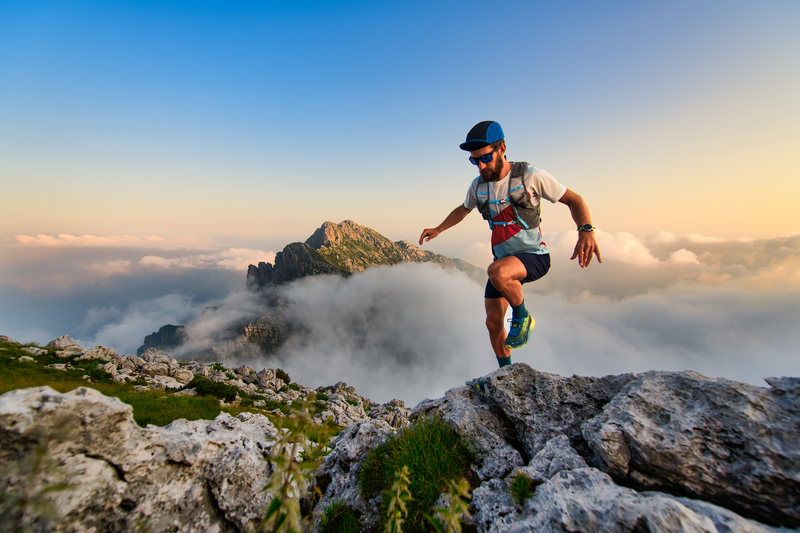
94% of researchers rate our articles as excellent or good
Learn more about the work of our research integrity team to safeguard the quality of each article we publish.
Find out more
ORIGINAL RESEARCH article
Front. Pediatr. , 16 April 2020
Sec. Obstetric and Pediatric Pharmacology
Volume 8 - 2020 | https://doi.org/10.3389/fped.2020.00171
Background: This study proposes a quantitative 2-stage procedure to detect potential drug-induced liver injury (DILI) signals in pediatric inpatients using an data warehouse of electronic health records (EHRs).
Methods: Eight years of medical data from a constructed database were used. A two-stage procedure was adopted: (i) stage 1: the drugs suspected of inducing DILI were selected and (ii) stage 2: the associations between the drugs and DILI were identified in a retrospective cohort study.
Results: 1,196 drugs were filtered initially and 12 drugs were further potentially identified as suspect drugs inducing DILI. Eleven drugs (fluconazole, omeprazole, sulfamethoxazole, vancomycin, granulocyte colony-stimulating factor (G-CSF), acetaminophen, nifedipine, fusidine, oseltamivir, nystatin and meropenem) were showed to be associated with DILI. Of these, two drugs, nystatin [odds ratio[OR]=1.39, 95%CI:1.10–1.75] and G-CSF (OR = 1.91, 95%CI:1.55–2.35), were found to be new potential signals in adults and children. Three drugs [nifedipine [OR = 1.77, 95%CI:1.26–2.46], fusidine [OR = 1.43, 95%CI:1.08–1.86], and oseltamivi r [OR = 1.64, 95%CI:1.23–2.18]] were demonstrated to be new signals in pediatrics. The other drug-DILI associations had been confirmed in previous studies.
Conclusions: A quantitative algorithm to detect potential signals of DILI has been described. Our work promotes the application of EHR data in pharmacovigilance and provides candidate drugs for further causality assessment studies.
Drug-induced liver injury (DILI) is a serious public health issue and potentially serious adverse reaction that can acute liver failure. The incidence of DILI in developed countries is estimated to be 19/100,000 in the general population (1). Rates of DILI in inpatient wards are higher, ranging from 0.12 to 1.4 per 100 admissions (2). It accounts for 4–10% of all adverse drug reactions (ADR) and up to 13–15% of liver failure, with 29% of the liver failure cases had liver transplantation in American adults (3, 4). Recently, DILI has become the most important cause of post-marketing warnings and drug withdrawals (5). Moreover, children and adolescents, with a lack of clinical trials and immature liver and kidney function, are more prone to DILI than adults (6, 7). Thus, the detection of DILI signals is very important for post-marketing surveillance, especially in pediatric patients.
