- 1Neonatal Research Unit, Centre for the Studies of Asphyxia and Resuscitation, Royal Alexandra Hospital, Edmonton, AB, Canada
- 2Department of Pediatrics, Faculty of Medicine and Dentistry, University of Alberta, Edmonton, AB, Canada
- 3Department of Educational Psychology, Faculty of Education, University of Alberta, Edmonton, AB, Canada
- 4Department of Computing Science, Faculty of Science, University of Alberta, Edmonton, AB, Canada
Purpose: To safely care for their newborn patients, health-care professionals (HCP) must undergo frequent training to improve and maintain neonatal resuscitation knowledge and skills. However, the current approach to neonatal resuscitation simulation training is time and resource-intensive, and often inaccessible. Digital neonatal resuscitation simulation may present a convenient alternative for more frequent training.
Method: Fifty neonatal HCPs participated in the study (44 female; 27 nurses, 3 nurse practitioners, 14 respiratory therapists, 6 doctors). This study was conducted at a tertiary perinatal center in Edmonton, Canada from April–August 2019, with 2-month (June–October 2019) and 5-month (September 2019–January 2020) follow-up. Neonatal HCPs were recruited by volunteer sampling to complete a demographic survey, pre-test (baseline knowledge), two digital simulation scenarios (intervention), and post-test (knowledge acquisition). Two months later, participants repeated the post-test (knowledge retention). Five months after the initial intervention, participants completed a post-test using a table-top simulation (knowledge transfer). Longitudinal analyses were used to compare participants' performance over time.
Results: Overall the proportion of correct performance increased: 21/50 (42%) passed the pre-test, 39/50 (78%) the post-test, 30/43 (70%) the 2-month post-test, and 32/40 (80%) the 5-month post-test. GLMM and GEE analyses revealed that performance on all post-tests was significantly better than the performance on the pre-test. Therefore, training with the RETAIN digital simulation effectively improves, maintains, and transfers HCPs' neonatal resuscitation knowledge.
Conclusions: Digital simulation improved, maintained, and helped transfer HCPs' neonatal resuscitation knowledge over time. Digital simulation presents a promising approach for frequent neonatal resuscitation training, particularly for distance-learning applications.
Introduction
Each year, ~10% of infants worldwide need help to breathe at birth. To safely care for these newborn patients, health-care professionals (HCPs) must master their knowledge and decision-making skills outlined by the neonatal resuscitation algorithm. However, one million infants still die each year from asphyxia at birth (1). Alarmingly, half of these deaths are caused by deficiencies in HCPs' competence to safely provide care (2). To improve these deficiencies, guidelines recommended simulation-based education to target this root cause of mortality and prepare HCPs to safely provide neonatal resuscitative care (2, 3).
However, despite widespread uptake of the simulation-based Neonatal Resuscitation Program (NRP), HCPs remain dangerously underprepared for clinical neonatal resuscitation events due to decay in clinical skills after training (3). One reason for this persistent problem is that current simulation-based education is resource demanding, requiring lab space, specialized equipment, and trained instructors (4). Therefore, frequent simulation-based education opportunities are overall inaccessible by most HCPs (5).
Alternative approaches to traditional in-person simulation, such as digital simulation, may provide a solution. Digital simulation offers a scalable online platform for learners to readily access educational content and achieve many of the learning objectives of traditional simulation-based education, particularly for training HCPs' knowledge, including the recall of the correct sequence of steps for a clinical procedure (6). Digital simulation has been used successfully for a wide range of health-care education needs, such as surgical skills training (7) and disaster preparedness (8). Therefore, digital simulation may also offer a convenient approach to improve HCPs' competence to provide neonatal resuscitation care by improving knowledge and correct recall of the neonatal resuscitation algorithm (6).
