- 1Department of General Surgery, The First Affiliated Hospital of Xi'an Jiaotong University, Xi’an, Shaanxi, China
- 2Center for Gut Microbiome Research, Med-X Institute, The First Affiliated Hospital of Xi’an Jiaotong University, Xi’an, Shaanxi, China
- 3Department of High Talent, The First Affiliated Hospital of Xi’an Jiaotong University, Xi’an, Shaanxi, China
Objective: To determine whether transthyretin (TTR) influences the prognosis of patients with colorectal cancers and establish a predictive model based on TTR.
Methods: Between January 2013 and February 2019, the clinical data of 1322 CRC patients aged from 18 years to 80 years who underwent surgical treatment were retrospectively analyzed. The preoperative TTR level, clinicopathological data, and follow-up data were recorded. The X-tile program was used to determine the optimal cut-off value. Cox proportional hazard regression analysis was conducted to evaluate the correlation between the TTR and the cumulative incidence of cancer-specific survival (CSS). Nomograms were then developed to predict CSS. Furthermore, an additional cohort of 377 CRC patients enrolled between January 2014 and December 2015 was included as an external validation.
Results: Based on the optimal cut-off value of 121.3 mg/L, we divided the patients into the TTR-lower group (<121.3 mg/L) and the TTR-higher group (≥121.3 mg/L). Comparative analysis revealed that the TTR-higher group exhibited a younger demographic, a higher prevalence of low colorectal cancers, an elevated R0 resection rate, superior differentiation, earlier stage and lower levels of carcinoembryonic antigen (CEA) in contrast to the TTR-lower group. The Cox multivariable analysis underscored the significance of TTR and various clinicopathological factors, encompassing age, tumor location, R0 resection status, differentiation grade, disease stage, postoperative chemoradiotherapy, and preoperative CEA levels, as substantial prognostic indicators. The postoperative survival nomogram, when internally and externally assessed, demonstrated commendable performance across multiple metrics, including the area under the receiver operating characteristic curve (AUC), calibration plots, and decision curve analysis (DCA). Compared with other models, the proportional hazards model combined with TTR demonstrates superior performance in terms of C-index, AUC, calibration chart, and DCA within the prognostic column chart.
Conclusions: The preoperative TTR was identified as a prognostic factor for predicting the long-term prognosis of CRC patients who underwent surgical treatment, supporting its role as a prognostic biomarker in clinical practice.
1 Introduction
Colorectal cancer (CRC) has risen to become the third most common cancer globally, with the second-highest mortality rate among all cancers. In 2020, over 1.8 million cases of colorectal cancer were reported, resulting in nearly 1 million mortalities, constituting approximately one-tenth of all cancer cases and fatalities (1). Increased exposure to tobacco use, unhealthy lifestyle, alcohol consumption, stress, and overweight has contributed to the rapid surge in CRC incidence and mortality rates (2). Notably, from 1990 to 2019, a significant upsurge in incidence and mortality rates has been observed among individuals under 50 years old, particularly in countries with a high sociodemographic index. This trend imposes a substantial financial burden on society (3). Despite the widespread adoption of the tumor-node-metastasis (TNM) staging system for evaluating CRC prognosis (4), advancements in clinical research and proteomics have unveiled multiple factors—such as R0 resection, pathology, immunity, metabolism, and the tumor microenvironment—significantly impacting prognosis (5–9). Moreover, extensive research has indicated that several serological indicators and plasma markers hold potential for predicting the prognosis of CRC patients (10–14). The quest for novel markers to assess the status of CRC patients holds paramount importance in determining survival and prognosis (15).
Transthyretin (TTR) is an extracellular protein and is mainly synthesized in the liver, choroid plexus, and retinal pigment epithelium (16). It transports the thyroid hormone thyroxine and the retinol-binding protein bound to retinol (17). Some cardiologists and neurologists have reported that TTR is also associated with cardiac amyloidosis and familial amyloid polyneuropathy (18–20). Also, TTR has been used as an indicator to assess the nutritional status along with serum albumin, body mass index (BMI), and prognostic nutritional index (PNI), especially in patients with terminal cancer or patients in severe conditions (21–24). Various levels of TTR have been detected in screening trial specimens among prostate cancer, lung cancer, and ovarian cancer, which indicates TTR could be a potential marker of malignant tumors (25). Some researchers have confirmed that lower serum TTR levels may be a factor in the poor prognosis of gastric cancers, hepatocellular carcinoma, intrahepatic cholangiocarcinoma, and non-small cell lung cancer (26–28). The up-regulated transthyretin can improve the chemosensitivity of ovarian cancer, thereby improving the prognosis and survival of patients (29).
However, few literatures have reported the interaction between TTR and CRC, and whether TTR can influence the prognosis of patients who were diagnosed with colorectal cancer. Additionally, there has been no research to establish a prognostic survival prediction model based on TTR. Considering its key role in physiology and clinical nutriology, we believe that TTR might affect the prognosis of colorectal cancer.
To confirm the assumption, we examined the relationship between TTR and clinical outcomes through a retrospective case-control study and established a prognosis model. This research aimed to evaluate the clinical predictive value of TTR for cancer-specific survival (CSS) in CRC patients undergoing surgical treatment. As far as we know, this research is the first to evaluate the importance of TTR in the prognosis of colorectal cancer patients and establish a predictive model.
2 Materials and methods
2.1 Patients
This retrospective case-control study enrolled 2277 consecutive patients diagnosed with colorectal cancer who received surgical resection at First Affiliated Hospital of Xi ‘an Jiaotong University between January 2013 and February 2019. The exclusion criteria and study flow chart are presented in Figure 1. Therefore, a total of 1322 patients diagnosed with colorectal cancer were finally included in this study as the training cohort. Additionally, 377 patients from the Second Affiliated Hospital of Xi’an Jiaotong University, diagnosed between January 2014 and December 2015, were included with the same inclusion and exclusion criteria to form the external validation cohort. The study adhered to the principles outlined in the Declaration of Helsinki. All patients provided written informed consent for data collection and subsequent analyses. The Institutional Review Board and Ethical Committee of the First Affiliated Hospital of Xi’an Jiaotong University approved this study (XJTU1AF2019LSK−227).
2.2 Preoperative evaluation and diagnosis
Upon admission, patients underwent a comprehensive evaluation, including imaging studies and preoperative assessments such as serological tests. Liver biochemical parameters and TTR levels were measured using the Beckman Coulter automated biochemical analysis system, performed by the Clinical Laboratory Department of our hospital. Based on the preoperative findings, an initial diagnosis was established.
2.3 Treatment for colorectal cancer
For patients with colon cancer clinically staged as cT1-4, N0-2, M0 (stages I-III), laparoscopic or robot-assisted colectomy with regional lymph node dissection is recommended. If postoperative pathology confirms negative surgical margins, the procedure is defined as an R0 resection. Among these patients, those classified as non-low risk or stage II and above are recommended to undergo adjuvant chemotherapy. Chemotherapy regimens include single-agent chemotherapy (oral capecitabine or biweekly intravenous administration of 5-FU/LV) and combination chemotherapy. Combination regimens consist of CAPEOX (XELOX), which includes oxaliplatin and capecitabine, or mFOLFOX6, which includes oxaliplatin, leucovorin (LV), and 5-FU. For patients with colon cancer clinically staged as T4bM0, palliative surgery is performed, which is classified as non-R0 resection. Postoperative treatment includes single-agent chemotherapy with 5-FU or combination chemotherapy. For patients with T4b sigmoid colon cancer, local radiotherapy may also be employed. For metastatic colon cancer with resectable liver metastases, both colectomy and liver metastasectomy are performed. If postoperative pathology confirms negative margins, the procedure is defined as R0 resection. Postoperative chemotherapy regimens include single-agent chemotherapy or combination regimens. For metastatic colon cancer deemed unresectable but complicated by symptoms like bleeding, perforation, or obstruction, palliative surgery is performed and classified as non-R0 resection. Postoperative chemoradiotherapy is recommended for these patients.
For rectal cancer patients with a preoperative clinical stage of cT1-2N0, we recommend laparoscopic or robot-assisted radical rectal resection. If postoperative pathology confirms negative surgical margins, it is defined as an R0 resection. For patients with a strong desire to preserve the anus, postoperative concurrent chemoradiotherapy is advised. Postoperative radiotherapy parameters are set at 50-54 Gy over 25-30 fractions. Postoperative chemotherapy regimens include single-agent chemotherapy, as well as combination regimens such as CAPEOX (XELOX) and mFOLFOX6. For rectal cancer patients with a preoperative clinical stage of cT3/cT4 or N+, we recommend radical rectal resection. If postoperative pathology confirms negative surgical margins, it is defined as an R0 resection. Adjuvant concurrent chemoradiotherapy is advised, with postoperative chemotherapy options including single-agent chemotherapy or combination regimens. For metastatic rectal cancer with isolated, resectable liver metastases, radical rectal resection combined with liver metastasectomy is performed. If postoperative pathology confirms negative surgical margins, it is defined as an R0 resection. Postoperative chemotherapy regimens include single-agent chemotherapy or combination regimens such as CAPEOX (XELOX) and mFOLFOX6. For metastatic rectal cancer assessed as unresectable but accompanied by acute symptoms such as bleeding, perforation, or obstruction, palliative surgery is performed. These cases are defined as non-R0 resections, and postoperative chemoradiotherapy is recommended.
