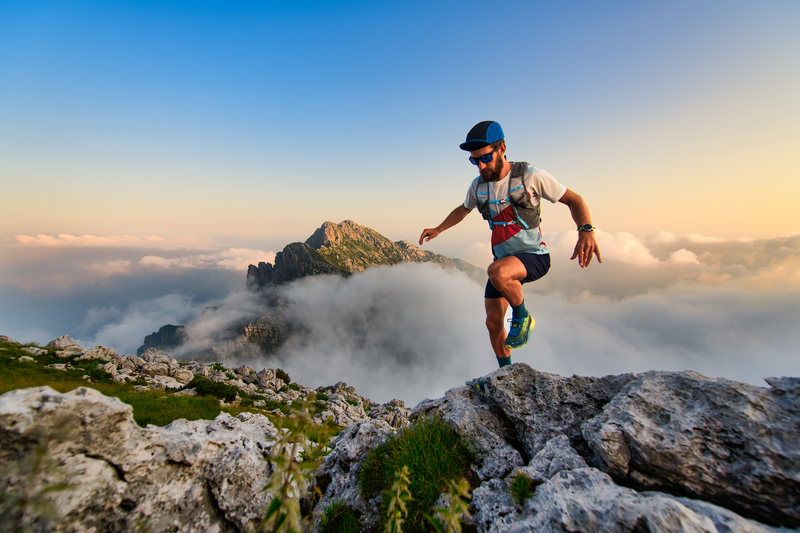
94% of researchers rate our articles as excellent or good
Learn more about the work of our research integrity team to safeguard the quality of each article we publish.
Find out more
ORIGINAL RESEARCH article
Front. Pain Res. , 18 January 2024
Sec. Musculoskeletal Pain
Volume 5 - 2024 | https://doi.org/10.3389/fpain.2024.1288024
Objectives: This explorative study analyses interrelationships between peripheral compounds in saliva, plasma, and muscles together with body composition variables in healthy subjects and in fibromyalgia patients (FM). There is a need to better understand the extent cytokines and chemokines are associated with body composition and which cytokines and chemokines differentiate FM from healthy controls.
Methods: Here, 32 female FM patients and 30 age-matched female healthy controls underwent a clinical examination that included blood sample, saliva samples, and pain threshold tests. In addition, the subjects completed a health questionnaire. From these blood and saliva samples, a panel of 68 mainly cytokines and chemokines were determined. Microdialysis of trapezius and erector spinae muscles, phosphorus-31 magnetic resonance spectroscopy of erector spinae muscle, and whole-body magnetic resonance imaging for determination of body composition (BC)—i.e., muscle volume, fat content and infiltration—were also performed.
Results: After standardizing BC measurements to remove the confounding effect of Body Mass Index, fat infiltration and content are generally increased, and fat-free muscle volume is decreased in FM. Mainly saliva proteins differentiated FM from controls. When including all investigated compounds and BC variables, fat infiltration and content variables were most important, followed by muscle compounds and cytokines and chemokines from saliva and plasma. Various plasma proteins correlated positively with pain intensity in FM and negatively with pain thresholds in all subjects taken together. A mix of increased plasma cytokines and chemokines correlated with an index covering fat infiltration and content in different tissues. When muscle compounds were included in the analysis, several of these were identified as the most important regressors, although many plasma and saliva proteins remained significant.
Discussion: Peripheral factors were important for group differentiation between FM and controls. In saliva (but not plasma), cytokines and chemokines were significantly associated with group membership as saliva compounds were increased in FM. The importance of peripheral factors for group differentiation increased when muscle compounds and body composition variables were also included. Plasma proteins were important for pain intensity and sensitivity. Cytokines and chemokines mainly from plasma were also significantly and positively associated with a fat infiltration and content index.
Conclusion: Our findings of associations between cytokines and chemokines and fat infiltration and content in different tissues confirm that inflammation and immune factors are secreted from adipose tissue. FM is clearly characterized by complex interactions between peripheral tissues and the peripheral and central nervous systems, including nociceptive, immune, and neuroendocrine processes.
Fibromyalgia (FM), which has a prevalence of 1%–4% in the population and a female predominance, is a complex chronic pain condition characterized by generalized widespread pain and hyperalgesia/allodynia (1–4). Psychological distress, fatigue, cognitive problems, inflammatory bowel disease, and insomnia are frequent symptoms and comorbidities. Modern management of chronic pain conditions rely on a biopsychosocial approach. However, mechanism-based diagnoses are lacking in the chronic pain field. Interventions that do not target causal mechanisms (e.g., molecular mechanisms) but modulate symptoms and risk factors are associated with high numbers needed to treat (NNT) (5). The International Association for the Study of Pain (IASP) in 2017 added to the existing mechanistic pain descriptors, nociceptive pain and neuropathic pain, a new mechanistic descriptor—nociplastic pain; FM was considered as a nociplastic pain condition (6).
Internationally agreed central or peripheral biomarkers are lacking for the International Classification of Diseases (ICD) diagnosis of FM, which partially may be because no definite pathophysiology for FM has been established. Alterations in the central and peripheral nervous systems as well as peripheral tissues have been reported in FM (7–18); central alterations are clinically recognized. Investigating peripheral and systemic alterations are now given increased attention. Hence, signs of mitochondrial dysfunction from muscles of patients with FM have been reported [e.g., increased muscle interstitial lactate, pyruvate and/or glutamate levels (19–22), decreased muscle concentrations of adenosine triphosphate (ATP) or nucleoside triphosphates (NTP) and phosphocreatine (PCr) (19, 23–25)], and alterations in metabolism of N-acylethanolamines (26, 27), and high prevalence of small fibre pathology (18, 28). Moreover, altered gut microbiome composition has been reported in FM (29, 30). Systemic alterations have also been found e.g., significant alterations in lipid mediators (31, 32). Blood proteomic studies (generally proteins at nano- and micromolar levels) show significant signs of immunity and inflammation alterations, which may indicate systemic low-grade inflammation (28, 33–36). However, the roles of commonly investigated blood cytokines and chemokines (typically found at picomolar levels) are unclear. Three recent systematic reviews of FM are not in consensus about which cytokines are significantly altered, except for IL-6 (37–39).
There are several indications that widespread pain including FM, obesity and low-grade inflammation are interrelated. Hence, obesity is a risk factor for FM development and is prevalent in this pain condition (approx. 36%) (40, 41). Severe clinical presentation and worse outcomes in pharmacotherapy are found in FM patients with obesity (28, 42–44), but it is unclear whether obesity is a causal factor or a result of the condition. Several studies indicate that obesity has an inflammatory component and is associated with chronic low-grade inflammation in, for example, adipose tissue, skeletal muscle, liver, pancreas, intestine and brain (45). In obese subjects, visceral and abdominal fat tissues secrete, for example, cytokines, chemokines (e.g., TNF-α, IL-6, IL-18, MCP-1/CCL2, CCL19, HGF, CSF-1, and VEGF-A), osteopontin, interferon-gamma, adipokines and micro-RNAs that can exert functional and pathophysiological alterations in various tissues (46–49). However, the knowledge concerning such secretions in chronic widespread pain including FM is lacking and the number of cytokines and chemokines per study is generally limited. Moreover, their influence on the clinical presentation is not understood. Body Mass Index (BMI), traditionally used to indicate overweight and obesity, has several disadvantages compared to more quantitative organ specific variables indicating fat content and infiltration. Recently, we reported significantly increased fat infiltration and content in different organs in this FM cohort (28). As there is a strong link between adipose tissue and BMI (28), there is a need to standardize such body composition variables (i.e., removing the association to BMI) to increase the knowledge about fat infiltration and content in FM.
Thus, there is a knowledge gap concerning the relationship between body composition (abdominal adipose tissue volumes and fat infiltration as well as muscle volumes and fat infiltration) and cytokines, chemokines and growth factors in FM compared to healthy controls. This study is part of a major project investigating mainly peripheral and systemic alterations in FM. In addition to finding that this FM cohort had increased fat infiltration and content in different organs, we found that this FM cohort had muscle alterations (i.e., increased pyruvate levels and lowered levels of ATP and PCr) (19, 28). Data from these studies are included for a broad coverage of peripheral factors.
Thus, this explorative study has two main aims:
I. To study whether FM patients have different profiles regarding inflammatory proteins, metabolites, markers of muscle energetic system, fat infiltration and body composition.
II. To investigate whether there are significant associations between the specific profiles of biochemical compounds and clinical variables (pain intensity, pressure pain thresholds, psychological distress, and health).
This study includes 32 female FM patients and 30 age-matched female controls (CON). The recruitment process and sample size calculations have been presented elsewhere (19). Brief descriptions of inclusion and exclusion criteria as well as the clinical examinations are given below.
The Regional Ethical Review Board in Linköping (Dnr: 2016/239-31) approved the study. All participants gave their written informed consent, and the study was performed in accordance with the Helsinki Declaration.
At the first visit, the subjects underwent a clinical examination that included collecting blood and saliva samples, registrations of pain thresholds. In addition, the subjects completed a health questionnaire. Microdialysis of the interstitium of the trapezius and erector spinae muscles, phosphorus-31 magnetic resonance spectroscopy (P-31 MRS) of the erector spinae muscle, and whole-body magnetic resonance imaging were performed during other visits.
The questionnaire captured age (years) and aspects of pain, psychological distress, and health. Pain aspects were determined using a numeric rating scale (0 = no pain and 10 = worst possible pain) and the global pain intensity for the previous seven days was indicated (NRS-7d). The duration of FM (years) was also reported. Psychological distress was determined using the Hospital Anxiety and Depression Scale (HADS) (0–42) (50–52). A lower score indicates fewer psychological distress symptoms.
Health aspects were determined using the European Quality of Life Instrument (EQ-5D). The ED-D has two parts. One captures a patient's perceived state of health, and one is a self-estimation of today's health on a 100-point scale (EQ5D-VAS) where low values indicate low perceived health (53, 54). This study uses the latter.
As the clinical examination used has been described in detail elsewhere, here we provide a brief description (19). Both patients and controls underwent a brief clinical examination of heart and lungs, which included recording diastolic and systolic blood pressure (mm Hg) after 2 min of rest in the horizontal position. In addition, their weight (kg) and height (m) were recorded. Body Mass Index (BMI) (kg/m2) was calculated. The clinical examination also ensured that the controls were healthy with respect to anamnesis for rheumatic diseases, neurological diseases, diabetes, cardio-vascular diseases, psychiatric diseases, and high alcohol consumption. The clinical examination of the patients ensured that they met the American College of Rheumatology (ACR) 1990 criteria (1), although newer FM criteria (2010/2011 and 2016) have been presented (55–57). However, we wanted to compare our study with earlier studies, so we chose the 1990 ACR criteria. Both in the FM and in the CON the number of tender points was registered as an indicator of hyperalgesia/allodynia.
The measurements of pressure pain thresholds (PPT) have been described in detail elsewhere (58, 59). PPT were determined using a manual pressure algometer (contact area of 1 cm2; pressure was increased by 30 kPa/s) (Somedic AB, Sweden). The erector spinae, tibialis anterior, and trapezius were investigated bilaterally. The subject-perceived pain was indicated by pushing a stop button or until the maximum threshold of 600 kPa was reached. At each site, two measurements were made with a minimum interval of 30 s. The mean of the six anatomical locations (PPT-tot) is reported as a global measure of pain sensitivity (i.e., low values indicate hyperalgesia).
Subjects were instructed not to smoke, drink caffeine, eat, or brush their teeth 1 h before sampling and to rest, preferably lying down, 30 min before each sampling. Saliva samples were collected using Salivette tubes (Sarstedt, Landskrona, Sweden). Samples were stored at 20 °C until they were assayed. Venous blood samples were collected in two 8-ml EDTA tubes. The samples were centrifuged at 1,000×g for 15 min, and the separate layers of plasma from the two blood samples, approximately 5–6 ml in total, were collected into a 12 ml Falcon-tube and mixed gently. The plasma was aliquoted into small portions and stored in −86 °C until analysis.
