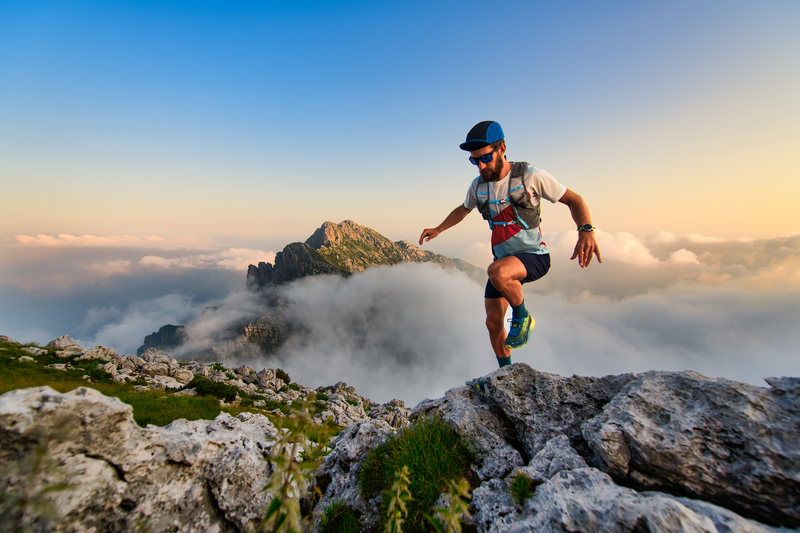
95% of researchers rate our articles as excellent or good
Learn more about the work of our research integrity team to safeguard the quality of each article we publish.
Find out more
EDITORIAL article
Front. Pediatr. , 05 November 2015
Sec. Neonatology
Volume 3 - 2015 | https://doi.org/10.3389/fped.2015.00095
This article is part of the Research Topic Perinatology in the era of big data and nanoparticles View all 6 articles
How do prenatal exposures to various stimuli impact postnatal development for the duration of a person’s life? To answer this, the tripartite challenge is biological, medical, and technological. The biological challenge is to understand the plethora of effects causing trajectory shifts. The medical and technological challenges are to identify and follow fetuses and babies at risk for diseases in later life. Systems to accomplish this must be deployable across variably equipped healthcare settings at a reasonable cost.
Many researchers believe that such challenges can be tackled with complex signals bioinformatics. This research topic attracted articles on fetal heart rate (FHR) monitoring during labor, optimization of technologies for pediatric ventilation and the impact of the developing neonatal microbiome on health over the life span. How do these topics connect? They all share a clinical and translational demand for integrating relatively large amounts of spatio-temporally distributed data from various modalities to reveal patterns not clearly discernible to the human eye, with the goal of optimizing medical decision-making.
The right idea has to arrive at the right time to be met by a technology that can implement it, to be taken up by practitioners, and to produce change in health outcomes (1–3). Although technology seems at hand, a paradigm shift is required for practitioners to embrace this challenge. We can no longer afford to rely on human perception alone to detect patterns in the twenty-first century’s onslaught of multi-modal and multi-dimensional data streams reflecting human health in acute and chronic care settings (4). Such data streams are commonly referred as Big Data.
Big data can be defined with three Vs: “high-volume, high-velocity, and high-variety information assets that demand cost-effective, innovative forms of information processing for enhanced insight and decision making” (5).
In this research topic, Durosier et al. show that sampling rate affects the ability of FHR monitoring to detect acidemia as it occurs during human labor (6). Acquiring ~250 times more data points per second than currently practiced in delivery rooms worldwide improves accuracy. New technologies, some re-discovered form the early 1980s, are now coming to market to address this need, although their indication for use in delivery rooms is not yet fully exploiting their potential (7–11). As current computing capacity no longer limits the sampling rate possibilities for online monitoring, “bigger” data are becoming a logical next step in improving health care (12). One challenge is the integration of live streams into electronic medical records to facilitate retrieval for diagnostic and research purposes and for medical decision-making in real time.
The common thread connecting Durosier’s work to the next study in the research topic is the notion of unveiling physiological variability using higher temporal resolution of data acquisition and modalities of human–machine interaction that account for the natural biological variability. In the case of fetal monitoring, this variability is contained in the subtle temporal FHR fluctuations that remain hidden to human eye when acquired with the conventional tools at a 4-Hz sampling rate.
Baudin et al. explore how biological variability is impacted by the various mechanical ventilation regimes when it comes to monitoring breathing patterns in infants requiring machine support (13, 14). Intuitively, machine ventilation algorithms that are most closely attuned to the physiological respiratory pattern produce breathing signals that most closely resemble those of the control infants. Although larger prospective studies are necessary to understand the differential impact of ventilatory modes on cardio-respiratory variability and their effect on clinical outcomes, this study shows the possibility of deploying off- or online tools to quantify the physiological variability in respiration from bedside pediatric data streams. In the long term, this might help to fine-tune the ventilation parameters beyond the current possibilities, accounting for the non-linear nature of respiratory patterns. Again, the theme of how biological variability can be usefully monitored in real time emerges.
