4 principles on a population level. Therefore, participation in this project is voluntary, independent of sex, gender, or ethnicity, and reflects the rich diversity of the US. The study is totally transparent as each participant receives individual results, including their genetic data. Participants provide clinical data and can provide additional access to their electronic health records (EHR) which include all their information about health problems as well as any medications they take. Blood and urine samples, as well as physical measurements, including those gathered by wearable devices, are also collected. In the future this program is planned to facilitate execution of clinical trials. In addition, blood samples are genotyped. By June 2020, enrollment reached approximately 350,000 individuals. Eighty percent of those people are from groups that have been traditionally underrepresented in biomedical research making All of US the first study focused on diversity. The Million Veteran Program (26) (https://www.mvp.va.gov/) is another innovative study sponsored by the Department of Veterans Affairs Office of Research and Development. So far, it has been possible to enroll 825,000 individuals. Similar to previous cohorts, demographic and clinical data, as well as biological samples were collected. Importantly, genotyping has already been conducted and enables testing of many hypotheses related to psychiatric diseases (27). Yale's Generations project (https://medicine.yale.edu/ycci/trial/6326/) was launched in 2019 and is targeted to be another precision medicine cohort. It will gather genetic and clinical data from at least 100,000 participants, including pediatric participants. DNA patterns will be linked to EHR.
Another important resource is China Kadoorie Biobank (https://www.ckbiobank.org/). It enabled the acquisition of genomic and clinical data on chronic diseases in half a million Chinese participants (28). The baseline data collection was carried out in years 2004–08 and included biological samples, along with demographic and clinical data. Participants were aged 30–79 years old. A select subset of participants is also retested every few years. Similar to UK Biobank, data regarding mental disorders are also available (29–31). Analogous projects were also launched in Japan (https://biobankjp.org/english/index.html) and Qatar (https://www.qatarbiobank.org.qa/home).
All in all, Big Data resources enable quick and unlimited access to previously restricted resources as researchers from all over the world can solicit permit to work on the data of interest. This can lead to democratization of science. Moreover, thanks to these resources, it is possible to investigate both common and rare diseases. Finally, the sample size is large enough to achieve previously unthinkable statistical power. The majority of biobanks offer not only information at baseline, but also follow-up, which enable high-quality longitudinal analysis. Finally, biobanks, in contrast to cohort studies, gather complex clinical, neuroimaging, and genetic data, not only about one restricted disease, but whole variety of phenotypes or even enroll mainly healthy individuals. Good example is previously described UK Biobank aiming to enroll any middle aged individual or Health and Retirement Study at University of Michigan, study investigating the dynamics of aging. As a result, information provided by Big Data resources is more approximated to the distribution of phenotypes and risk factors in the population.
Apart from population-based Biobanks, a number of cohorts related to OCD and/or mental health could be used to investigate OCD phenotype variability. However, the results obtained from diverse studies differ due to differences in the sample collection and diverse description of clinical phenotype. For example, ENIGMA OCD protocol includes only participants with available neuroimaging data while the majority of other cohorts did not include this criterion. Therefore, these data have to be interpreted with caution, taking together all the limitations mentioned.
Psychiatric Genomics Consortium (PGC) (https://www.med.unc.edu/pgc/) (32–34) incorporates more than 800 scientists worldwide coming from more than 150 institutions and 40 countries. One of the nine disorders working groups is dedicated to OCD and Gilles de la Tourette syndrome (GTS) and is headed by primary investigators in genetics of these disorders, Jeremiah Scharf and Manuel Mattheisen (13, 35). Participation in PGC is inclusive as anyone willing to contribute with samples can take part in the entire data analysis. The majority of data are available upon request.
The International OCD Foundation Genetics Collaborative (IOCDF-GC) (https://iocdf.org/programs/genetics/) (13) is a group of genetics investigators from North America, South America, Europe and Africa who collect data from OCD patients for genetic analysis, including GWAS (detailed results presented in section Genomics of Obsessive-Compulsive Disorder).
OCD Collaborative Genetic Association Study (OCGAS) (36) is a six-site, collaborative, genetic linkage study of OCD. Specimens and blinded clinical data are made available through the National Institute of Mental Health repository. In this project, clinical data and blood specimens were collected from 238 families containing 299 OCD-affected sibling pairs and their parents, and additional affected relative pairs, for a GWAS (detailed results presented in section Genomics of Obsessive-Compulsive Disorder).
ENIGMA OCD (http://enigma.ini.usc.edu/ongoing/enigma-ocd-working-group/) (37– 40) currently consists of 47 samples from 34 institutes in 15 countries on 5 continents, with a total sample of 2,323 OCD patients and 2,325 healthy controls. The main aim of this consortium is to collectively analyze brain imaging, clinical, and genetic data. Initially formed to detect genetic influences on brain measures, ENIGMA has grown to over 30 working groups studying 12 major brain diseases and comparing brain data. The total number of enrolled subjects so far is of 2,323 OCD patients and 2,325 healthy controls. Although vast majority of studies focused on different modalities of neuroimaging investigating subcortical volume (41), cortical thickness (42), structural connectivity (38), or brain lateralization (37), there are reports about correlation between genomic and neuroimaging data (43, 44). Recent efforts have focused on using modern technologies, in particular machine learning (39).
Table 1 summarizes Big Data resources in population genetics and related to OCD, in particular.
As mentioned in the introduction, research on complex diseases has been revolutionized by GWAS, which enables the simultaneous analysis of SNPs and the search for statistical relationships between them. This type of analysis, based on the achievements of modern genomic technologies, goes beyond the possibilities of candidate gene association studies and creates the possibility to discover genetic risk factors for diseases without the need to select specific genes and formulate a priori hypotheses (58). The main difference between genomics and genetics is that genetics focuses on functioning and composition of the single gene whereas genomics addresses all genes and their relationships to each other in order to identify their combined influence on the growth and development of the organism (59). In the following sections we discuss studies tackling the topic of genomics of OCD (Table 2). Findings provided by studies targeting the genomics of OCD are of great importance since only these studies could help to unravel complex genetic architecture of OCD. As a consequence, they can help to find pathophysiological pathways involved in the occurrence of OCD and plan treatment, especially in the context of personalized medicine. Nevertheless, results of these studies are often contradictory as studies included different sample size and included participants with diverse phenotype. This is the case for other GWAS examing genetic background of heterogenous traits, such as height (60), diabetes (61), and schizophrenia (62).