Traditionally, spontaneous reporting systems (SRSs), as the passive systems collecting reports of adverse drug events (ADEs), are the most common resources for monitoring DILI signals. However, these passive surveillance methods are limited by under-reporting, poor report quality, reporting bias, and unable to calculate the frequency of ADEs (8). A previous study showed that <6% of hepatic adverse reactions were reported (9). The expanding use of electronic health records (EHRs) during these years provides another potentially abundant source for pharmacovigilance and allows the use of larger populations, including children and adolescents, in population-based studies. These data are more practical and contribute to a more precise benefit-risk assessment.
Several studies have explored the signals of DILI in routinely collected data from EHRs, such as laboratory results and diagnosis codes (10–13). However, few studies focused on the drugs suspected of inducing DILI in children and adolescents. The study aims to conduct a two-stage algorithm with retrospective cohort designs to explore and evaluate potential DILI signals from large dataset of EHR. Thus, it can offer suspect drugs for pharmacovigilance and causality assessment researches of ADRs.
A database containing the inpatients of Beijing Children's Hospital (BCH) was established previously, which including detailed visits, medications, clinical diagnosis as well as laboratory tests from January 1, 2010 to December 31, 2017. The database used in this study contained 379,160 hospital records from 247,136 patients aged 28 days to 18 years old, involving a total of 49,685,862 laboratory tests and 8,927,894 prescriptions. A hospitalization record represented one hospitalization, so there were multiple records if the same patients was hospitalized more than one time. In this study, all the data we used for eligible patients were exported from the data warehouse and deidentified to protect patients' privacy and confidentiality.
Potentially DILI is mainly identified by temporary changes in laboratory chemical indicators related to drug use. According to the Guidelines for Medical Nomenclature Use of Adverse Drug Reactions, which was issued by the National Center for ADR Monitoring of the China Food and Drug Administration (CFDA) in 2016 (14), DILI was defined when the drug was administered within the therapeutic dose range, and the following events occurred within 90 days of initial administration: (1) any elevation of alanine aminotransferase (ALT) or total bilirubin (TB) greater than the upper limit of normal range (>ULN) in two successive tests; or (2) any elevation of ALT or TB greater than two times the ULN (>2 × ULN) in one test. The ULNs for the laboratory tests at the BCH were 40 IU/L and 20.5 mmol/L for ALT and TB, respectively. Alkaline phosphomonoesterase (ALP) was not chosen because increases in ALP activities in pediatric patients are mostly due to bones or other organs rather than liver disease (15).
The purpose of stage 1 was to identify potentially offending medications that deserved further research regarding their associations with DILI (shown in Figure 1). Only chemical medicine was involved in this study. When a patient used two or more drugs in one record, the record will be included in each drug's signal exploration, respectively. The main steps were as follows:
(1) The hospital records that obtained at least two laboratory tests (ALT or TB) from admission to discharge were included;
(2) The hospital records that obtained an initial ALT/TB results under the ULN and its report time (T1) were retained;
(3) The hospital records containing a diagnosis of hepatobiliary disease (16) (shown in Table S1) that influenced the ALT or TB levels were excluded. The rest hospital records were defined as Group 1. This step was executed because the changes in ALT/TB levels of patients with hepatobiliary disease might be largely due to the progression of hepatobiliary disease itself, rather than DILI.
(4) The hospital records of patients with DILI according to ALT and TB levels from Group 1 were included in Group 2.
(5) The time of the first abnormal ALT/TB test were considered as T2. All drug prescriptions during the period from T1 to T2 in every record were collected. Duplicate prescription information was deleted.
(6) The hospital records in Group 2 and Group1 was considered to be the number of drug adverse events (a) and the total number of drug users (b), respectively. Then ratio (a/b) for each drug was calculated.
(7) The suspect drug that met following criteria were selected after expert consultation: (1) ratio (a/b)>0.15; (2) total users (b) >1,000. The a/b values of adjuvant drugs, such as normal saline and glucose injection, ranged from 0.09 to 0.11, which can be regarded as the value of background. And if b is too small, there may be a greater risk of bias when doing subsequent statistical analysis.