2.4 Data collection and follow-up
The basic information and the clinicopathological data were collected from the prospective cancer database in our institution. Basic information included the patient’s name, ID, gender, age, tobacco use, alcohol consumption, and family history of cancer, while the clinicopathological data contains tumor location, R0 resection, tumor differentiation, TNM stage, operative method, surgical duration, intraoperative blood loss, postoperative chemoradiotherapy, preoperative CEA, and preoperative TTR. Tobacco use was categorized into three levels: non-smoker, former smoker, and smoker. Alcohol consumption was similarly classified into three levels: non-drinker, light-to-moderate drinker (daily alcohol intake less than 2 units or occasional drinkers), and heavy drinker (daily alcohol intake greater than 2 units). Tumor location was further specified as proximal colon (from cecum to colon splenic flexure), distal colon (from descending colon to sigmoid colon), and rectum (from rectal junction to rectum) according to the International Classification of Diseases for Oncology. The pathology of each tissue sample was independently assessed by two experienced pathologists who were blinded to the TTR status. The Eighth Edition of the American Joint Committee on Cancer (AJCC) Staging for colorectal cancer was applied to determine the TNM stage system.
The follow-up protocol for all patients at our institution included regular assessments, involving telephone or outpatient reviews every 3 months during the initial 2 years post-surgery and subsequent evaluations every 6 months. Overall survival (OS) was characterized as the duration from the surgery date to the date of death, whereas cancer-specific survival (CSS) was specified as the duration from the surgery date to the date of death from cancer. The final follow-up date for the training cohort in this study was March 31, 2020, while for the validation cohort, it extended until December 31, 2021.
2.5 Statistical analysis
All analyses were performed with IBM SPSS Statistics 22.0 software, R version 4.3.1, and the X-tile program. Differences in two-tailed P values <0.05 were considered statistically significant. The X-tile software was utilized to estimate the optimal cutoff values for TTR (30). Continuous variables were presented as medians with interquartile ranges, while categorical variables were expressed as absolute numbers or percentages. Categorical variable comparisons were performed using the χ2 test or Fisher’s exact test, ordinal data were analyzed with the Kruskal-Wallis test, and continuous variables were compared using the Mann-Whitney U test. Kaplan-Meier method was used to calculate the survival rate and draw the survival curve, with the Log-Rank test utilized for survival analysis. The risk factors for CSS were determined by Univariate and multivariate Cox proportional hazards regression analysis and expressed using hazard ratio (HR) with a 95% confidence interval (CI). Based on risk factors identified through multivariate Cox analysis, several postoperative CSS prediction nomograms were developed. The nomograms underwent internal validation using the bootstrap method. External validation was performed by validating 378 patients in the validation cohort to evaluate the performance of the nomograms. The receiver operating characteristic (ROC) curve, calibration curve, concordance index (C-index), and decision curve analysis (DCA) were used to evaluate the discrimination ability and prediction effect of nomograms (31).
3 Results
3.1 Cut-off value of TTR
We used two methods to determine the optimal cut-off value for TTR classification. The first method involved grouping patients based on the lower normal value of 100 mg/L found in the literature, dividing the 1,322 colorectal cancer (CRC) patients into Group A (TTR < 100 mg/L) and Group B (TTR ≥ 100 mg/L). The second method used the X-tile software to determine the optimal cut-off value, categorizing the 1,322 CRC patients into Group C (TTR < 121.3 mg/L) and Group D (TTR ≥ 121.3 mg/L). The relationship between these two grouping methods and the cancer-specific survival is shown in Table 1. The results indicated that the hazard ratio (HR) for the second grouping method was 0.31(0.24-0.40), while the HR for the first grouping method was 0.35(0.25-0.48). Clearly, the most appropriate cut-off value of TTR for predicting cancer-specific survival (CSS) in CRC patients was determined to be 121.3 mg/L, as established by the X-tile software in the training cohort (Figure 2). Consequently, the 1,322 consecutive CRC patients were stratified into two groups: the TTR-lower group (TTR < 121.3 mg/L) and the TTR-higher group (TTR ≥ 121.3 mg/L).
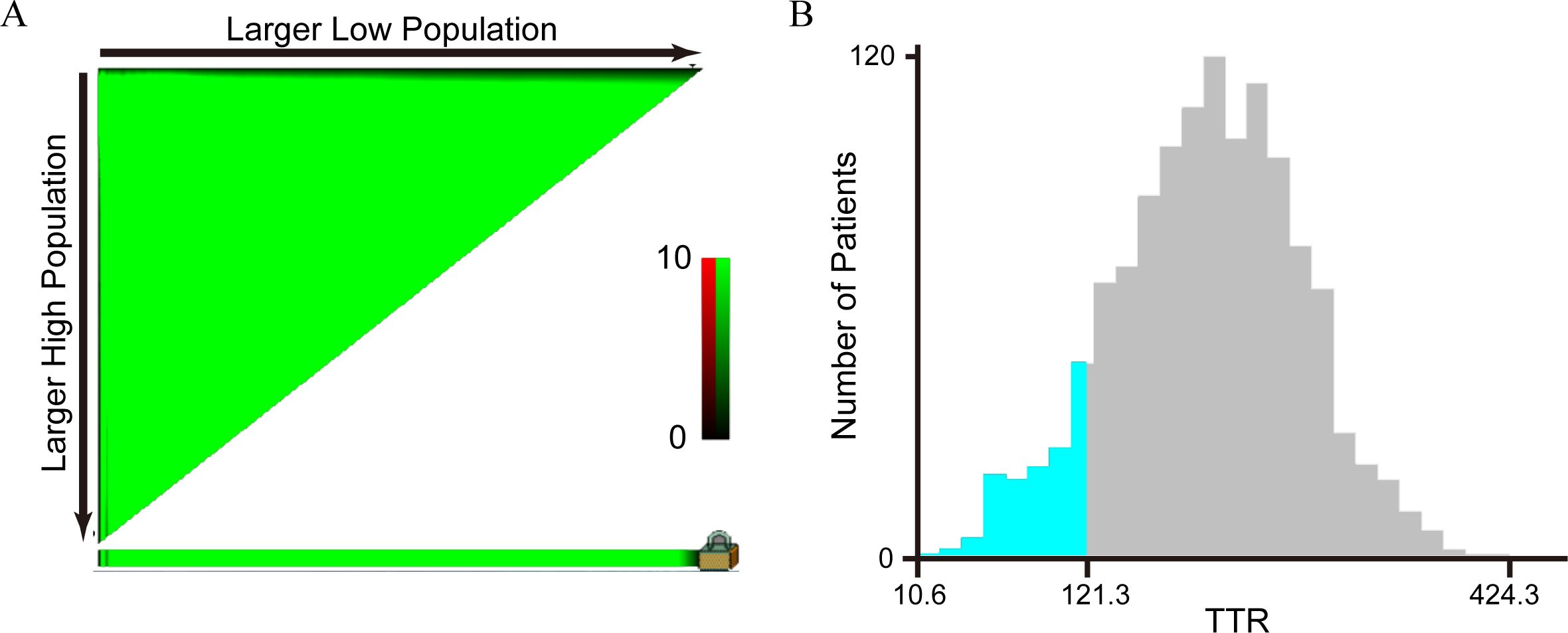
Figure 2. Analysis of the optimal cut-off value of TTR by X-tile software. (A) An X-tile plot of TTR; (B) The optimal cut-off value was highlighted by a histogram. The optimal cut-off value for TTR was 121.3 mg/L.
3.2 Transthyretin and clinicopathological features
Table 2 provides a comprehensive overview of the characteristics of all included patients and shows the division of the 1322 CRC patients. 133 patients (10.1%) were classified into the TTR-lower group, while 1189 (89.9%) were assigned to the TTR-higher group. In the TTR-lower group, the median age of the CRC patients was 66.0 years, which was older than the median age of 62.0 years observed in the TTR-higher group (p=0.004). Similar trends were observed in preoperative CEA levels, with the median CEA in the TTR-lower group being 4.74μg/L, significantly higher than the 3.39μg/L in the TTR-higher group (p=0.022). Furthermore, TTR was significantly associated with gender (p=0.032), location (p<0.001), R0 resection (p<0.001), differentiation (p=0.004) and stage (p<0.001). However, no significant differences were observed in tobacco use, alcohol consumption, family history of cancer, operative method, surgical duration, intraoperative blood loss and postoperative chemoradiotherapy (p>0.05). The basic characteristics of the validation cohort are shown in Supplementary Table 1. Additionally, we collected liver function parameters of the 1,322 CRC patients, as shown in Table 3. The results indicated that the TTR-lower group had slightly lower ALT and slightly higher GLOB levels compared to the TTR-higher group. However, the quartiles and medians of liver function parameters for both groups remained within the clinically normal range, suggesting that patients in the TTR-lower group did not exhibit significant liver dysfunction or impaired hepatic secretory function.