Important attempt to determine the genetic variation responsible for OCD was a study performed by Stewart et al. (45). To tackle this problem, IOCDF collected a set of individuals affected with OCD, diagnosed according to Diagnostic and Statistical Manual of Mental Disorders (DSM-IV) criteria, a subset of their parents, and unselected controls. Participants were then genotyped with Illumina SNP microarrays, which reduced the group to 1,465 cases, 5,557 ancestry-matched controls, and 400 parent–child trios. Study revealed a significant enrichment of methylation quantitative trait locus (QTLs) (p < 0.001) and frontal lobe expression quantitative trait loci (eQTLs) (p = 0.001) within the top-ranked SNPs (p < 0.01) in the combined trio-case-control sample, but no SNPs associated with OCD at a genome-wide significance level were recognized. The analysis including trios one SNP, rs6131295, located near the BTB domain-containing 3 (BTBD3) gene, reached genome wide statistical significance (p = 3.8 × 10−8), but in the combined trio-case-control meta-analysis this significance was not maintained. The abovementioned SNP is an eQTL for BTBD3, dehydrogenase/reductase 11 (DHRS11), and isthmin 1 (ISM1) genes. BTBD3 is a member of the transcription factors family and its functions include cytoskeleton dynamics, ion channel modulation, and protein degradation. DHRS11 and ISM1 are highly correlated with the expression of some of the other genes that have been identified among the top outcomes of both the case—control and trio—control meta-analysis and are linked to glutamatergic neurotransmission and signaling. Although no significant genome-wide correlations have been found in the whole sample, the findings indicate that BTBD3, FAIM2, correlated with DHRS11, and adenylate cyclase type 8 gene (ADCY8), correlated with ISM1, may be active in OCD pathogenesis. In addition, the top two SNPs with the lowest p-values were mapped within DLGAP1, a gene homologous to SAPAP, involved in the post-synaptic density of glutamatergic synapses.
Another GWAS was conducted by the OCGAS and published by Mattheisen et al. (46). This study is comprised of 1,406, comprehensively assessed, early onset, OCD patients combined with population-based samples. The smallest p-value (p = 4.13 × 10−7) was observed for the locus rs4401971, mapped near protein tyrosine phosphatase receptor type D (PTPRD) gene, which is responsible for differentiation of glutamatergic and, together with SLIT and NTRK Like Family Member 3 (SLITRK3) gene, GABAergic synapses. The second strongest correlation result was located in the cadherin cluster area. Compared to the hit regions found in the GWAS performed by Stewart et al. (45), 12 of the 15 strongest signals in the sample demonstrated correlations with the same direction of effects (sign test p = 0.0176). In regard to the region of Discs Large Homolog Associated Protein 1 (DLGAP1) gene, different outcomes were obtained. In the case-control analysis conducted by IOCDF-GC (45), signals in this gene reached top value. Even though in a study by Mattheisen et al. (46) the same significance was not detected, a nearby marker showed a significant value of p = 2.67 × 10−4, suggesting the association exists. Moreover, the region containing Glutamate Ionotropic Receptor Kainate Type Subunit 2 (GRIK2) gene, which rendered as a top signal in IOCDF-GC study, showed nominal, although not experiment-wide (p = 0.045), significance in the one performed by OCGAS (46). Finally, in a gene-set study for high-confidence interactions (51), the DLGAP1 and GRIK2 revealed a pattern of association and pointed to the possible role of DLGAP1 and GRIK2 interactors in the etiology of OCD, including genes such as Neuronal Differentiation 6 (NEUROD6) gene, Synaptic Vesicle Glycoprotein 2A gene (SV2A), Glutamate Ionotropic Receptor AMPA Type Subunit 4 gene (GRIA4), and Solute Carrier Family 1 Member 2 gene (SLC1A2). Other associations have been found with IQ Motif Containing K (IQCK) gene (p < 1 × 10−6 with experiment-wide significance) and Orofacial Cleft 1 Candidate 1 (OFCC1) (p = 6.29 × 10−5).
The two above-mentioned studies were meta-analyzed (13), and the results detected an absence of genome-wide significant SNPs: rs4733767 [p = 7.1 × 10−7; Cancer Susceptibility 8 and Cancer Susceptibility 11 genes (CASC8/CASC11)], rs1030757 [p = 1.1 × 10−6; Glutamate Ionotropic Receptor Delta Type Subunit 2 gene (GRID2)], and rs12504244 [p = 1.6 × 10−6; Proto-Oncogene, Receptor Tyrosine Kinase gene, (KIT)] were marked as top haplotypic blocks, while the top signals were localized within or around Ankyrin Repeat And SOCS Box Containing 13 gene (ASB13), R-Spondin 4 gene (RSPO4), Disks large-associated protein 1 gene (DLGAP1), Receptor-type tyrosine-protein phosphatase delta gene (PTPRD), GRIK2, Fas Apoptotic Inhibitory Molecule 2 gene (FAIM2), and Cadherin 20 gene (CDH20). Typical heritability variance of OCD was estimated by combined analyses of both samples resulting in a value of 25–30%. The findings of this meta-analysis confirm some of the conclusions of two prior OCD GWASs, with glutamatergic system genes, such as GRID2, DLGAP1, being involved in OCD pathogenesis.
Another study examining genetic basis of OCD was performed by den Braber et al. (47). This study included a homogeneous population from the Netherlands. Heritability of OCD, based on SNP analysis, was estimated to be 14% and one SNP, rs8100480, appeared to be significantly associated with OCD in GWAS (p = 2.56 × 10−8). Additionally, four more genes, Myocyte enhancer binding factor 2B (MEF2BNB), DNA-binding protein RFXANK gene (RFXANK), MEF2BNB-MEF2B, and MEF2B, were found to be involved in OCD etiology.
Additionally, attempts were made to demonstrate differences in the structure of the CNS in people with OCD compared to the general population. Hibar et al. (63) investigated the relationship between data obtained in GWAS of OCD by Stewart et al. (45) and data of a large-scale meta-analysis by the ENIGMA Consortium (64). Proof of substantial, positive correspondence between variants linked to the greater nucleus accumbens and the putamen volumes and OCD risk variants was identified. Additionally, the putamen, amygdala, and thalamus were brain regions which showed correlation with genetic risk of OCD.
It is worth mentioning that some scientists dealing with the subject of the genetic determinants of OCD have explored sex differences. In the study performed by Khramtsova et al. (51), two genes (GRID2 and G Protein-Coupled Receptor 135, GPR135) were found to be associated with OCD exclusively in females, but there were no genome-wide associations found in either genotype–sex interaction analysis or sex-stratified GWAS. Moreover, heritability of OCD did not differ and there were no significant distinctions in the cross-trait genetic correlations between sexes. The highest variability of effect size between males and females was reached for SNPs linked to gene regulatory function (eQTLs) in the immune system and brain.