This study aimed to examine if training with a digital simulation improves HCPs' neonatal resuscitation knowledge over time. This study also aimed to measure HCPs' knowledge transfer from the digital simulation. We hypothesized that HCPs would experience short- and long-term knowledge improvement of the correct steps of neonatal resuscitation after training with the digital simulation and transfer this knowledge into a novel learning environment.
Methods
Participant Recruitment
Neonatal HCPs were recruited by voluntary sample from the Royal Alexandra Hospital Neonatal Intensive Care Unit (NICU), Edmonton, Canada, a tertiary perinatal center delivering over 6,500 infants annually. Recruited participants included registered nurses, respiratory therapists, doctors, and neonatal nurse practitioners. The demographic characteristics (e.g., clinical position, gender, etc.) of the study sample was representative of the HCP population within the NICU. The study was performed at the bedside in the NICU and was approved by the Human Research Ethics Board at the University of Alberta, Edmonton, Canada (Pro00081221). Written informed consent was obtained from HCPs prior to participation.
RETAIN Digital Simulator
RETAIN (REsuscitation TrAINing; RETAIN Labs Medical Inc. Edmonton, Canada; https://www.retainlabsmedical.com/index.html) is a digital simulator for HCPs to practice their knowledge and decision-making of neonatal resuscitation (9–12). In RETAIN, users are presented with scenarios of varying difficulty and medical conditions. After reviewing the case history and gathering a virtual team of HCPs, users make assessments and perform actions in response to continuous visual and cardiorespiratory feedback from the infant. Appropriate actions improve the infant's health, whereas inappropriate actions cause the infant's health to worsen. To improve realism, information from the delivery room was incorporated, including the average time taken to complete each available action (e.g., the time needed to attach a pulse oximeter), and all scenarios are based on information recorded during real deliveries from the Royal Alexandra Hospital (Edmonton, Canada) (11).
Participants and Procedure
Participants were n = 50 neonatal HCPs (44 females, 6 males; 27 registered nurses, 3 nurse practitioners, 14 respiratory therapists, and 6 doctors) who completed NRP-recertification within the last 24-months. The study consisted of four different assessments administered over three different timepoints: an initial session (including a pre-test and a post-test), a 2-month follow-up session, and a 5-month follow-up session (Figure 1).
Initial Session
All participants completed a demographic survey, including their clinical position, years of neonatal experience, and time elapsed since last NRP certification course. Following the demographic survey, participants were allowed to familiarize themselves with the digital simulator interface and completed a guided tutorial of a neonatal resuscitation scenario.
Once familiarized, participants completed the pre-test to measure participants' baseline knowledge. The pre-test consisted of a neonatal resuscitation simulation scenario requiring correct escalation through the NRP algorithm up to chest compressions to successfully stabilize the infant (the complete assessment is available in Appendix 1). There was no formal feedback provided to participants about their performance during or after the pre-test.
After the pre-test, participants completed two digital simulation training scenarios. The training scenarios were followed by a post-test to measure changes in participants' knowledge after training with the RETAIN digital simulator. The post-test scenario required correct escalation through the NRP algorithm up to chest compressions (the complete assessment is available in Appendix 1).
Two-Month Follow-Up
Two-months after the initial session, participants' long-term knowledge retention was assessed using a 2-month follow-up post-test. The 2-month follow-up scenario required correct escalation through the NRP algorithm up to chest compressions (the complete assessment is available in Appendix 1).
Five-Month Follow-Up
Five-months after the initial session, participants' knowledge transfer to a new learning environment was examined with a 5-month follow-up post-test using a low-fidelity table-top simulation (Figure 1). In the table-top simulator, players use action cards (e.g., initiate positive pressure ventilation), equipment pieces (e.g., T-piece mask), and adjustable monitors (e.g., increase peak inspiratory pressure), to stabilize a low-fidelity simulated newborn infant presented on a 2D board. More information about the table-top simulator is presented in the literature (6, 9, 11). The action cards are identical to the action buttons presented in the digital simulator. Throughout the scenario, players receive standardized feedback (i.e., heart rate, oxygen saturation, etc.) from a facilitator (trained researcher) in response to their actions. The 5-month follow-up scenario required correct escalation through the NRP algorithm up to administering one dose of epinephrine via the umbilical venous catheter (the complete assessment is available in Appendix 1).