Figure 1. The workflow of stage 1 for screening drugs potentially causing DILI. ALT, alanine aminotransferase; ULN, upper limit of normal range; DILI, drug-induced liver injury; TB, total bilirubin.
The overall framework of stage 2 was displayed in Figure 2. The purpose of this step was to study the associations between drugs and DILI by comparing differences in DILI event rates between the exposed and unexposed group after adjusting for four confounders. According to retrospective cohort designs, every suspect drug filtered out in stage 1 was analyzed as follows:
(1) Exposed group:
1) The hospital records with the suspect drugs were included.
2) The hospital records that obtained at least two ALT or TB results before and after taking the suspect drug were included.
3) The hospital records that obtained the latest ALT or TB results within the ULNs before the first dose of medication were retained.
4) The hospital records that obtain diagnosed hepatobiliary diseases were also excluded (shown in Table S1).
5) For the rest records that obtained abnormal ALT or TB levels, the records which used the hepatoprotectants before the first report time of abnormal test were excluded (shown in Table S2). And for the rest records that did not obtained abnormal ALT or TB levels, the records which used the hepatoprotectants during the entire hospitalization were excluded.
(2) Unexposed group:
1) The hospital records without the suspect drugs were selected.
2) The hospital records that obtained at least two ALT or TB results from admission to discharge were identified.
3) The hospital records that obtained initial ALT or TB results within the ULNs were included.
4) The hospital records that obtained diagnosed hepatobiliary diseases were also excluded.
5) For the rest records that obtained abnormal ALT or TB levels, the records with hepatoprotectants before the time of the first abnormal ALT or TB levels were excluded. For the rest records that did not obtained abnormal ALT or TB levels, the records with the hepatoprotectants during hospitalization were excluded.
(3) DILI signal detection
1) Each exposed record was paired to four unexposed records randomly after adjusting age, gender, admission time, and major diagnosis (based on the classification in ICD-10).
2) The odds ratio (OR) and its 95% confidence interval (CI) was calculated using the unconditional logistic regression.
3) An OR>1.0 indicated a positive signal, otherwise a negative signals (OR ≤ 1).
Figure 2. The overall design of stage 2 for the detection of DILI signals based on retrospective cohort designs. ALT, alanine aminotransferase; ULN, upper limit of normal range; DILI, drug-induced liver injury; OR, odds ratio; TB, total bilirubin.
The available knowledge from literature search as well as summary of product characteristics (SPCs) was used to evaluate the novelty of the DILI signals. The SPCs reviewed from micromedex (https://www.ibm.com/watson-health/learn/micromedex), FDA website (https://www.fda.gov) or drug instructions. Literature reviewed through PUBMED (https://pubmed.ncbi.nlm.nih.gov), Wanfang (http://www.wanfangdata.com.cn/index.html) as well as CNKI (http://www.cnki.net/).
Data management was performed by MySQL (Version 14.14). Statistical analysis was performed using R3.5.1 software. The GraphPad Prism 8.0.1 software was used to produce figures.
The possible confounding factors in exposed groups and unexposed groups were matched by propensity score matching (PSM) approach. Logistic regression model was used to calculate propensity scores, with drug exposure or not as dependent variables and four confounding factors (age, gender, admission time, and main diagnosis) as covariates. The nearest neighbor matching principle was used and matching ratio was set to 1: 4 in this process. The balance of covariates across the two groups in the matched sample was finally verified.
All P-values were reported two-sided. P < 0.05 represented statistical significance. The missing data was processed by the listwise deletion approach due to the low missing probabilities (<5%).
In stage 1, 1,196 drugs were filtered initially. After combining the same ingredient drugs with different dosages, specifications or manufacturers, 171 drugs remained. After excluding hepatoprotectants and adjuvant drugs, such as normal saline, 53 drugs were left. Among them, 12 drugs (fluconazole, omeprazole, sulfamethoxazole, vancomycin, phenobarbital, granulocyte colony-stimulating factor (G-CSF), acetaminophen, nifedipine, fusidine, oseltamivir, nystatin, and meropenem) met the inclusion criteria (b>1,000 and a/b>0.15). These twelve drugs were considered as suspect drugs and chosen for stage 2 with regard to DILI signals detection. More information was shown in Table 1.