Just recently, Burton et al. (56) examined genetic variants associated with obsessive-compulsive symptoms (OCS) and tested whether OCS and OCD shared genetic risk. The authors carried out GWAS of OCS using the Toronto Obsessive-Compulsive Scale (TOCS) in 5018 unrelated Caucasian children and adolescents. A locus tagged by rs7856850 in an intron of PTPRD (protein tyrosine phosphatase δ) was significantly associated with OCS at the genome-wide significance level (p = 2.48 × 10−8). rs7856850 was also associated with OCD in a meta-analysis of OCD case/control genome-wide datasets (p = 0.0069). Obsessive-compulsive symptoms polygenic risk score was correlated with OCD (p < 0.01). Obsessive-compulsive symptoms was highly, but not significantly, genetically correlated with OCD (p = 0.062).
Smit et al. (55) performed GWAS of obsessions, including ruminations and impulsions, and compulsions, such as checking, washing, and ordering/precision, assessed by subscales of the abbreviated edition of the Padua Inventory. While the obsession subscale and the total Padua score reached insignificant values, the compulsion subscale demonstrated a strong positive genetic association with the case-control OCD GWAS (p = 0.017) conducted prior to the analysis by the Psychiatric Genomics Consortium (PGC-OCD). Similar to the studies mentioned above, there were no significant SNPs identified in the study. In addition to the KIT and GRID2 genes, which were previously described, the study showed potential impact of two novel genes, WD repeat-containing protein 7 gene (WDR7) and AarF domain-containing protein kinase 1 gene (ADCK1). Genes expressed in the hippocampus, amygdala, and caudate nucleus were correlated with OCS. Moreover, gene-level analyses demonstrated increased correlation with brain regions involved in the reward system, emotions, memory, and fear-formation and enrichment for genes linked to psychiatric conditions.
Alemany-Navarro et al. (53) also tested whether a relationship exists between genes and specific obsessions and/or compulsions. There was no correlation between SNPs and OCD dimensions at the genome-wide level (p < 5 × 10−8). One gene, SET Domain Containing 3 gene (SETD3), reached genome-wide significant association with hoarding (p = 1.89 × 10−8), while another, Carboxypeptidase E gene (CPE), was found to be linked to aggressive symptoms (p = 4.42 × 10−6). Aggressive symptoms were also associated with zinc ion response and lipid metabolism. Among other pathways, ordering OCS were correlated with lipid metabolism, while sexual/religious OCS with G protein-mediated processes; finally, hoarding was correlated with metabolic processes and anion transport. In another study, performed by Bralten et al. (65), genetic correlations between OCD/OCS in the general population and insulin signaling in the central and peripheral nervous system were found. In this study, total OCS score and OCS factors from an exploratory factor analysis were the subject of GWAS in the population-based Philadelphia Neurodevelopmental Cohort (650 children and adolescents). The Spit for Science cohort (5,047 children and adolescents) served to validate the Bralten et al. findings. Researchers used PRS to evaluate shared genetic basis between clinical OCD, the total OCS score, and OCS factors. Gene-set analyses were then conducted with a set of OCD-linked genes focused on central nervous system (CNS) synaptic activity controlled by insulin and analyzed for five peripheral insulin-related traits based on PRS. The authors found a common genetic basis between OCD and “guilty taboo thoughts” and a correlation between CNS, insulin-linked, gene-sets and symmetry/counting/ordering in the Philadelphia Neurodevelopmental Cohort, while the association between “symmetry/counting/ordering” and “contamination/cleaning” found in the Spit for Science cohort was confirmed. Genetically-determined, peripheral, insulin-related, signaling traits such as type 2 diabetes were found to be related to aggressive taboo thinking while genetically-determined, fasting, insulin levels and 2 h glucose levels were correlated with OCD.
Researchers have also attempted to answer the question about whether links exist between OCD and other disorders. One of the most widely described associations is the link between tics and OCD. In the study conducted by Yu et al. (66), there were no genome-wide significant SNPs. PRS for OCD was found to be significant (p = 2 × 10−4), predicting 3.2% of the phenotypic variance in an independent data set, in contrast to non-significant polygenic component in GTS, predicting only 0.6% of the phenotypic variance (p = 0.06). Finally, across OCD and GTS there was no significant polygenic signal present. In the study conducted by Davis et al. (67) variance in predisposition to GTS and OCS was assessed and heritability point was evaluated to be 0.58 (se = 0.09, p = 5.64 × 10−12) and 0.37 (se = 0.07, p = 1.5 × 10−7), respectively. Moreover, 21% of the GTS heritability was connected to SNPs with a minor allele frequency of <5%, while in the case of OCD they accounted for 0% of the heritability. Genetic correlation between OCD and GTS reached the value of 0.41 (p = 0.002) in this study.
Associations between anorexia nervosa (AN) and OCD have also been analyzed. The aim of the study by Yilmaz et al. (68) was to evaluate the genetic origin of these two disorders, however, no significant genome-wide results for shared AN–OCD risk were found. Despite the absence of significant hits, prominent, reliable signals were located in the leucine-rich repeat-containing 16A gene (LRRC16A), both for AN (p = 4.19 × 10−5) and OCD (p = 1.53 × 10−3); upstream of KIT gene, both for AN (p = 1.62 × 10−6) and OCD (p = 0.011). In this study, a high genetic association between AN and OCD (rg = 0.49 ± 0.13, p = 9.07 × 10−7) and a sizable SNP heritability (SNP h2 = 0.21 ± 0.02) for the cross-disorder phenotype were reported.
Another disorder suspected to be associated with OCD at the genome level is attention deficit hyperactivity disorder (ADHD). According to the study by Ritter et al. (69), which aims to identify the potential genetic overlap between the two disorders, none of the SNPs were significant at the genome-wide level, implying the lack of evidence for genetic correlation between these two disorders.
Also, as OCD and autism spectrum disorder (ASD) are both heritable disorders of neurodevelopmental origin, Guo et al. (50) assumed that their genetic bases may share some similarities. rs4785741, located in chromosome 16, was the SNP with the top signal in this study (p = 6.9 × 10−7). In addition, enrichment analyses showed that the following genes: melanocortin 1 receptor MC1R, tubulin Beta 3 (TUBB3), dimethylarginine dimethylaminohydrolase 1 (DDAH1), inositol monophosphatase 2 (IMPA2), and parathyroid hormone 2 receptor (PTH2R) could theoretically lead to coexistence of ASD and OCD. Additionally, the application of PRS analyses identified a significant, polygenic component of ASD, predicting 0.11% of the phenotypic variance in an independent OCD data collection. With the use of Genome-wide Complex Trait Analysis, global heritability was estimated to be 0.427 (se = 0.093) in OCD and 0.174 (se = 0.053) in ASD.
Another disorder investigated in the context of its co-existence with OCD is schizophrenia. This subject was investigated by Costas et al. (54). The Dynamin 3 (DNM3) gene, involved in the endocytosis of synaptic vesicles, had a significant association at the gene-based test (p = 7.9 × 10−5) and appears to possibly be involved in OCD pathogenesis. Significant correlation was observed between disease status in OCD sample and the polygenic risk model of schizophrenia data set (PGC-SCZ2), especially when the major histocompatibility complex region was eliminated.