Analyses of inflammatory proteins (cytokines, chemokines, and growth factors) were performed directly in plasma and saliva using the multiplex immunoassay technology Meso Scale (MSD) (Maryland, USA). Using the manufacturer's protocols for MSD, we analysed up to 71 custom kit-panel inflammatory substances with the electro-chemo-luminescence method. Data were collected and analysed using MESO QUICKPLEX SQ 120 instrument equipped with DISCOVERY WORKBENCH® data analysis software. On the day of analysis, samples were thawed, and randomly mixed. An 8-points standard curve with known concentrations of each protein was performed in duplicate and samples were run in single analysis. The CV for the analytes together with the lower and upper limits of detection (LLOD-ULOD), expressed in pg/ml, for each substance is presented in Supplementary Table 1. Proteins not detected in at least 50% of the samples in either patients or healthy controls were excluded from the statistical analysis. Therefore 68 proteins were included in the data analysis.
Body composition was determined by quantitative analysis of magnetic resonance imaging (MRI). Details have been reported elsewhere (28, 60–64). In brief, subjects were scanned in a Philips Ingenia 3T MRI scanner (Philips Healthcare, Best, The Netherlands) using a 6-min dual-echo Dixon protocol, providing water and fat separated volumetric data covering a region from the neck to the knees (60).
The body composition profiling was performed using AMRA® Researcher (AMRA Medical AB, Linköping, Sweden) (65). The following variables were obtained for this study: visceral adipose tissue volume [VAT (L)]; abdominal subcutaneous adipose tissue volume [ASAT (L)]; liver fat by proton density fat-fraction [LF (%)]; total thigh fat-tissue free muscle volume (FFMV) of the thigh muscles [T-FFMV (L)]; and erector spinae [ES-FFMV (L)] muscles and mean muscle fat infiltration [MFI (%)] of the anterior thigh (T-MFI) and erector spinae (ES-MFI) muscles. These variables are not standardized (see below) and are summarized below as non-standardized body composition variables. The spinal erector group, a region between the top of first lumbar vertebrae (L1) and the bottom of the fifth lumbar vertebrae (L5), includes the iliocostalis, longissimus, spinalis, and the transversospinales as this small muscle group cannot be excluded from the spinal erector group given the spatial resolution of the image data.
The body composition measurements were standardized (i.e., not normalized) in the manner described below resulting in body composition standard scores (commonly referred to as z-scores) using individualized virtual control groups (VCG) obtained from a very large reference population. The procedure results in a standardization of a subject's body composition measurements by subtracting the reference VCG mean from the subject's measurement and then dividing the difference by the reference VCG standard deviation. As this VCG is based on sex and BMI matching to the target subject, the standardized body composition measurements (z-scores) following this operation are effectively no longer confounded by sex and BMI. Non-standardized body composition data are also presented as a comparison.
Using data from a sample of 40,178 participants of a normal reference population scanned in the UK Biobank imaging study (66, 67), we calculated standardized body composition variables (i.e., z-scores, which are summarized as the standardized body composition variables below). Specifically, for each subject, an ASAT z-score (z-ASAT), VAT z-score (z-VAT), LF z-score (z-LF), T-MFI z-score (z-T-MFI), and T-FFMV z-score (z-T-FFMV) were calculated using the distribution of matched VCG. To create a VCG, we matched the target study subject to participants in the UK Biobank with the same sex and within ±1 kg/m2 of their BMI (except for T-FFMV, which was within ±2 kg/m2). If fewer than 150 controls were stratified by these criteria, the BMI interval was incrementally and symmetrically increased by 0.1 kg/m2 until the VCG contained at least 150 subjects.
Each subject's body compartment z-scores were calculated as the number of standard deviations between the VCG mean and study participants' body compartments measurement. In other words, the z-score measures how much the study subject deviates for each specific body compartment—i.e., from what is expected by their sex and BMI. VAT, ASAT, and FFMV values were divided by height squared (h2) before calculation of the z-score. Because of the observed skewed distribution of liver fat, LF values were log transformed before calculating z-LF. MFI was used without any transformation (68–70). We label the variables from the whole-body magnetic resonance imaging body composition (BC) variables. Specifically, BMI will not be included in the multivariate analyses as it is not a formal measure of body composition.
This study also includes some data presented elsewhere obtained from microdialysis of the interstitium of trapezius and erector spinae muscles and from phosphorus-31 magnetic resonance spectroscopy (P-31 MRS) of the erector spinae muscle (19). Hence, from the microdialysis, we include concentrations of lactate, pyruvate, and glutamate immediately after the trauma period—i.e., at baseline (denoted as 140 min)—and the mean value of the time points from baseline to the end of the recovery (140–220 min). From P-31 MRS, the concentrations of nucleotide triphosphate (NTP, mainly composed of ATP), phosphocreatine (PCr), and inorganic phosphate (Pi) together with tissue pH (derived from the P-31 MRS measurements) were included in this study.
The statistics were performed using the statistical packages IBM SPSS Statistics (version 27.0; IBM Corporation, Route 100 Somers, New York, USA) and SIMCA-P+ (version 17.0; Sartorius Stedim Biotech, Umeå, Sweden). A P-value of <0.05 was considered statistically significant. Text and tables report the mean value ± one standard deviation (±1 SD) of continuous variables, and percentages (%) are reported for categorical variables. To compare groups, we used Student's t-test for un-paired observations and Chi square test for proportions.
Previous studies have discussed the necessity of using advanced multivariate analyses (MVDA) when accounting for system-wide aspects, including missing data and multicollinearity problems (71, 72). Using SIMCA-P+, we applied advanced Principal Component Analysis (PCA) to determine multivariate outliers and multivariate correlation patterns. Outliers were identified using two methods: score plots in combination with Hotelling's T2 and distance to model in X-space. PCA extracts and displays systematic variation in the data matrix (i.e., a variant of multivariate correlation analysis). A cross validation technique was used to identify nontrivial components [p]. Variables loading on the same component [p] were correlated, and variables with high loadings but opposing signs were negatively correlated. Variables with high absolute loadings were considered significant. Per definition, the obtained components are not correlated and are arranged in decreasing order with respect to explained variation. Goodness of fit (R2) is the fraction of sum of squares of all the variables explained by a principal component (73). Q2 describes the goodness of prediction—the fraction of the total variation of the variables that can be predicted using principal component cross validation methods (73).
To determine group associations, we used Orthogonal Partial Least Square Regressions discriminant analysis (OPLS-DA). Orthogonal Partial Least Square Regressions (OPLS) was used for the multivariate regression analyses of the indices indicating fat infiltration and content and fat free muscle volume. SIMCA-P+ uses the Non-linear Iterative Partial Least Squares (NIPALS) algorithm to handle missing data: max 50% missing data for variables/scales and max 50% missing data for subjects. The Variable Influence on Projection (VIP) indicates the relevance of each X-variable pooled over all dimensions and Y-variables—i.e., the group of variables that best explains Y (73). VIP >1.0 (or VIPpred if more than one component is identified) was considered significant if VIP had 95% jack-knife uncertainty confidence interval non-equal to zero. P(corr) was used to note the direction of the relationship (positive or negative)—i.e., the loading of each variable was scaled as a correlation coefficient and therefore standardized the range from −1 to +1 (71). P(corr) is typically stable during iterative variable selection and comparable between models. Thus, a variable/regressor was considered statistically significant when VIP or VIPpred >1.0. If certain predefined internal criteria of SIMCA-P are fulfilled, a regression model will be obtained, including one or several components (the first is always the predictive component). The validity of the model is then determined using cross validation.
Hence, for each regression, we report R2, Q2, and the P-value of a cross-validated analysis of variance (CV-ANOVA). When more than 50 regressors were included, the OPLS analysis was made in two steps. In the first step, all relevant compounds were included in the analysis. In the second step, the compounds with VIP or VIPpred >1.5 were used in a new OPLS-DA or OPLS provided that the first analysis resulted in one or several significant components according to the internal rules used in SIMCA-P+ (73). The results of the second (final) regression are presented in text and tables including R2, Q2, and P-value of CV-ANOVA. The parameters of the initial regression are given in Supplementary Table 2.
Data obtained from the questionnaires, the clinical examinations, and the body composition (BC) variables (except the standardized variables) for healthy controls (CON) and in FM patients have been presented elsewhere (Table 1) (19, 28). FM had lower PPT and reported lower perceived health. The FM group reported medium pain intensity on the group level. As previously reported, pyruvate levels in trapezius and erector spinae were significantly higher, but NTP and PCr of erector spinae were significantly lower in FM (Table 1) (19). We recently reported that BMI and all non-standardized fat infiltration and content variables as well as T-FFMV differed significantly between the two groups (Table 1) (28). The group differences were more pronounced for the non-standardized BC variables than for the standardized (Table 1). Following standardization (i.e., correcting for BMI differences), all standardized BC variables except z-LF remained significantly different between the two groups—i.e., the differences in these BC variables between groups cannot be explained by the group difference in BMI.
Table 1. Data obtained from questionnaires, the clinical examinations, the body composition variables, and variables from muscle microdialysis and spectroscopy in controls (CON) and in fibromyalgia patients (FM) [mean and standard deviation (SD)].
The interrelationships between all BC variables were investigated, resulting in a PCA model with two significant components: R2(cumulative) = 0.59 and Q2(cumulative) = 0.20. One patient (no. 16) was a multivariate outlier and therefore omitted from the analyses. Also, in the final analysis, two significant components were obtained (Supplementary Table 3). The first and thereby the most important component p[1] was dominated by body fat content and muscle infiltration variables: VAT, T-MFI, ASAT, ES-MFI, z-VAT and z-T-MFI. The second component p[2] mainly reflected the intercorrelations between the three fat free muscle volume variables: T-FFMV, ES-FFMV and z-T-FFMV.
We also performed separate PCA analyses for the non-standardized and the standardized BC variables. No significant model was achieved for the PCA of the standardized BC variables. For the non-standardized body composition variables, a significant PCA with two components was obtained: R2(cumulative) = 0.70 and Q2(cumulative) = 0.28. Fat infiltration and content variables—which were all positively intercorrelated—mainly determined the first component and the fat free muscle volume variables were important for the second component (Supplementary Table 4). The scores of the first component were defined as a fat infiltration and content index (FIC-index), and the scores of the second component were labelled Muscle-index. These two indices are per definition un-correlated. Significant group differences were noted for both indices: FIC-index: CON = −0.91 ± 1.01 vs. FM = 0.85 ± 2.02, P < 0.001; Muscle-index: CON = 0.46 ± 0.85 vs. FM = −0.43 ± 1.43, P = 0.005. Thus, the higher FIC scores in FM than in CON indicate more adipose tissue, whereas higher Muscle-index scores indicate larger fat-free muscle volume in CON than in FM.
To understand the relative importance of the BC variables in relation to group membership, we performed an OPLS-DA using the non-standardized and the standardized BC variables as regressors. For both regressions, significant models of group membership were obtained. Hence, both these regressions included intramuscular fat infiltration of the thigh and visceral adipose tissue volume as significant regressors. However, in the regression using the standardized BC variables, the fat free muscle volume of the thigh was the most important significant variable.
Specifically, the significant OPLS regression using the non-standardized BC variables as regressors (R2 = 0.40, Q2 = 0.33, CV-ANOVA P-value = 0.00014, one predictive and one orthogonal component) identified the following variables as significant: T-MFI [VIP = 1.32, p(corr) = 0.80], VAT [VIP = 1.28, p(corr) = 0.77], and ASAT [VIP = 1.10, p(corr) = 0.67]. The corresponding significant analysis using the standardized BC variables (R2 = 0.26, Q2 = 0.20, CV-ANOVA P-value = 0.0017, one predictive component) identified the following variables as significant: z-T-FFMV [VIP = 1.29, p(corr) = −0.86], z-T-MFI [VIP = 1.07, p(corr) = 0.71], and z-VAT [VIP = 1.03, p(corr) = 0.69].