Table 2 listed the data extraction workflow of the above 12 suspect drugs. According to the design of stage 2, there were 2,004 cases in which fluconazole was used, along with 2,295 cases with omeprazole, 878 cases with sulfamethoxazole, 1,970 cases with vancomycin, 982 cases with phenobarbital, 2,051 cases with G-CSF, 1,609 cases with acetaminophen, 714 cases with nifedipine, 712 cases with fusidine, 957 cases with oseltamivir, 1,105 cases with nystatin, and 1,687 cases with meropenem in exposed groups. The exposed group and unexposed group were matched by gender, age, admission time, and major diagnosis with the ratio 1:4. The basic clinical information between two groups was described in Table S3.
Out of the 12 suspect drugs, fluconazole (OR = 2.04, 95%CI: 1.71–2.42, P < 0.0001), omeprazole (OR = 1.56, 95%CI: 1.30–1.87, P < 0.0001), sulfamethoxazole (OR = 2.97, 95%CI: 2.28–3.87, P < 0.0001), vancomycin (OR = 1.73, 95%CI: 1.44–2.07, P < 0.0001), G-CSF (OR = 1.91, 95%CI: 1.55–2.35, P < 0.0001), acetaminophen (OR = 2.28, 95%CI: 1.89–2.75, P < 0.0001), nifedipine (OR = 1.77, 95%CI: 1.26–2.46, P < 0.001), fusidine (OR = 1.43, 95%CI: 1.08–1.86, P = 0.01), oseltamivir (OR = 1.64, 95%CI: 1.23, 2.18, P < 0.001), nystatin (OR = 1.39, 95%CI: 1.10–1.75, P = 0.01), and meropenem (OR = 2.37, 95%CI: 1.99–2.82, P < 0.0001) were found to be associated with DILI as positive signals (shown in Figure 3). Although phenobarbital tended toward being a positive signal with regard to DILI, it did not reach statistically significance (OR = 1.25, 95%CI:0.98, 1.59, P = 0.068). Table 3 described the results of 12 drugs with regard to their associations with DILI.
Figure 3. The forest plot for the 12 suspect drugs-DILI associations. DILI, drug-induced liver injury; OR, odds ratio; G-CSF, granulocyte colony-stimulating factor; CI, confidence interval.
According to available knowledge at present, the novelty of 11 positive DILI signals observed in stage 2 were further evaluated (shown in Table 4). Two drugs, namely, nystatin and G-CSF, were found to be possible new DILI signals as they had not been previously documented in researches in pediatric population and adults. In addition, three other drugs, namely, nifedipine, fusidine and oseltamivir, have not been reported as being associated with liver injury in pediatric patients, although these associations have been found in adults. The remaining drugs have been reported as being associated with liver injury in both adults and pediatric individuals. In addition, for all drugs except nystatin, there were currently details regarding DILI as potentially ADEs in the SPCs.
We conducted a study on the development and application of a quantitative pharmacovigilance algorithm to identify signals of DILI from routine EHR data. This study used a two-stage designed algorithm with selecting offending medications firstly and then determining the associations between DILI and drugs. Two new DILI signals that have never been documented in pediatric population were found using the real world data from EHR. These may become candidate drugs for pharmacovigilance and causality assessment studies.
The association of nystatin with DILI was found to be a possible new signal. As far as we know, this has not previously been reported in published documents for patients of any age and was also not labeled in the SPCs. Nystatin is an antifungal agent widely used to treat oropharyngeal candidiasis, candidiasis of the skin, and cutaneous and mucocutaneous infections in pediatrics. The adverse effects listed in its SPCs include diarrhea, nausea, vomiting, abdominal pain, hypersensitivity reaction, and Stevens-Johnson syndrome. Unlike in the United States, nystatin tablets are still marketed for Chinese children and adolescents (>5 years old). Although liver injury has never been specifically mentioned in association with nystatin, it has been reported as an undesirable side effect of the use of other systemic antifungal agents. An in vitro study found that nystatin may decrease P-gp activity, indicating the possible mechanism of hepatotoxicity (17). A recent case report showed elevated liver enzymes after combining the cyclosporine and nystatin, due to drug interactions (18). Further investigations about the potential association between nystatin and hepatotoxicity are needed.