Measures
All scenarios were scored based on the 7th edition NRP guidelines (3). Participants' time-stamped actions (i.e., mouse input and keystrokes) during each scenario were recorded within the digital simulator. During the table-top simulation scenario, participants' actions were recorded by a trained researcher (SKG) using a sequential checklist (Appendix 1).
Participants completed all scenarios individually without assistance. Feedback was only provided through visual and cardiorespiratory assessment of the infant throughout the scenario (e.g., heart rate via electrocardiogram monitor, oxygen saturation via pulse oximeter). Depending on the steps taken by the participant, the infant's health improved, worsened, or remained unchanged (i.e., heart rate, oxygen saturation, etc.). If all steps of the NRP algorithm were performed correctly, the infant's health would improve, the infant would stabilize, and the scenario would end. If participants completed any step of the NRP algorithm incorrectly, the infant would fail to stabilize, and participants would exit the scenario.
The pre-test, post-test, 2-month follow-up, and 5-month follow-up measures represented the cumulative score across all actions, interventions, or tasks initiated by participants during the assessment (Appendix 1). For each measure, participants were assigned a score of either pass or fail. A passing score represented 100% correct adherence to the NRP guidelines (3). A failing score represented <100% correct adherence to the NRP guidelines (3). Because deviation from the algorithm resulted in deterioration of the simulated infant's health, and this deterioration may have prompted participants to deviate further from the sequence expected by the digital simulator, a binary pass or fail outcome was used. The pre-test, post-test, 2-month post-test, and 5-month post-test shared common actions across all four scenarios (NRP algorithm up to 1 min of chest compressions). However, the 5-month post-test to measure knowledge transfer included two additional actions (obtaining vascular access and administering one dose of epinephrine).
Participants had no access to the RETAIN simulator in between data collection timepoints (digital simulator and table-top simulator were kept locked in the NICU research office), nor had any of the participants previously used the digital nor tabletop simulator described in the present study. The only training scenarios for participants to practice their neonatal resuscitation knowledge occurred during the initial session (two digital simulation scenarios administered). In contrast, the pre-test, post-test, 2-month post-test, and 5-month post-test were assessment scenarios. The RETAIN table-top simulation was previously reported as a method for summative assessment of neonatal resuscitation providers (9).
Analyses Plan
Statistical analyses were performed using RStudio AGPL v3 Desktop Open Source Edition (RStudio Inc., Boston, MA) with R version 4.0.1 (13). We conducted descriptive analyses to ascertain whether the performance outcome changed over time. Correlations between performance scores at different timepoints were explored to ascertain whether a possible dependency among the multiple measurements across the longitudinal data changed over time. Following this, we conducted repeated-measure analyses with binary outcomes (i.e., solving the test scenario correctly or incorrectly): generalized linear mixed models (GLMMs) and generalized estimating equations (GEEs). Data are presented as median [interquartile range (IQR)] for non-normal or mean [standard deviation (SD)] for normally distributed continuous variables.
Results
The number of months since participants completed their last NRP course was median (IQR) 9 (5–12) months, and number of years of clinical neonatal experience was median (IQR) 9 (6.4–15.2) years. All participants completed the initial session, consisting of the pre-test (baseline assessment), training scenarios, and post-test (immediate knowledge improvement). Forty-three participants (n = 3 job relocation, n = 2 maternity leave, n = 3 unavailable) completed the 2-month follow-up session, consisting of the 2-month post-test (long-term knowledge retention). Forty participants (n = 1 maternity leave, n = 2 unavailable) completed the 5-month follow-up session, consisting of the 5-month post-test (knowledge transfer).