The first regression included all compounds from saliva, plasma, and muscles as regressors (X variables). Certain saliva proteins and one microdialysis variable were particularly important significant regressors as shown in Table 2 (R2 = 0.36, Q2 = 0.22, CV-ANOVA P-value = 0.0165, one predictive component and one orthogonal component). The most significant saliva proteins—MIP-1α/CCl3, I-TAC/CXCL11, MCP-4/CCL13, MIP-3β/CCL19, and IL-2—showed positive associations with the group and therefore were increased in FM (Table 2). As expected from this regression, after including only saliva proteins as regressors, a significant model was also obtained. The significant proteins (R2 = 0.18, Q2 = 0.14, CV-ANOVA P-value = 0.019, one predictive component) are shown in Supplementary Table 5. The most important significant saliva proteins were identical to those presented in Table 2. As it was not possible to significantly differentiate the two groups of subjects using only plasma proteins as regressors, a combination with other data was required.
Table 2. OPLS-DA of group membership (FM denoted 1 and CON denoted 0) using compounds from saliva, plasma, and muscles as regressors. Variables with VIP > 1.5 are shown.
Using the muscle compounds obtained from microdialysis and MR spectroscopy when regressing group membership also resulted in a significant model (R2 = 0.28, Q2 = 0.16, CV-ANOVA P-value = 0.009; one predictive component). The following significant regressors were identified: Pyruvate Trapezius 140 min [VIP = 1.64, p(corr) = 0.75], Pyruvate Trapezius 140–220 min [VIP = 1.62, p(corr) = 0.75], PCr [VIP = 1.56, p(corr) = −0.72], NTP [VIP = 1.48, p(corr) = −0.69], Pyruvate Erector spinae 140–220 min [VIP = 1.11, p(corr) = 0.50], and Pi [VIP = 1.11, p(corr) = −0.52].
In this step, we regressed group membership using the BC variables and all molecular compounds. The most important significant variables are shown in Table 3 (R2 = 0.46, Q2 = 0.43, CV-ANOVA P-value = 1.25 × 10−7). Several of the BC variables were significant in this regression together with five compounds from muscles, three from plasma (IL-2Ra, IL-1-Ra and MDC/CCL22), and four proteins from saliva (IFN-α2α, MIP-1/CCL3, IL-27, and TPO/THPO).
Table 3. OPLS-DA of group membership (FM denoted 1 and CON denoted 0) using all body composition (BC) variables (left columns), only non-standardized BC variables (middle columns) and only standardized BC variables (right columns) together with all biochemical variables in saliva, plasma, and muscles as regressors. Variables with VIP > 1.5 are shown.
Very similar results with respect to the significant biochemical variables from muscles, saliva and plasma were obtained when the regressions included either the non-standardized BC variables or the standardized BC variables (Table 3). In the regression including the non-standardized BC variables VAT, ASAT, and muscle, pyruvate levels of the trapezius were the strongest significant regressors, and in the regression including the standardized BC variables z-T-FFMV and the muscle, pyruvate levels of the trapezius were the most important significant regressors (Table 3).
In Table 4 is given a summary of the significant biochemical regressors of pain intensity, PPT, FIC-index, z-VAT and z-T-FFMV; details are reported in the Supplementary Tables 6–10.
Table 4. An overview of the significant biochemical regressors (marked with X) for the OPLS regressions of pain intensity (NRS), pressure pain thresholds (PPT), FIC-index, z-VAT and z-T-FFMV. For details of these regressions see Supplementary Material i.e., NRS: Supplementary Table 6, PPT: Supplementary Table 7, FIC-index: Supplementary Table 8, z-VAT: Supplementary Table 9 and z-T-FFMV: Supplementary Table 10.
As the controls were determined to be pain-free, pain intensity was regressed only for the FM group. The plasma proteins MCP-1/CCL2, MIP-1β/CCL4, TSLP, and IL-1RA and four IL-17 interleukins (B, C, D and F) were the strongest significant regressors and all correlated positively with pain intensity (R2 = 0.63, Q2 = 0.36, CV-ANOVA P-value = 0.033, one predictive component and one orthogonal component) (Supplementary Table 6). Plasma proteins dominated among the significant proteins (Supplementary Table 6).
The strongest significant regressors of PPT (R2 = 0.33, Q2 = 0.29, CV-ANOVA P-value = 7.45 × 10−5, one predictive component) are shown in Table 4 and in Supplementary Table 7, left part). A mix of muscle and plasma compounds were significant. Since plasma proteins were relatively important in this model, it was possible to regress PPT using only plasma proteins as regressors. This significant model is shown in Table 4 and in Supplementary Table 7, right part) (R2 = 0.30, Q2 = 0.19, CV-ANOVA P-value = 0.006, one predictive component). Low PPT (i.e., hyperalgesia) was associated with high levels of plasma proteins. MDC/CCL22, IL-2Ra, MIF, IL-16, and MCSF/CSF-1 showed the strongest significant correlations with PPT. It was not possible to regress PPT in the two groups separately using compounds from saliva, plasma, and/or muscles.
It was not possible to achieve stable significant regressions of HAD-tot or EQ-VAS in all subjects together or in each of the two subgroups using compounds from saliva, plasma, and muscles.
The most significant compounds from plasma, saliva, and/or muscles when regressing FIC-index—i.e., the fat infiltration and content index obtained from the PCA of the non-standardized BC variables (see above) (R2 = 0.41, Q2 = 0.31, CV-ANOVA P-value = 2.75 × 10−5; one predictive component—are shown in Table 4 and in Supplementary Table 8, left part). Plasma proteins dominated as significant regressors (Table 4 and Supplementary Table 8, left part).
Also, the regression of FIC-index using only plasma proteins as regressors was significant (Supplementary Table 8, right part) (R2 = 0.36, Q2 = 0.28, CV-ANOVA P-value <0.001; one predictive component). The plasma proteins that were strongest (and positively) associated with FIC-index were M-CSF, MDC, IL-1RA, MIP-1α and IL-2Ra (Supplementary Table 8, right part).
It was not possible to significantly regress FIC-index only using saliva proteins.
The relationship between FIC-index and only the muscle compounds was also analysed (Table 4). The significant OPLS regression (R2 = 0.52, Q2 = 0.31, CV-ANOVA P-value = 9.83 × 10−5, one predictive component and one orthogonal component) identified the following compounds as significant: NTP [VIP=1.76, p(corr) = −0.62]; PCr [VIP = 1.68, p(corr) = −0.58]; Pyruvate Trapezius 140 min [VIP = 1.54, p(corr) = 0.53]; Pyruvate Trapezius 140–220 min [VIP = 1.50; p(corr) = 0.50]; Pyruvate Erector spinae 140–220 min [VIP = 1.38, p(corr) = 0.47]; and muscle pH [VIP = 1.23, p(corr) = −0.44].
It was not possible to obtain a significant regression of Muscle-index using compounds from plasma, saliva, and muscles in all subjects.
No significant models of FIC-index or Muscle-index were obtained from FM or CON when using all compounds as regressors.
As reported above, unlike the non-standardized BC variables, it was not possible to achieve a significant PCA of the standardized BC variables and determine indices. Therefore, we first regressed the four standardized fat infiltration and content variables simultaneously (i.e., four Y variables simultaneously—z-VAT, z-ASAT, z-LF and z-T-MFI). According to CV-ANOVA, this regression indicated that only z-ASAT had significant associations with the compounds. However, it was not possible to significantly regress z-VAT in all subjects taken together or in FM. The regression for CON was significant. The significant compounds—a mix of saliva and plasma proteins—are shown in Table 4 and in Supplementary Table 9 (R2 = 0.76, Q2 = 0.64, CV-ANOVA P-value = 7.26 × 10−6; one predictive component). Four saliva proteins (IL-6, IL-15, VEGF-A and MIP-5/CCL15) and the plasma protein TRAIL were most strongly correlated with z-VAT.
Secondly, we regressed the fifth standardized variable (i.e., z-T-FFMV). The regression of the most important substances was significant (R2 = 0.45, Q2 = 0.31, CV-ANOVA P-value = 1.84 × 10−5; one predictive component) (Table 4 and Supplementary Table 10). The strongest significant predictors were NTP, PCr, P-IL-7, pyruvate 140 min-Tr, and P-IL-2Ra. No significant regressions were obtained in the two groups of subjects separately.
In this explorative study we investigated whether FM patients have different profiles regarding inflammatory proteins, metabolites, markers of muscle energetic system, fat infiltration and body composition. Moreover, we explored whether there were significant associations between the specific profiles of biochemical compounds and clinical variables.
After standardizing the body composition measurements to remove the cofounding effect of differences in BMI, we found that fat infiltration and content generally were increased, and fat-free muscle volume of the thigh decreased in FM. The latter variable correlates strongest with intramuscular compounds and with two plasma proteins. In other words, there are differences in fat infiltration and content that cannot be explained by differences in BMI.
Saliva cytokines and chemokines contributed to the significant differentiation between FM and CON, and saliva compounds were increased in FM. When BC variables and muscle compounds were included, a range of variables were significantly associated with FM or CON; fat infiltration and content BC variables together with fat free muscle volume (z-T-FFMV) were most important.
Plasma chemokines and cytokines were significantly and positively associated with pain intensity in FM, and negatively with PPT for all subjects taken together. Several of these compounds were also important for the group differentiation and FIC-index. Mainly plasma cytokines and chemokines were significantly and positively associated with the FIC-index in the total sample.
Our study agrees with two other studies using targeted panels of inflammation-related cytokines and chemokines in FM (74, 75). These three studies together indicate the presence of peripheral low-grade systemic inflammation in FM. In the two earlier studies, plasma samples were analysed (74, 75) using a panel from OLINK Bioscience (Uppsala, Sweden), and the present study used a panel from Meso Scale Discovery (MSD; Maryland, USA). The two panels only partially cover the same proteins. Several of the important plasma proteins found in Bäckryd et al. and Gerdle et al. were identified in saliva in the present study (Table 2)—e.g., MIP-1α/CCL3, MCP-4/CCL13, MIP-3α/CCL20, and GRO-alpha/CXCL1 (74, 75). The findings in these studies and our study partially contradict findings identified in three recent systematic reviews (SR), which are only partially consistent with each other (37–39). One SR reported higher blood levels of IL-6, IL-17A, and IL-4 in FM (37). Another SR reported higher IL-6, IL-8, TNF-α and chemokine eotaxin (CCL11, CCL24, and CCL26) levels and decreased IL-10 in FM (38). The third SR reported lower levels of IL-1β and increased levels of IL-6, IL-8, TNF-α, Interferon-γ, CRP and BDNF (39). The latter SR concluded that there was significant heterogeneity between studies. Several of their identified proteins were found in the present regression (i.e., IL-17A, CCL24 and IL-10) although the latter was increased in this FM cohort (Table 2). These SRs are mainly based on studies investigating a few substances at a time, whereas the present study and Bäckryd et al.'s and Gerdle et al.'s studies are exploratory and based on many cytokines and chemokines in the same arrays (74, 75). Also, explorative proteomic studies of plasma and serum report significant systemic immunity and inflammation alterations in FM compared to healthy controls (33–36).
Specifically, a mix of cytokines and chemokines were important for the differentiation between FM and CON (Table 2). Most of the cytokines were proinflammatory, but there were also increased levels of cytokines generally considered as anti-inflammatory in FM (e.g., IL-10, IL-13, and IL-27). This may reflect mechanisms trying to suppress inflammation in a delicate balance with pro-inflammatory responses. Some cytokines—e.g., IL-27 and IL-10—can exhibit both pro-inflammatory and anti-inflammatory properties depending on the micro-environment (76–78). It may be relevant to briefly relate the five most important saliva proteins (MIP-1α/CCL3, I-TAC/CXCL11, MCP-4/CCL13, MIP-3β/CCL19 and IL-2) to studies concerning nociception and pain. The chemokine MIP-1α/CCL3 has inflammatory properties (79), and elevated blood levels have been reported in chronic widespread pain (mainly FM), lumbar intervertebral disk degeneration, migraine, and prostatitis pelvic pain (75, 80–82). I-TAC/CXCL11 is a proinflammatory chemokine and increased blood levels have been found in FM and in endometriosis patients (79, 83, 84). The functions of MCP-4/CCL13 include secretion of proinflammatory cytokines (85); increased serum levels of MCP-4/CCL13 were found in patients with knee osteoarthritis (OA) and rheumatoid arthritis (RA) (86). MIP-3β/CCL19 both promotes inflammation processes and has a homeostatic function (79, 87); a fourfold increase was found in FM (74). In patients with joint injury and OA, the levels correlated with clinical severity (88). IL-2 is a cytokine that can display both pro- and anti-inflammatory properties (89–92). Increased blood levels of IL-2 have been found in FM according to some studies although some studies have not found altered blood levels of IL-2 (38, 39).
