- 1Division of Pediatrics, School of Medicine, Pontificia Universidad Catolica de Chile, Santiago, Chile
- 2Asthma and Airway Disease Research Center, University of Arizona, Tucson, AZ, United States
Asthma is no longer considered a single disease, but a common label for a set of heterogeneous conditions with shared clinical symptoms but associated with different cellular and molecular mechanisms. Several wheezing phenotypes coexist at preschool age but not all preschoolers with recurrent wheezing develop asthma at school-age; and since at the present no accurate single screening test using genetic or biochemical markers has been developed to determine which preschooler with recurrent wheezing will have asthma at school age, the asthma diagnosis still needs to be based on clinical predicted models or scores. The purpose of this review is to summarize the existing and most frequently used asthma predicting models, to discuss their advantages/disadvantages, and their accomplishment on all the necessary consecutive steps for any predictive model. Seven most popular asthma predictive models were reviewed (original API, Isle of Wight, PIAMA, modified API, ucAPI, APT Leicestersher, and ademAPI). Among these, the original API has a good positive LR~7.4 (increases the probability of a prediction of asthma by 2–7 times), and it is also simple: it only requires four clinical parameters and a peripheral blood sample for eosinophil count. It is thus an easy model to use in any rural or urban health care system. However, because its negative LR is not good, it cannot be used to rule out the development of asthma.
Introduction
Wheezing is a sign associated with a highly heterogeneous set of conditions, with different pathogenesis and different outcomes during early life. Many epidemiological studies have shown, for example, that a large proportion of preschool children who wheeze, even recurrently, show remission of their symptoms either by age three, or during the school years (1, 2). Based on these findings, several early childhood wheezing phenotypes were described based on their natural history and associated risk factors (2). Since these phenotypes were described based on longitudinal data, they are of little use to the clinician when making therapeutic decisions affecting children whose future course is obviously unknown to him. Much more useful was a recent clinical trial showing that, although as a group, preschool children respond better to inhaled corticosteroids (ICS) than to montelukast, children who are not sensitized to aeroallergens or do not have peripheral blood eosinophilia respond equally well to both medicines (3). Thus, the clinician has now easily available tools to assess which preschool children are more likely to respond to ICS and in which children alternative approaches are still needed.
Of similar importance for the clinician, however, is the prognosis of the preschool child requiring medical attention for recurrent wheezing. Once atypical causes of wheezing are ruled out (e.g., cystic fibrosis, airway malformations, foreign bodies, etc.), parents are still eager to know if their child has “asthma,” which in their mind and in that of many clinicians is equated with a chronic disease that will persist and require treatment for extended periods during childhood and into adult life. Moreover, the great challenge toward the future is asthma prevention, and there is now solid data suggesting that in most cases of persistent asthma, the first symptoms most often develop during the school years (4), and it is during this age that the deficits in lung function growth, which are characteristic of the disease first appear (5–7). This has stimulated the search for indices or models that, combining different risk factors known to increase persistence of symptoms in young wheezing children, would allow identifying children more likely to have symptoms during the school years.
The purpose of this review is to compare and contrast different prediction tools developed during the last two decades. As stated, earlier, the role of these tools is not to facilitate attributing labels (“asthma”) that have little meaning in the face of the complexity and heterogeneity of wheezing conditions in this age group. At this moment, indices and models are also not useful for identifying children who will or will not respond to asthma therapy during the school years. Much more appropriate for this latter purpose are clinical trials such as the INFANT study (3) in which phenotypes and biomarkers are directly tested for their capacity to predict which children will respond to a certain therapy as compared to other therapies or placebo. The lain purpose of these indices and models is, as stated to predict outcomes and therefore, to allow clinicians to have meaningful discussions about prognosis with parents and caregivers, and to open the way to prevention studies that will be targeted to children at the highest risk of developing persistent symptoms later in life.
Clinical prediction rules (CPR) are decision-making clinical tools that use variables of medical history, physical examination, and simple laboratory tests to provide the probability of an outcome, prognosis, or likely response to treatment in an individual patient (8). At this point, it is very important to remark that the clinical usefulness of a diagnostic test is determined by the extent to which it helps to modify the pretest probability of occurrence of a certain diagnosis. For this purpose, the calculation and application of likelihood ratios (LR) is a very useful tool, reflecting the magnitude by which the pretest probability increases or decreases and thereby helping the physician rule out, confirm, or continue investigating a diagnosis with new tests (9). Therefore, LR, not the sensitivity or positive predicted value, is the best parameter reflecting the diagnostic accuracy of any diagnosis or prognosis model (10, 11).
It is important to remember that four consecutive steps are needed for prognostic or diagnostic prediction rules to become universal accepted for massive use. These steps are: development, validation, impact, and implementation (12).
Predictive Models for Prognostic or Diagnostic Tools
Step 1: Development
At this moment, at least seven predictive models or scores for asthma have been developed. The first model was the Asthma Predictive Index (API) or original API (13), followed by the PIAMA (14), Isle of Wight (15), modified API (mAPI) (16), University of Connecticut (ucAPI) (17), Asthma Prediction Tool (APT) from Leicester (18), and Asthma Detection and Monitoring (ademAPI) (19). The characteristics and predictors used in these models were shown in Table 1.
In clinical prediction rules, each score level represents a different LR. Calculation is based on a proportion, in which we consider in the numerator, the proportion of patients with the condition with the given score level, and in the denominator, the proportion of patients without the condition with the given score level (9). For the purpose of simplifying, scores are sometimes dichotomized into positive and negative, i.e., scores are collapsed into two categories yielding LR considered as positive or negative, when given group of predictors are present or absent.