Descriptive Statistics
We reported an overall increase in the proportion of correct performance across tests: 21 out of 50 participants passed the pre-test (42%), 39 of 50 participants passed the post-test (78%), 30 of 43 participants passed the 2-month post-test (70%), and 32 of 40 passed the 5-month post-test (80%). The distributions of the performance at each timepoint were explored using violin plots from the ggplot2 package (available in Appendix 2).
Although performance slightly declined at the 2-month testing point (as expected with the passage of time), mean performance after 5-months was double the mean performance on the pre-test, suggesting that learning was successfully sustained and transferred. Note that the 5-month post-test was a more difficult scenario than that of the previous tests.
Tests of Association
Pearson correlation analyses revealed that the pre-test was significantly associated with the 5-month post-test (r = 0.43, p < 0.05). Also, the association between the pre-test and the immediate post-test did not reach significance (r = 0.26, p > 0.05). Results were identical for the Spearman correlation as well.
Repeated-Measure Analysis
Generalized Linear Mixed-Effects Models (GLMM)
GLMM models with dichotomous longitudinal outcomes were fit using the glmer function of the lme4 package in R (14) by maximum likelihood (Laplace Approximation), with the family argument set to binomial, and the logit link function. The results of the model fit are summarized in Table 1. Positive fixed effects for time suggest that performance on all post-tests is significantly better than the performance on the pre-tests (see the βtime1, βtime2, and βtime3 estimates).
The ggplot function from the ggplot2 R package was used to plot the results of the prediction expressed in probabilities shown in Figures 2A,B, which displays the individual participant-predicted trajectories based on the final GLMM model. The results observe that participants vary in their starting performance on the pre-test, captured by the differences in starting points displayed on the left side of Figures 2A,B, and that some participants did not complete one or two of the last two post-tests. The variability in the intercepts modeled with the random-intercept term indicates that some groups of participants had high model implied probabilities of performing correctly throughout all testing stages, whereas others had lower probabilities throughout all of the four testing points.
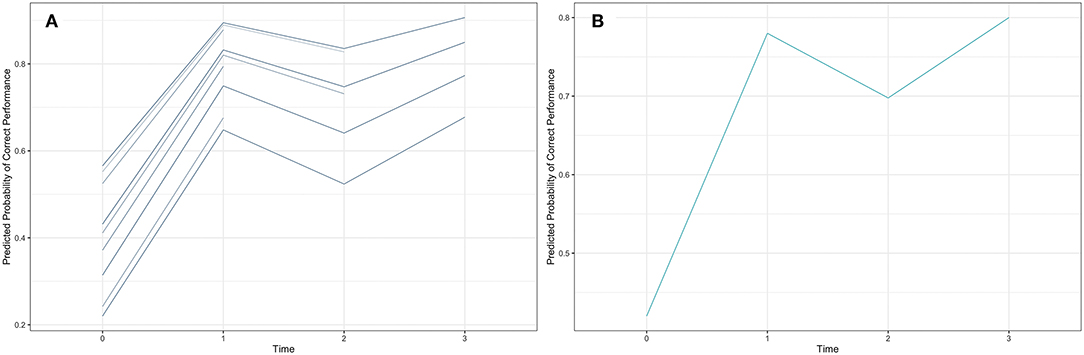
Figure 2. The visualization of the predicted probabilities of correct performance at each testing time point (0: pre-test, 1: post-test, 2: 2-month post-test, and 3: 5-month post-test), for the GLMM random-intercept with time model (A) and the GEE model (B).
Generalized Estimating Equations (GEE)
The geeglm function of the geepack package in R, with binomial distribution and logit link function, was used to fit the GEE models that estimate the population aggregate (average) trend directly to make inferences about the population rather than about an individual. The QIC function of the MuMIn package in R was used to compute the quasi-information criterion (QIC) statistic that guides model selection (where a smaller value indicates a better fit). The GEE model selection is detailed in Appendix 2. As in the case of the GLMM model, the results of the GEE model fit show an increasing average trend in performance over time, as illustrated in Table 2 and Figure 2.