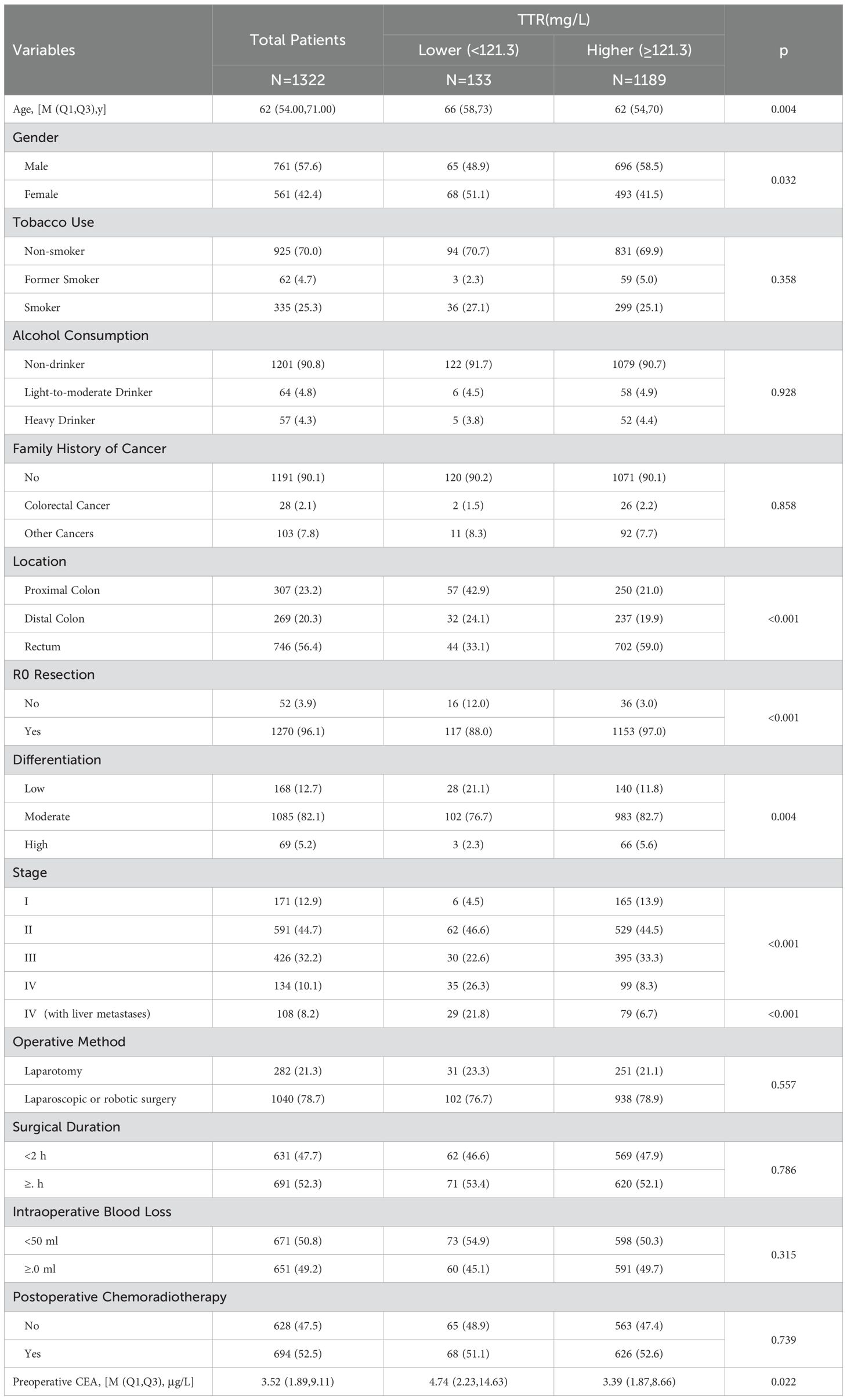
Table 2. Characteristics and clinicopathologic features of 1322 colorectal patients according to preoperative transthyretin levels.
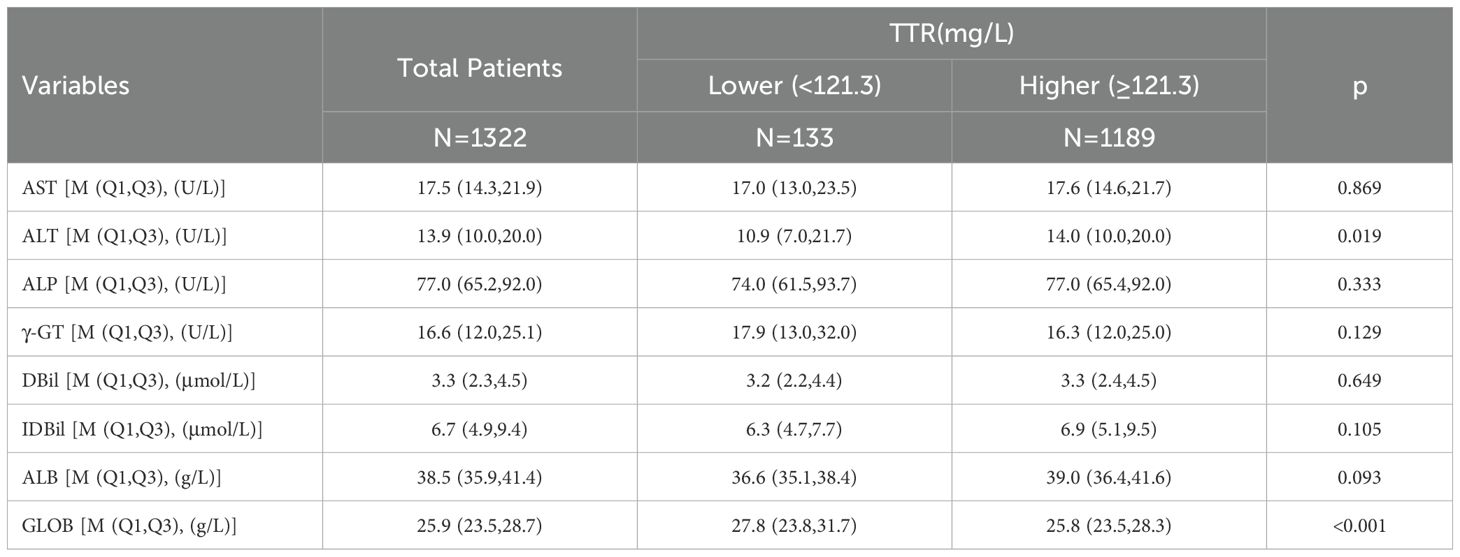
Table 3. Liver function parameters of 1322 colorectal patients according to preoperative transthyretin levels.
3.3 Associations between transthyretin level and patient survival
By the end of the follow-up period, a total of 317 deaths were observed in 1322 patients, including 298 cancer-specific deaths. The cumulative 1-year, 3-year, and 5-year of colorectal cancer-specific survival (CSS) rates in the TTR-lower group were 72.2%, 50.2%, and 41.0%, respectively. These were significantly shorter than the corresponding rates in the TTR-higher group, which were 92.3%, 81.1%, and 75.3%, respectively (p < 0.001 for all comparisons). Similar trends were observed in overall survival (OS), with the cumulative 1-year, 3-year, and 5-year OS rates in the TTR-lower group and TTR-higher group being 72.2% vs 92.3%, 50.2% vs 80.4%, and 40.0% vs 72.5% (p < 0.001 for all comparisons). The Kaplan-Meier survival estimate also indicated that the TTR-low group exhibited significantly worse OS and CSS than the TTR-high group (Figure 3).
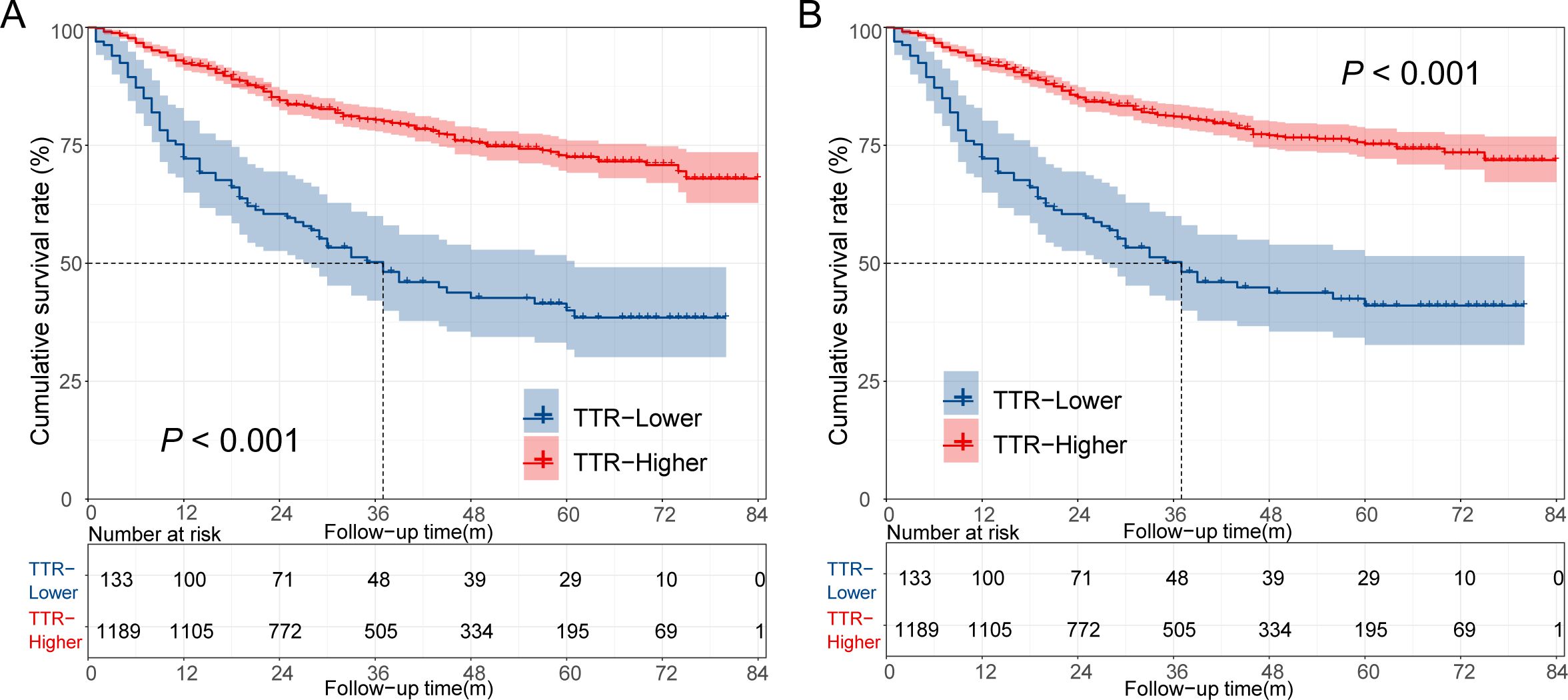
Figure 3. Overall survival curve (A) and cancer-specific survival curve (B) for different TTR levels in CRC patients underwent surgical treatment.