Some investigators tried to examine variety of psychiatric disorders that share pathophysiological background with OCD. Strom et al. (57) examined polygenic heterogeneity across OCD subgroups defined by a comorbid diagnosis. The authors hypothesized that OCD shares common genetic background with other psychiatric comorbidities. In particular, they used a framework of different approaches to study the genetic relationship of OCD with three commonly observed comorbidities, namely major depressive disorder (MDD), attention-deficit hyperactivity disorder (ADHD), and ASD. They found that PRS of such traits as neuroticism, bipolar disorder, AN, age at first birth, educational attainment, and insomnia were significantly associated with OCD across all subgroups. Cross-Disorder Group of the PGC published results of their study investigating genomic relationships, novel loci, and pleiotropic mechanisms across eight psychiatric disorders (52). They performed analyses of 232,964 cases and 494,162 controls from genome-wide studies of AN, ADHD, ASD, bipolar disorder, MDD, OCD, schizophrenia, and TS. As a result they were able to determine three groups of co-related disorders. Meta-analysis across eight disorders revealed 109 loci associated with at least two psychiatric disorders. Detected loci were mainly related to neurodevelopement.
Finally, one study investigated polygenic contributions to therapeutic responses in OCD patients. In the study by Qin et al. (48), which assessed genetic variations potentially influencing sensitivity to selective serotonin reuptake inhibitor (SSRI) treatment, rs17162912, near the Dispatched RND Transporter Family Member 1 (DISP1) gene, was the top SNP (p = 1.76 × 10−8), while rs9303380, rs12437601, rs16988159, rs723815, rs7676822, and rs1911877 were SNPs with possible association. The authors concluded that glutamatergic and serotonergic neurotransmission could be involved in treatment response in OCD. Another GWAS performed by Umehara et al. (49) on the subject of pharmacotherapy in OCD and assessed genetic variants involved in the response to combined SSRI and antipsychotic treatment. Despite the lack of a genome-wide significance level of association between one suggestive SNP and treatment outcomes, five pathways appeared enriched, with the strongest link to calcium signaling pathway.
A number of studies explored the gene-environment interaction in context of OCD. Wang et al. (70) demonstrated interaction between progranulin (PGRN) gene and the early trauma on clinical characteristics in patients with OCD. Alemany-Navarro (71) et al. explored the predictive ability of a PRS built from OCD-risk variants, for treatment response in OCD, and the modulation role of stressful life events (SLEs) at the onset of the disorder. The authors failed to demonstrate that PRS predicted treatment response. Nevertheless, PRS predicted basal and post-intervention YBOCS. Importantly, SLEs at onset were not a predictor for treatment response when included in the regression model. Real et al. (72) assessed whether genetic variants in SLC1A1 and life stress at onset of the disorder interact and modulate pharmacological resistance in OCD. For one SNP (rs3087879), one copy of the risk allele increased the probability of higher treatment resistance. Hemmings et al. (73) investigated interactions between childhood trauma and the BDNF Val66Met variant in patients with OCD. The authors observed no significant association between BDNF Val66Met and the development of OCD, but interaction analysis demonstrated that the BDNF Met-allele interacted with childhood emotional abuse and increased the risk of OCD.
In recent years, risk gene discovery has also been achieved by studying rare de novo (DN) coding variants. For OCD/OCS only two studies have been published so far. Cappi et al. (74) performed whole-exome sequencing in 222 OCD parent-child trios and estimated the contribution of de novo mutations to OCD risk and the number of genes involved. The authors identified two high-confidence risk genes, CHD8 and SCUBE1. Just recently, Halvorsen et al. (75) conducted exome sequencing aiming to identify rare damaging coding variants that could influence the occurrence of OCD. In case–control analyses, the most significant result was observed in SLITRK5 gene. All in all, it could be concluded that there is a contribution of rare variants to OCD, but more replication studies are needed.
The emergence of Big Data collaborations in OCD and innovative technologies has afforded new insights into OCD such as discovery of new genetic and pathophysiological pathways involved in this disorder. This stays in line with the genomic studies regarding other neuropsychiatric disorders, such as GTS (35), anxiety disorder (76), depression (77), ASD (78), or schizophrenia (79), which have demonstrated shared genetic background between different symptoms and comorbidities.
Nevertheless, the results of these studies are still limited by diverse populations included in the studies, especially when it comes to genetic ethnicity, diverse sample sizes, and inclusion criteria. At the moment, the majority of studies are limited to genetically white individuals, and we are still lacking studies that are more inclusive regarding other genetic groups, especially minorities. Similarly, phenotype assessment is not homogeneous between all studies. Disease-specific initiatives usually use more elaborate, physician-implemented instruments, such as YBOCS, while phenotype assessment in population-based studies is based on the more general criteria, primarily ICD classification or self-report. Furthermore, population-based studies may not accurately reflect the population-level phenotype due to certain selection bias, such the “heathy volunteer effect” mentioned by Davis et al. (24). A good example is UKB, where the prevalence of self-report OCD is 0.6%, which is well below known population prevalence estimate of OCD (1–3%). Finally, the sample size achieved in population-based studies is limited and, therefore, biobank samples may be better suited as replication samples rather than as discovery. On the other hand, biobanks contain diverse information (clinical, biomarkers, neuroimaging) usually gathered in the longitudinal fashion. Moreover, the methodology of GWASes and data analysis is also not harmonized. All these factors could contribute to the heterogeneity of results obtained in the studies presented in this article.
Considering the evidence presented in the previous sections, it can be concluded that from the point of view of genetics OCD is a highly heterogenous disorder. This is also reflected in the diverse clinical phenotypes as well as complex responses to treatment. Tools aimed toward developing personalized diagnostic and therapeutic approach in OCD are in dire need. The methodological techniques from the field of genomics are poised to unravel the complexity of personalized medicine. They will enable adjustment of diagnosis and treatment in accordance to individual genetic variability of the patient. Finally, the rapid development of bioinformatics and its application to medicine will also render new possibilities. In particular, artificial intelligence and one of its varieties, machine learning, are already used to diagnose (80), predict severity and outcome (81, 82), and trajectories of treatment response (83–85) in OCD. The advancements promised by Big Data catapulted in the field and provided new insights over the past 10 years. As bioinformatics and innovative technologies become ubiquitous in clinical practice, the present the potential (and promise) of personalized medicine. Another future avenue offers creation of international or even intercontinental databases which gather information about more diverse groups, including minorities.
NS and AD conceived and designed the study, acquired data, and wrote the original draft of the manuscript. NS, AD, JM, JP, and AL interpreted the data, reviewed and edited the manuscript, and updated the revised version. All authors contributed to the article and approved the submitted version.