Discussion
Lifetime risk of mortality is the highest on the day of birth (15). Birth asphyxia is the third biggest cause of neonatal mortality, claiming the lives of one million infants each year (16). Effective resuscitation helps reduce neonatal mortality (17); therefore, effective resuscitation training for HCPs will help more infants survive their first day after birth. While there is a clearly defined algorithm describing the steps of neonatal resuscitation, two-thirds of infant mortality during neonatal resuscitation is caused by deficiencies in HCPs' competence to safely provide care (2, 3). Neonatal resuscitation is a stressful, high-acuity medical intervention; therefore, deviations from the algorithm are dangerously common (18, 19).
Simulation-based education is recommended to address these deficiencies by replicating a real-world clinical situation, while simultaneously maintaining patient safety (20). Simulation-based education improves HCPs' neonatal resuscitation competence (21); however, frequent training sessions are needed to retain competence (22). Unfortunately, the current approach to simulation-based education is limited by intense time (e.g., scheduling sessions, developing scenarios) and resource (e.g., simulation lab, manikin, trained instructor) requirements. Therefore, frequent training sessions are often overall inaccessible.
In contrast, digital simulation presents a promising alternative to overcome traditional time and resource limitations of neonatal resuscitation simulation. Digital simulation may offer a convenient solution for neonatal resuscitation providers to access more frequent training to foster sustained learning (23). Several digital simulators have been developed for neonatal resuscitation, including RETAIN (6, 11). The RETAIN digital simulator presents HCPs with iterative resuscitation scenarios based on real deliveries recorded at a tertiary perinatal center. We sought to understand if training with the RETAIN digital simulator improved HCPs' knowledge of the correct steps of neonatal resuscitation, and if the improvement was sustained long-term.
Our participants were experienced neonatal HCPs recruited from a tertiary perinatal care center (median of 9 years of clinical experience). When compared to their baseline scores, we observed that HCPs significantly improved their performance after training with the RETAIN digital simulator, although there was some variation in the pre-test, likely due to the variety and size of the sample. Therefore, a single session with the RETAIN digital simulator immediately improved performance demonstrated by highly experienced neonatal resuscitation providers. Furthermore, HCPs' improvements were sustained 2-months after training. The proportion of correct performance tended to increase over time, with only small variations. The median of the pre-test was smaller than the mean, suggesting that there are a few participants who performed very well on the pre-test and pulled the mean of the group above the median. Performance on the pre-test tended to be slightly poor, but the trend was reversed for all three post-tests, more so for the immediate post-test and for the 5-month post-test. All post-tests had similar variation, higher than that of the pre-test. Therefore, training with the RETAIN digital simulation resulted in HCPs' long-term knowledge retention.
We expected the correlation between performance scores at different timepoints to decline. That was the case between the pre-test and the 2-month post-test. However, the strongest correlation found was between the first (pre-test) and the last (5-month post-test) timepoints. This result may be explained by the different nature of the 5-month post-test; however, this result is promising, considering the 5-month post-test administered a more complex scenario compared to previous tests.
To measure knowledge transfer, we used a table-top simulator previously reported as a valid summative assessment tool to evaluate neonatal resuscitation performance (9). Knowledge transfer from one situation to another constitutes the essence of analogical thinking but rarely happens spontaneously because it hinges on the learner's deep understanding of a concept before being able to recognize its general form across widely different instances (24). However, knowledge transfer is important for developing good problem-solving and decision-making skills because it helps learners to apply their knowledge to unfamiliar learning contexts, when they need to make difficult decisions on their own (25). The results from this study indicate that RETAIN effectively improves, maintains, and transfers HCPs' neonatal resuscitation knowledge.