Hence, the available literature including the present study applying targeted (broad panels of cytokines/chemokines) and nontargeted (proteomics) analyses indicate that FM is associated with peripheral low-grade inflammation and immune alterations, and the mechanisms involved cannot be limited to processes in the nociceptive systems. The circulatory system, mouth, and saliva have important roles for immune system host defence mechanisms and tissue homeostasis (93, 94). Nociceptors have bidirectional neuro-immune and neural-vascular interactions (95). At peripheral and central levels, bidirectional signalling pathways exist between the immune and nervous systems (96–101). Top-down mechanisms enable the CNS to influence peripheral inflammatory activity via neuroendocrine and autonomic mechanisms (100, 102). Down-top information on peripheral inflammatory activity is transmitted via peripheral nociceptors and humoral and neuronal pathways to the CNS, which may lead to decreased endogenous pain inhibition, neuroinflammation and sickness behaviour (99, 101, 103–105). In FM/chronic widespread pain, signs of neuroinflammation in CNS have been found (74, 106). During neuroinflammation, the blood-brain barrier endothelium undergoes changes, including increased expression of proinflammatory cytokines and chemokines (107). Blood-brain barrier permeability can alter due to influences from cytokines and complement factors, which facilitates this communication to the CNS (108–110). The importance of recognizing immune alterations for the development and clinical picture of FM is emphasised by results that suggest immune molecules (IgG) from FM patients can transfer fibromyalgia symptoms to mice (111). A longitudinal study of low back pain and temporomandibular disorders in the acute and chronic stages indicate that up-regulation of inflammatory responses in the acute stage protects against chronic development (112). Hence, increased knowledge about the trajectories of the immune system in FM is desirable.
We cannot fully explain why compounds from saliva were more important than compounds from plasma for group differentiation (Table 2). Human saliva is attracting increasing research interest since it reflects local and systemic physiological and pathophysiological conditions together with the low costs for sampling and the non-invasive methodology (113, 114). It is already utilized for diagnostic purposes in certain areas e.g., differential diagnosis of Cushing's syndrome, quantification of 25-hydroxy vitamin D, detection of drug and alcohol abuse (113, 115). Proteomic studies indicate that saliva proteins can differentiate between FM and controls (116, 117). A study of neuropathic pain patients with healthy controls showed that saliva proteins were slightly more important than plasma proteins for the group differentiation (118). Saliva exchanges substances with blood through active carriage, passive diffusion, or facilitated diffusion across the cell membrane (119). Saliva has been characterized as functionally equivalent to serum (120). The salivary glands are regulated by the autonomic nervous system (121). As saliva might partially also reflect central alterations (122, 123), saliva has been used to identify biomarkers of neurodegenerative diseases (124). However, the ability for saliva cytokines and chemokines to differentiate FM from controls needs to be confirmed in other studies.
For the present subjects, we report that both standardized and non-standardized BC can predict group membership. Adding these variables as regressors of group membership increased the explained variation (Table 3). One possibility is that the significance of the BC variables just reflects that patients with FM have more adipose tissue and less fat free muscle volume, without any causal explanation. However, the literature as well as our regression of FIC-index (Table 4 and Supplementary Table 8) show that adipose tissues are indeed active tissues secreting proteins actively involved in immunological and inflammatory processes (46–48). Moreover, most of the identified compounds that correlated with FIC-index have previously been reported as important variables for discriminating FM with obesity from non-obese FM patients (see Supplementary Table 8) (44). Plasma proteomic studies investigating the associations with BMI both in FM/chronic widespread pain and in healthy controls provide further support for this hypothesis (125). Hence, the reasons for the importance of BC variables in the regression (Table 3) may include that they reflect uncovered inflammatory and immune processes. Combining targeted (panels of cytokines and chemokines) and untargeted (e.g., proteomics and metabolomics) methods may increase the knowledge about involved molecular mechanisms.
The significant regression of pain intensity in FM (Table 4 and Supplementary Table 6) is consistent with plasma and muscle proteomic studies, which have shown significant relationships between proteins and pain intensity in FM and CWP (mainly FM) (35, 125, 126). The plasma proteins MCP-1/CCL2, MIP-1β/CCL4, TSLP, IL-1Ra and four IL-17 interleukins (B, C, D and F) showed the strongest and positive associations with pain intensity (Table 4 and Supplementary Table 6).
Specifically, the chemokine MCP-1/CCL2 is considered proinflammatory (79), but may also have anti-nociceptive functions (76). MCP-1/CCL2 correlated positively with pain intensity in FM (127) and was increased in FM and in chronic neck patients (79, 127–129), although a systematic review of neck pain did not find significant alterations (130). MIP-1β/CCL4, a proinflammatory chemokine (79), correlated positively with pain intensity in women with work-related neck and/or shoulder pain (131). It is upregulated in several CNS disorders, which may indicate a key role in the neuroinflammatory process (132, 133). TSLP has been primarily linked to itching rather than pain behaviour (134). However, serum levels were higher in stage III/IV endometriosis than in controls (135). IL-1Ra inhibits the activity of IL-1 and therefore has anti-inflammatory properties (136), and serum level was significantly increased in psoriatic arthritis and the levels were associated with joint severity (137). Several members (B, C, D, E/IL-25, and F) of the IL-17 family were important for pain intensity (Table 4 and Supplementary Table 6). IL-17A is the prototypical member of the IL-17 family; the other members are less investigated (138). IL-17 is known for its pro-inflammatory role in autoimmune diseases (138). IL-17 plays a significant role in chronic pain initiation and progression (139), although it also has protective functions (138).
The most important plasma proteins negatively correlated with PPT were MDC/CCL22, IL-2Ra, MIF, IL-16, and M-CSF/CSF-1 (Table 4 and Supplementary Table 7, right part). Thus, high concentrations of these proteins were associated with hyperalgesia for pressure. MDC/CCL22 and IL-2Ra were also important regressors of group and pain intensity. MIF is mainly a pro-inflammatory cytokine (140), but it also can have a protective role (140). Recently, we reported that MIF from plasma is increased in obese FM patients compared to non-obese FM patients (44). Elevated serum level has been found in chronic back pain (141). IL-16 plays a crucial role in the inflammatory process as it acts as a chemoattractant for peripheral immune cells and has been linked to inflammatory diseases such as asthma, Crohn's disease, and RA (142). Elevated blood level has been found in acute myocardial infarction and in chronic back pain (141, 142). M-CSF/CSF-1 has proinflammatory and homeostatic functions (143); high circulating levels has been found in patients with cancer, inflammation, and autoimmune disorders (144).
We found no significant correlations between the peripheral compounds and HAD-tot or EQ-VAS in all subjects together or in each of the two subgroups. Plasma proteomic studies of FM patients have found protein patterns correlating with psychological distress aspects (35, 125). Also, studies using targeted panels of proteins have reported significant corelations between plasma protein patterns and anxiety and depression in a mixed group of chronic pain patients (145). We are not aware of studies investigating the relationships with health. Hence, we have not been able to confirm earlier studies and the reasons for this are unclear.
Plasma proteins alone could significantly predict FIC-index in all subjects taken together. A mix of increased plasma proteins—i.e., the five most significant were M-CSF/CSF-1, MDC/CCL22, IL-1RA, MIP-1α/CCL3, and IL-2RA—were associated with FIC-index (Table 4 and Supplementary Table 8, right part). These positive associations are consistent with obesity studies reporting activated peripheral immune and inflammation processes (45, 46, 146). For example, VAT and SAT secrete cytokines and chemokines as well as several compounds also found here (Supplementary Table 8): MIP-1α/CCL3, MCP1/CCl2, I-309/CCL1, and IL-18 (46).
The two most important chemokines were MDC/CCL22 and MIP-1α/CCL3 (Supplementary Table 8, right part). The pro-inflammatory chemokine MDC/CCL22 is increased in serum and in monocytes from FM patients, (83, 147); increased levels were also found in ischemic heart disease (148). MIP-1α/CCL3 was also important for group differentiation, IL-1-Ra was a significant regressor of pain intensity in FM, and M-CSF/CSF-1 was important for PPT (see above). IL-2Ra is a receptor for the pro-inflammatory cytokine IL-2, which belongs to the IL-1 family. The IL2-IL-2 receptor pathway is incompletely understood but may be involved in the balance between immunity and tolerance (149).
When muscle compounds were included in the analysis of FIC-index, the explained variation was higher (Supplementary Table 8, left part) than when only using plasma proteins as regressors. NTP and PCr (both negatively associated) and pyruvate levels in trapezius and erector spinae were the most important muscle compounds, although 20 plasma proteins and three saliva proteins were also important (Supplementary Table 8, left part). The causal relationships between the muscle compounds and fat infiltration and content are currently unknown. However, the relationship can potentially be related to physical deconditioning and altered dietary habits in FM.
When standardizing the BC variables to remove the confounding effect of BMI, the group difference in the fat compartment variables became slightly less significant, as expected given the strong link between adipose tissue and body weight and group difference in body weight. Nevertheless, there was still a difference that is beyond what can be explained by body size. That is, the FM group has more adipose tissues than expected in all tissues investigated except the liver. In addition, the standardized thigh muscle volume variable (z-T-FFMV) had a stronger group difference than before standardizing, so the FM group had smaller muscles than expected (Table 1).
For z-VAT in CON, we found significant associations with compounds from saliva, plasma, and muscle (Table 4 and Supplementary Table 9). Four saliva proteins (IL-6, IL-15, VEGF-A and MIP-5/CCL15) and the plasma protein TRAIL were positively and strongest associated with z-VAT (i.e., larger volume of visceral adipose tissue than expected given the subject's BMI). Hence, saliva proteins were more important in this regression, unlike the non-standardized BC variables as indicated by FIC-index (Table 4 and Supplementary Table 8). On the other hand, this regression only considered CON, whereas the regression of FIC-index included all subjects, and no subgroup analyses were significant. Brief descriptions about the most important significant proteins are given in Supplementary text #1.
The standardized fat free muscle volume of the thigh (z-T-FFMV) was significantly higher in CON than in FM. The substances that most strongly correlated with z-T-FFMV were NTP, PCr, P-IL-7, pyruvate 140 min-Trapezius, and P-IL-2Ra (Table 4 and Supplementary Table 10). Thus, a high z-T-FFMV was associated with high levels of NTP and PCr together with low pyruvate levels at baseline. IL-7 promoting, for example, muscle hypertrophy correlated positively, and IL-2Ra correlated negatively with z-T-FFMV (150). Both IL-7 and the receptor for IL-2 belong to the IL-2 family, which can promote either cell survival or cell death and modulate differentiation of cells into more terminally differentiated (151). In muscle tissue, the IL-2/IL-2 receptor complex via expansion of regulatory T cells (Treg) mitigate muscle inflammation in situations with muscle repair and regeneration (152). These and earlier results from our group clearly indicate a muscle involvement in FM (19–27). FM patients benefit from physical exercise with respect to important clinical outcomes (28). Future studies may investigate to what extent such improvements are found for z-T-FFMV and if such alterations are related to molecular muscle normalizations.
The cytokines and chemokines investigated mainly cover proteins at the picomolar levels. The complete understanding of the activated biological processes related to muscle fat infiltration and body fat content in subjects with and without chronic pain may require broader approaches with respect to concentration scales (i.e., from micro- to nano-levels) as well as specific tissue types. Traditional statistical analyses are not advisable with low ratios of subjects to variables (i.e., proteins) as many of the proteins likely are highly intercorrelated. Hence, we applied MVDA, which is a backbone in such situations (71, 153). Moreover, we use a state-of-the art method to remove the confounding effect of BMI for BC measurements. This novel ability to identify actual group differences is a strength of our method.