The association of G-CSF with liver injury can be considered another new signal. G-CSF is a blood modifying agent widely used to treat neutropenia in patients with non-myeloid malignancies, marrow transplantation, and acute myeloid leukemia treated with chemotherapy in pediatric patients. It can be used in children (except premature neonates, newborns and infants) with close monitoring in China. The adverse effects listed in the SPCs including rash, anemia, diarrhea, and bone pain. Although G-CSF has been on the market for many years, its liver safety in children is still unclear due to insufficient researches in specific population. Despite no report of such an association in pediatrics, several case reports have demonstrated that G-CSF may increase ALT or AST levels in adults (19). Our results are the first to show that G-CSF might be associated with adverse hepatic reactions in children, which needs further investigation.
Three other drug-DILI associations (nifedipine, fusidine and oseltamivir) were identified as potentially new signals in pediatrics. Nifedipine is a dihydropyridine calcium channel blocker that remains a commonly prescribed medication for hypertension in pediatric patients. A very rare but known drug adverse reaction of nifedipine is hepatotoxicity, which has been described in the literature in adults (20). Fusidine is widely used in treating severe staphylococcal infections in children. The hepatotoxicity of fusidine in adults, manifested as jaundice and abnormal liver function tests, has been reported in many adult studies. Oseltamivir is an ethyl ester prodrug used to prevent and treat infections caused by influenza A and B viruses. A report launched by Medicines and Healthcare Products Regulatory Agency (MHRA) showed that oseltamivir could induce DILI, without clearly indicating the patients' ages. In summary, although these drugs have been used worldwide, there are still some controversies regarding their hepatic safety in children due to the lack of evidence. Our study may provide more clues for further research in pediatrics.
Finally, the remaining 6 drug-DILI associations found in this study have been widely known in both the adult and pediatric populations based on the available descriptions in the SPCs and literature. This may suggest to some degree that our method can produce reliable results. On the other hand, some of the drugs implicated in DILI that were widely known were not found in our study, such as rifampicin, isoniazid, atorvastatin and so on. This result does not mean no such associations, but rather because the prevalence of drugs exposure was too limited to detect DILI signals in pediatric or in BCH hospital.
Data-driven analytic methods are a valuable aid to the detection of ADEs from large EHRs for drug safety monitoring (21). One of the most valuable methods is based on the traditional pharmacoepidemiological approach (22). The basic principle of these designs is to identify two groups of patients due to exposures or events retrospectively or prospectively and calculate the ratio of the drug-event associations (21). The cohort design provides more solutions for addressing putative confounders than the modified disproportionality analysis (DPA), which was originally developed on SRS (23, 24). Different designs based on this type of method, such as the new user cohort design, matched case-control designs and self-controlled designs, were determined to have the ability to track ADEs linked to medical products by many agencies. For instance, the Korean researchers have developed an approach, namely, Comparison of the Laboratory Extreme Abnormality Ratio (CLEAR), to identify possible ADE signals from abnormal value of laboratory test (11, 25, 26).
In the present study, our algorithm is a matched case-control design pharmacoepidemiological approach. In comparison with CLEAR, our 2-stage designed approach has certain advantages with regard to methodology. In the process of selecting the drugs suspected of causing DILI, we roughly assessed the potentialities by computing the ratio of ADEs to drug users. This important additional step increased the efficiency and speed of subsequent steps. In addition, more complicated confounders, such as relevant diagnoses with clear competing causes and medications that may affect the level of relevant laboratory indicators, were excluded to enhance the reliability and accuracy of the results. These final results suggest that our method is a valuable tool to facilitate earlier signal detection using routine EHR data.
Some limitations of this study should be considered. First, this study focuses on the detection of DILI signals using routine EHR database, whereas causality assessment was not involved. Large retrospective medical datasets have certain inherent difficulties for performing ADR causality assessments such as its incomplete data, uncontrollable confounding factors as well as difficulties in data extraction and algorithm execution. Next step we will prospectively collect data and use well-known causality assessment scales, such as Roussel Uclaf Causality Assessment Method (RUCAM), to verify the potential candidate drugs found in this study (15, 27). Second, some possible residual confounders, such as concomitant drugs, dose-related effects and the time-varying confounding by underlying diseases, were not excluded and could have led to potential bias or imprecision. Third, this study included only drugs with a large number of users for screening their possibility of causing DILI. This may lead a risk for missing potential drugs. We will mine the DILI signals for the remaining drugs in our next study.