Regarding prediction of asthma, the positive LR is the probability of a child with active asthma to have been classified as being at risk divided by the probability of a child without active asthma to have been classified as being at risk. The negative LR is the probability of a child with active asthma to have been classified as not being at risk divided by the probability of a child without active asthma to have been classified as not being at risk. Traditionally the negative LRs < 0.1 and positive LRs > 10 are considered to be conclusive, whilst LRs in the range of 0.2–5 are of limited usefulness. However, beside the number, it is more important how big the change between the pre-test probabilities and the post-test probability is (9).
The LRs of these different prediction models for assessing the development of asthma at school age were: original stringent API (13) (+LR = 7.43 and –LR = 0.75, for asthma at age 6 years); Isle of Wight (14) (+LR = 3.41 and –LR = 0.56, for asthma at age 10 years); PIAMA (15) (score ≥ 20, +LR = 2.5 and –LR = 0.53, for asthma at age 7–8 years); mAPI (16) (+LR = 21 and –LR = 0.84, for asthma at age 6 years); ucAPI (17) (+LR = 7.5 and –LR = 0.6, for asthma at age 7 years); APT (18) (+LR = 2.5 and –LR = 0.4, for asthma at 6–8 years); and the adem-API (19) (+LR = 8.8 and –LR = 0.13, for asthma at age 6 years) (Table 2). For example, to determine the risk of asthma in preschoolers from countries with high (e.g., Brazil), medium (e.g., Chile), and low (e.g., China) asthma prevalence, using the positive original stringent API, the probability for developing asthma increases by 2, 4, and 7 times (the pretest probability of asthma moves from 40 to 80%, from 14 to 62%, and from 3 to 21%, respectively) (20) (Figure 1). However, since their negative LR is not good, it cannot be used to rule out the probability for the development of asthma.
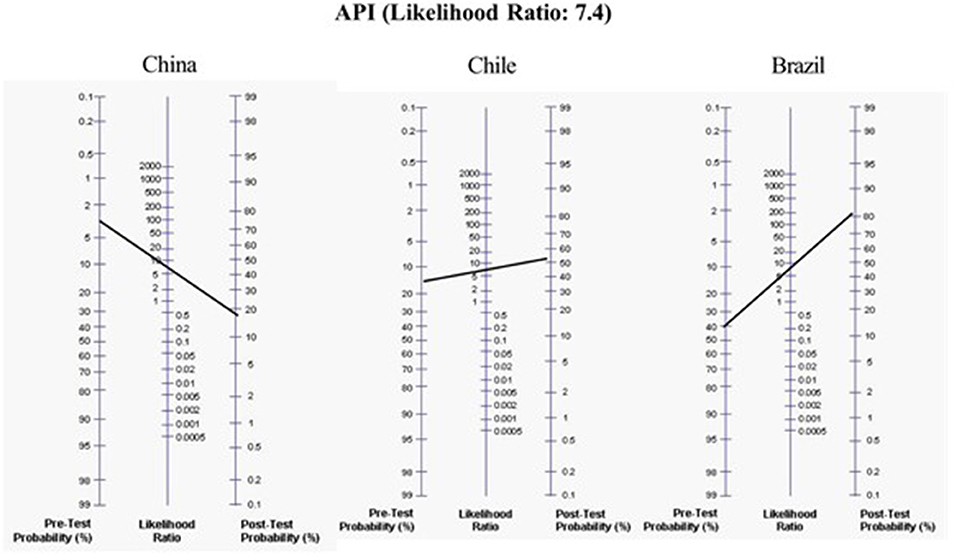
Figure 1. Application of the original positive Asthma predictive index (API) in hypothetical different scenarios with a low, moderate, or high-risk population of having asthma at school age.
Several important issues need to be addressed to determine the internal validity of the optimal prediction model. Among these seven models, only the original API (13) is relatively generalizable since it was developed in an unselected ethnically diverse birth cohort. The Isle of Wight (14) and PIAMA (15) included patients with respiratory tract/recurrent chest infections among their many criteria which could misrepresent the reporting of episodes of recurrent wheezing. The PIAMA (15) is more laborious to determine because the many criteria used have different weights, in addition, its generalizability may be reduced since it includes health beliefs and socioeconomic information that may vary between ethnicities and countries. The ucAPI (17) even though it was the first one that used an objectively confirmed asthma (change in FEV1 of ≥ 12% post bronchodilator or a methacholine challenge test PC20 ≤ 4 mg/ml), it was developed in a high-risk cohort. Similarly, the mAPI initially proposed by expert opinion was recently also developed in a high-risk of atopy birth cohort (16). Moreover, it is well-known that peripheral blood eosinophilia is a better predictor of remission of asthma, than specific IgE or skin prick test (21, 22). Therefore, predictive indices that use peripheral blood eosinophilia (a cheaper and worldwide common test) instead of specific or total IgE or skin prick test, will be more useful for predicting asthma. In addition, in the literature (23) recently started the debate about the cutoff for specific IgE (> 0.35 kUa/L) or positive skin test (≥3 mm) generally considered as indices of sensitization, being arbitrary and having not been defined by a scientific study; moreover, they depend on age and sex (23). Therefore, the incorporation of specific IgE or positive skin test in any predictive model without taking these consideration are questionable. The APT (18) tool was developed in high risk population (children came from health care visits because of respiratory problems) and incorporated age, gender, and eight clinical wheezing/atopy characteristics.
The latest model developed was the ademAPI (19). It included a prospective case-control study, adding to original API (but replacing eosinophilia by specific IgE) the following parameters: 17 exhaled volatile organic compounds (collected and detected by gas chromatography–time of flight–mass spectrometry), 10 exhaled breath condensate biomarkers (cytokines and chemokines analyzed with a multiplex immunoassay), 31 genes expression, and lung function measured by airway resistance using MicroRint®. This model is maybe the most complete and sophisticated model, but although it reaches the best positive and negative LRs (8.8 and 0.13, respectively), the possibility to be used massively in any healthy setting worldwide is very unlikely due to the high costly and sophisticated predictors included. Moreover, the increase of positive LR from 7.4 (original API) to 8.8 (ademAPI) might not justify all the sophisticate/expensive biomarkers used in the later tool.