This study has some limitations, including the relatively small sample size and the cross-sectional design. Moreover, only women were included since FM has a strong female predominance (1–4). Future studies should also include men with FM and employ repeated concentration measurements of proteins. In addition, to understand the initiating factors prospective studies of healthy subjects and patients with acute and local pain conditions are necessary. Research using inflammatory panels and omics studies in FM is still in a descriptive and exploratory stage. In future studies it will be important to identify the involved biological processes; it is less likely that only one or few proteins will be important for group differentiation and for certain aspects of the clinical presentation. Larger studies are clearly required to understand which alterations are common for several diagnoses and which are specific for FM. Here, we focus on the five most important proteins of each regression and their relationships in earlier studies to nociception and pain. When scrutinizing all proteins displayed in Tables 2–4, it is obvious that some proteins (e.g., MIP-1α/CCL3, MDC/CCL22, IFN-α2α, IL-1Ra, and IL-6) are involved in several regressions while other are specific. Within each diagnosis, it will be important to understand the influences of comorbidities as immunological and inflammatory biological processes have been reported for depression and ageing (154–156). In this explorative study we did not standardize the sampling of saliva with respect to the time of the day (157, 158). In future confirmatory studies, standardization is important to reduce the risk of bias (157, 158). Another limitation is that we did not screen the subjects for oral cavity diseases.
In FM, peripheral factors are clearly important for group differentiation, pressure pain threshold, as well as pain intensity. Saliva cytokines and chemokines were elevated in FM. Plasma proteins were correlated with both sensitivity and intensity of pain. In addition, cytokines and chemokines were also clearly associated with muscle fat infiltration and body fat content variables. The standardized fat free muscle volume of the thigh (z-T-FFMV) was significantly lower in FM and associated with intramuscular energy metabolites and plasma proteins. FM is clearly associated with the presence of systemic chronic low-grade systemic inflammation, including immune involvement, and one of the main sources for this is peripheral adipose tissue. Therefore, it is reasonable to believe that FM is characterized by a range of interactions between peripheral tissues, such as muscles and the peripheral and central nervous systems, including nociceptive, immune, and neuroendocrine processes. Clearly, future research of FM should focus on complex patterns of molecules and biological processes in several systems.
The original contributions presented in the study are included in the article/Supplementary Material, further inquiries can be directed to the corresponding author.
The Regional Ethical Review Board in Linköping (Dnr: 2016/239-31) Sweden approved the study. The studies were conducted in accordance with the local legislation and institutional requirements. The participants provided their written informed consent to participate in this study.
BGe: Conceptualization, Data curation, Formal Analysis, Funding acquisition, Investigation, Methodology, Project administration, Resources, Supervision, Visualization, Writing – original draft, Writing – review & editing. ODL: Conceptualization, Formal Analysis, Methodology, Resources, Software, Validation, Writing – review & editing. EL: Conceptualization, Methodology, Writing – review & editing. PL: Conceptualization, Investigation, Methodology, Resources, Writing – review & editing. MFF: Conceptualization, Data curation, Formal Analysis, Investigation, Methodology, Resources, Software, Validation, Visualization, Writing – review & editing. BGh: Conceptualization, Investigation, Methodology, Resources, Software, Validation, Writing – review & editing.
The author(s) declare financial support was received for the research, authorship, and/or publication of this article.
This research was funded by grants from the Swedish Research Council (grant number: 2018-02470) and a fibromyalgia patient organization (Fibromyalgia Foundation).
MD PhD Ann Bengtsson, research nurse Eva-Britt Lind and research physiotherapist Ulrika Wentzel Olausson are acknowledged for skillful data collection and support during the clinical examinations.
OD and MF were employed by AMRA Medical AB.
The remaining authors declare that the research was conducted in the absence of any commercial or financial relationships that could be construed as a potential conflict of interest.
All claims expressed in this article are solely those of the authors and do not necessarily represent those of their affiliated organizations, or those of the publisher, the editors and the reviewers. Any product that may be evaluated in this article, or claim that may be made by its manufacturer, is not guaranteed or endorsed by the publisher.
The Supplementary Material for this article can be found online at: https://www.frontiersin.org/articles/10.3389/fpain.2024.1288024/full#supplementary-material
1. Wolfe F, Smythe HA, Yunus MB, Bennett RM, Bombardier C, Goldenberg DL, et al. The American college of rheumatology 1990 criteria for the classification of fibromyalgia. Report of the multicenter criteria committee. Arthritis Rheum. (1990) 33(2):160–72. doi: 10.1002/art.1780330203
2. Wolfe F, Walitt B, Perrot S, Rasker JJ, Hauser W. Fibromyalgia diagnosis and biased assessment: sex, prevalence and bias. PLoS One. (2018) 13(9):e0203755. doi: 10.1371/journal.pone.0203755
3. Gran JT. The epidemiology of chronic generalized musculoskeletal pain. Best Pract Res Clin Rheumatol. (2003) 17(4):547–61. doi: 10.1016/S1521-6942(03)00042-1
4. Queiroz LP. Worldwide epidemiology of fibromyalgia. Curr Pain Headache Rep. (2013) 17(8):356. doi: 10.1007/s11916-013-0356-5
5. Schork NJ. Personalized medicine: time for one-person trials. Nature. (2015) 520(7549):609–11. doi: 10.1038/520609a
6. Kosek E, Cohen M, Baron R, Gebhart GF, Mico JA, Rice AS, et al. Do we need a third mechanistic descriptor for chronic pain states? Pain. (2016) 157(7):1382–6. doi: 10.1097/j.pain.0000000000000507
7. Jensen KB, Kosek E, Petzke F, Carville S, Fransson P, Marcus H, et al. Evidence of dysfunctional pain inhibition in fibromyalgia reflected in rACC during provoked pain. Pain. (2009) 144(1–2):95–100. doi: 10.1016/j.pain.2009.03.018
8. Schrepf A, Harper DE, Harte SE, Wang H, Ichesco E, Hampson JP, et al. Endogenous opioidergic dysregulation of pain in fibromyalgia: a PET and fMRI study. Pain. (2016) 157(10):2217–25. doi: 10.1097/j.pain.0000000000000633
9. Sluka KA, Clauw DJ. Neurobiology of fibromyalgia and chronic widespread pain. Neuroscience. (2016) 338:114–29. doi: 10.1016/j.neuroscience.2016.06.006
10. Goubert D, Danneels L, Graven-Nielsen T, Descheemaeker F, Meeus M. Differences in pain processing between patients with chronic low back pain, recurrent low back pain, and fibromyalgia. Pain Physician. (2017) 20(4):307–18. 28535553.28535553
11. Gerdle B, Larsson B. Muscle. In: Häuser W, Perrot S, editors. Fibromyalgia Syndrome and Widespread Pain—From Construction to Relevant Recognition. Philadelphia: Wolters Kluwer (2018). p. 215–31.
12. Littlejohn G, Guymer E. Neurogenic inflammation in fibromyalgia. Semin Immunopathol. (2018) 40(3):291–300. doi: 10.1007/s00281-018-0672-2
13. Üçeyler N, Sommer C. Small nerve fiber pathology. In: Häuser W, Perrot S, editors. Fibromylagia Syndrome and Widespread Pain—From Construction to Relevant Recognition. Philadelphia: Wolters Kluwer (2018). p. 204–14.
14. Albrecht DS, Forsberg A, Sandstrom A, Bergan C, Kadetoff D, Protsenko E, et al. Brain glial activation in fibromyalgia—a multi-site positron emission tomography investigation. Brain Behav Immun. (2019) 75:72–83. doi: 10.1016/j.bbi.2018.09.018
15. Benlidayi I C. Role of inflammation in the pathogenesis and treatment of fibromyalgia. Rheumatol Int. (2019) 39(5):781–91. doi: 10.1007/s00296-019-04251-6
16. van Ettinger-Veenstra H, Boehme R, Ghafouri B, Olausson H, Wicksell RK, Gerdle B. Exploration of functional connectivity changes previously reported in fibromyalgia and their relation to psychological distress and pain measures. J Clin Med. (2020) 9:3560. doi: 10.3390/jcm9113560
17. van Ettinger-Veenstra H, Lundberg P, Alfoldi P, Sodermark M, Graven-Nielsen T, Sjors A, et al. Chronic widespread pain patients show disrupted cortical connectivity in default mode and salience networks, modulated by pain sensitivity. J Pain Res. (2019) 12:1743–55. doi: 10.2147/JPR.S189443
18. Grayston R, Czanner G, Elhadd K, Goebel A, Frank B, Uceyler N, et al. A systematic review and meta-analysis of the prevalence of small fiber pathology in fibromyalgia: implications for a new paradigm in fibromyalgia etiopathogenesis. Semin Arthritis Rheum. (2019) 48(5):933–40. doi: 10.1016/j.semarthrit.2018.08.003
19. Gerdle B, Ghafouri B, Lund E, Bengtsson A, Lundberg P, Ettinger-Veenstra HV, et al. Evidence of mitochondrial dysfunction in fibromyalgia: deviating muscle energy metabolism detected using microdialysis and magnetic resonance. J Clin Med. (2020) 9(11):3527. doi: 10.3390/jcm9113527
20. Gerdle B, Larsson B, Forsberg F, Ghafouri N, Karlsson L, Stensson N, et al. Chronic widespread pain: increased glutamate and lactate concentrations in the trapezius muscle and plasma. Clin J Pain. (2014) 30(5):409–20. doi: 10.1097/AJP.0b013e31829e9d2a
21. Gerdle B, Soderberg K, Salvador Puigvert L, Rosendal L, Larsson B. Increased interstitial concentrations of pyruvate and lactate in the trapezius muscle of patients with fibromyalgia: a microdialysis study. J Rehabil Med. (2010) 42(7):679–87. doi: 10.2340/16501977-0581
22. Gerdle B, Ernberg M, Mannerkorpi K, Larsson B, Kosek E, Christidis N, et al. Increased interstitial concentrations of glutamate and pyruvate in Vastus Lateralis of women with fibromyalgia syndrome are normalized after an exercise intervention—a case-control study. PLoS One. (2016) 11(10):e0162010. doi: 10.1371/journal.pone.0162010
23. Gerdle B, Forsgren M, Bengtsson A, Dahlqvist Leinhard O, Sören B, Karlsson A, et al. Decreased muscle concentrations of ATP and PCR in the quadriceps muscle of fibromyalgia patients—a 31P MRS study. Eur J Pain. (2013) 17:1205–15. doi: 10.1002/j.1532-2149.2013.00284.x
24. Park JH, Phothimat P, Oates CT, Hernanz-Schulman M, Olsen NJ. Use of P-31 magnetic resonance spectroscopy to detect metabolic abnormalities in muscles of patients with fibromyalgia. Arthritis Rheum. (1998) 41(3):406–13. doi: 10.1002/1529-0131(199803)41:3%3C406::AID-ART5%3E3.0.CO;2-L
25. Bengtsson A, Henriksson KG, Larsson J. Reduced high-energy phosphate levels in the painful muscles of patients with primary fibromyalgia. Arthritis Rheum. (1986) 29(7):817–21. doi: 10.1002/art.1780290701
26. Ghafouri N, Ghafouri B, Larsson B, Stensson N, Fowler CJ, Gerdle B. Palmitoylethanolamide and stearoylethanolamide levels in the interstitium of the trapezius muscle of women with chronic widespread pain and chronic neck-shoulder pain correlate with pain intensity and sensitivity. Pain. (2013) 154(9):1649–58. doi: 10.1016/j.pain.2013.05.002