Regulatory agencies have spared no efforts for facilitating ADE signal detection through multiple heterogeneous data sources at present (28–31). Notable progress has been made in China in establishing the project “China ADR Sentinel Surveillance Alliance” (CASSA). At present, we have developed an automated program based on this algorithm, and adapted to other ADEs besides DILI, such as drug-induced thrombocytopenia, neutropenia, anemia, and so on. In the next step, more attention will be paid to integrate these multiple modules to a drug safety monitoring platform to afford quick-response tools for pediatric clinicians and pharmacists. Future research will also focus on tighter integration of the structured data and clinical narratives in EHRs to improve the accuracy and scalability of the method.
In this work, we demonstrated a pharmacovigilance method to explore potentially DILI signals using real word data. The two-stage designed algorithm was performed to select suspect drugs firstly and then determine the associations between DILI and drugs, respectively. We found that 11 drugs were possibly associated with hepatotoxicity, including two previously undocumented signals, three potentially new signals in children and six well-known signals. Our work promotes the application of EHR datasets in pharmacovigilance and offers candidate drugs for further causality assessment studies.
The raw data supporting the conclusions of this article will be made available by the authors, without undue reservation, to any qualified researcher.
The study was reviewed and approved by the Institutional Ethics Committee of Beijing Children's Hospital in China (2019-k-5).
LJ and XW undertook work of framework design and overall guidance of whole research. YY, ZS, YX, XZ, ZD, RW, DF, and YL took responsibility for the data collection. YY and XN performed the data processing and statistical analysis. YY and LJ were responsible for the article writing and data interpretation. XP and QZ provided important methodological advice.
This work were supported by Major Project of National Science and Technology Major New Drug Creation (No. 2017ZX09304029), Natural Science Foundation of Beijing Municipality (No. 7194265), and National Natural Science Foundation of China (No. 81400766).
The authors declare that the research was conducted in the absence of any commercial or financial relationships that could be construed as a potential conflict of interest.
The Supplementary Material for this article can be found online at: https://www.frontiersin.org/articles/10.3389/fped.2020.00171/full#supplementary-material
1. Bjornsson ES, Bergmann OM, Bjornsson HK, Kvaran RB, Olafsson S. Incidence, presentation, and outcomes in patients with drug-induced liver injury in the general population of Iceland. Gastroenterology. (2013) 144:1419–25, 25 e1–3; quiz e19–20. doi: 10.1053/j.gastro.2013.02.006
2. Sobhonslidsuk A, Poovorawan K, Soonthornworasiri N, Pan-Ngum W, Phaosawasdi K. The incidence, presentation, outcomes, risk of mortality and economic data of drug-induced liver injury from a national database in Thailand: a population-base study. BMC Gastroenterol. (2016) 16:135. doi: 10.1186/s12876-016-0550-0
3. Shin J, Hunt CM, Suzuki A, Papay JI, Beach KJ, Cheetham TC. Characterizing phenotypes and outcomes of drug-associated liver injury using electronic medical record data. Pharmacoepidemiol Drug Saf. (2013) 22:190–8. doi: 10.1002/pds.3388