Step 2: Validation
After developing any prognosis model the next step is the validation, i.e., “testing the accuracy of the prediction rule in patients that were not included in the development study. Temporal, geographical, and domain validations can be distinguished. If necessary, the prediction rule can be updated, by combining the information captured in the rule (development study) and the data of the new patients (validation study)” (12).
At this moment, only the original API, PIAMA, and APT models were validated in new populations. The original API was validated in three different independent large cohorts and one small high-risk cohort. In the general population of Leicester Cohort from Switzerland (24) (n = 3,392), the performance of API to predict asthma at 7 years was LR (+) 5.3 and LR (–) 0.7 for the stringent API, and LR (+) 2.9 and LR (–) 0.5 for the loose API; at 10 years the figure was similar. In the high risk wheezer population (n = 1,573) of the PIAMA (15) cohort, the API performance to predict asthma at 7.8 years was LR (+) 5.3 for the stringent API and LR (+) 2.8 for the loose API. And in a nested case control study (n = 616) from a birth cohort in Norway (25) the stringent API for asthma at 10 years. was LR (+) 3.3 LR (–) 0.5. In a small study in Colombian recurrent wheezer preschoolers (n = 130) from outpatient clinic the API performance for predict asthma at 5–6 years was LR (+) 2.1 for stringent and 1.1 for the loose API (26). It is important to note that a different combination of API criteria was used in two validated studies (15, 24) that made the interpretation difficult.
In contrast, the PIAMA was validated in a high-risk population (n = 2,877) the Dutch R-Generation (27) where their performance for asthma at 6 years was AUC = 0.74; and in the small Colombian study where their LR (+) was 2.6 for asthma at 5–6 years (26). Also, the PIAMA score used in the Dutch study was not identical to the one use in the developed study. Finally, the APT score was validated in only a small high-risk population German study (28) (n = 140) where their performance for predicted asthma at 8 years was LR (+) 2.6, LR (–) 0.3, and AUC = 0.83.
Step 3: Impact
The next step is to determine the impact of the CPR, i.e., “determining whether a validated prediction rule is used by physicians, changes therapeutic decisions, improves clinically relevant process parameters, improves patient outcome or reduces costs” (12). The ideal design for an impact study, is a randomized controlled study, in which the “intervention” group is randomized to use the CPR and the control group to use standard or usual judgement. Only a limited number of CPR worldwide have completed this step, probably because of the big methodological challenge and high costs of such studies for CPRs.
At this time, none of the prediction models has moved forward to this stage. Therefore, there is little evidence to support impact and implementation. Nevertheless, at this moment the original stringent API has been the most common predicted model tested worldwide in different non-randomized studies, the other was the mAPI. For example, Wi et al. (29) performed a cross-sectional study on 105 American children (5.8 ± 1.5 years. old) assessing performance of “retrospective” API against Predetermined Asthma Criteria, showing that the agreement rate and kappa between those were 89.5% and 0.66 (p < 0.01). This could suggest that application of API to retrospective studies for ascertaining asthma status is suitable. Similarly, a case-control study (30) on 202 Chilean children aged 6–7 years showed that those with current asthma had a positive API when they already were preschoolers [OR = 84.3 (24–436)]. In a case-control study (31) on 113 Brazilian children (aged 6–24 months) comparing recurrent wheezing vs. controls, the positive stringent API remained an important risk factor for recurrent wheezing [aOR = 5.57 [2.23–7.96], p < 0.001]. A study (32) on 529 Turkish school children with history of recurrent wheezing, reported that those with negative API in the past, had significantly shortened wheezing duration.
A recent cross-sectional study (33), nested in a US birth cohort, was done to develop and validate a natural language processing (NLP) algorithm to identify patients that meet the original API criteria. Asthma status ascertained by manual chart review based on API criteria served as gold standard. NLP-API was developed on a training cohort (n = 87) and validated on a test cohort (n = 427, median age of 5.3 years). The NLP-API predicted asthma with sensitivity 86%, specificity 98%, positive PV 88%, negative PV 98%. NLP-API was able to ascertain asthma status in children mining from electronic health records and has a potential to enhance asthma care and research through population management and large-scale studies when identifying children who meet API criteria.
Also, the original API was used to explore the effect of a smart nebulizing device on the rate of adherence to ICS in Chinese preschoolers with positive API (n = 65), showing a significant improve in the rate of adherence, and reducing the frequency of emergency visits and respiratory infections, as well as the usage of antibiotics or systemic steroids (34). A recent study (35) in Chinese Han preschoolers (n = 385) reported association of four-gene model consisting of IL13 rs20541, IL4 rs2243250, ADRB2 rs1042713, and FCER1B rs569108 with the original API [OR = 4.08, p < 0.0001; and OR = 2.36, p < 0.0001, for stringent and loose API, respectively].
Also, the original stringent API was compared and correlated with surrogated markers of airway inflammation using two non-invasive tests, i.e., fractional exhaled nitric oxide (FeNO) and exhaled breath condensate (EBC) pH. In a study on 391 Switzerland preschoolers (age 3–47 mo), FeNO was significantly higher in those with positive stringent API than positive loose API or recurrent cough without history of wheeze controls (36). Also, in a study on 32 Spanish infants (median age of 12 mo) with recurrent wheezing, those with positive API had significant higher FeNO levels than those with negative API (37). Similarly, a study on 52 Argentinian preschoolers (aged 5–36 mo) showed a significantly higher levels of FeNO in the positive API group than in those with negative API (38). Finally, a study on 191 German preschoolers (median age 4.4 years) reported that reduced EBC pH value combined with API improved the chance to identify asymptomatic children at high risk of asthma (AUC = 0.88 for positive API vs. AUC = 0.94 for positive API plus pH) (39).