27. Stensson N, Ghafouri B, Ghafouri N, Gerdle B. High levels of endogenous lipid mediators (N-acylethanolamines) in women with chronic widespread pain during acute tissue trauma. Mol Pain. (2016) 12:1–11. doi: 10.1177/1744806916662886
28. Gerdle B, Dahlqvist Leinhard O, Lund E, Bengtsson A, Lundberg P, Ghafouri B, et al. Fibromyalgia: association between fat infiltration and physical capacity and pain intensity and disability. J Pain Res. (2022) 15:2517–35. doi: 10.2147/JPR.S376590
29. Minerbi A, Gonzalez E, Brereton NJB, Anjarkouchian A, Dewar K, Fitzcharles MA, et al. Altered microbiome composition in individuals with fibromyalgia. Pain. (2019) 160(11):2589–602. doi: 10.1097/j.pain.0000000000001640
30. Clos-Garcia M, Andres-Marin N, Fernandez-Eulate G, Abecia L, Lavin JL, van Liempd S, et al. Gut microbiome and serum metabolome analyses identify molecular biomarkers and altered glutamate metabolism in fibromyalgia. EBioMedicine. (2019) 46:499–511. doi: 10.1016/j.ebiom.2019.07.031
31. Stensson N, Ghafouri N, Ernberg M, Mannerkorpi K, Kosek E, Gerdle B, et al. The relationship of endocannabinoidome lipid mediators with pain and psychological stress in women with fibromyalgia: a case-control study. J Pain. (2018) 19(11):1318–28. doi: 10.1016/j.jpain.2018.05.008
32. Stensson N, Ghafouri B, Gerdle B, Ghafouri N. Alterations of anti-inflammatory lipids in plasma from women with chronic widespread pain—a case control study. Lipids Health Dis. (2017) 16(1):112. doi: 10.1186/s12944-017-0505-7
33. Wåhlén K, Olausson P, Carlsson A, Ghafouri N, Gerdle B. Systemic alteration of plasma proteins from women with chronic widespread pain compared to healthy controls; a proteomic study. J Pain Res. (2017) 10:797–809. doi: 10.2147/JPR.S128597
34. Han CL, Sheng YC, Wang SY, Chen YH, Kang JH. Serum proteome profiles revealed dysregulated proteins and mechanisms associated with fibromyalgia syndrome in women. Sci Rep. (2020) 10(1):12347. doi: 10.1038/s41598-020-69271-w
35. Wåhlén K, Ernberg M, Kosek E, Mannerkorpi K, Gerdle B, Ghafouri B. Significant correlation between plasma proteome profile and pain intensity, sensitivity, and psychological distress in women with fibromyalgia. Sci Rep. (2020) 10(1):12508. doi: 10.1038/s41598-020-69422-z
36. Ramirez-Tejero JA, Martinez-Lara E, Rus A, Camacho MV, Del Moral ML, Siles E. Insight into the biological pathways underlying fibromyalgia by a proteomic approach. J Proteomics. (2018) 186:47–55. doi: 10.1016/j.jprot.2018.07.009
37. Andres-Rodriguez L, Borras X, Feliu-Soler A, Perez-Aranda A, Angarita-Osorio N, Moreno-Peral P, et al. Peripheral immune aberrations in fibromyalgia: a systematic review, meta-analysis and meta-regression. Brain Behav Immun. (2020) 87:881–9. doi: 10.1016/j.bbi.2019.12.020
38. O'Mahony LF, Srivastava A, Mehta P, Ciurtin C. Is fibromyalgia associated with a unique cytokine profile? A systematic review and meta-analysis. Rheumatology (Oxford). (2021) 60(6):2602–14. doi: 10.1093/rheumatology/keab146
39. Kumbhare D, Hassan S, Diep D, Duarte FCK, Hung J, Damodara S, et al. Potential role of blood biomarkers in patients with fibromyalgia: a systematic review with meta-analysis. Pain. (2022) 163:1232–53. doi: 10.1097/j.pain.0000000000002510
40. Markkula RA, Kalso EA, Kaprio JA. Predictors of fibromyalgia: a population-based twin cohort study. BMC Musculoskelet Disord. (2016) 17:29. doi: 10.1186/s12891-016-0873-6
41. Mork PJ, Vasseljen O, Nilsen TI. Association between physical exercise, body mass index, and risk of fibromyalgia: longitudinal data from the Norwegian nord-trondelag health study. Arthritis Care Res (Hoboken). (2010) 62(5):611–7. doi: 10.1002/acr.20118
42. Migliorini F, Maffulli N, Eschweiler J, Tingart M, Driessen A, Colarossi G. BMI but not age and sex negatively impact on the outcome of pharmacotherapy in fibromyalgia: a systematic review. Expert Rev Clin Pharmacol. (2021) 14(8):1029–38. doi: 10.1080/17512433.2021.1929923
43. D'Onghia M, Ciaffi J, Lisi L, Mancarella L, Ricci S, Stefanelli N, et al. Fibromyalgia and obesity: a comprehensive systematic review and meta-analysis. Semin Arthritis Rheum. (2021) 51(2):409–24. doi: 10.1016/j.semarthrit.2021.02.007
44. Ghafouri B, Edman E, Lof M, Lund E, Leinhard OD, Lundberg P, et al. Fibromyalgia in women: association of inflammatory plasma proteins, muscle blood flow, and metabolism with body mass index and pain characteristics. Pain Reports. (2022) 7(6):e1042. doi: 10.1097/PR9.0000000000001042
45. Wu H, Ballantyne CM. Metabolic inflammation and insulin resistance in obesity. Circ Res. (2020) 126(11):1549–64. doi: 10.1161/CIRCRESAHA.119.315896
46. Verdu E, Homs J, Boadas-Vaello P. Physiological changes and pathological pain associated with sedentary lifestyle-induced body systems fat accumulation and their modulation by physical exercise. Int J Environ Res Public Health. (2021) 18:13333. doi: 10.3390/ijerph182413333
47. Taylor EB. The complex role of adipokines in obesity, inflammation, and autoimmunity. Clin Sci (Lond). (2021) 135(6):731–52. doi: 10.1042/CS20200895
48. Khanna D, Khanna S, Khanna P, Kahar P, Patel BM. Obesity: a chronic low-grade inflammation and its markers. Cureus. (2022) 14(2):e22711. doi: 10.7759/cureus.22711
49. Ponce-de-Leon M, Linseisen J, Peters A, Linkohr B, Heier M, Grallert H, et al. Novel associations between inflammation-related proteins and adiposity: a targeted proteomics approach across four population-based studies. Transl Res. (2022) 242:93–104. doi: 10.1016/j.trsl.2021.11.004
50. Zigmond AS, Snaith RP. The hospital anxiety and depression scale. Acta Psychiatr Scand. (1983) 67(6):361–70. doi: 10.1111/j.1600-0447.1983.tb09716.x
51. LoMartire R, Ang BO, Gerdle B, Vixner L. Psychometric properties of short form-36 health survey, EuroQol 5-dimensions, and hospital anxiety and depression scale in patients with chronic pain. Pain. (2020) 161(1):83–95. doi: 10.1097/j.pain.0000000000001700
52. Bjelland I, Dahl AA, Haug TT, Neckelmann D. The validity of the hospital anxiety and depression scale. An updated literature review. J Psychosom Res. (2002) 52(2):69–77. doi: 10.1016/S0022-3999(01)00296-3
53. EuroQol. Euroqol: a new facility for the measurement of health-related quality of life. Health Policy. (1990) 16:199–208. doi: 10.1016/0168-8510(90)90421-9
54. Brooks R. Euroqol: the current state of play. Health Policy. (1996) 37(1):53–72. doi: 10.1016/0168-8510(96)00822-6
55. Wolfe F, Clauw DJ, Fitzcharles MA, Goldenberg DL, Katz RS, Mease P, et al. The American college of rheumatology preliminary diagnostic criteria for fibromyalgia and measurement of symptom severity. Arthritis Care Res (Hoboken). (2010) 62(5):600–10. doi: 10.1002/acr.20140
56. Wolfe F, Clauw DJ, Fitzcharles MA, Goldenberg DL, Hauser W, Katz RS, et al. Fibromyalgia criteria and severity scales for clinical and epidemiological studies: a modification of the ACR preliminary diagnostic criteria for fibromyalgia. J Rheumatol. (2011) 38(6):1113–22. doi: 10.3899/jrheum.100594
57. Wolfe F, Clauw DJ, Fitzcharles MA, Goldenberg DL, Hauser W, Katz RL, et al. 2016 Revisions to the 2010/2011 fibromyalgia diagnostic criteria. Semin Arthritis Rheum. (2016) 46(3):319–29. doi: 10.1016/j.semarthrit.2016.08.012
58. Wallin M, Liedberg G, Börsbo B, Gerdle B. Thermal detection and pain thresholds but not pressure pain thresholds are correlated with psychological factors in women with chronic whiplash-associated pain. Clin J Pain. (2012) 28(3):211–21. doi: 10.1097/AJP.0b013e318226c3fd
59. Lemming D, Borsbo B, Sjors A, Lind EB, Arendt-Nielsen L, Graven-Nielsen T, et al. Single-point but not tonic cuff pressure pain sensitivity is associated with level of physical fitness–a study of non-athletic healthy subjects. PLoS One. (2015) 10(5):e0125432. doi: 10.1371/journal.pone.0125432
60. Borga M, Ahlgren A, Romu T, Widholm P, Dahlqvist Leinhard O, West J. Reproducibility and repeatability of MRI-based body composition analysis. Magn Reson Med. (2020) 84(6):3146–56. doi: 10.1002/mrm.28360
61. Borga M, Thomas EL, Romu T, Rosander J, Fitzpatrick J, Dahlqvist Leinhard O, et al. Validation of a fast method for quantification of intra-abdominal and subcutaneous adipose tissue for large-scale human studies. NMR Biomed. (2015) 28(12):1747–53. doi: 10.1002/nbm.3432
62. Karlsson A, Rosander J, Romu T, Tallberg J, Gronqvist A, Borga M, et al. Automatic and quantitative assessment of regional muscle volume by multi-atlas segmentation using whole-body water-fat MRI. J Magn Reson Imaging. (2015) 41(6):1558–69. doi: 10.1002/jmri.24726
63. West J, Romu T, Thorell S, Lindblom H, Berin E, Holm AS, et al. Precision of MRI-based body composition measurements of postmenopausal women. PLoS One. (2018) 13(2):e0192495. doi: 10.1371/journal.pone.0192495
64. West J, Dahlqvist Leinhard O, Romu T, Collins R, Garratt S, Bell JD, et al. Feasibility of MR-based body composition analysis in large scale population studies. PLoS One. (2016) 11(9):e0163332. doi: 10.1371/journal.pone.0163332
65. Linge J, Borga M, West J, Tuthill T, Miller MR, Dumitriu A, et al. Body composition profiling in the UK biobank imaging study. Obesity (Silver Spring). (2018) 26(11):1785–95. doi: 10.1002/oby.22210
66. Sudlow C, Gallacher J, Allen N, Beral V, Burton P, Danesh J, et al. UK biobank: an open access resource for identifying the causes of a wide range of complex diseases of middle and old age. PLoS Med. (2015) 12(3):e1001779. doi: 10.1371/journal.pmed.1001779
67. Littlejohns TJ, Holliday J, Gibson LM, Garratt S, Oesingmann N, Alfaro-Almagro F, et al. The UK biobank imaging enhancement of 100,000 participants: rationale, data collection, management and future directions. Nat Commun. (2020) 11(1):2624. doi: 10.1038/s41467-020-15948-9
68. Linge J, Petersson M, Forsgren MF, Sanyal AJ, Dahlqvist Leinhard O. Adverse muscle composition predicts all-cause mortality in the UK biobank imaging study. J Cachexia Sarcopenia Muscle. (2021) 12(6):1513–26. doi: 10.1002/jcsm.12834
69. Linge J, Heymsfield SB, Dahlqvist Leinhard O. On the definition of sarcopenia in the presence of aging and obesity-initial results from UK biobank. J Gerontol A Biol Sci Med Sci. (2020) 75(7):1309–16. doi: 10.1093/gerona/glz229
70. Linge J, Widholm P, Nilsson D, Kugelberg A, Olbers T, Dahlqvist Leinhard O. Risk stratification using MRI-derived, personalized visceral-, subcutaneous-, and liver fat z-scores in persons with obesity. Surg Obes Relat Dis. (2023) 19(6 - Supplement):S3–S4.
71. Wheelock AM, Wheelock CE. Trials and tribulations of ‘omics data analysis: assessing quality of SIMCA-based multivariate models using examples from pulmonary medicine. Mol Biosyst. (2013) 9(11):2589–96. doi: 10.1039/c3mb70194h
72. Ringqvist Å, Dragioti E, Björk M, Larsson B, Gerdle B. Moderate and stable pain reductions as a result of interdisciplinary pain rehabilitation—a cohort study from the Swedish quality registry for pain rehabilitation (SQRP). J Clin Med. (2019) 8:905. doi: 10.3390/jcm8060905
73. Eriksson L, Byrne T, Johansson E, Trygg J. Vikström C. Multi- and Megavariate Data Analysis: Basic Principles and Applications. 3rd revised edn ed. Malmö: MKS Umetrics AB (2013).