4. Ostapowicz G, Fontana RJ, Schiodt FV, Larson A, Davern TJ, Han SH, et al. Results of a prospective study of acute liver failure at 17 tertiary care centers in the United States. Ann Intern Med. (2002) 137:947–54. doi: 10.7326/0003-4819-137-12-200212170-00007
5. Temple R. Hy's law: predicting serious hepatotoxicity. Pharmacoepidemiol Drug Saf. (2006) 15:241–3. doi: 10.1002/pds.1211
6. Amin MD, Harpavat S, Leung DH. Drug-induced liver injury in children. Curr Opin Pediatr. (2015) 27:625–33. doi: 10.1097/MOP.0000000000000264
7. Kumar A, Sood V, Khanna R, Verma SK, Mehra N, Rawat D, et al. Clinical spectrum and outcome of pediatric drug induced liver injury. Indian J Pediatr. (2018) 85:676–8. doi: 10.1007/s12098-017-2570-3
8. Pitts PJ, Le Louet H. Advancing drug safety through prospective pharmacovigilance. Ther Innov Regul Sci. (2018) 52:400–2. doi: 10.1177/2168479018766887
9. Sgro C, Clinard F, Ouazir K, Chanay H, Allard C, Guilleminet C, et al. Incidence of drug-induced hepatic injuries: a French population-based study. Hepatology. (2002) 36:451–5. doi: 10.1053/jhep.2002.34857
10. Hohl CM, Karpov A, Reddekopp L, Doyle-Waters M, Stausberg J. ICD-10 codes used to identify adverse drug events in administrative data: a systematic review. J Am Med Inform Assoc. (2014) 21:547–57. doi: 10.1136/amiajnl-2013-002116
11. Yoon D, Park MY, Choi NK, Park BJ, Kim JH, Park RW. Detection of adverse drug reaction signals using an electronic health records database: comparison of the laboratory extreme abnormality ratio (CLEAR) algorithm. Clin Pharmacol Ther. (2012) 91:467–74. doi: 10.1038/clpt.2011.248
12. Harpaz R, DuMouchel W, Shah NH, Madigan D, Ryan P, Friedman C. Novel data-mining methodologies for adverse drug event discovery and analysis. Clin Pharmacol Ther. (2012) 91:1010–21. doi: 10.1038/clpt.2012.50
13. Lin C, Karlson EW, Dligach D, Ramirez MP, Miller TA, Mo H, et al. Automatic identification of methotrexate-induced liver toxicity in patients with rheumatoid arthritis from the electronic medical record. J Am Med Inform Assoc. (2015) 22:e151–61. doi: 10.1136/amiajnl-2014-002642
14. National Center for ADR Monitoring C. Guidelines for Medical Nomenclature Use of Adverse Drug Reactions. Beijing: Center for Drug Reevaluation, NMPA (2016).
15. Murray KF, Hadzic N, Wirth S, Bassett M, Kelly D. Drug-related hepatotoxicity and acute liver failure. J Pediatr Gastroenterol Nutr. (2008) 47:395–405. doi: 10.1097/MPG.0b013e3181709464
16. Suzuki A, Brunt EM, Kleiner DE, Miquel R, Smyrk TC, Andrade RJ, et al. The use of liver biopsy evaluation in discrimination of idiopathic autoimmune hepatitis versus drug-induced liver injury. Hepatology. (2011) 54:931–9. doi: 10.1002/hep.24481
17. Jodoin J, Demeule M, Fenart L, Cecchelli R, Farmer S, Linton KJ, et al. P-glycoprotein in blood-brain barrier endothelial cells: interaction and oligomerization with caveolins. J Neurochem. (2003) 87:1010–23. doi: 10.1046/j.1471-4159.2003.02081.x
18. Liu W, Guan X, Yu Z, Chen K, Benet L, Zhai S. A Drug-drug Interaction between cyclosporine and nystatin. Clin Ther. (2018) 40:660–2. doi: 10.1016/j.clinthera.2018.02.008
19. Li S G YJ, Chen L. Literature analysis of adverse reactions of recombinant human granulocyte colony-stimulating factor. Cent South Pharm. (2011) 9:932–36.