Additionally, we conducted a stratified analysis based on the age and gender of the patients, dividing them into several subgroups. In patients aged ≤60 years, the TTR-higher group exhibited a more favorable prognosis compared to the TTR-lower group, with both the 5-year overall survival (OS) and cancer-specific survival (CSS) rates being higher in the TTR-high group than in the TTR-low group. (81.8%vs 52.5%, 81.8% vs 55.3%, p < 0.001 for both). Comparable conclusions were observed within the subgroups aged >60 years, male, and female, indicating a consistent trend across these demographic subsets (Figure 4).
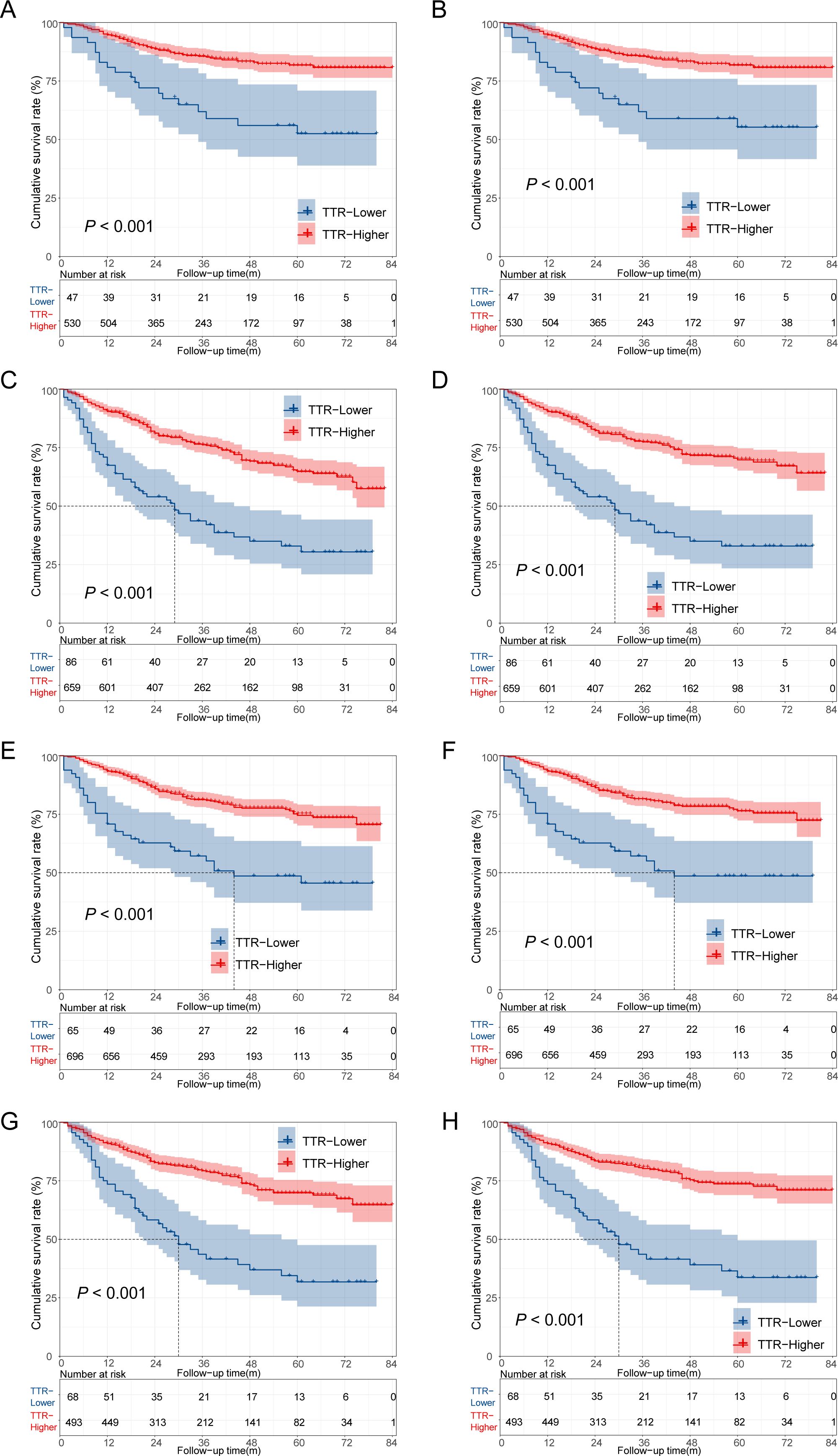
Figure 4. Kaplan–Meier survival analysis of colorectal cancer patients who underwent surgery. (A) The overall survival of CRC patients aged ≤60 years; (B) The cancer-specific survival of CRC patients aged ≤60 years; (C) The overall survival of CRC patients aged >60 years; (D) The cancer-specific survival of CRC patients aged >60 years; (E) The overall survival of male CRC patients; (F) The cancer-specific survival of male CRC patients; (G) The overall survival of female CRC patients; (H) The cancer-specific survival of female CRC patients.
3.4 Prognostic factors analysis for postoperative colorectal cancer-specific mortality
To identify the most appropriate variables for constructing a postoperative survival predictive model, we utilized a Cox proportional hazards regression model. In the univariate Cox regression analyses, age, gender, tobacco use, location, R0 resection, differentiation, stage, postoperative chemoradiotherapy, preoperative CEA, and TTR were identified as factors significantly affecting the prognosis of colorectal cancer patients undergoing surgery (all p <0.05) (Figure 5).
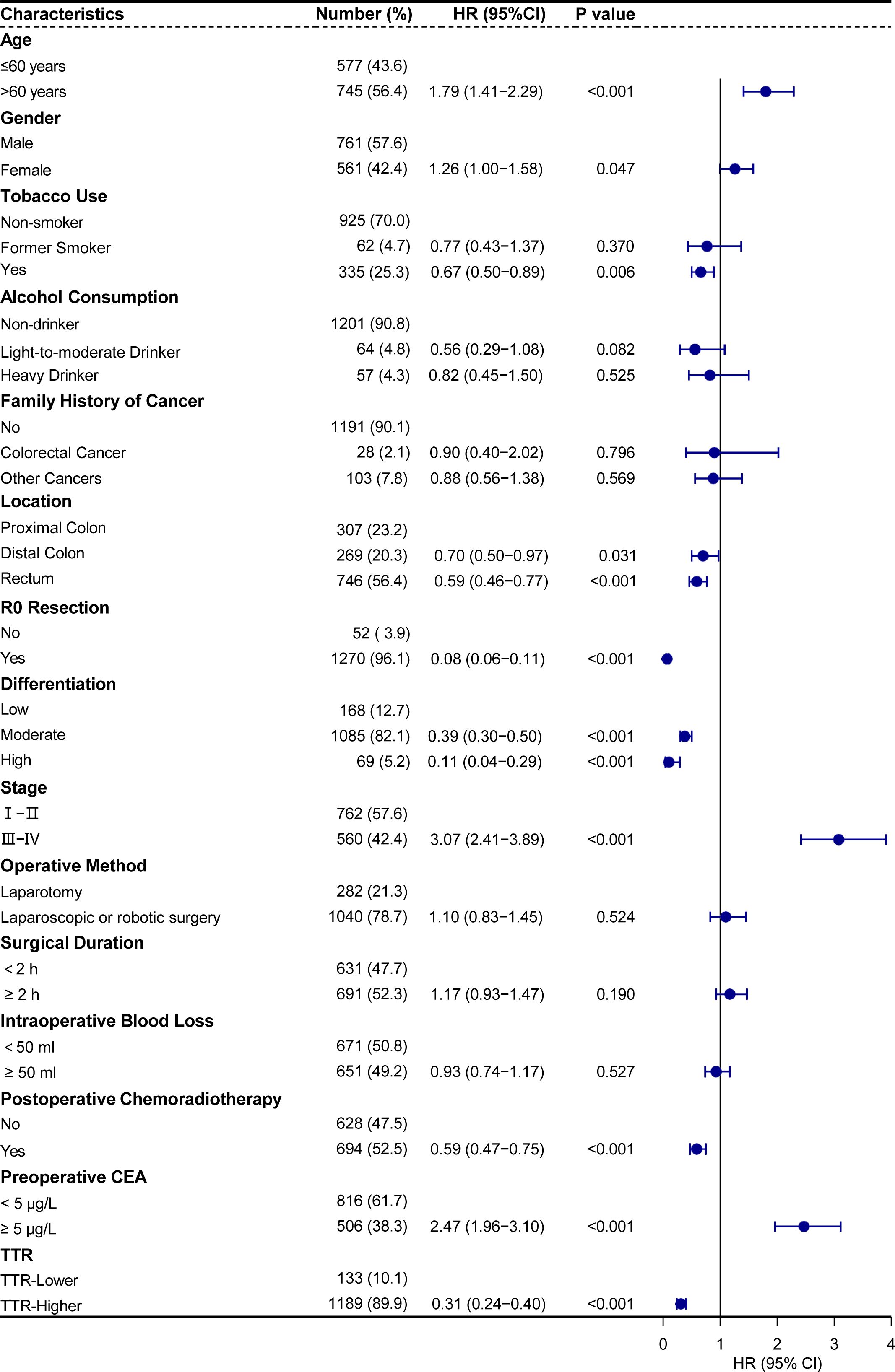
Figure 5. Univariate analysis of cancer-specific survival showed that TTR and other clinicopathological parameters were significant prognostic factors.