JP has received research support from the National Institute of Mental Health, PCORI, the TLC Foundation for BFRBs, and Pfizer Pharmaceuticals; speaking honoria and travel expenses from the International OCD Foundation and Tourette Association of America; and book royalties from Guilford and Oxford University Press. JM reports receiving research support from the Tourette Association of American (TAA), the American Academy of Neurology (AAN), the Hilda and Preston Davis Foundation, and the American Psychological Foundation. He has served as a consultant to Bracket Global, Syneos Health, and Luminopia, and also received royalties from Elsevier.
The remaining authors declare that the research was conducted in the absence of any commercial or financial relationships that could be construed as a potential conflict of interest.
All claims expressed in this article are solely those of the authors and do not necessarily represent those of their affiliated organizations, or those of the publisher, the editors and the reviewers. Any product that may be evaluated in this article, or claim that may be made by its manufacturer, is not guaranteed or endorsed by the publisher.
1. Fullana MA, Vilagut G, Rojas-Farreras S, Mataix-Cols D, de Graaf R, Demyttenaere K, et al. Obsessive-compulsive symptom dimensions in the general population: results from an epidemiological study in six European countries. J Affect Disord. (2010) 124:291–9. doi: 10.1016/j.jad.2009.11.020
2. Lissemore JI, Sookman D, Gravel P, Berney A, Barsoum A, Diksic M, et al. Brain serotonin synthesis capacity in obsessive-compulsive disorder: effects of cognitive behavioral therapy and sertraline. Transl Psychiatry. (2018). 8:82. doi: 10.1038/s41398-018-0128-4
3. Baumgarten HG, Grozdanovic Z. Role of serotonin in obsessive-compulsive disorder. Br J Psychiatry Suppl. (1998) 35:13–20. doi: 10.1192/S0007125000297857
4. Barr LC, Goodman WK, Price LH, McDougle CJ, Charney DS. The serotonin hypothesis of obsessive compulsive disorder: implications of pharmacologic challenge studies. J Clin Psychiatry. (1992). 53(Suppl):17–28.
5. Szechtman H, Shivji S, Woody EZ. Pathophysiology of obsessive-compulsive disorder: insights from normal function and neurotoxic effects of drugs, infection, and brain injury. In: Kostrzewa RM, editor. Handbook of Neurotoxicity. New York, NY: Springer New York (2014). p. 2231–53. doi: 10.1007/978-1-4614-5836-4_118
6. Nazeer A, Latif F, Mondal A, Azeem MW, Greydanus DE. Obsessive-compulsive disorder in children and adolescents: epidemiology, diagnosis and management. Transl Pediatr. (2020) 9:S76–93. doi: 10.21037/tp.2019.10.02
7. Coetzer BR. Obsessive-compulsive disorder following brain injury: a review. Int J Psychiatry Med. (2004) 34:363–77. doi: 10.2190/XENN-NNWT-7N2K-R26A
8. Crum RM, Anthony JC. Cocaine use and other suspected risk factors for obsessive-compulsive disorder: a prospective study with data from the Epidemiologic Catchment Area surveys. Drug Alcohol Depend. (1993) 31:281–95. doi: 10.1016/0376-8716(93)90010-N
9. Swedo SE, Leonard HL, Garvey M, Mittleman B, Allen AJ, Perlmutter S, et al. Pediatric autoimmune neuropsychiatric disorders associated with streptococcal infections: clinical description of the first 50 cases. Am J Psychiatry. (1998) 155:264–71.
10. Wilbur C, Bitnun A, Kronenberg S, Laxer RM, Levy DM, Logan WJ, et al. PANDAS/PANS in childhood: controversies and evidence. Paediatr Child Health. (2019) 24:85–91. doi: 10.1093/pch/pxy145
11. Stein DJ, Costa DLC, Lochner C, Miguel EC, Reddy YCJ, Shavitt RG, et al. Obsessive-compulsive disorder. Nat Rev Dis Primers. (2019) 5:52. doi: 10.1038/s41572-019-0102-3
12. Purty A, Nestadt G, Samuels JF, Viswanath B. Genetics of obsessive-compulsive disorder. Indian J Psychiatry. (2019) 61:S37–42. doi: 10.4103/psychiatry.IndianJPsychiatry_518_18
13. Arnold PD, Askland KD, Barlassina C, Bellodi L, Bienvenu OJ, Black D, et al. Revealing the complex genetic architecture of obsessive–compulsive disorder using meta-analysis. Mol Psychiatry. (2018) 23:1181–8. doi: 10.1038/mp.2017.154
14. Pauls DL, Abramovitch A, Rauch SL, Geller DA. Obsessive-compulsive disorder: an integrative genetic and neurobiological perspective. Nat Rev Neurosci. (2014) 15:410–24. doi: 10.1038/nrn3746
15. Uitterlinden AG. An introduction to genome-wide association studies: GWAS for dummies. Semin Reprod Med. (2016) 34:196–204. doi: 10.1055/s-0036-1585406
16. Chen X, Sullivan PF. Single nucleotide polymorphism genotyping: biochemistry, protocol, cost and throughput. Pharmacogenomics J. (2003) 3:77–96. doi: 10.1038/sj.tpj.6500167
17. Davies NM, Holmes MV, Davey Smith G. Reading Mendelian randomisation studies: a guide, glossary, and checklist for clinicians. BMJ. (2018) 362:k601. doi: 10.1136/bmj.k601
18. Lambert SA, Abraham G, Inouye M. Towards clinical utility of polygenic risk scores. Hum Mol Genet. (2019) 28:R133–42. doi: 10.1093/hmg/ddz187
19. Singer JB. Candidate gene association analysis. Methods Mol Biol. (2009) 573:223–30. doi: 10.1007/978-1-60761-247-6_13
20. Bowden J, Holmes MV. Meta-analysis and Mendelian randomization: a review. Res Synth Methods. (2019) 10:486–96. doi: 10.1002/jrsm.1346
21. Ottman R. Gene-environment interaction: definitions and study designs. Prev Med. (1996) 25:764–70. doi: 10.1006/pmed.1996.0117
22. Bycroft C, Freeman C, Petkova D, Band G, Elliott LT, Sharp K, et al. The UK Biobank resource with deep phenotyping and genomic data. Nature. (2018) 562:203–9. doi: 10.1038/s41586-018-0579-z
23. Miller KL, Alfaro-Almagro F, Bangerter NK, Thomas DL, Yacoub E, Xu J, et al. Multimodal population brain imaging in the UK Biobank prospective epidemiological study. Nat Neurosci. (2016) 19:1523–36. doi: 10.1038/nn.4393
24. Davis KAS, Coleman JRI, Adams M, Allen N, Breen G, Cullen B, et al. Mental health in UK Biobank - development, implementation and results from an online questionnaire completed by 157 366 participants: a reanalysis. BJPsych Open. (2020). 6:e18. doi: 10.1192/bjo.2019.100
25. Carrasco-Ramiro F, Peiró-Pastor R, Aguado B. Human genomics projects and precision medicine. Gene Ther. (2017) 24:551–61. doi: 10.1038/gt.2017.77
26. Gaziano JM, Concato J, Brophy M, Fiore L, Pyarajan S, Breeling J, et al. Million veteran program: a mega-biobank to study genetic influences on health and disease. J Clin Epidemiol. (2016) 70:214–23. doi: 10.1016/j.jclinepi.2015.09.016
27. Levey DF, Gelernter J, Polimanti R, Zhou H, Cheng Z, Aslan M, et al. Reproducible genetic risk loci for anxiety: results from ~200,000 participants in the million veteran program. Am J Psychiatry. (2020) 177:223–32. doi: 10.1176/appi.ajp.2019.19030256
28. Chen Z, Chen J, Collins R, Guo Y, Peto R, Wu F., et al. China Kadoorie Biobank of 05 million people: survey methods, baseline characteristics and long-term follow-up. Int J Epidemiol. (2011) 40:1652–66. doi: 10.1093/ije/dyr120
29. Yu C, Lyu J, Chen Y, Guo Y, Paul S, Bian Z, et al. [Epidemiology of major depressive episodes among Chinese adults aged 30-79 years: data from the China Kadoorie Biobank]. Zhonghua Liu Xing Bing Xue Za Zhi. (2015) 36:52–6.