Other inflammatory biomarkers e.g., periostin, CC16 and YK-40, were also tested in population with positive and negative original API. A study done in 48 Chilean preschoolers (aged 24–71 mo) reported no significant differences in serum periostin levels for those with positive API and negative API; and no significant correlation between serum periostin levels and peripheral blood eosinophils (40). Also, no difference in level of serum CC16 levels for preschoolers with a positive API and negative API were found in the same population (41). A correlation between serum CC16 levels and age was found [r = 0.36 [0.07–0.59], p = 0.01], but not between serum CC16 levels and peripheral blood eosinophils. Similarly, a recent study done in 98 Turkish preschoolers showed that periostin and angiopoietin serum levels were similar between positive and negative mAPI (42). Finally, a longitudinal Sweden study of 156 preschoolers with recurrent wheeze and 101 healthy controls, showed that YKL-40 levels were elevated during acute wheeze exacerbation in positive and negative API (maybe related with current neutrophilic inflammation) compared to controls, but not at 3 and 12 month follow-up after the acute exacerbation (43).
The API was also compared and correlated with airway inflammation in bronchial biopsies. A study on endobronchial biopsies obtained from 30 Czech preschoolers (median age 13.5 mo) who underwent flexible bronchoscopy for various clinical reasons found significant difference in the thickness of the basement membrane, subepithelial deposition of laminin, and collagen IV in the basement membrane between children predisposed to asthma-by positive API- and control group (44).
Since there were some criticism about the value of the original API, some studies were performed changing or adding new biomarkers in order to improve the API performance for predicting asthma at 6 years of age. For example, on 191 Germany preschoolers (median age 4.4 years), when adding an exhaled breath condensate pH to the original stringent API, the positive LR was lower compared to the original stringent API (LR (+) 5.88 vs. 7.43, respectively) (39). Similarly, replacing FeNO instead of blood eosinophil determination from the original API in 391 Swiss preschoolers (aged 3–47 mo) results in lower positive LR (1.99) for predicting asthma at age of 4 years than original stringent API (45). In other study, interleukin-1-receptor-like 1 or sST2 (a well-replicated asthma-gene and associates with eosinophilia) was compared to the API in 202 Dutch wheezing children and 50 healthy controls reporting that serum sST2 levels at 2–3 years could not distinguish which of the preschoolers developed asthma at school age; consequently, serum sST2 did not significantly add to the prediction of asthma diagnosis than the used of API (API alone vs. API+sST2: AUC = 0.60, p = 0.02 vs. AUC = 0.57, p = 0.12, respectively) (46).
Step 4: Implementation
Finally, the last step is the implementation, i.e., “actual dissemination of the diagnostic or prognostic prediction rule in daily practice to guide physicians with their patient management” (12). Although, like formerly exposed, none of the CPR has completed the impact step, the original API and mAPI are the only asthma prediction models that have been implemented worldwide over the years (Table 3).
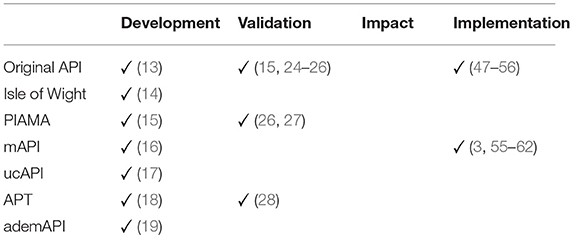
Table 3. Steps for develop a prognostic or diagnostic prediction model (12).
For example, the API has been used to explore the airway lung function in young asthmatic children. Using the tidal rapid thoraco-abdominal compression and raised volume technique, 50 Portuguese recurrent wheezing children aged 8–20 mo were compared to 30 controls, showing a significant lower z scores for FVC and FEF25−75 in those with positive than those with negative API (47). Later, a study of thoraco-abdominal compression technique on 91 recurrent wheezing Spanish preschoolers (aged up to 24 mo) showed that those with positive API had a lower Vmax FRC Z-score than negative API (48). A case-control study (49) on 108 Chilean recurrent wheezing preschoolers (aged 24–72 mo) showed no differences in basal lung function and post-bronchodilator response to salbutamol (by IOS or spirometry) between positive and negative API preschoolers. However, positive API preschoolers with ICS had significantly higher central basal airway resistance (RA at 20 Hz) and higher post-BD response (% change in FEF25−75 and FEV0.5) than those positive API without ICS; suggesting that preschoolers with positive API and ICS use may have some airway dysfunction (49). Recently, a Finish longitudinal study (50) about lung function assessed with IOS measured at preschool age (n = 255) and at adolescence, showed that abnormal baseline values (poor R5) during preschool were significantly associated with: low lung function, need for asthma medication, and asthma symptoms during adolescence. And a positive API at preschool age was associated with asthma symptoms (OR: 13.7 [1.4–147.1]) and need for asthma medication (OR: 14.6 [1.4–147.1]), but not with abnormal lung function at adolescence (50).