In further multivariate Cox regression analyses, age>60 years (hazard ratio [HR] = 1.60, 95% confidence interval [CI] : 1.25–2.06, p<0.001), stage III-IV(HR = 2.34, 95% CI: 1.79–3.04, p<0.001), preoperative CEA≥5 (HR = 2.04, 95% CI: 1.62–2.58, p<0.001) were identified as risk factors for postoperative cancer-specific mortality. Conversely, tumor located in the distal colon (HR = 0.71, 95% CI: 0.51–0.99, p = 0.043), located in the rectum (HR = 0.76, 95% CI: 0.58–0.99, p = 0.045), R0 resection (HR = 0.19, 95% CI: 0.13–0.27, p < 0.001), moderate differentiation (HR = 0.52, 95% CI: 0.40–0.69, p < 0.001), high differentiation (HR = 0.22, 95% CI: 0.08–0.60, p = 0.003), postoperative chemoradiotherapy (HR = 0.55, 95% CI: 0.43–0.69, p < 0.001), and higher TTR (HR = 0.45, 95% CI: 0.34–0.60, p < 0.001) were identified as protective factors for postoperative cancer-specific mortality (Figure 6).
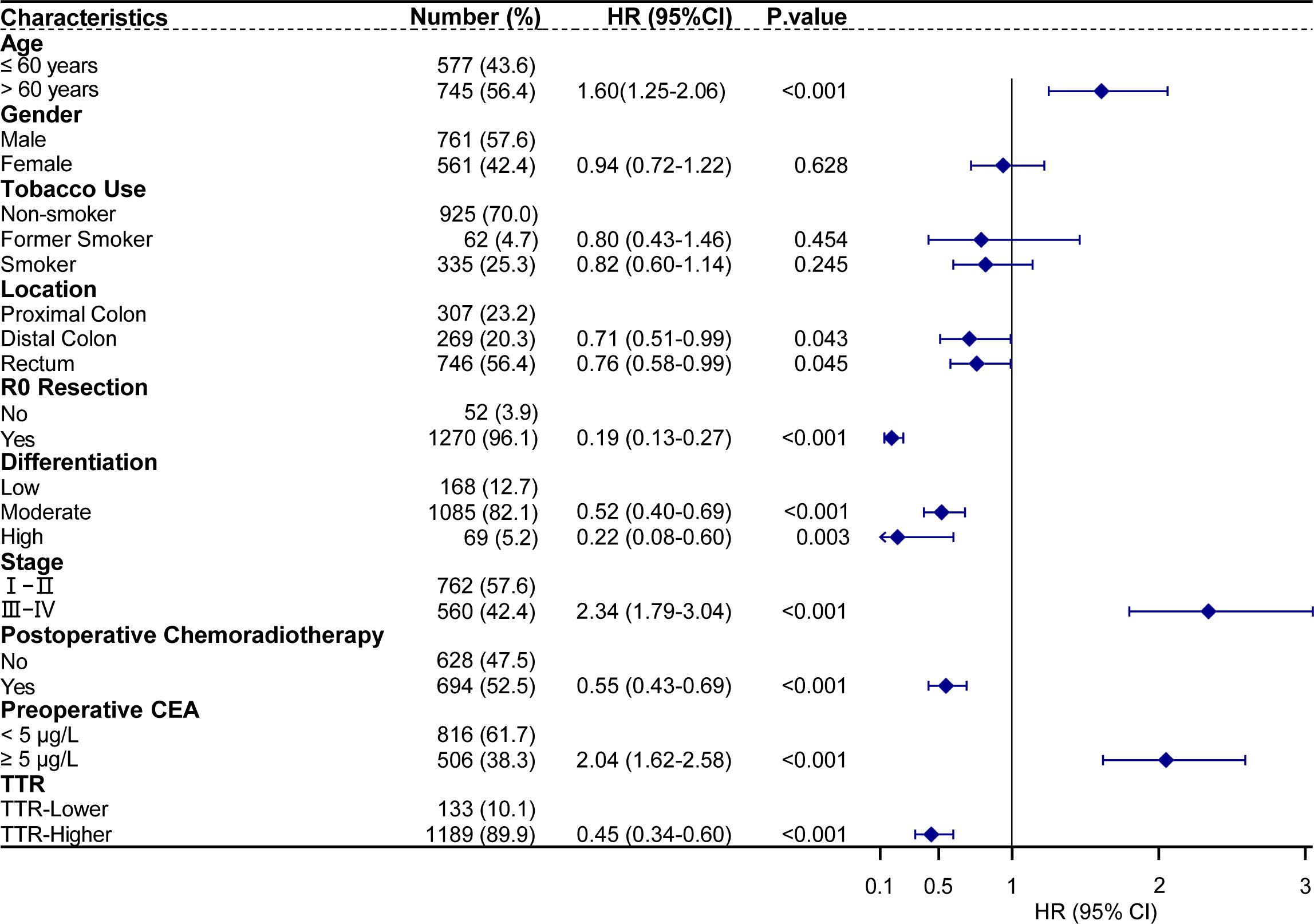
Figure 6. Multivariate analysis of prognostic factors with cancer-specific survival in 1322 CRC patients underwent surgical treatment.
3.5 Construction and internal validation of cancer-specific mortality prediction models
We established Model A based on multivariate Cox regression analysis and accordingly could predict the 1-year, 3-year, and 5-year CSS of CRC patients (Figure 7A). The 1-year, 3-year and 5-year AUC predicted by Model A were 0.824 (95%CI: 0.785-0.863), 0.821 (95%CI: 0.791-0.852) and 0.797 (95%CI: 0.757-0.837) respectively, which exhibited its robust predictive performance. The C-index for Model A was 0.795 (95%CI: 0.769-0.821), underscoring its excellence as a classifier with high accuracy, particularly in short-term postoperative survival prediction (Figure 7B). Additionally, calibration curves for 1-year, 3-year, and 5-year intervals demonstrated the model’s strong calibration and discrimination capabilities (Figure 7C). Compared to the TNM staging used by the most common AJCC in clinical practice, model A consistently displayed higher AUC values at any given time point within the first 5 years post-surgery (Supplementary Figure 1).
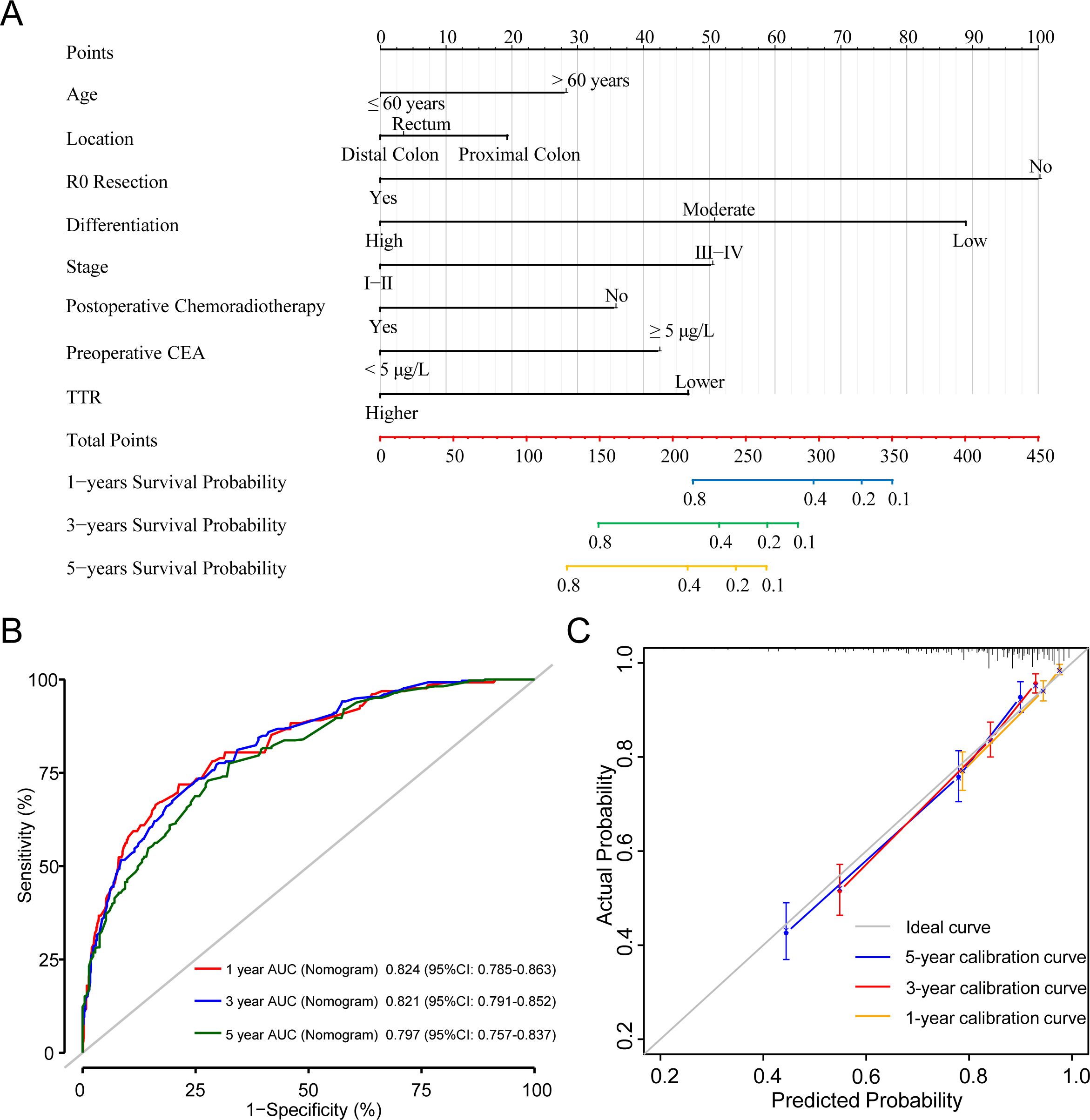
Figure 7. A Cox proportional hazards Model A of cancer specific mortality for CRC patients underwent surgical treatment. (A) The nomogram of Model A. (B) 1-, 3-, and 5-year ROC comparison of Model A. (C) 1-, 3-, and 5-year calibration curves of Model A.