30. Sun J, Ma H, Yu C, Lv J, Guo Y, Bian Z., et al. Association of major depressive episodes with stroke risk in a prospective study of 05 million chinese adults. Stroke. (2016) 47:2203–8. doi: 10.1161/STROKEAHA.116.013512
31. Chen Y, Kartsonaki C, Clarke R, Guo Y, Yu C, Bian Z., et al. Characteristics and correlates of sleep duration, daytime napping, snoring and insomnia symptoms among 05 million Chinese men and women. Sleep Med. (2018) 44:67–75. doi: 10.1016/j.sleep.2017.11.1131
32. Psychiatric GWAS Consortium Steering Committee. A framework for interpreting genome-wide association studies of psychiatric disorders. Mol Psychiatry. (2009). 14:10–7. doi: 10.1038/mp.2008.126
33. Sullivan PF. The psychiatric GWAS consortium: big science comes to psychiatry. Neuron. (2010) 68:182–6. doi: 10.1016/j.neuron.2010.10.003
34. Cichon S, Craddock N, Daly M, Faraone SV, Gejman PV, Kelsoe J, et al. Genomewide association studies: history, rationale, and prospects for psychiatric disorders. Am J Psychiatry. (2009) 166:540–56. doi: 10.1176/appi.ajp.2008.08091354
35. Yu D, Sul JH, Tsetsos F, Nawaz MS, Huang AY, Zelaya I, et al. interrogating the genetic determinants of Tourette's syndrome and other tic disorders through genome-wide association studies. Am J Psychiatry. (2019) 176:217–27. doi: 10.1176/appi.ajp.2018.18070857
36. Samuels JF, Riddle MA, Greenberg BD, Fyer AJ, McCracken JT, Rauch SL, et al. The OCD collaborative genetics study: methods and sample description. Am J Med Genet B Neuropsychiatr Genet. (2006) 141B:201–7. doi: 10.1002/ajmg.b.30224
37. Kong X-Z, Boedhoe PSW, Abe Y, Alonso P, Ameis SH, Arnold PD, et al. Mapping cortical and subcortical asymmetry in obsessive-compulsive disorder: findings from the ENIGMA consortium. Biol Psychiatry. (2020) 87:1022–34. doi: 10.1016/j.biopsych.2019.04.022
38. Yun JY, Boedhoe PSW, Vriend C, Jahanshad N, Abe Y, Ameis SH, et al. Brain structural covariance networks in obsessive-compulsive disorder: a graph analysis from the ENIGMA Consortium. Brain. (2020) 143:684–700. https://doi.org/10.1093/brain/awaa001
39. Bruin WB, Taylor L, Thomas RM, Shock JP, Zhutovsky P, Abe Y, et al. Structural neuroimaging biomarkers for obsessive-compulsive disorder in the ENIGMA-OCD consortium: medication matters. Transl Psychiatry. (2020). 10:342. doi: 10.1038/s41398-020-01013-y
40. Thompson PM, Andreassen OA, Arias-Vasquez A, Bearden CE, Boedhoe PS, Brouwer RM, et al. ENIGMA and the individual: predicting factors that affect the brain in 35 countries worldwide. Neuroimage. (2017) 145:389–408. doi: 10.1016/j.neuroimage.2015.11.057
41. Boedhoe PS, Schmaal L, Abe Y, Ameis SH, Arnold PD, Batistuzzo MC, et al. Distinct subcortical volume alterations in pediatric and adult OCD: a worldwide meta- and mega-analysis. Am J Psychiatry. (2017) 174:60–9. doi: 10.1176/appi.ajp.2016.16020201
42. Boedhoe PSW, Schmaal L, Abe Y, Alonso P, Ameis SH, Anticevic A, et al. Cortical abnormalities associated with pediatric and adult obsessive-compulsive disorder: findings from the ENIGMA obsessive-compulsive disorder working group. Am J Psychiatry. (2018) 175:453–62. doi: 10.1176/appi.ajp.2017.17050485
43. Thompson PM, Stein JL, Medland SE, Hibar DP, Vasquez AA, Renteria ME, et al. The ENIGMA Consortium: large-scale collaborative analyses of neuroimaging and genetic data. Brain Imaging Behav. (2014) 8:153–82. doi: 10.1007/s11682-013-9269-5
44. Novak NM, Stein JL, Medland SE, Hibar DP, Thompson PM, Toga AW. EnigmaVis: online interactive visualization of genome-wide association studies of the Enhancing NeuroImaging Genetics through Meta-Analysis (ENIGMA) consortium. Twin Res Hum Genet. (2012) 15:414–8. doi: 10.1017/thg.2012.17
45. Stewart SE, Yu D, Scharf JM, Neale BM, Fagerness JA, Mathews CA, et al. Genome-wide association study of obsessive-compulsive disorder. Mol Psychiatry. (2013) 18:788–98. doi: 10.1038/mp.2012.85
46. Mattheisen M, Samuels JF, Wang Y, Greenberg BD, Fyer AJ, McCracken JT, et al. Genome-wide association study in obsessive-compulsive disorder: results from the OCGAS. Mol Psychiatry. (2015) 20:337–44. doi: 10.1038/mp.2014.43
47. den Braber A, Zilhão NR, Fedko IO, Hottenga JJ, Pool R, Smit DJA, et al. Obsessive–compulsive symptoms in a large population-based twin-family sample are predicted by clinically based polygenic scores and by genome-wide SNPs. Transl Psychiatry. (2016). 6:e731. doi: 10.1038/tp.2015.223
48. Qin H, Samuels JF, Wang Y, Zhu Y, Grados MA, Riddle MA, et al. Whole-genome association analysis of treatment response in obsessive-compulsive disorder. Mol Psychiatry. (2016) 21:270–6. doi: 10.1038/mp.2015.32
49. Umehara H, Numata S, Tajima A, Nishi A, Nakataki M, Imoto I, et al. Calcium signaling pathway is associated with the long-term clinical response to selective serotonin reuptake inhibitors (SSRI) and SSRI with Antipsychotics in patients with obsessive-compulsive disorder. PLoS ONE. (2016). 11:e0157232. doi: 10.1371/journal.pone.0157232
50. Guo W, Samuels JF, Wang Y, Cao H, Ritter M, Nestadt PS, et al. Polygenic risk score and heritability estimates reveals a genetic relationship between ASD and OCD. Eur Neuropsychopharmacol. (2017) 27:657–66. doi: 10.1016/j.euroneuro.2017.03.011