The mAPI has been also used to explore airway lung function. A study to assess whether the IOS has a diagnostic value to predict the mAPI in 115 Turkish preschoolers (median age 39 mo) with recurrent wheezing showed a significant improvement in the rate R5-R20% in children with positive mAPI compared to negative mAPI. The R5–R20% levels >14.4 had a sensitivity of 75% and specificity of 53% for predicting a positive mAPI [AUC: 0.656, p = 0.003] (57). In 34 Finnish children (aged 3–7 years) with recurrent wheezing measured by impedance pneumography at home during sleep, those with positive mAPI had significantly lower min curve shape correlation and minimum noise limit than negative mAPI, indicating a stronger change in flow profile shape and momentarily lowered chaoticity (58). Recently, the same group reported that children with positive mAPI presented reduced sympathvoagal balance, suggesting that high-risk of developing asthma might be related with a lack of adaptability of parasympathetic nervous system (59).
The original API has been also used in studies aimed to correlate with some nutrients. A Turkish study of 186 infants with recurrent wheezing compared with 118 healthy control peers, showed that those recurrent wheezing with negative API had significantly lower levels of vitamin D than those with positive API, and both had lower levels than controls (51). Similarly, a case-control study in n = 148 Turkish children (mean age 20.7 mo) showed lower serum levels of vitamin D and zinc, and higher Cu and Cu/Zn ratio in recurrent wheezing with positive API compared to those with negative API (52).
Finally, only the original API and mAPI has been used as recruitment tools in randomized control trials (RCTs). The API was used in two RCTs comparing ICS vs. placebo (53) and ICS vs. montelukast (54); and mAPI in four RCTs comparing ICS vs. placebo (60) and ICS vs. montelukast (3, 61, 62). Also, the original stringent API was endorsed by two asthma guidelines (55, 56), and the mAPI by one (55). A positive original API and mAPI preschool wheezer was proposed as one endotype, e.g., a Th2 mechanism and good responder to daily ICS, under the asthma syndrome umbrella (63). Two recent systematic reviews identified other prediction models for asthma but with considerable limitations (64, 65). A critical appraisal of asthma predictive models using the CHARMS checklist, revealed that original API, ATP, and PIAMA have low-moderate risk of bias (65). Finally, “several major strengths of the original API are in the design, with a simple set of equally weighted criteria with a binary scoring system: the criteria are either met or not met, allows easy implementation (only requires clinical parameters plus a peripheral blood sample for eosinophils count) and interpretation of the API” (66) in all type of rural and urban health services setting worldwide.
Conclusion
Among all the prediction models for asthma prognosis, the original API is in most use because it derives from unselected multiethnic population, is simple to recollect, cheaper, little invasive (e.g., peripheral blood count) and has been validated in external populations. It is a very promising predictive tool and the positive original stringent API should be able to be used by clinicians worldwide in any health setting, to identify at-risk children and educate parents on the importance of asthma maintenance therapy and treatment of flares. Its major strength is its positive LR~7.4 that allows an important effect on post-test probability of disease, improving significantly by 2–7 times the probability (Figure 1). But since its negative LR is not very low enough, it cannot be used to rule out the development of asthma.
Author Contributions
JC-R contributed to the study concept, literature search, data collection, and manuscript writing. LC and FM contributed to review the manuscript and incorporated significant thought.
Funding
JC-R contribution was funded in part by CONICYT PIA/ANILLO (Grant No. 170925013) from the Chilean Comisión Nacional de Investigación Científica y Tecnológica (CONICYT).
Conflict of Interest Statement
The authors declare that the research was conducted in the absence of any commercial or financial relationships that could be construed as a potential conflict of interest.
References
1. Martinez FD, Wright AL, Taussig LM, Holberg CJ, Halonen M, Morgan WJ. Asthma and wheezing in the first six years of life. The Group Health Medical Associates. N Engl J Med. (1995) 332:133–8. doi: 10.1056/NEJM199501193320301
2. Guilbert TW, Mauger DT, Lemanske RF Jr. Childhood asthma-predictive phenotype. J Allergy Clin Immunol Pract. (2014) 2:664–70. doi: 10.1016/j.jaip.2014.09.010
3. Fitzpatrick AM, Jackson DJ, Mauger DT, Boehmer SJ, Phipatanakul W, Sheehan WJ, et al. NIH/NHLBI AsthmaNet. Individualized therapy for persistent asthma in young children. J Allergy Clin Immunol. (2016) 138:1608–18.e12. doi: 10.1016/j.jaci.2016.09.028
4. Yunginger JW, Reed CE, O'Connell EJ, Melton LJ III, O'Fallon WM, Silverstein MD. A community-based study of the epidemiology of asthma. Incidence rates, 1964–1983. Am Rev Respir Dis. (1992) 146:888–94. doi: 10.1164/ajrccm/146.4.888
5. Phelan PD, Robertson CF, Olinsky A. The melbourne asthma study: 1964–1999. J Allergy Clin Immunol. (2002) 109:189–94. doi: 10.1067/mai.2002.120951
6. Sears MR, Greene JM, Willan AR, Wiecek EM, Taylor DR, Flannery EM, et al. A longitudinal, population-based, cohort study of childhood asthma followed to adulthood. N Engl J Med. (2003) 349:1414–22. doi: 10.1056/NEJMoa022363
7. Morgan WJ, Stern DA, Sherrill DL, Guerra S, Holberg CJ, Guilbert TW, et al. Outcome of asthma and wheezing in the first 6 years of life: follow-up through adolescence. Am J Respir Crit Care Med. (2005) 172:1253–8. doi: 10.1164/rccm.200504-525OC
8. McGinn T, Wyer P, McCullagh L, Wisvinesky J, Deveraux P, Stiell I, et al. Clinical prediction rules. In: Guyatt G, Rennie D, Meade M, Cook D, editors. Users' Guides to the Medical Literature. New York, NY: Mc Graw Hill (2008). p.407–18.