74. Backryd E, Tanum L, Lind AL, Larsson A, Gordh T. Evidence of both systemic inflammation and neuroinflammation in fibromyalgia patients, as assessed by a multiplex protein panel applied to the cerebrospinal fluid and to plasma. J Pain Res. (2017) 10:515–25. doi: 10.2147/JPR.S128508
75. Gerdle B, Ghafouri B, Ghafouri N, Bäckryd E, Gordh T. Signs of ongoing inflammation in female patients with chronic widespread pain—a multivariate, explorative, cross-sectional study of blood samples. Medicine (Baltimore). (2017) 96(9):e6130. doi: 10.1097/MD.0000000000006130
76. Goncalves Dos Santos G, Delay L, Yaksh TL, Corr M. Neuraxial cytokines in pain states. Front Immunol. (2019) 10:3061. doi: 10.3389/fimmu.2019.03061
77. Jones GW, Hill DG, Cardus A, Jones SA. IL-27: a double agent in the IL-6 family. Clin Exp Immunol. (2018) 193(1):37–46. doi: 10.1111/cei.13116
78. Fonseca MM, Davoli-Ferreira M, Santa-Cecilia F, Guimaraes RM, Oliveira FFB, Kusuda R, et al. IL-27 counteracts neuropathic pain development through induction of IL-10. Front Immunol. (2019) 10:3059. doi: 10.3389/fimmu.2019.03059
79. Li H, Wu M, Zhao X. Role of chemokine systems in cancer and inflammatory diseases. MedComm (2020). (2022) 3(2):e147. doi: 10.1002/mco2.147
80. Zhang YL, Li B, Zhou ZH. A cross-sectional study: serum CCL3/MIP-1alpha levels may reflect lumbar intervertebral disk degeneration in Han Chinese people. J Pain Res. (2018) 11:497–503. doi: 10.2147/JPR.S152349
81. Domingues RB, Duarte H, Senne C, Bruniera G, Brunale F, Rocha NP, et al. Serum levels of adiponectin, CCL3/MIP-1alpha, and CCL5/RANTES discriminate migraine from tension-type headache patients. Arq Neuropsiquiatr. (2016) 74(8):626–31. doi: 10.1590/0004-282X20160096
82. Quick ML, Mukherjee S, Rudick CN, Done JD, Schaeffer AJ, Thumbikat P. CCL2 and CCL3 are essential mediators of pelvic pain in experimental autoimmune prostatitis. Am J Physiol Regul Integr Comp Physiol. (2012) 303(6):R580–9. doi: 10.1152/ajpregu.00240.2012
83. Garcia JJ, Cidoncha A, Bote ME, Hinchado MD, Ortega E. Altered profile of chemokines in fibromyalgia patients. Ann Clin Biochem. (2014) 51(Pt 5):576–81. doi: 10.1177/0004563213506413
84. Weisheng B, Nezhat CH, Huang GF, Mao YQ, Sidell N, Huang RP. Discovering endometriosis biomarkers with multiplex cytokine arrays. Clin Proteomics. (2019) 16:28. doi: 10.1186/s12014-019-9248-y
85. Li L, Dai F, Wang L, Sun Y, Mei L, Ran Y, et al. CCL13 and human diseases. Front Immunol. (2023) 14:1176639. doi: 10.3389/fimmu.2023.1176639
86. Yamaguchi A, Nozawa K, Fujishiro M, Kawasaki M, Suzuki F, Takamori K, et al. CC motif chemokine ligand 13 is associated with rheumatoid arthritis pathogenesis. Mod Rheumatol. (2013) 23(5):856–63. doi: 10.3109/s10165-012-0752-4
87. Noor S, Wilson EH. Role of C-C chemokine receptor type 7 and its ligands during neuroinflammation. J Neuroinflammation. (2012) 9:77. doi: 10.1186/1742-2094-9-77
88. Scanzello CR. Chemokines and inflammation in osteoarthritis: insights from patients and animal models. J Orthop Res. (2017) 35(4):735–9. doi: 10.1002/jor.23471
89. Blanco-Vazquez M, Vazquez A, Fernandez I, Novo-Diez A, Martinez-Plaza E, Garcia-Vazquez C, et al. Inflammation-related molecules in tears of patients with chronic ocular pain and dry eye disease. Exp Eye Res. (2022) 219:109057. doi: 10.1016/j.exer.2022.109057
90. Hoyer KK, Dooms H, Barron L, Abbas AK. Interleukin-2 in the development and control of inflammatory disease. Immunol Rev. (2008) 226:19–28. doi: 10.1111/j.1600-065X.2008.00697.x
91. Fazzari J, Sidhu J, Motkur S, Inman M, Buckley N, Clemons M, et al. Applying Serum cytokine levels to predict pain severity in cancer patients. J Pain Res. (2020) 13:313–21. doi: 10.2147/JPR.S227175
92. Turner MD, Nedjai B, Hurst T, Pennington DJ. Cytokines and chemokines: at the crossroads of cell signalling and inflammatory disease. Biochim Biophys Acta. (2014) 1843(11):2563–82. doi: 10.1016/j.bbamcr.2014.05.014
93. Bruderer R, Muntel J, Muller S, Bernhardt OM, Gandhi T, Cominetti O, et al. Analysis of 1508 plasma samples by capillary-flow data-independent acquisition profiles proteomics of weight loss and maintenance. Mol Cell Proteomics. (2019) 18(6):1242–54. doi: 10.1074/mcp.RA118.001288
94. Yurkovich JT, Hood L. Blood is a window into health and disease. Clin Chem. (2019) 65(10):1204–6. doi: 10.1373/clinchem.2018.299065
95. Baral P, Udit S, Chiu IM. Pain and immunity: implications for host defence. Nat Rev Immunol. (2019) 19(7):433–47. doi: 10.1038/s41577-019-0147-2
96. Iwata M, Ota KT, Duman RS. The inflammasome: pathways linking psychological stress, depression, and systemic illnesses. Brain Behav Immun. (2013) 31:105–14. doi: 10.1016/j.bbi.2012.12.008
97. Pavlov VA, Tracey KJ. Neural circuitry and immunity. Immunol Res. (2015) 63(1–3):38–57. doi: 10.1007/s12026-015-8718-1
98. Verma V, Sheikh Z, Ahmed AS. Nociception and role of immune system in pain. Acta Neurol Belg. (2015) 115(3):213–20. doi: 10.1007/s13760-014-0411-y
99. Eisenberger NI, Moieni M, Inagaki TK, Muscatell KA, Irwin MR. In sickness and in health: the co-regulation of inflammation and social behavior. Neuropsychopharmacology. (2017) 42(1):242–53. doi: 10.1038/npp.2016.141
100. Kraynak TE, Marsland AL, Wager TD, Gianaros PJ. Functional neuroanatomy of peripheral inflammatory physiology: a meta-analysis of human neuroimaging studies. Neurosci Biobehav Rev. (2018) 94:76–92. doi: 10.1016/j.neubiorev.2018.07.013
101. Karshikoff B, Tadros M, Mackey S, Zouikr I. Neuroimmune modulation of pain across the developmental spectrum. Curr Opin Behav Sci. (2019) 28:85–92. doi: 10.1016/j.cobeha.2019.01.010
102. Vichaya EG, Dantzer R. Inflammation-induced motivational changes: perspective gained by evaluating positive and negative valence systems. Curr Opin Behav Sci. (2018) 22:90–5. doi: 10.1016/j.cobeha.2018.01.008
103. Lampa J, Westman M, Kadetoff D, Agreus AN, Le Maitre E, Gillis-Haegerstrand C, et al. Peripheral inflammatory disease associated with centrally activated IL-1 system in humans and mice. Proc Natl Acad Sci U S A. (2012) 109(31):12728–33. doi: 10.1073/pnas.1118748109
104. Karshikoff B, Jensen KB, Kosek E, Kalpouzos G, Soop A, Ingvar M, et al. Why sickness hurts: a central mechanism for pain induced by peripheral inflammation. Brain Behav Immun. (2016) 57:38–46. doi: 10.1016/j.bbi.2016.04.001
105. Kempuraj D, Thangavel R, Selvakumar GP, Zaheer S, Ahmed ME, Raikwar SP, et al. Brain and peripheral atypical inflammatory mediators potentiate neuroinflammation and neurodegeneration. Front Cell Neurosci. (2017) 11:216. doi: 10.3389/fncel.2017.00216
106. Olausson P, Ghafouri B, Bäckryd E, Gerdle B. Clear differences in cerebrospinal fluid proteome between women with chronic widespread pain and healthy women—a multivariate explorative cross-sectional study. J Pain Res. (2017) 10:575–90. doi: 10.2147/JPR.S125667
107. Marchetti L, Engelhardt B. Immune cell trafficking across the blood-brain barrier in the absence and presence of neuroinflammation. Vasc Biol. (2020) 2(1):H1–H18. doi: 10.1530/VB-19-0033
108. Ji RR, Nackley A, Huh Y, Terrando N, Maixner W. Neuroinflammation and central sensitization in chronic and widespread pain. Anesthesiology. (2018) 129(2):343–66. doi: 10.1097/ALN.0000000000002130
109. Palada V, Ahmed AS, Finn A, Berg S, Svensson CI, Kosek E. Characterization of neuroinflammation and periphery-to-CNS inflammatory cross-talk in patients with disc herniation and degenerative disc disease. Brain Behav Immun. (2019) 75:60–71. doi: 10.1016/j.bbi.2018.09.010
110. Heurich M, Focking M, Mongan D, Cagney G, Cotter DR. Dysregulation of complement and coagulation pathways: emerging mechanisms in the development of psychosis. Mol Psychiatry. (2022) 27:127–140. doi: 10.1038/s41380-021-01197-9
111. Goebel A, Krock E, Gentry C, Israel MR, Jurczak A, Urbina CM, et al. Passive transfer of fibromyalgia symptoms from patients to mice. J Clin Invest. (2021) 131(13):e144201. doi: 10.1172/JCI144201
112. Parisien M, Lima LV, Dagostino C, El-Hachem N, Drury GL, Grant AV, et al. Acute inflammatory response via neutrophil activation protects against the development of chronic pain. Sci Transl Med. (2022) 14(644):eabj9954. doi: 10.1126/scitranslmed.abj9954
113. Boroumand M, Olianas A, Cabras T, Manconi B, Fanni D, Faa G, et al. Saliva, a bodily fluid with recognized and potential diagnostic applications. J Sep Sci. (2021) 44(19):3677–90. doi: 10.1002/jssc.202100384
114. Shakeeb N, Varkey P, Ajit A. Human Saliva as a diagnostic specimen for early detection of inflammatory biomarkers by real-time RT-PCR. Inflammation. (2021) 44(5):1713–23. doi: 10.1007/s10753-021-01484-1
115. Kulak-Bejda A, Waszkiewicz N, Bejda G, Zalewska A, Maciejczyk M. Diagnostic value of salivary markers in neuropsychiatric disorders. Dis Markers. (2019) 2019:4360612. doi: 10.1155/2019/4360612
116. Ciregia F, Giacomelli C, Giusti L, Boldrini C, Piga I, Pepe P, et al. Putative salivary biomarkers useful to differentiate patients with fibromyalgia. J Proteomics. (2019) 190:44–54. doi: 10.1016/j.jprot.2018.04.012
117. Bazzichi L, Ciregia F, Giusti L, Baldini C, Giannaccini G, Giacomelli C, et al. Detection of potential markers of primary fibromyalgia syndrome in human saliva. Proteomics Clin Appl. (2009) 3(11):1296–304. doi: 10.1002/prca.200900076
118. Jonsson M, Gerdle B, Ghafouri B, Backryd E. The inflammatory profile of cerebrospinal fluid, plasma, and saliva from patients with severe neuropathic pain and healthy controls-a pilot study. BMC Neurosci. (2021) 22(1):6. doi: 10.1186/s12868-021-00608-5
119. Jasim H, Ghafouri B, Gerdle B, Hedenberg-Magnusson B, Ernberg M. Altered levels of salivary and plasma pain related markers in temporomandibular disorders. J Headache Pain. (2020) 21(1):105. doi: 10.1186/s10194-020-01160-z
120. Jasim H, Carlsson A, Hedenberg-Magnusson B, Ghafouri B, Ernberg M. Saliva as a medium to detect and measure biomarkers related to pain. Sci Rep. (2018) 8(1):3220. doi: 10.1038/s41598-018-21131-4