Munyaka et al. explore early postnatal maturation of immune regulation as a function of the exposure to gut microbiome (15). The human gut houses up to 1014 bacteria, exceeding by ~10-fold the number of host cells. Microbiome–host immune system interactions appear to have a profound and life-lasting impact on the host’s health status beginning well before the baby is born (16–21). We are faced with the challenge of quantifying microbial diversity in space and time, which approximates at least two of the above “3 Vs” definition of the big data, variety, and volume. Although the previous manuscripts represent intuitive approaches to temporal profiling and pattern analysis of streaming data, embodying all three Vs, current approaches to microbiome analysis need to catch up to the third V (velocity) to provide higher spatiotemporal resolution (microbiome in different organs at various time points). Meanwhile, current studies are cross-sectional in nature, sometimes with multiple sampling of the same cohort over time. They can offer population-level insights into changes in the microbiome due to various exposures, but with low temporal or spatial resolution to gage intra- and interindividual microbial dynamics.
Sorani et al. provided an early proof-of-principle for creation of multivariate pattern recognition within physiological time series commonly acquired in an intensive care unit setting (22). Heat maps in which genes are displayed across the top row and related genes cluster together are commonly used in genetics. In their neurocritical care heat map, Sorani et al. replaced genes by physiological variables that cluster on the basis of association within and across patients into three groups of patients. Surprisingly, intracranial pressure (ICP) and fraction of inspired oxygen were clustered, leading to the identification of previously unrecognized ICP elevations during bedside suctioning. As a perinatal example of unexpected connections, multi-dimensional properties of fHRV encode signatures of inflammation (23–26) or progressive labor acidemia (10, 11, 27) and may relate to EEG parameters (28, 29). Modern machine learning will help to integrate microbiome indices and continuous bedside acquisition of multi-modal data to elucidate clinically relevant patterns and optimize treatment (4, 22). Data intelligence is the next logical step in evolution of health monitoring (2, 3, 30).
Focusing machine learning approaches on clinical questions that arise in perinatal medicine is needed as relatively little progress has been made in the past decades in regard to monitoring and translation into clinical practice, with the notable exception of the HeRo score monitors (31, 32). Recent advances in artificial intelligence have brought the vision of data-driven case identification and decision-making closer to reality (33–37).
Martin G. Frasch is a co-inventor of the related patent application entitled “EEG Monitor of Fetal Health” including U.S. Patent Application Serial No. 12/532,874 and CA 2681926 National Stage Entries of PCT/CA08/00580 filed March 28, 2008, with priority to US provisional patent application 60/908,587, filed March 28, 2007. No other conflicts of interest are declared.
MF gratefully acknowledges funding support from the Canadian Institutes of Health Research (CIHR) and Fonds de Recherche du Québec – Santé (FRQS).
1. Gawande A. Slow ideas. Some innovations spread fast. How do you speed the ones that don’t? The New Yorker (2013).
2. Moorman JR. The modern age of physiological measurement. Physiol Meas (2014) 35(2):93–5. doi: 10.1088/0967-3334/35/2/93
3. Seely AJ. Data intelligence is the future of monitoring. J Clin Monit Comput (2014) 28(4):325–7. doi:10.1007/s10877-014-9561-y
4. Hemphill JC, Andrews P, De Georgia M. Multimodal monitoring and neurocritical care bioinformatics. Nat Rev Neurol (2011) 7(8):451–60. doi:10.1038/nrneurol.2011.101
5. Laney D, Linden A, Buytendijk F, White A, Beyer MA, Chandler N, et al. Answering Big Data’s 10 Biggest Vision and Strategy Questions. #G00263047 accessed at Gartner, Inc. (2014). Available from: https://www.gartner.com/doc/2822220/answering-big-datas--biggest?docdisp=share&srcId=1-4398736771
6. Durosier LD, Green G, Batkin I, Seely AJ, Ross MG, Richardson BS, et al. Sampling rate of heart rate variability impacts the ability to detect acidemia in ovine fetuses near-term. Front Pediatr (2014) 2:38. doi:10.3389/fped.2014.00038
7. Patrick J, Campbell K, Carmichael L, Natale R, Richardson B. Daily relationships between fetal and maternal heart rates at 38 to 40 weeks of pregnancy. Can Med Assoc J (1981) 124(9):1177–8.