51. Khramtsova EA, Heldman R, Derks EM, Yu D, Tourette Syndrome/Obsessive-Compulsive Disorder Working Group of the Psychiatric Genomics Consortium, Davis LK. Sex differences in the genetic architecture of obsessive-compulsive disorder. Am J Med Genet B Neuropsychiatr Genet. (2019) 180:351–64. doi: 10.1002/ajmg.b.32687
52. Cross-Disorder Group of the Psychiatric Genomics Consortium. Electronic address: cGxlZTBAbWdoLmhhcnZhcmQuZWR1; Cross-Disorder Group of the Psychiatric Genomics Consortium. Genomic relationships, novel loci, and pleiotropic mechanisms across eight psychiatric disorders. Cell. (2019) 179:1469.e11–82.e11. doi: 10.1016/j.cell.2019.11.020
53. Alemany-Navarro M, Cruz R, Real E, Segalàs C, Bertolín S, Rabionet R, et al. Looking into the genetic bases of OCD dimensions: a pilot genome-wide association study. Transl Psychiatry. (2020) 10:151. doi: 10.1038/s41398-020-0804-z
54. Costas J, Carrera N, Alonso P, Gurriarán X, Segalàs C, Real E, et al. Exon-focused genome-wide association study of obsessive-compulsive disorder and shared polygenic risk with schizophrenia. Transl Psychiatry. (2016). 6:e768. doi: 10.1038/tp.2016.34
55. Smit DJA, Cath D, Zilhão NR, Ip HF, Denys D, den Braber A, et al. Genetic meta-analysis of obsessive-compulsive disorder and self-report compulsive symptoms. Am J Med Genet B Neuropsychiatr Genet. (2020) 183:208–16. doi: 10.1002/ajmg.b.32777
56. Burton CL, Lemire M, Xiao B, Corfield EC, Erdman L, Bralten J, et al. Genome-wide association study of pediatric obsessive-compulsive traits: shared genetic risk between traits and disorder. Transl Psychiatry. (2021) 11:91. http://dx.doi.org/10.1101/858241
57. Strom NI, Grove J, Meier SM, Bækvad-Hansen M, Nissen JB, Als TD, et al. Polygenic heterogeneity across obsessive-compulsive disorder subgroups defined by a comorbid diagnosis. medRxiv. 2021:2021.05.21.21257530. doi: 10.1101/2021.05.21.21257530
58. Hayes B. Overview of statistical methods for genome-wide association studies (GWAS). Methods Mol Biol. (2013) 1019:149–69. doi: 10.1007/978-1-62703-447-0_6
59. Marchant G, Barnes M, Evans JP, LeRoy B, Wolf SM, LawSeq Liability Task Force. from genetics to genomics: facing the liability implications in clinical Care. J Law Med Ethics. (2020) 48:11–43. doi: 10.1177/1073110520916994
60. Yengo L, Sidorenko J, Kemper KE, Zheng Z, Wood AR, Weedon MN, et al. Meta-analysis of genome-wide association studies for height and body mass index in ~700000 individuals of European ancestry. Hum Mol Genet. (2018) 27:3641–9. doi: 10.1093/hmg/ddy271
61. Xue A, Wu Y, Zhu Z, Zhang F, Kemper KE, Zheng Z, et al. Genome-wide association analyses identify 143 risk variants and putative regulatory mechanisms for type 2 diabetes. Nat Commun. (2018) 9:2941. doi: 10.1038/s41467-018-04951-w
62. Dennison CA, Legge SE, Pardiñas AF, Walters JTR. Genome-wide association studies in schizophrenia: recent advances, challenges and future perspective. Schizophr Res. (2020) 217:4–12. doi: 10.1016/j.schres.2019.10.048
63. Hibar DP, Cheung JW, Medland SE, Mufford MS, Jahanshad N, Dalvie S, et al. Significant concordance of genetic variation that increases both the risk for obsessive–compulsive disorder and the volumes of the nucleus accumbens and putamen. Br J Psychiatry. (2018) 213:430–6. doi: 10.1192/bjp.2018.62
64. Hibar DP, Stein JL, Renteria ME, Arias-Vasquez A, Desrivières S, Jahanshad N, et al. Common genetic variants influence human subcortical brain structures. Nature. (2015) 520:224–9. doi: 10.1038/nature14101
65. Bralten J, Widomska J, Witte WD, Yu D, Mathews CA, Scharf JM, et al. Shared genetic etiology between obsessive-compulsive disorder, obsessive-compulsive symptoms in the population, and insulin signaling. Transl Psychiatry. (2020). 10:121. doi: 10.1038/s41398-020-0793-y
66. Yu D, Mathews CA, Scharf JM, Neale BM, Davis LK, Gamazon ER, et al. Cross-disorder genome-wide analyses suggest a complex genetic relationship between Tourette's syndrome and OCD. Am J Psychiatry. (2015) 172:82–93. doi: 10.1176/appi.ajp.2014.13101306
67. Davis LK, Yu D, Keenan CL, Gamazon ER, Konkashbaev AI, Derks EM, et al. Partitioning the heritability of Tourette syndrome and obsessive compulsive disorder reveals differences in genetic architecture. PLoS Genet. (2013) 9:e1003864. doi: 10.1371/journal.pgen.1003864
68. Yilmaz Z, Halvorsen M, Bryois J, Yu D, Thornton LM, Zerwas S, et al. Examination of the shared genetic basis of anorexia nervosa and obsessive-compulsive disorder. Mol Psychiatry. (2020) 25:2036–46. doi: 10.1038/s41380-018-0115-4
69. Ritter ML, Guo W, Samuels JF, Wang Y, Nestadt PS, Krasnow J, et al. Genome Wide Association Study (GWAS) between Attention Deficit Hyperactivity Disorder (ADHD) and Obsessive Compulsive Disorder (OCD). Front Mol Neurosci. (2017). 10:83. doi: 10.3389/fnmol.2017.00083
70. Wang P, Zhao Q, Xu T, Gu Q, Liu Q, Wang Y, et al. Interaction between PGRN gene and the early trauma on clinical characteristics in patients with obsessive-compulsive disorder. J Affect Disord. (2020) 263:134–40. doi: 10.1016/j.jad.2019.11.111
71. Alemany-Navarro M, Costas J, Real E, Segalàs C, Bertolín S, Domènech L, et al. Do polygenic risk and stressful life events predict pharmacological treatment response in obsessive compulsive disorder? A gene-environment interaction approach. Transl Psychiatry. (2019) 9:70. doi: 10.1038/s41398-019-0410-0