9. Cifuentes L, Cerda J. [Clinical use of diagnostic tests (Part 2). Clinical application and usefulness of a diagnostic test]. Rev Chil Infectol. (2010) 27: 316–9 doi: 10.4067/S0716-10182010000300004
10. Castro-Rodriguez JA. Another predictive score for childhood asthma: the search remains. J Allergy Clin Immunol Pract. (2014) 2:716–8. doi: 10.1016/j.jaip.2014.10.007
11. Richardson WS, Wilson MC. The process of diagnosis. In: Guyatt G, Rennie D, Meade M, Cook D, editors. Users' Guides to the Medical Literature. New York, NY: McGraw-H (2008). p. 399–406.
12. Toll DB, Janssen KJM, Vergouwe Y, Moons KGM. Validation, updating and impact of clinical prediction rules: a review. J Clin Epidemiol. (2008) 61:1085–94. doi: 10.1016/j.jclinepi.2008.04.008
13. Castro-Rodriguez JA, Holberg CJ, Wright AL, Martinez FD. A clinical index to define risk of asthma in young children with recurrent wheezing. Am J Respir Crit Care Med. (2000) 162:1403–6. doi: 10.1164/ajrccm.162.4.9912111
14. Kurukulaaratchy RJ, Matthews S, Holgate ST, Arshad SH. Predicting persistent disease among children who wheeze during early life. Eur Respir J. (2003) 22:767–71. doi: 10.1183/09031936.03.00005903
15. Caudri D, Wijga A, Schipper CMA, Hoekstra M, Postma DS, Koppelman GH, et al. Predicting the long-term prognosis of children with symptoms suggestive of asthma at preschool age. J Allergy Clin Immunol. (2009) 124:903–10.e1–7. doi: 10.1016/j.jaci.2009.06.045
16. Chang TS, Lemanske RF Jr, Guilbert TW, Gern JE, Coen MH, Evans MD, et al. Evaluation of the modified asthma predictive index in high-risk preschool children. J Allergy Clin Immunol Pract. (2013) 1:152–6. doi: 10.1016/j.jaip.2012.10.008
17. Amin P, Levin L, Epstein T, Ryan P, Le Masters G, Khurana-Hershey G, et al. Optimum predictors of childhood asthma: persistent wheeze or the asthma predictive index? J Allergy Clin Immunol Pract. (2014) 2:709–15. doi: 10.1016/j.jaip.2014.08.009
18. Pescatore AM, Dogaru CM, Duembgen L, Silverman M, Gaillard EA, Spycher BD, et al. A simple asthma prediction tool for preschool children with wheeze or cough. J Allergy Clin Immunol. (2014) 133:111–8.e1–13. doi: 10.1016/j.jaci.2013.06.002
19. Klaassen EM, van de Kant KD, Jöbsis Q, van Schayck OC, Smolinska A, Dallinga JW, et al. Exhaled biomarkers and gene expression at preschool age improve asthma prediction at 6 years of age. Am J Respir Crit Care Med. (2015) 191:201–7. doi: 10.1164/rccm.201408-1537OC
20. Castro-Rodriguez JA, Rodriguez-Martinez CE, Custovic A. Infantile and preschool asthma. In: Carlsen K-H, Gerritsen J, editors. Paediatric Asthma. Norwich: Eur Respir Mon (2012). p. 10–21.
21. Just J, Nicoloyanis N, Chauvin M, Pribil C, Grimfeld A, Duru G. Lack of eosinophilia can predict remission in wheezy infants? Clin Exp Allergy. (2008) 38:767–73. doi: 10.1111/j.1365-2222.2008.02966.x
22. Karakoc F, Remes ST, Martinez FD, Wright AL. The association between persistent eosinophilia and asthma in childhood is independent of atopic status. Clin Exp Allergy. (2002) 32:51–6. doi: 10.1046/j.0022-0477.2001.01273.x
23. Mohammad HR, Belgrave D, Kopec Harding K, Murray CS, Simpson A, Custovic A. Age, sex, and the association between skin test responses and IgE titres with asthma. Pediatr Allergy Immunol. (2016) 27:313–9. doi: 10.1111/pai.12534
24. Leonardi NA, Spycher BD, Strippoli MP, Frey U, Silverman M, Kuehni CE. Validation of the Asthma Predictive Index and comparison with simpler clinical prediction rules. J Allergy Clin Immunol. (2011) 127:1466–72. doi: 10.1016/j.jaci.2011.03.001
25. Devulapalli CS, Carlsen KC, Håland G, Munthe-Kaas MC, Pettersen M, Mowinckel P, et al. Severity of obstructive airways disease by age 2 years predicts asthma at 10 years of age. Thorax. (2008) 63:8–13. doi: 10.1136/thx.2006.060616
26. Rodriguez-Martinez CE, Sossa-Briceño MP, Castro-Rodriguez JA. Discriminative properties of two predictive indices for asthma diagnosis in a sample of preschoolers with recurrent wheezing. Pediatr Pulmonol. (2011) 46:1175–81. doi: 10.1002/ppul.21493
27. Hafkamp-de Groen E, Lingsma HF, Caudri D, Levie D, Wijga A, Koppelman GH, et al. Predicting asthma in preschool children with asthma-like symptoms: validating and updating the PIAMA risk score. J Allergy Clin Immunol. (2013) 132:1303–10. doi: 10.1016/j.jaci.2013.07.007
28. Grabenhenrich LB, Reich A, Fischer F, Zepp F, Forster J, Schuster A, et al. The novel 10-item asthma prediction tool: external validation in the German MAS birth cohort. PLoS ONE. (2014) 9:e115852. doi: 10.1371/journal.pone.0115852
29. Wi CI, Park MA, Juhn YJ. Development and initial testing of Asthma Predictive Index for a retrospective study: an exploratory study. J Asthma. (2015) 52:183–90. doi: 10.3109/02770903.2014.952438
30. Krause E, Grob K, Barria M, Calvo M. Association of the asthma predictive index and presence of the disease in children in Valdivia, Chile. Rev Chil Enf Respir. (2015) 31:8–16. doi: 10.4067/S0717-73482015000100002
31. de Sousa RB, Medeiros D, Sarinho E, Rizzo JÂ, Silva AR, Bianca AC. Risk factors for recurrent wheezing in infants: a case-control study. Rev Saude Publica. (2016) 50:15. doi: 10.1590/S1518-8787.2016050005100