3.6 Comparison and external validation of mortality prediction models
In addition to Model A, we introduced Model B and Model C into our analysis. Model B incorporated all parameters that were significant in the multivariate Cox regression analysis without preoperative TTR level, while Model C was established only containing the TNM stage and tumor differentiation (Figure 8). The 1-year,3-year, and 5-year ROC of the three models are shown in Supplementary Figure 2, revealing that Model A exhibited the highest AUC. Additionally, the time-ROC curve analysis of the three models indicated that the AUC of Model A for predicting postoperative CSS in colorectal cancer is the highest across all periods (Supplementary Figure 3). The DCA curve shows that compared to Model B and Model C, the most effective decision-making results can be obtained when utilizing the Model A to determine the prognosis of colorectal cancer patients undergoing surgery (Supplementary Figure 4). In the external cohort validation comprising 377 individuals, ROC curves, calibration plots, and Decision Curve Analysis (DCA) plots consistently revealed similar results, emphasizing the distinctive superiority of Model A in predicting the prognosis of colorectal cancer patients undergoing surgery (Supplementary Figures 5–7).
4 Discussion
Colorectal cancer constitutes a global public health challenge that demands comprehensive solutions. This malignancy not only poses a severe threat to human health but also imposes significant burdens on families and society at large. The considerable variability in prognosis and survival among patients with the same cancer type is attributed to the diverse biological behaviors of tumors. The identification of meaningful prognostic factors and the development of predictive models serve multifaceted purposes. They not only aid patients in formulating personalized treatment strategies based on scientific evidence but also play a pivotal role in preventing overtreatment and conserving valuable medical resources. Furthermore, these efforts assist patients in planning their remaining lives and contribute to enhancing their sense of life dignity.
In this clinical study, we explored the relationship between TTR and clinical outcomes in colorectal patients undergoing surgery through a training cohort of 1322 patients. In the process of patient recruitment, we excluded patients over 80 years old for the consideration of cardiovascular accidents, stroke, depression, lung disease, and other factors that can affect OS, and we were more concerned about the impact of TTR on CSS. The findings indicated a correlation between reduced TTR levels and unfavorable postoperative prognosis, proposing TTR as a potential marker for predicting adverse outcomes in colorectal cancer surgery patients. These results align with studies on other digestive tract tumors like gastric and liver cancers (26, 27). We then conducted Cox regression analysis on the training cohort to identify risk factors for CSS of CRC. Subsequently, based on the results of multivariate Cox analysis, we developed a prognostic nomogram. Additionally, several nomograms were constructed based on various clinical-pathological information. The outcomes of internal validation using the bootstrap method demonstrated a strong concordance between predicted and observed results, which was supported by robust ROC curve analysis, a C-index exceeding 0.75, and a well-fitted calibration curve, indicating substantial discriminatory ability. Furthermore, the external validation was also conducted in the validation cohort of 377 patients, which indicates the high accuracy of CSS predictions for 1-, 3- and 5-year periods. The DCA demonstrated that the nomogram we developed was superior to other models, which means the nomogram possesses substantial clinical practical value (32). This emphasizes the enhanced prognostic accuracy achieved through the incorporation of TTR in the predictive model.
Malignant tumors are recognized as chronic, consumptive diseases, particularly within the digestive system, exerting a substantial and notable impact on nutrient depletion and weight loss. The stress and trauma associated with major surgical procedures exacerbate nutrient catabolism, leading to inadequate nutritional reserves and slower postoperative recovery. Some studies indicate that malnutrition can impact the postoperative recovery of surgical patients, leading to unfavorable outcomes (33). Therefore, preoperative nutritional markers deserve attention and should not be overlooked as they play a crucial role in evaluating the prognostic status of patients. Many nutritional indicators have been considered for clinical practice, including BMI, albumin, and prognostic nutritional index (PNI) (34). However, different nutritional indicators have their scope of application and limitations. BMI focuses on weight, but it ignores factors that affect one person’s health, including gender, age, and ethnicity (35). In other literature, a series of indicators based on albumin have been used to predict the prognosis of cancer, including PNI and nutrition risk index. Some researchers insisted that they may be useful indicators in the prognosis of digestive system neoplasms (36, 37). However, other scholars have argued that the prognostic significance of indicators based on albumin was not significant in patients with early-stage gastric cancer (38).
TTR is nowadays often preferred over albumin, given its shorter half-life of 2 days, significantly shorter than the 3 weeks of albumin (39). This characteristic allows TTR to capture more rapid changes in the nutritional state compared to other indicators. Furthermore, an epidemiological survey in Australia showed that TTR is not affected by racial differences or genetic factors, suggesting that it can be used as a more sensitive and reliable indicator for evaluating liver function and recent nutritional status than albumin or PNI (40). It has been widely confirmed that serial measurement of TTR allows monitoring of fluctuations in lean body mass (LBM) depletion and predicting the outcome of critically ill patients, including burns, polytrauma, septicemic, or neoplastic invasion (41, 42). Some research institutions in Europe and East Asia have suggested that TTR should be used as a routine nutritional monitoring indicator (24, 43–47). Therefore, TTR has been employed to predict the occurrence of surgical complications, differentiate between inflammation and malignancy, and predict the prognosis of patients diagnosed with malignant tumors. This underscores its potential utility as a marker in oncology.
The normal TTR concentration in adult males is stable at approximately 310 mg/L, while it is approximately 260 mg/L in adult females (39). Different researchers have chosen different TTR concentrations as the cut-off value in their studies. In a clinical study around non-small cell lung cancer, researchers chose 220 mg/L as the cutoff value for TTR, and patients below this level would have poorer disease-free survival (28). The researchers selected 200 mg/L as the optimal cut-off value based on the most prominent points on the ROC curves and verified that the preoperative TTR was a prognostic factor in patients with adenocarcinoma of the esophagogastric junction (48). The X-tile program identified 180 mg/L as the TTR cut-off value, revealing that TTR levels serve as a more sensitive index of nutritional change and superior indicators of prognosis compared to albumin levels in stage II/III gastric cancer (49). In a clinical trial studying hepatocellular carcinoma, researchers used a cut-off value of 170 mg/L for preoperative prealbumin level and confirmed that preoperative prealbumin level could be used in predicting long-term prognosis for patients undergoing liver resection (50). In adult subjects with other diseases, a threshold of 100 mg/L has been associated with ominous prognostic significance, potentially indicating the depletion of Lean Body Mass (LBM) resources (51, 52). The range of cut-off values from 200 mg/L to 100 mg/L delineates a gray zone within which TTR concentrations may fluctuate, indicating potential outcomes ranging from the best to the worst. The chosen cut-off value for TTR in our study was 121.3 mg/L, falling within the range of 100 mg/L to 200 mg/L, but lower than values ranging from 170 mg/L to 220 mg/L. The distinction in cut-off values can be attributed to the differential impact of colorectal cancer and gastric cancer on physiological functions. Colorectal cancer predominantly affects absorption function, resulting in symptoms such as diarrhea and loss of intestinal fluid. In contrast, gastric cancer primarily interferes with the stomach’s ability to grind food. Meanwhile, colorectal cancer might be detected at more advanced stages, leading to more severe nutritional deficiencies by the time of diagnosis compared to gastric cancer, which might be detected earlier. Despite slight variations in the cut-off value utilized within our study, the results consistently indicate a strong association between preoperative TTR and long-term prognosis.