The RETAIN digital simulator provides neonatal resuscitation scenarios of varying difficulty and patient risk factors, accessible by HCPs anywhere and anytime (6, 11). Digital simulation could be used to improve access to neonatal resuscitation training for HCPs from low-resource or rural health-care sites, as well as allow for more frequent and convenient training for HCPs at high-resource urban sites. Interestingly, evidence-based digital simulation may also help solve the emerging urgent need for remote health-care education strategies. In response to the recent coronavirus disease 2019 (COVID-19) pandemic, simulation-based education has faced significant interruptions that will likely continue for the foreseeable future. Many simulation centers have closed in an effort to maintain social distancing, preserve personal protective equipment, redistribute resources, and transition to clinical space. Health-care education, training, certification, and licensure has either been postponed indefinitely or transitioned to remote delivery. Many institutions have relied on remote training methods like online lectures or textbooks, which often constitute passive learning activities and may lack clinical relevance.
In contrast, digital simulation offers a feasible approach toward active remote learning, which may be readily taken up by neonatal resuscitation providers during COVID-19. Widespread implementation of digital simulation can provide a practical tool for health-care institutions to transition to effective and clinically relevant remote learning strategies. In consideration of current public health challenges, continuation of health-care education is essential to improve HCP performance so they may continue to provide safe and effective health care for their patients during these unprecedented times.
Limitations
The RETAIN digital simulator was not designed with the goal of improving psychomotor practical skills. Therefore, the current version of the RETAIN digital simulator should be used to reinforce rather than replace traditional simulation-based education. It is not clear whether improved performance on a digital simulator indicates competence in providing neonatal resuscitation care. Other limitations of the study include convenience sampling, lack of random assignment and control group. However, our attrition rate was reasonable, considering participants were HCPs on a busy intensive-care unit, which made retesting difficult. Further, there was no difference between pre-test scores of HCPs who dropped out compared to those who remained. Additional studies are needed to compare digital simulation to other remote learning methods and to measure changes in HCPs' performance in the delivery room after training with digital simulation.
Conclusions
After using the RETAIN digital simulator, HCPs demonstrated improved adherence to the neonatal resuscitation algorithm, which was sustained 2- and 5-months after the initial training. HCPs also successfully demonstrated knowledge transfer to a new learning environment. The RETAIN digital simulator may be useful to supplement formal neonatal resuscitation education, with potential application for remote learning during the COVID-19 pandemic.
Data Availability Statement
The original contributions presented in the study are included in the article/Supplementary Material, further inquiries can be directed to the corresponding author/s.
Ethics Statement
The study was performed at the bedside in the NICU and was approved by the Human Research Ethics Board at the University of Alberta, Edmonton, Canada (Pro00081221). Written informed consent was obtained from HCPs prior to participation. The patients/participants provided their written informed consent to participate in this study.
Author Contributions
GS attests that all listed authors meet authorship criteria and that no others meeting the criteria have been omitted. SG, MC, and GS conceptualized and designed the study, designed the data collection instruments, collected data, carried out the analyses, and interpretation, drafted the initial manuscript, and critically reviewed and revised the manuscript. All authors approved the final manuscript as submitted and agreed to be accountable for all aspects of the work.
Funding
The authors wish to thank the public for donating money to our funding agencies: SG was a recipient of the CIHR CGS-M and the Maternal and Child Health (MatCH) Scholarship (supported by the University of Alberta, Stollery Children's Hospital Foundation, Women and Children's Health Research Institute, and the Lois Hole Hospital for Women). MC was a recipient of the SSHRC Insight, SSHRC Insight Development, and NSERC Discovery Grants. GS was a recipient of the Heart and Stroke Foundation/University of Alberta Professorship of Neonatal Resuscitation, a National New Investigator of the Heart and Stroke Foundation Canada and an Alberta New Investigator of the Heart and Stroke Foundation Alberta.