20. Yusuf D, Christy J, Owen D, Ho M, Li D, Fishman MJ. A case report of nifedipine-induced hepatitis with jaundice. BMC Res Notes. (2018) 11:228. doi: 10.1186/s13104-018-3322-9
21. Arnaud M, Begaud B, Thurin N, Moore N, Pariente A, Salvo F. Methods for safety signal detection in healthcare databases: a literature review. Expert Opin Drug Saf. (2017) 16:721–32. doi: 10.1080/14740338.2017.1325463
22. Schuemie MJ. Methods for drug safety signal detection in longitudinal observational databases: LGPS and LEOPARD. Pharmacoepidemiol Drug Saf. (2011) 20:292–9. doi: 10.1002/pds.2051
23. Schuemie MJ, Coloma PM, Straatman H, Herings RM, Trifiro G, Matthews JN, et al. Using electronic health care records for drug safety signal detection: a comparative evaluation of statistical methods. Med Care. (2012) 50:890–7. doi: 10.1097/MLR.0b013e31825f63bf
24. Ryan PB, Schuemie MJ, Gruber S, Zorych I, Madigan D. Empirical performance of a new user cohort method: lessons for developing a risk identification and analysis system. Drug Saf. (2013) 36(Suppl. 1):S59–72. doi: 10.1007/s40264-013-0099-6
25. Park MY, Yoon D, Lee K, Kang SY, Park I, Lee SH, et al. A novel algorithm for detection of adverse drug reaction signals using a hospital electronic medical record database. Pharmacoepidemiol Drug Saf. (2011) 20:598–607. doi: 10.1002/pds.2139
26. Tham MY, Ye Q, Ang PS, Fan LY, Yoon D, Park RW, et al. Application and optimisation of the comparison on extreme laboratory tests (CERT) algorithm for detection of adverse drug reactions: transferability across national boundaries. Pharmacoepidemiol Drug Saf. (2018) 27:87–94. doi: 10.1002/pds.4340
27. Danan G, Teschke R. RUCAM in drug and herb induced liver injury: the update. Int J Mol Sci. (2015) 17:14. doi: 10.3390/ijms17010014
28. Trifiro G, Fourrier-Reglat A, Sturkenboom MC, Diaz Acedo C, Van Der Lei J, Group E-A. The EU-ADR project: preliminary results and perspective. Stud Health Technol Inform. (2009) 148:43–9. doi: 10.3233/978-1-60750-043-8-43
29. Platt R, Carnahan RM, Brown JS, Chrischilles E, Curtis LH, Hennessy S, et al. The U.S. Food and Drug Administration's Mini-Sentinel program: status and direction. Pharmacoepidemiol Drug Saf. (2012) 21:1–8. doi: 10.1002/pds.2343
30. Stang PE, Ryan PB, Racoosin JA, Overhage JM, Hartzema AG, Reich C, et al. Advancing the science for active surveillance: rationale and design for the observational medical outcomes partnership. Ann Intern Med. (2010) 153:600–6. doi: 10.7326/0003-4819-153-9-201011020-00010
Keywords: post-marketing surveillance, drug safety, drug-induced liver injury, electronic health records, pediatrics
Citation: Yu Y, Nie X, Song Z, Xie Y, Zhang X, Du Z, Wei R, Fan D, Liu Y, Zhao Q, Peng X, Jia L and Wang X (2020) Signal Detection of Potentially Drug-Induced Liver Injury in Children Using Electronic Health Records. Front. Pediatr. 8:171. doi: 10.3389/fped.2020.00171
Received: 05 November 2019; Accepted: 25 March 2020;
Published: 16 April 2020.
Edited by:
Judith Ann Smith, University of Texas Health Science Center at Houston, United StatesReviewed by:
Saskia N. De Wildt, Radboud University Nijmegen, NetherlandsCopyright © 2020 Yu, Nie, Song, Xie, Zhang, Du, Wei, Fan, Liu, Zhao, Peng, Jia and Wang. This is an open-access article distributed under the terms of the Creative Commons Attribution License (CC BY). The use, distribution or reproduction in other forums is permitted, provided the original author(s) and the copyright owner(s) are credited and that the original publication in this journal is cited, in accordance with accepted academic practice. No use, distribution or reproduction is permitted which does not comply with these terms.
*Correspondence: Lulu Jia, amx1eXVAMTI2LmNvbQ==; Xiaoling Wang, d2FuZ3hpYW9saW5nQGJjaC5jb20uY24=
†These authors have contributed equally to this work and share first authorship
‡These authors have contributed equally to this work and share senior authorship
Disclaimer: All claims expressed in this article are solely those of the authors and do not necessarily represent those of their affiliated organizations, or those of the publisher, the editors and the reviewers. Any product that may be evaluated in this article or claim that may be made by its manufacturer is not guaranteed or endorsed by the publisher.
Research integrity at Frontiers
Learn more about the work of our research integrity team to safeguard the quality of each article we publish.