While the exact reasons behind the robust connection between low TTR levels and the unfavorable prognosis of CRC patients remain unclear, potential mechanisms may be attributed to the following factors: Firstly, the initiation and progression of colorectal cancer are associated with an imbalance in the microecology of the digestive tract. Harmful strains such as Fusobacterium nucleatum and Bacteroides fragilis play a role in activating the NF-κB signaling pathway. This activation results in the secretion of cytokines, including tumor necrosis factor-alpha (TNF-α), interleukin-1 (IL-1), and IL-6, leading to inflammation which may further exacerbates the development of tumors (53). At the same time, these inflammatory factors are absorbed by the mucosa and enter the portal vein system into the liver, inhibiting the synthesis of prealbumin in an inflammatory state (54). Secondly, TTR carries retinoic acid by transporting retinol-binding protein. Research indicates that retinoic acid plays a crucial role in inhibiting the occurrence and development of colon cancer (55, 56). The decrease in TTR produced by the liver results in a reduction in the blood’s retinoic acid content, diminishing its inhibitory effect on cancer progression and potentially contributing to the rapid development of colorectal cancer. Furthermore, TTR has demonstrated potential roles in immune system regulation by influencing the differentiation of myeloid cells, thus modulating the tumor environment and controlling immune cell function (57).
There are several deficiencies and limitations in our clinical study. Above all, it is essential to acknowledge that this clinical study is a non-randomized, observational, and retrospective study, with patient selection limited to a single research center; the strict exclusion criteria resulted in a considerable loss of many patients, potentially introducing some selection bias. Secondly, it has become a consensus that patients with advanced colorectal cancer should undergo neoadjuvant chemoradiotherapy before surgery in recent years. However, the patients in this study came from several years ago, when the concept of neoadjuvant therapy was not widely accepted; meanwhile, although neoadjuvant chemoradiotherapy can benefit patients by reducing tumor staging, it inevitably causes side effects, such as damage to liver function and affecting food intake, which in turn affects preoperative TTR levels. Therefore, this study does not apply to the prognosis prediction of patients with colorectal cancer undergoing neoadjuvant therapy. Thirdly, owing to the limited number of patients, and the majority originating from the northwest region of China, there may be a homogeneity in the genotype of CRC patients, potentially influencing the prognosis. Whether the results in different periods, different regions, or different populations are consistent with the model has not been verified. Therefore, we expect a multicenter, large sample cohort to validate our results to confirm its prognostic role in colorectal cancer patients in the future.
5 Conclusion
Our study showed that a preoperative TTR < 121.3 mg/L emerged as a prognostic factor, predicting the long-term outcomes of CRC patients following surgical intervention. The predictive nomogram established on the basis of TTR exhibited robust predictive efficacy, as confirmed through both internal and external validation, supporting its role as a prognostic biomarker in clinical practice and offering valuable guidance for patient management.
Data availability statement
The original contributions presented in the study are included in the article/Supplementary Material. Further inquiries can be directed to the corresponding author.
Ethics statement
The studies involving humans were approved by The Institutional Review Board and Ethical Committee of the First Affiliated Hospital of Xi’an Jiaotong University approved this study (XJTU1AF2019LSK−227). The studies were conducted in accordance with the local legislation and institutional requirements. The participants provided their written informed consent to participate in this study.
Author contributions
ZZ: Conceptualization, Data curation, Formal analysis, Investigation, Methodology, Project administration, Resources, Software, Writing – original draft, Writing – review & editing, Visualization. CH: Formal analysis, Investigation, Methodology, Writing – review & editing, Data curation. LZ: Data curation, Investigation, Methodology, Writing – review & editing. FS: Investigation, Writing – review & editing, Supervision. YW: Investigation, Writing – review & editing. YZ: Investigation, Writing – review & editing. XZ: Investigation, Writing – review & editing. JS: Funding acquisition, Project administration, Resources, Supervision, Writing – review & editing, Validation.
Funding
The author(s) declare financial support was received for the research, authorship, and/or publication of this article. This work was supported by the National Natural Science Foundation of China (No. 82173394) and the Shaanxi Province Science Foundation (2023-GHYB-13).
Conflict of interest
The authors declare that the research was conducted in the absence of any commercial or financial relationships that could be construed as a potential conflict of interest.
Publisher’s note
All claims expressed in this article are solely those of the authors and do not necessarily represent those of their affiliated organizations, or those of the publisher, the editors and the reviewers. Any product that may be evaluated in this article, or claim that may be made by its manufacturer, is not guaranteed or endorsed by the publisher.
Supplementary material
The Supplementary Material for this article can be found online at: https://www.frontiersin.org/articles/10.3389/fonc.2025.1397019/full#supplementary-material
Abbreviations
TTR, Transthyretin; CRC, Colorectal Cancer; AJCC, the American Joint Committee on Cancer; OS, Overall Survival; CSS, Cancer-specific Survival; CD, Cluster of Differentiation; CEA, Carcinoembryonic Antigen; AUC, Area Under Curve; ROC, Receiver Operating Characteristic Curve; DCA, Decision Curve Analysis; TNM, tumor-node-metastasis; BMI, Body Mass Index; HR, Hazard Ratio; OR, Odds Ratio; C-index, Concordance Index; CI, Confidence Interval; PNI, Prognostic Nutritional Index; AST, Aspartate Aminotransferase; ALT, Alanine Aminotransferase; ALP, Alkaline Phosphatase; γ-GT, γ-Glutamyl Transpeptidase; DBil, Direct Bilirubin; IDBil, Indirect Bilirubin; ALB, Albumin; GLOB, Globulin.
References
1. Sung H, Ferlay J, Siegel RL, Laversanne M, Soerjomataram I, Jemal A, et al. Global cancer statistics 2020: GLOBOCAN estimates of incidence and mortality worldwide for 36 cancers in 185 countries. Ca-a Cancer J Clin. (2021) 71:209–49. doi: 10.3322/caac.21660
2. Collaborators GBDCC. The global, regional, and national burden of colorectal cancer and its attributable risk factors in 195 countries and territories, 1990-2017: a systematic analysis for the Global Burden of Disease Study 2017. Lancet Gastroenterol Hepatol. (2019) 4:913–33. doi: 10.1016/S2468-1253(19)30345-0
3. Collaborators GBDCC. Global, regional, and national burden of colorectal cancer and its risk factors, 1990-2019: a systematic analysis for the Global Burden of Disease Study 2019. Lancet Gastroenterol Hepatol. (2022) 7:627–47. doi: 10.1016/S2468-1253(22)00044-9
4. Mlecnik B, Torigoe T, Bindea G, Popivanova B, Xu M, Fujita T, et al. Clinical performance of the consensus immunoscore in colon cancer in the asian population from the multicenter international SITC study. Cancers (Basel). (2022) 14. doi: 10.3390/cancers14184346
5. Bohlok A, Inchiostro L, Lucidi V, Vankerckhove S, Hendlisz A, Van Laethem J, et al. Tumor biology reflected by histological growth pattern is more important than surgical margin for the prognosis of patients undergoing resection of colorectal liver metastases. Eur J Surg Oncol. (2023) 49:217–24. doi: 10.1016/j.ejso.2022.08.006
6. Joanito I, Wirapati P, Zhao N, Nawaz Z, Yeo G, Lee F, et al. Single-cell and bulk transcriptome sequencing identifies two epithelial tumor cell states and refines the consensus molecular classification of colorectal cancer. Nat Genet. (2022) 54:963–75. doi: 10.1038/s41588-022-01100-4
7. Wang F, Lu S, Cao D, Qian J, Li C, Zhang R, et al. Prognostic and predictive value of Immunoscore and its correlation with ctDNA in stage II colorectal cancer. Oncoimmunology. (2023) 12:2161167. doi: 10.1080/2162402X.2022.2161167
8. Sedlak JC, Yilmaz OH, Roper J. Metabolism and colorectal cancer. Annu Rev Pathol. (2023) 18:467–92. doi: 10.1146/annurev-pathmechdis-031521-041113
9. Braoudaki M, Ahmad MS, Mustafov D, Seriah S, Siddiqui MN, Siddiqui SS. Chemokines and chemokine receptors in colorectal cancer; multifarious roles and clinical impact. Semin Cancer Biol. (2022) 86:436–49. doi: 10.1016/j.semcancer.2022.06.002
10. Wang G, Fu S, Li D, Chen Y. Expression and clinical significance of serum NT5E protein in patients with colorectal cancer. Cancer biomark. (2019) 24:461–8. doi: 10.3233/CBM-182207