Conflict of Interest
GS has registered the RETAIN table-top simulator (Tech ID 2017083) and the RETAIN digital simulator under Canadian copyright (Tech ID – 2017086) and is an owner of RETAIN Labs Medical Inc., Edmonton, Canada (https://www.retainlabsmedical.com/index.html).
The remaining authors declare that the research was conducted in the absence of any commercial or financial relationships that could be construed as a potential conflict of interest.
Acknowledgments
The authors wish to thank the resuscitation team at the Royal Alexandra Hospital for their generous participation in this study.
Supplementary Material
The Supplementary Material for this article can be found online at: https://www.frontiersin.org/articles/10.3389/fped.2020.599638/full#supplementary-material
Abbreviations
COVID-19, Coronavirus disease 2019; GEE, Generalized estimating equation; GLMM, Generalized linear mixed model; HCP, Healthcare professional; IQR, Interquartile range; NICU, Neonatal Intensive Care Unit; NRP, Neonatal Resuscitation Program; QIC, Quasi-information criterion; RETAIN, REsuscitation TrAINing; SD, Standard deviation.
References
1. Lawn JE, Manandhar A, Haws RA, Darmstadt GL. Reducing one million child deaths from birth asphyxia–a survey of health systems gaps and priorities. Heal Res Policy Syst. (2007) 5:4. doi: 10.1186/1478-4505-5-4
2. The Joint Commission. Sentinel Event Alert: Preventing Infant Death and Injury During Delivery. The Joint Commission (2004).
3. Weiner GM. Textbook of Neonatal Resuscitation (NRP). 7th ed. Zaichkin J, editor. Elk Grove Village, IL: American Academy of Pediatrics (2016).
4. Al-Elq AH. Simulation-based medical teaching and learning. J Fam Commun Med. (2010) 17:35–40. doi: 10.4103/1319-1683.68787
6. Ghoman SK, Patel SD, Cutumisu M, von Hauff P, Jeffery T, Brown MRG, et al. Serious games, a game changer in teaching neonatal resuscitation? A review. Arch Dis Child Fetal Neonatal Ed. (2020) 105:98–107. doi: 10.1136/archdischild-2019-317011
7. Harrington CM, Chaitanya V, Dicker P, Traynor O, Kavanagh DO. Playing to your skills: a randomised controlled trial evaluating a dedicated video game for minimally invasive surgery. Surg Endosc. (2018) 32:3813–21. doi: 10.1007/s00464-018-6107-2
8. Gray MM, Thomas AA, Burns B, Umoren RA. Evacuation of vulnerable and critical patients multimodal simulation for nurse-led patient evacuation. Simul Healthc. (2020) 15:382–7. doi: 10.1097/SIH.0000000000000451
9. Ghoman SK, Cutumisu M, Schmölzer GM. Simulation-based summative assessment of neonatal resuscitation providers using the RETAIN serious board game—a pilot study. Front Pediatr. (2020) 8:14. doi: 10.3389/fped.2020.00014
10. Cutumisu M, Patel SD, Brown MRG, et al. RETAIN: a board game that improves neonatal resuscitation knowledge retention. Front Pediatr. (2019) 7:13. doi: 10.3389/fped.2019.00013
11. Ghoman SK, Cutumisu M, Schmölzer GM. The RETAIN simulation-based serious game—a review of the literature. Healthcare. (2020) 8:3. doi: 10.3390/healthcare8010003
12. Cutumisu M, Brown MRG, Fray C, Schmölzer GM. Growth mindset moderates the effect of the Neonatal Resuscitation Program on performance in a computer-based game training simulation. Front Pediatr. (2018) 6:195. doi: 10.3389/fped.2018.00195
13. R Core Team. R: A Language and Environment for Statistical Computing (2020). Available online at: https://www.r-project.org
14. Bates D, Mächler M, Bolker B, Walker S. Fitting linear mixed-effects models using lme4. J. Stat. Softw. (2015) 67:1–48. doi: 10.18637/jss.v067.i01
15. Walker KF, Cohen AL, Walker SH, Allen KM, Baines DL, Thornton JG. The dangers of the day of birth. BJOG An Int J Obstet Gynaecol. (2014) 121:714–18. doi: 10.1111/1471-0528.12544
16. World Health Organization. Global Health Risks. (2009). Available online at: http://www.who.int/healthinfo/global_burden_disease/GlobalHealthRisks_report_full.pdf (accessed July 24, 2020).