72. Real E, Gratacòs M, Labad J, Alonso P, Escaramís G, Segalàs C, et al. Interaction of SLC1A1 gene variants and life stress on pharmacological resistance in obsessive-compulsive disorder. Pharmacogenomics J. (2013) 13:470–5. doi: 10.1038/tpj.2012.30
73. Hemmings SM, Lochner C, van der Merwe L, Cath DC, Seedat S, Stein DJ, et al. Val66Met modifies the risk of childhood trauma on obsessive-compulsive disorder. J Psychiatr Res. (2013) 47:1857–63. doi: 10.1016/j.jpsychires.2013.08.012
74. Cappi C, Oliphant ME, Péter Z, Zai G., Conceição do Rosário M, Sullivan CAW, et al. De novo damaging DNA coding mutations are associated with obsessive-compulsive disorder and overlap with Tourette's disorder and autism. Biol Psychiatry. (2020) 87:1035–44. doi: 10.1016/j.biopsych.2019.09.029
75. Halvorsen M, Samuels J, Wang Y, Greenberg BD, Fyer AJ, McCracken JT, et al. Exome sequencing in obsessive–compulsive disorder reveals a burden of rare damaging coding variants. Nat Neurosci. (2021) 24:1071–6. doi: 10.1038/s41593-021-00876-8
76. Lopes FL, Zhu K, Purves KL, Song C, Ahn K, Hou L, et al. Polygenic risk for anxiety influences anxiety comorbidity and suicidal behavior in bipolar disorder. Transl Psychiatry. (2020) 10:298. doi: 10.2139/ssrn.3578800
77. Fang Y, Scott L, Song P, Burmeister M, Sen S. Genomic prediction of depression risk and resilience under stress. Nat Hum Behav. (2020) 4:111–8. doi: 10.1038/s41562-019-0759-3
78. González-Peñas J, Costas JC, García-Alcón A, Penzol MJ, Rodríguez J, Rodríguez-Fontenla C, et al. Psychiatric comorbidities in Asperger syndrome are related with polygenic overlap and differ from other Autism subtypes. Transl Psychiatry. (2020) 10:258. doi: 10.1038/s41398-020-00939-7
79. Zheutlin AB, Dennis J, Karlsson Linnér R, Moscati A, Restrepo N, Straub P, et al. Penetrance and pleiotropy of polygenic risk scores for schizophrenia in 106,160 patients across four health care systems. Am J Psychiatry. (2019) 176:846–55. doi: 10.1176/appi.ajp.2019.18091085
80. Chandran D, Robbins DA, Chang CK, Shetty H, Sanyal J, Downs J, et al. Use of natural language processing to identify obsessive compulsive symptoms in patients with schizophrenia, schizoaffective disorder or bipolar disorder. Sci Rep. (2019) 9:14146. doi: 10.1038/s41598-019-49165-2
81. Hoexter MQ, Miguel EC, Diniz JB, Shavitt RG, Busatto GF, Sato JR. Predicting obsessive-compulsive disorder severity combining neuroimaging and machine learning methods. J Affect Disord. (2013) 150:1213–6. doi: 10.1016/j.jad.2013.05.041
82. Askland KD, Garnaat S, Sibrava NJ, Boisseau CL, Strong D, Mancebo M, et al. Prediction of remission in obsessive compulsive disorder using a novel machine learning strategy. Int J Methods Psychiatr Res. (2015) 24:156–69. doi: 10.1002/mpr.1463
83. Salomoni G, Grassi M, Mosini P, Riva P, Cavedini P, Bellodi L. Artificial neural network model for the prediction of obsessive-compulsive disorder treatment response. J Clin Psychopharmacol. (2009) 29:343–9. doi: 10.1097/JCP.0b013e3181aba68f
84. Yun JY, Jang JH, Kim SN, Jung WH, Kwon JS. Neural correlates of response to pharmacotherapy in obsessive-compulsive disorder: individualized cortical morphology-based structural covariance. Prog Neuropsychopharmacol Biol Psychiatry. (2015) 63:126–33. doi: 10.1016/j.pnpbp.2015.06.009
Keywords: genomics, genetics, obsessive-compulsive disorder, Big Data, genome-wide association study
Citation: Szejko N, Dunalska A, Lombroso A, McGuire JF and Piacentini J (2021) Genomics of Obsessive-Compulsive Disorder—Toward Personalized Medicine in the Era of Big Data. Front. Pediatr. 9:685660. doi: 10.3389/fped.2021.685660
Received: 25 March 2021; Accepted: 20 September 2021;
Published: 20 October 2021.
Edited by:
Janardhan Y. C. Reddy, National Institute of Mental Health and Neurosciences (NIMHANS), IndiaReviewed by:
Biju Viswanath, National Institute of Mental Health and Neurosciences (NIMHANS), IndiaCopyright © 2021 Szejko, Dunalska, Lombroso, McGuire and Piacentini. This is an open-access article distributed under the terms of the Creative Commons Attribution License (CC BY). The use, distribution or reproduction in other forums is permitted, provided the original author(s) and the copyright owner(s) are credited and that the original publication in this journal is cited, in accordance with accepted academic practice. No use, distribution or reproduction is permitted which does not comply with these terms.
*Correspondence: Anna Dunalska, YWR1bmFsc2thQGdtYWlsLmNvbQ==
†These authors have contributed equally to this work and share first authorship
Disclaimer: All claims expressed in this article are solely those of the authors and do not necessarily represent those of their affiliated organizations, or those of the publisher, the editors and the reviewers. Any product that may be evaluated in this article or claim that may be made by its manufacturer is not guaranteed or endorsed by the publisher.
Research integrity at Frontiers
Learn more about the work of our research integrity team to safeguard the quality of each article we publish.