121. Balasa AF, Chircov C, Grumezescu AM. Body fluid biomarkers for Alzheimer’s disease-an up-to-date overview. Biomedicines. (2020) 8:421. doi: 10.3390/biomedicines8100421
122. Wormwood KL, Aslebagh R, Channaveerappa D, Dupree EJ, Borland MM, Ryan JP, et al. Salivary proteomics and biomarkers in neurology and psychiatry. Proteomics Clin Appl. (2015) 9(9–10):899–906. doi: 10.1002/prca.201400153
123. Ashton NJ, Blennow K, Zetterberg H. Spitting image: can saliva biomarkers reflect Alzheimer’s disease? EBioMedicine. (2021) 68:103437. doi: 10.1016/j.ebiom.2021.103437
124. Schepici G, Silvestro S, Trubiani O, Bramanti P, Mazzon E. Salivary biomarkers: future approaches for early diagnosis of neurodegenerative diseases. Brain Sci. (2020) 10:245. doi: 10.3390/brainsci10040245
125. Wåhlén K, Ghafouri B, Ghafouri N, Gerdle B. Plasma protein pattern correlates with pain intensity and psychological distress in women with chronic widespread pain. Front Psychol. (2018) 9:2400. doi: 10.3389/fpsyg.2018.02400
126. Olausson P, Ghafouri B, Ghafouri N, Gerdle B. Specific proteins of the trapezius muscle correlate with pain intensity and sensitivity—an explorative multivariate proteomic study of the trapezius muscle in women with chronic widespread pain. J Pain Res. (2016) 9:345–56. doi: 10.2147/JPR.S102275
127. Zhao YC, Hu T, Chen Y, Du KT. Elevated Serum levels of monocyte chemotactic protein-1/chemokine C-C motif ligand 2 are linked to disease severity in patients with fibromyalgia syndrome. Balkan Med J. (2019) 36(6):331–6. doi: 10.4274/balkanmedj.galenos.2019.2019.6.47
128. Teodorczyk-Injeyan JA, Triano JJ, McGregor M, Woodhouse L, Injeyan HS. Elevated production of inflammatory mediators including nociceptive chemokines in patients with neck pain: a cross-sectional evaluation. J Manipulative Physiol Ther. (2011) 34(8):498–505. doi: 10.1016/j.jmpt.2011.08.010
129. Groven N, Fors EA, Stunes AK, Reitan SK. MCP-1 is increased in patients with CFS and FM, whilst several other immune markers are significantly lower than healthy controls. Brain Behav Immun Health. (2020) 4:100067. doi: 10.1016/j.bbih.2020.100067
130. Farrell SF, de Zoete RMJ, Cabot PJ, Sterling M. Systemic inflammatory markers in neck pain: a systematic review with meta-analysis. Eur J Pain. (2020) 24(9):1666–86. doi: 10.1002/ejp.1630
131. Matute Wilander A, Karedal M, Axmon A, Nordander C. Inflammatory biomarkers in serum in subjects with and without work related neck/shoulder complaints. BMC Musculoskelet Disord. (2014) 15:103. doi: 10.1186/1471-2474-15-103
132. Baba T, Mukaida N. Role of macrophage inflammatory protein (MIP)-1alpha/CCL3 in leukemogenesis. Mol Cell Oncol. (2014) 1(1):e29899. doi: 10.4161/mco.29899
133. Estevao C, Bowers CE, Luo D, Sarker M, Hoeh AE, Frudd K, et al. CCL4 induces inflammatory signalling and barrier disruption in the neurovascular endothelium. Brain Behav Immun Health. (2021) 18:100370. doi: 10.1016/j.bbih.2021.100370
134. Schmelz M. Itch processing in the skin. Front Med (Lausanne). (2019) 6:167. doi: 10.3389/fmed.2019.00167
135. Urata Y, Osuga Y, Izumi G, Takamura M, Koga K, Nagai M, et al. Interleukin-1beta stimulates the secretion of thymic stromal lymphopoietin (TSLP) from endometrioma stromal cells: possible involvement of TSLP in endometriosis. Hum Reprod. (2012) 27(10):3028–35. doi: 10.1093/humrep/des291
136. Agarwal R, Volkmer TM, Wang P, Lee LA, Wang Q, Garcia AJ. Synthesis of self-assembled IL-1Ra-presenting nanoparticles for the treatment of osteoarthritis. J Biomed Mater Res A. (2016) 104(3):595–9. doi: 10.1002/jbm.a.35601
137. Elkayam O, Yaron I, Shirazi I, Yaron M, Caspi D. Serum levels of IL-10, IL-6, IL-1ra, and sIL-2R in patients with psoriatic arthritis. Rheumatol Int. (2000) 19(3):101–5. doi: 10.1007/s002960050111
138. McGeachy MJ, Cua DJ, Gaffen SL. The IL-17 family of cytokines in health and disease. Immunity. (2019) 50(4):892–906. doi: 10.1016/j.immuni.2019.03.021
139. Jiang X, Zhou R, Zhang Y, Zhu T, Li Q, Zhang W. Interleukin-17 as a potential therapeutic target for chronic pain. Front Immunol. (2022) 13:999407. doi: 10.3389/fimmu.2022.999407
140. Sumaiya K, Langford D, Natarajaseenivasan K, Shanmughapriya S. Macrophage migration inhibitory factor (MIF): a multifaceted cytokine regulated by genetic and physiological strategies. Pharmacol Ther. (2022) 233:108024. doi: 10.1016/j.pharmthera.2021.108024
141. Gjefsen E, Gervin K, Goll G, Braten LCH, Wigemyr M, Aass HCD, et al. Macrophage migration inhibitory factor: a potential biomarker for chronic low back pain in patients with modic changes. RMD Open. (2021) 7:e001726. doi: 10.1136/rmdopen-2021-001726
142. Schernthaner C, Paar V, Wernly B, Pistulli R, Rohm I, Jung C, et al. Elevated plasma levels of interleukin-16 in patients with acute myocardial infarction. Medicine (Baltimore). (2017) 96(44):e8396. doi: 10.1097/MD.0000000000008396
143. Saleh R, Lee MC, Khiew SH, Louis C, Fleetwood AJ, Achuthan A, et al. CSF-1 in inflammatory and arthritic pain development. J Immunol. (2018) 201(7):2042–53. doi: 10.4049/jimmunol.1800665
144. Ushach I, Zlotnik A. Biological role of granulocyte macrophage colony-stimulating factor (GM-CSF) and macrophage colony-stimulating factor (M-CSF) on cells of the myeloid lineage. J Leukoc Biol. (2016) 100(3):481–9. doi: 10.1189/jlb.3RU0316-144R
145. Karshikoff B, Wahlen K, Astrom J, Lekander M, Holmstrom L, Wicksell RK. Inflammatory blood signature related to common psychological comorbidity in chronic pain. Biomedicines. (2023) 11(3):713. doi: 10.3390/biomedicines11030713
146. Kawai T, Autieri MV, Scalia R. Adipose tissue inflammation and metabolic dysfunction in obesity. Am J Physiol Cell Physiol. (2021) 320(3):C375–C91. doi: 10.1152/ajpcell.00379.2020
147. Garcia JJ, Carvajal-Gil J, Guerrero-Bonmatty R. Altered release of chemokines by phagocytes from fibromyalgia patients: a pilot study. Innate Immun. (2016) 22(1):3–8. doi: 10.1177/1753425915602959
148. Safa A, Rashidinejad HR, Khalili M, Dabiri S, Nemati M, Mohammadi MM, et al. Higher circulating levels of chemokines CXCL10, CCL20 and CCL22 in patients with ischemic heart disease. Cytokine. (2016) 83:147–57. doi: 10.1016/j.cyto.2016.04.006
149. Peerlings D, Mimpen M, Damoiseaux J. The IL-2–IL-2 receptor pathway: key to understanding multiple sclerosis. J Transl Autoimmun. (2021) 4:100123. doi: 10.1016/j.jtauto.2021.100123
150. Severinsen MCK, Pedersen BK. Muscle-organ crosstalk: the emerging roles of myokines. Endocr Rev. (2020) 41(4):594–609. doi: 10.1210/endrev/bnaa016
151. Zhang Y, Su J. Interleukin-2 family cytokines: an overview of genes, expression, signaling and functional roles in teleost. Dev Comp Immunol. (2023) 141:104645. doi: 10.1016/j.dci.2023.104645
152. Gama JFG, Romualdo RD, de Assis ML, de Oliveira LM, Quirico-Santos T, Alves LA, et al. Role of regulatory T cells in skeletal muscle regeneration: a systematic review. Biomolecules. (2022) 12:817. doi: 10.3390/biom12060817
153. Gerdle B, Ghafouri B. Proteomic studies of common chronic pain conditions—a systematic review and associated network analyses. Expert Rev Proteomics. (2020) 17(6):483–505. doi: 10.1080/14789450.2020.1797499
154. Silva-Costa LC, Carlson PT, Guest PC, de Almeida V, Martins-de-Souza D. Proteomic markers for depression. Adv Exp Med Biol. (2019) 1118:191–206. doi: 10.1007/978-3-030-05542-4_10
155. Santos-Lozano A, Valenzuela PL, Llavero F, Lista S, Carrera-Bastos P, Hampel H, et al. Successful aging: insights from proteome analyses of healthy centenarians. Aging (Albany NY). (2020) 12(4):3502–15. doi: 10.18632/aging.102826
156. Cominetti O, Nunez Galindo A, Corthesy J, Valsesia A, Irincheeva I, Kussmann M, et al. Obesity shows preserved plasma proteome in large independent clinical cohorts. Sci Rep. (2018) 8(1):16981. doi: 10.1038/s41598-018-35321-7
157. Szabo YZ, Slavish DC. Measuring salivary markers of inflammation in health research: a review of methodological considerations and best practices. Psychoneuroendocrinology. (2021) 124:105069. doi: 10.1016/j.psyneuen.2020.105069
Keywords: adipose, biomarker, body composition, fat, fibromyalgia, inflammation, immune, pain
Citation: Gerdle B, Dahlqvist Leinhard O, Lund E, Lundberg P, Forsgren MF and Ghafouri B (2024) Pain and the biochemistry of fibromyalgia: patterns of peripheral cytokines and chemokines contribute to the differentiation between fibromyalgia and controls and are associated with pain, fat infiltration and content. Front. Pain Res. 5:1288024. doi: 10.3389/fpain.2024.1288024
Received: 3 September 2023; Accepted: 5 January 2024;
Published: 18 January 2024.
Edited by:
Shivani Ruparel, The University of Texas Health Science Center at San Antonio, United StatesReviewed by:
Michael Jankowski, Cincinnati Children's Hospital Medical Center, United States© 2024 Gerdle, Dahlqvist Leinhard, Lund, Lundberg, Forsgren and Ghafouri. This is an open-access article distributed under the terms of the Creative Commons Attribution License (CC BY). The use, distribution or reproduction in other forums is permitted, provided the original author(s) and the copyright owner(s) are credited and that the original publication in this journal is cited, in accordance with accepted academic practice. No use, distribution or reproduction is permitted which does not comply with these terms.
*Correspondence: Björn Gerdle Ympvcm4uZ2VyZGxlQGxpdS5zZQ==
Disclaimer: All claims expressed in this article are solely those of the authors and do not necessarily represent those of their affiliated organizations, or those of the publisher, the editors and the reviewers. Any product that may be evaluated in this article or claim that may be made by its manufacturer is not guaranteed or endorsed by the publisher.
Research integrity at Frontiers
Learn more about the work of our research integrity team to safeguard the quality of each article we publish.