17. Ersdal HL, Vossius C, Bayo E, Mduma E, Perlman J, Lippert A, et al. A one-day “Helping Babies Breathe” course improves simulated performance but not clinical management of neonates. Resuscitation. (2013) 84:1422–7. doi: 10.1016/j.resuscitation.2013.04.005
18. Thomas EJ, Sexton JB, Lasky RE, Helmreich RL, Crandell DS, Tyson J. Teamwork and quality during neonatal care in the delivery room. J Perinatol. (2006) 26:163–9. doi: 10.1038/sj.jp.7211451
19. Carbine DN, Finer NN, Knodel E, Rich W. Video recording as a means of evaluating neonatal resuscitation performance. Pediatrics. (2000) 106:654–8. doi: 10.1542/peds.106.4.654
20. Garvey AA, Dempsey EM, Dempsey EM. Simulation in neonatal resuscitation. Front Pediatr. (2020) 8:59. doi: 10.3389/fped.2020.00059
21. Mileder L, Urlesberger B, Szyld E, Roehr CC. Simulation-based neonatal and infant resuscitation teaching : a systematic review of randomized controlled trials. Klin Padiatr. (2014) 226:259–67. doi: 10.1055/s-0034-1372621
22. Matterson HH, Szyld D, Green BR, Howell HB, Pusic MV, Mally PV, et al. Neonatal resuscitation experience curves : simulation based mastery learning booster sessions and skill decay patterns among pediatric residents. J Perinat Med. (2018) 46:934–41. doi: 10.1515/jpm-2017-0330
23. Dörner R, Göbel S, Effelsberg W, Wiemeyer J. Introduction. In: Dörner R, Göbel S, Effelsberg W, Wiemeyer J, editors. Serious Games: Foundations, Concepts and Practice. Cham: Springer International Publishing (2016). p. 1–34.
24. Gick ML, Holyoak KJ. Schema induction and analogical transfer. Cogn Psychol. (1983) 15:1–38. doi: 10.1016/0010-0285(83)90002-6
Keywords: neonatal resuscitation, simulation based education, digital simulation, healthcare education, simulation, table-top simulator, digital simulator
Citation: Ghoman SK, Cutumisu M and Schmölzer GM (2021) Digital Simulation Improves, Maintains, and Helps Transfer Health-Care Providers' Neonatal Resuscitation Knowledge. Front. Pediatr. 8:599638. doi: 10.3389/fped.2020.599638
Received: 27 August 2020; Accepted: 02 December 2020;
Published: 18 January 2021.
Edited by:
Arjan Te Pas, Leiden University, NetherlandsReviewed by:
Marije Hogeveen, Radboud University Nijmegen Medical Centre, NetherlandsBritt Nakstad, University of Oslo, Norway
Copyright © 2021 Ghoman, Cutumisu and Schmölzer. This is an open-access article distributed under the terms of the Creative Commons Attribution License (CC BY). The use, distribution or reproduction in other forums is permitted, provided the original author(s) and the copyright owner(s) are credited and that the original publication in this journal is cited, in accordance with accepted academic practice. No use, distribution or reproduction is permitted which does not comply with these terms.
*Correspondence: Georg M. Schmölzer, georg.schmoelzer@me.com