8. Doret M, Helgason H, Abry P, Goncalves P, Gharib C, Gaucherand P. Multifractal analysis of fetal heart rate variability in fetuses with and without severe acidosis during labor. Am J Perinatol (2011) 28(4):259–66. doi:10.1055/s-0030-1268713
9. Goncalves H, Costa A, Ayres-de-Campos D, Costa-Santos C, Rocha AP, Bernardes J. Comparison of real beat-to-beat signals with commercially available 4 Hz sampling on the evaluation of foetal heart rate variability. Med Biol Eng Comput (2013) 51(6):665–76. doi:10.1007/s11517-013-1036-7
10. Frasch MG, Xu Y, Stampalija T, Durosier LD, Herry C, Wang X, et al. Correlating multidimensional fetal heart rate variability analysis with acid-base balance at birth. Physiol Meas (2014) 35(12):L1–12. doi:10.1088/0967-3334/35/12/L1
11. Li X, Xu Y, Herry C, Durosier LD, Casati D, Stampalija T, et al. Sampling frequency of fetal heart rate impacts the ability to predict pH and BE at birth: a retrospective multi-cohort study. Physiol Meas (2015) 36(5):L1–12. doi:10.1088/0967-3334/36/5/L1
12. Hruban L, Spilka J, Chudáček V, Janků P, Huptych M, Burša M, et al. Agreement on intrapartum cardiotocogram recordings between expert obstetricians. J Eval Clin Pract (2015) 21(4):694–702. doi:10.1111/jep.12368
13. Baudin F, Wu HT, Bordessoule A, Beck J, Jouvet P, Frasch MG, et al. Impact of ventilatory modes on the breathing variability in mechanically ventilated infants. Front Pediatr (2014) 2:132. doi:10.3389/fped.2014.00132
14. Wu HT, Baudin F, Frasch MG, Emeriaud G. Respiratory variability during NAVA ventilation in children: authors’ reply. Front Pediatr (2015) 3:13. doi:10.3389/fped.2015.00013
15. Munyaka PM, Khafipour E, Ghia JE. External influence of early childhood establishment of gut microbiota and subsequent health implications. Front Pediatr (2014) 2:109. doi:10.3389/fped.2014.00109
16. Onderdonk AB, Delaney ML, DuBois AM, Allred EN, Leviton A; Extremely Low Gestational Age Newborns (ELGAN) Study Investigators. Detection of bacteria in placental tissues obtained from extremely low gestational age neonates. Am J Obstet Gynecol (2008) 198(1):e111–7. doi:10.1016/j.ajog.2007.05.044
17. Hecht JL, Onderdonk A, Delaney M, Allred EN, Kliman HJ, Zambrano E, et al. Characterization of chorioamnionitis in 2nd-trimester C-section placentas and correlation with microorganism recovery from subamniotic tissues. Pediatr Dev Pathol (2008) 11(1):15–22. doi:10.2350/07-06-0285.1
18. Onderdonk AB, Hecht JL, McElrath TF, Delaney ML, Allred EN, Leviton A, et al. Colonization of second-trimester placenta parenchyma. Am J Obstet Gynecol (2008) 199(1):e51–2. doi:10.1016/j.ajog.2007.11.068
19. Prince AL, Chu DM, Seferovic MD, Antony KM, Ma J, Aagaard KM. The perinatal microbiome and pregnancy: moving beyond the vaginal microbiome. Cold Spring Harb Perspect Med (2015) 5(6). doi:10.1101/cshperspect.a023051
20. Sherman MP, Zaghouani H, Niklas V. Gut microbiota, the immune system, and diet influence the neonatal gut-brain axis. Pediatr Res (2015) 77(1–2):127–35. doi:10.1038/pr.2014.161
21. Liu HL, Butcher J, Romain G, Cao M, Durosier LD, Burns P, et al. Fetal gut microbiome diversity is modulated by subclinical ileum inflammation due to systemic endotoxin exposure and by vagal denervation. ESPGHAN (Journal of Pediatric Gastroenterology Nutrition, Amsterdam) (2015) 60(Suppl 1):227.