32. Sahiner UM, Buyuktiryaki B, Cavkaytar O, Arik Yilmaz E, Soyer O, Sackesen C, et al. Recurrent wheezing in the first three years of life: short-term prognosis and risk factors. J Asthma. (2013) 50:370–5. doi: 10.3109/02770903.2013.770013
33. Kaur H, Sohn S, Wi CI, Ryu E, Park MA, Bachman K, et al. Automated chart review utilizing natural language processing algorithm for asthma predictive index. BMC Pulm Med. (2018) 18:34. doi: 10.1186/s12890-018-0593-9
34. Zhou Y, Lu Y, Zhu H, Zhang Y, Li Y, Yu Q. Short-term effect of a smart nebulizing device on adherence to inhaled corticosteroid therapy in Asthma Predictive Index-positive wheezing children. Patient Prefer Adherence. (2018) 12:861–8. doi: 10.2147/PPA.S162744
35. Bai S, Hua L, Wang X, Liu Q, Bao Y. Association of a four-locus gene model including IL13, IL4, FCER1B, and ADRB2 with the Asthma Predictive Index and atopy in Chinese Han children. J Investig Allergol Clin Immunol. (2018) 28:407–134. doi: 10.18176/jiaci.0272
36. Moeller A, Diefenbacher C, Lehmann A, Rochat M, Brooks-Wildhaber J, Hall GL, et al. Exhaled nitric oxide distinguishes between subgroups of preschool children with respiratory symptoms. J Allergy Clin Immunol. (2008) 121:705–9. doi: 10.1016/j.jaci.2007.11.008
37. Castro-Rodriguez JA, Sardón O, Pérez-Yarza EG, Korta J, Aldasoro A, Corcuera P, et al. Young infants with recurrent wheezing and positive asthma predictive index have higher levels of exhaled nitric oxide. J Asthma. (2013) 50:162–5. doi: 10.3109/02770903.2012.754030
38. Balinotti JE, Colom A, Kofman C, Teper A. Association between the Asthma Predictive Index and levels of exhaled nitric oxide in infants and toddlers with recurrent wheezing. Arch Argent Pediatr. (2013) 111:191–5. doi: 10.5546/aap.2013.eng.191
39. von Jagwitz M, Pessler F, Akmatov M, Li J, Range U, Vogelberg C. Reduced breath condensate pH in asymptomatic children with prior wheezing as a risk factor for asthma. J Allergy Clin Immunol. (2011) 128:50–5. doi: 10.1016/j.jaci.2011.03.005
40. Castro-Rodriguez JA, Atton I, Villarroel G, Serrano CA. Serum periostin is not related to asthma predictive index. Allergol Immunopathol. (2018) 46:235–40. doi: 10.1016/j.aller.2017.05.012
41. Castro-Rodriguez JA, Atton I, Villarroel G, Serrano CA. Relation between serum CC16 levels and asthma predictive index in pre-schoolers with recurrent wheezing. Allergol Immunopathol. (2018) 46:460–6. doi: 10.1016/j.aller.2017.09.024
42. Koksal BK, Aydin BO, Tekindal A, Ozbek OY. Angiopoietin-1, Angiopoietin-2, and Periostin levels in children with recurrent wheeze. Pediatr Aller Immunol Pulmonol. (2018) 31:230–33. doi: 10.1089/ped.2018.0953
43. James A, Stenberg Hammar K, Reinius L, Konradsen JR, Dahlén SE, Söderhäll C, Hedlin G. A longitudinal assessment of circulating YKL-40 levels in preschool children with wheeze. Pediatr Allergy Immunol. (2017) 28:79–85. doi: 10.1111/pai.12669
44. Berankova K, Uhlik J, Honkova L, Pohunek P. Structural changes in the bronchial mucosa of young children at risk of developing asthma. Pediatr Allergy Immunol. (2014) 25:136–42. doi: 10.1111/pai.12119
45. Singer F, Luchsinger I, Inci D, Knauer N, Latzin P, Wildhaber JH, et al. Exhaled nitric oxide in symptomatic children at preschool age predicts later asthma. Allergy. (2013) 68:531–8. doi: 10.1111/all.12127
46. Ketelaar ME, van de Kant KD, Dijk FN, Klaassen EM, Grotenboer NS, Nawijn MC, et al. Predictive value of serum sST2 in preschool wheezers for development of asthma with high FeNO. Allergy. (2017) 72:1811–5. doi: 10.1111/all.13193
47. Borrego LM, Stocks J, Leiria-Pinto P, Peralta I, Romeira AM, Neuparth N, et al. Lung function and clinical risk factors for asthma in infants and young children with recurrent wheeze. Thorax. (2009) 64:203–9. doi: 10.1136/thx.2008.099903
48. Keklikian E, Sanchez-Solis M, Bonina AJ, Meneguzzi A, Pastor-Vivero MD, Mondejar-Lopez P, et al. Do risk factors for persistent asthma modify lung function in infants and young children with recurrent wheeze? Pediatr Pulmonol. (2010) 45:914–8. doi: 10.1002/ppul.21260
49. Lezana V, Gajardo A, Bofill L, Gutierrez M, Mora S, Castro-Rodriguez JA. Airway tone dysfunction among pre-schoolers with positive asthma predictive index: a case-control study. Allergol Immunopathol. (2017) 45:169–74. doi: 10.1016/j.aller.2016.05.006
50. Lajunen K, Kalliola S, Kotaniemi-Syrjänen A, Sarna S, Malmberg LP, Pelkonen AS, et al. Abnormal lung function at preschool age asthma in adolescence? Ann Allergy Asthma Immunol. (2018) 120:520–6. doi: 10.1016/j.anai.2018.03.002
51. Ozdemir A, Dogruel D, Yilmaz O. Vitamin D status in infants with two different wheezing phenotypes. Indian J Pediatr. (2016) 83:1386–91. doi: 10.1007/s12098-016-2184-1
52. Uysalol M, Uysalol EP, Yilmaz Y, Parlakgul G, Ozden TA, Ertem HV, et al. Serum level of vitamin D and trace elements in children with recurrent wheezing: a cross-sectional study. BMC Pediatr. (2014) 14:270. doi: 10.1186/1471-2431-14-270
53. Brand PL, Luz Garcia-Garcia M, Morison A, Vermeulen JH, Weber HC. Ciclesonide in wheezy preschool children with a positive asthma predictive index or atopy. Respir Med. (2011) 105:1588–95. doi: 10.1016/j.rmed.2011.07.017
54. Li YQ, Xue HY, Chen W, Cao LF. [Application of asthma predictive index-based group therapy in wheezing children under 5 years of age]. Zhongguo Dang Dai Er Ke Za Zhi. (2014) 16:795–9.