22. Sorani MD, Hemphill JC III, Morabito D, Rosenthal G, Manley GT. New approaches to physiological informatics in neurocritical care. Neurocrit Care (2007) 7(1):45–52. doi:10.1007/s12028-007-0043-7
23. Gotsch F, Romero R, Kusanovic JP, Mazaki-Tovi S, Pineles BL, Erez O, et al. The fetal inflammatory response syndrome. Clin Obstet Gynecol (2007) 50(3):652–83. doi:10.1097/GRF.0b013e31811ebef6
24. Prout AP, Frasch MG, Veldhuizen RA, Hammond R, Ross MG, Richardson BS. Systemic and cerebral inflammatory response to umbilical cord occlusions with worsening acidosis in the ovine fetus. Am J Obstet Gynecol (2010) 202(1):e81–9. doi:10.1016/j.ajog.2009.08.020
25. Ross MG, Jessie M, Amaya K, Matushewski B, Durosier LD, Frasch MG, et al. Correlation of arterial fetal base deficit and lactate changes with severity of variable heart rate decelerations in the near-term ovine fetus. Am J Obstet Gynecol (2013) 208(4):e281–6. doi:10.1016/j.ajog.2012.10.883
26. Xu A, Durosier LD, Ross MG, Hammond R, Richardson BS, Frasch MG. Adaptive brain shut-down counteracts neuroinflammation in the near-term ovine fetus. Front Neurol (2014) 5:110. doi:10.3389/fneur.2014.00110
27. Durosier LD, Herry CL, Cortes M, Cao M, Burns P, Desrochers A, et al. Does heart rate variability reflect the systemic inflammatory response in a fetal sheep model of lipopolysaccharide-induced sepsis? Physiol Meas (2015) 36(10):2089–102. doi:10.1088/0967-3334/36/10/2089
28. Wang X, Durosier LD, Ross MG, Richardson BS, Frasch MG. Online detection of fetal acidemia during labour by testing synchronization of EEG and heart rate: a prospective study in fetal sheep. PLoS One (2014) 9(9):e108119. doi:10.1371/journal.pone.0108119
29. Frasch MG, Durosier LD, Gold N, Cao M, Matushewski B, Keenliside L, et al. Adaptive shut-down of EEG activity predicts critical acidemia in the near-term ovine fetus. Physiol Rep (2015) 3(7). doi:10.14814/phy2.12435
30. Seely AJ, Kauffman SA, Bates JH, Macklem PT, Suki B, Marshall JC, et al. Proceedings from the Montebello Round Table Discussion. Second annual conference on complexity and variability discusses research that brings innovation to the bedside. J Crit Care (2011) 26(3):325–7. doi:10.1016/j.jcrc.2011.04.002
31. Lake DE, Fairchild KD, Moorman JR. Complex signals bioinformatics: evaluation of heart rate characteristics monitoring as a novel risk marker for neonatal sepsis. J Clin Monit Comput (2014) 28(4):329–39. doi:10.1007/s10877-013-9530-x
32. Sullivan BA, Fairchild KD. Predictive monitoring for sepsis and necrotizing enterocolitis to prevent shock. Semin Fetal Neonatal Med (2015) 20(4):255–61. doi:10.1016/j.siny.2015.03.006
33. Oh S, Cha J, Ji M, Kang H, Kim S, Heo E, et al. Architecture design of healthcare software-as-a-service platform for cloud-based clinical decision support service. Healthc Inform Res (2015) 21(2):102–10. doi:10.4258/hir.2015.21.2.102
34. Van Eaton EG, Devlin AB, Devine EB, Flum DR, Tarczy-Hornoch P. Achieving and sustaining automated health data linkages for learning systems: barriers and solutions. EGEMS (Wash DC) (2014) 2(2):1069. doi:10.13063/2327-9214.1069
35. Lee H. Paging Dr. Watson: IBM’s Watson supercomputer now being used in healthcare. J AHIMA (2014) 85(5):44–7; quiz 48.
36. Dixon BE, Simonaitis L, Goldberg HS, Paterno MD, Schaeffer M, Hongsermeier T, et al. A pilot study of distributed knowledge management and clinical decision support in the cloud. Artif Intell Med (2013) 59(1):45–53. doi:10.1016/j.artmed.2013.03.004
Keywords: monitoring, labor, microbiome, fetus, neonate, big data integration, electronic medical record, perinatal care
Citation: Frasch MG (2015) Editorial: Perinatology in the era of big data and nanoparticles. Front. Pediatr. 3:95. doi: 10.3389/fped.2015.00095
Received: 01 September 2015; Accepted: 22 October 2015;
Published: 05 November 2015
Edited by:
Timothy James Moss, Monash University, AustraliaReviewed by:
Charles Christoph Roehr, Charité Universitätsmedizin Berlin, GermanyCopyright: © 2015 Frasch. This is an open-access article distributed under the terms of the Creative Commons Attribution License (CC BY). The use, distribution or reproduction in other forums is permitted, provided the original author(s) or licensor are credited and that the original publication in this journal is cited, in accordance with accepted academic practice. No use, distribution or reproduction is permitted which does not comply with these terms.
*Correspondence: Martin G. Frasch, bWZyYXNjaEB1dy5lZHU=
Disclaimer: All claims expressed in this article are solely those of the authors and do not necessarily represent those of their affiliated organizations, or those of the publisher, the editors and the reviewers. Any product that may be evaluated in this article or claim that may be made by its manufacturer is not guaranteed or endorsed by the publisher.
Research integrity at Frontiers
Learn more about the work of our research integrity team to safeguard the quality of each article we publish.