55. Expert Panel, National Asthma Education and Prevention Program. Guidelines for the Diagnosis and Management Of Asthma. (2007). Available online at: www.nhlbi.nih.gov/guidelines/asthma/asthgdln.htm (accessed June 1, 2019).
56. From the Global Strategy for Asthma Management and Prevention, Global Initiative for Asthma (GINA). (2018). Available online at: https://ginasthma.org/ (accessed June 1, 2019).
57. Arikoglu T, Batmaz SB, Yildirim DD, Tezol Ö, Bozlu G, Kuyucu S. Asthma predictive index in relation to respiratory mechanics by impulse oscillometry in recurrent wheezers. Allergol Immunopathol. (2018) 46:190–5. doi: 10.1016/j.aller.2017.09.028
58. Seppä VP, Pelkonen AS, Kotaniemi-Syrjänen A, Viik J, Mäkelä MJ, Malmberg LP. Tidal flow variability measured by impedance pneumography relates to childhood asthma risk. Eur Respir J. (2016) 47:1687–96. doi: 10.1183/13993003.00989-2015
59. Milagro J, Gil E, Lazaro J, Seppa VP, Malmberg LP, Pelkonen AS, et al. Nocturnal heart rate variability spectrum characterization in preschool children with asthmatic symptoms. IEEE J Biomed Health Inform. (2018) 22:1332–40. doi: 10.1109/JBHI.2017.2775059
60. Guilbert TW, Morgan WJ, Zeiger RS, Mauger DT, Boehmer SJ, Szefler SJ, et al. Long-term inhaled corticosteroids in preschool children at risk for asthma. N Engl J Med. (2006) 354:1985–97. doi: 10.1056/NEJMoa051378
61. Bacharier LB, Phillips BR, Zeiger RS, Szefler SJ, Martinez FD, Lemanske RF Jr, et al. Episodic use of an inhaled corticosteroid or leukotriene receptor antagonist in preschool children with moderate to severe intermittent wheezing. J Allergy Clin Immunol. (2008) 122:1127–35. doi: 10.1016/j.jaci.2008.09.029
62. Zeiger RS, Mauger D, Bacharier LB, et al. Daily or intermittent budesonide in preschool children with recurrent wheezing. N Engl J Med. (2011) 365:1990–2001. doi: 10.1056/NEJMoa1104647
63. Lötvall J, Akdis CA, Bacharier LB, Bjermer L, Casale TB, Custovic A, et al. Asthma endotypes: a new approach to classification of disease entities within the asthma syndrome. J Allergy Clin Immunol. (2011) 127:355–60. doi: 10.1016/j.jaci.2010.11.037
64. Luo G, Nkoy FL, Stone BL, Schmick D, Johnson MD. A systematic review of predictive models for asthma development in children. BMC Med Inform Decis Mak. (2015) 15:99. doi: 10.1186/s12911-015-0224-9
65. Smit HA, Pinart M, Antó JM, Keil T, Bousquet J, Carlsen KH, et al. Childhood asthma prediction models: a systematic review. Lancet Respir Med. (2015) 3:973–84. doi: 10.1016/S2213-2600(15)00428-2
Keywords: asthma, asthma predictive index, biomarkers, preschool, predictive models, recurrent wheezing
Citation: Castro-Rodriguez JA, Cifuentes L and Martinez FD (2019) Predicting Asthma Using Clinical Indexes. Front. Pediatr. 7:320. doi: 10.3389/fped.2019.00320
Received: 12 February 2019; Accepted: 15 July 2019;
Published: 31 July 2019.
Edited by:
Kostas N. Priftis, National and Kapodistrian University of Athens, GreeceReviewed by:
Ching Ching, Kate Chan, The Chinese University of Hong Kong, ChinaAhmad Kantar, Independent Researcher, Bergamo, Italy
Copyright © 2019 Castro-Rodriguez, Cifuentes and Martinez. This is an open-access article distributed under the terms of the Creative Commons Attribution License (CC BY). The use, distribution or reproduction in other forums is permitted, provided the original author(s) and the copyright owner(s) are credited and that the original publication in this journal is cited, in accordance with accepted academic practice. No use, distribution or reproduction is permitted which does not comply with these terms.
*Correspondence: Jose A. Castro-Rodriguez, amFjYXN0cm8xN0Bob21haWwuY29t