11. Jin LJ, Chen WB, Zhang XY, Bai J, Zhao HC, Wang ZY. Analysis of factors potentially predicting prognosis of colorectal cancer. World J Gastrointest Oncol. (2019) 11:1206–17. doi: 10.4251/wjgo.v11.i12.1206
12. El Asmar A, Delcourt M, Kamden L, Khaled C, Bohlok A, Moreau M, et al. Prognostic value of preoperative serological biomarkers in patients undergoing curative-intent cytoreductive surgery for colorectal cancer peritoneal metastases. Ann Surg Oncol. (2023) 30:1863–9. doi: 10.1245/s10434-022-12736-1
13. Tagai N, Goi T, Shimada M, Kurebayashi H. Plasma Prokineticin 1, a prognostic biomarker in colorectal cancer patients with curative resection: a retrospective cohort study. World J Surg Oncol. (2021) 19:302. doi: 10.1186/s12957-021-02421-0
14. Montalban-Hernandez K, Cantero-Cid R, Lozano-Rodriguez R, Pascual-Iglesias A, Avendano-Ortiz J, Casalvilla-Duenas JC, et al. Soluble SIGLEC5: A new prognosis marker in colorectal cancer patients. Cancers (Basel). (2021) 13. doi: 10.3390/cancers13153896
15. Ponomaryova AA, Rykova EY, Solovyova AI, Tarasova AS, Kostromitsky DN, Dobrodeev AY, et al. Genomic and transcriptomic research in the discovery and application of colorectal cancer circulating markers. Int J Mol Sci. (2023) 24. doi: 10.3390/ijms241512407
16. Magalhaes J, Eira J, Liz MA. The role of transthyretin in cell biology: impact on human pathophysiology. Cell Mol Life Sci. (2021) 78:6105–17. doi: 10.1007/s00018-021-03899-3
17. Maurer MS, Elliott P, Merlini G, Shah SJ, Cruz MW, Flynn A, et al. Design and rationale of the phase 3 ATTR-ACT clinical trial (Tafamidis in transthyretin cardiomyopathy clinical trial). Circ Heart Fail. (2017) 10. doi: 10.1161/CIRCHEARTFAILURE.116.003815
18. Kittleson MM, Maurer MS, Ambardekar AV, Bullock-Palmer RP, Chang PP, Eisen HJ, et al. Cardiac amyloidosis: evolving diagnosis and management: A scientific statement from the American heart association. Circulation. (2020) 142:e7–e22. doi: 10.1161/CIR.0000000000000792
19. Griffin JM, Rosenthal JL, Grodin JL, Maurer MS, Grogan M, Cheng RK. ATTR amyloidosis: current and emerging management strategies: JACC: cardioOncology state-of-the-art review. JACC CardioOncol. (2021) 3:488–505. doi: 10.1016/j.jaccao.2021.06.006
20. Gasparotti R, Salvalaggio A, Corbo D, Agazzi G, Cacciavillani M, Lozza A, et al. Magnetic resonance neurography and diffusion tensor imaging of the sciatic nerve in hereditary transthyretin amyloidosis polyneuropathy. J Neurol. (2023) 270(10):4827–40. doi: 10.1007/s00415-023-11813-z
21. Nakajima N. Differential diagnosis of cachexia and refractory cachexia and the impact of appropriate nutritional intervention for cachexia on survival in terminal cancer patients. Nutrients. (2021) 13. doi: 10.3390/nu13030915
22. He F, Huang H, Xu W, Cui K, Ruan Y, Guo Y, et al. Prognostic impact of malnutrition in patients with coronary artery disease: a systematic review and meta-analysis. Nutr Rev. (2023) 82(8):1013–27. doi: 10.1093/nutrit/nuad108
23. Hu X, Deng H, Wang Y, Chen L, Gu X, Wang X. Predictive value of the prognostic nutritional index for the severity of coronavirus disease 2019. Nutrition. (2021) 84:111123. doi: 10.1016/j.nut.2020.111123
24. Godala M, Gaszynska E, Walczak K, Malecka-Wojciesko E. Evaluation of albumin, transferrin and transthyretin in inflammatory bowel disease patients as disease activity and nutritional status biomarkers. Nutrients. (2023) 15. doi: 10.3390/nu15153479
25. Cramer DW, Bast RC Jr., Berg CD, Diamandis EP, Godwin AK, Hartge P, et al. Ovarian cancer biomarker performance in prostate, lung, colorectal, and ovarian cancer screening trial specimens. Cancer Prev Res (Phila). (2011) 4:365–74. doi: 10.1158/1940-6207.CAPR-10-0195
26. Shimura T, Shibata M, Gonda K, Okayama H, Saito M, Momma T, et al. Serum transthyretin level is associated with prognosis of patients with gastric cancer. J Surg Res. (2018) 227:145–50. doi: 10.1016/j.jss.2018.02.035
27. Luo H, Huang J, Zhu Z, Zhu P. Prognostic value of pretreatment serum transthyretin level in patients with gastrointestinal cancers. Dis Markers. (2019) 2019:7142065. doi: 10.1155/2019/7142065
28. Shimura T, Shibata M, Inoue T, Owada-Ozaki Y, Yamaura T, Muto S, et al. Prognostic impact of serum transthyretin in patients with non-small cell lung cancer. Mol Clin Oncol. (2019) 10:597–604. doi: 10.3892/mco.2019.1837
29. Zhang Z, Qin K, Zhang W, Yang B, Zhao C, Zhang X, et al. Postoperative recurrence of epithelial ovarian cancer patients and chemoresistance related protein analyses. J Ovarian Res. (2019) 12:29. doi: 10.1186/s13048-019-0499-z
30. Camp RL, Dolled-Filhart M, Rimm DL. X-tile: a new bio-informatics tool for biomarker assessment and outcome-based cut-point optimization. Clin Cancer Res. (2004) 10:7252–9. doi: 10.1158/1078-0432.CCR-04-0713
31. Vickers AJ, Elkin EB. Decision curve analysis: a novel method for evaluating prediction models. Med Decis Making. (2006) 26:565–74. doi: 10.1177/0272989X06295361
32. Kerr KF, Brown MD, Zhu K, Janes H. Assessing the clinical impact of risk prediction models with decision curves: guidance for correct interpretation and appropriate use. J Clin Oncol. (2016) 34:2534–40. doi: 10.1200/JCO.2015.65.5654
33. Weimann A, Braga M, Carli F, Higashiguchi T, Hubner M, Klek S, et al. ESPEN practical guideline: Clinical nutrition in surgery. Clin Nutr. (2021) 40:4745–61. doi: 10.1016/j.clnu.2021.03.031
34. Keller U. Nutritional laboratory markers in malnutrition. J Clin Med. (2019) 8. doi: 10.3390/jcm8060775
35. Visaria A, Setoguchi S. Body mass index and all-cause mortality in a 21st century U.S. population: A National Health Interview Survey analysis. PloS One. (2023) 18:e0287218. doi: 10.1371/journal.pone.0287218
36. Li J, Zhu N, Wang C, You L, Guo W, Yuan Z, et al. Preoperative albumin-to-globulin ratio and prognostic nutritional index predict the prognosis of colorectal cancer: a retrospective study. Sci Rep. (2023) 13:17272. doi: 10.1038/s41598-023-43391-5
37. Qian W, Xiao-Jian J, Jun H, Liang L, Xiao-Yong C. Comparison of the value of multiple preoperative objective nutritional indices for the evaluation of prognosis after hepatectomy for hepatocellular carcinoma. Nutr Cancer. (2022) 74:3217–27. doi: 10.1080/01635581.2022.2069276
38. Liu X, Qiu H, Kong P, Zhou Z, Sun X. Gastric cancer, nutritional status, and outcome. Onco Targets Ther. (2017) 10:2107–14. doi: 10.2147/OTT.S132432
39. Ingenbleek Y. Plasma transthyretin is a nutritional biomarker in human morbidities. Front Med. (2022) 16:540–50. doi: 10.1007/s11684-022-0940-3
40. Watson F, Dick M. Distribution and inheritance of low serum thyroxine-binding globulin levels in Australian Aborigines: a new genetic variation. Med J Aust. (1980) 2:385–7. doi: 10.5694/j.1326-5377.1980.tb131879.x
41. Sergi G, Coin A, Enzi G, Volpato S, Inelmen EM, Buttarello M, et al. Role of visceral proteins in detecting malnutrition in the elderly. Eur J Clin Nutr. (2006) 60:203–9. doi: 10.1038/sj.ejcn.1602289
42. Devakonda A, George L, Raoof S, Esan A, Saleh A, Bernstein LH. Transthyretin as a marker to predict outcome in critically ill patients. Clin Biochem. (2008) 41:1126–30. doi: 10.1016/j.clinbiochem.2008.06.016
43. James E, Goodall S, Nichols S, Walker K, Carroll S, O’Doherty AF, et al. Serum transthyretin and aminotransferases are associated with lean mass in people with coronary heart disease: Further insights from the CARE-CR study. Front Med (Lausanne). (2023) 10:1094733. doi: 10.3389/fmed.2023.1094733
44. Habib C, Maor I, Shoris I, Tsuprun S, Bader D, Riskin A. Umbilical cord and neonatal transthyretin and their relationship to growth and nutrition in preterm infants. Rambam Maimonides Med J. (2022) 13. doi: 10.5041/RMMJ.20769172
45. Delliere S, Pouga L, Neveux N, Hernvann A, De Bandt JP, Cynober L. Assessment of transthyretin cut-off values for a better screening of malnutrition: Retrospective determination and prospective validation. Clin Nutr. (2021) 40:907–11. doi: 10.1016/j.clnu.2020.06.017
46. Cui N, Tong H, Li Y, Ge Y, Shi Y, Lv P, et al. Role of prealbumin in predicting the prognosis of severely and critically ill COVID-19 patients. Am J Trop Med Hyg. (2021) 105:718–26. doi: 10.4269/ajtmh.21-0234
47. Takeuchi M, Tsuboi A, Minato S, Yano M, Kitaoka K, Kurata M, et al. Elevated serum adiponectin and tumor necrosis factor-alpha and decreased transthyretin in Japanese elderly women with low grip strength and preserved muscle mass and insulin sensitivity. BMJ Open Diabetes Res Care. (2018) 6:e000537. doi: 10.1136/bmjdrc-2018-000537
48. Han WX, Chen ZM, Wei ZJ, Xu AM. Preoperative pre-albumin predicts prognosis of patients after gastrectomy for adenocarcinoma of esophagogastric junction. World J Surg Oncol. (2016) 14:279. doi: 10.1186/s12957-016-1035-x
49. Shen Q, Liu W, Quan H, Pan S, Li S, Zhou T, et al. Prealbumin and lymphocyte-based prognostic score, a new tool for predicting long-term survival after curative resection of stage II/III gastric cancer. Br J Nutr. (2018) 120:1359–69. doi: 10.1017/S0007114518002854
50. Li JD, Xu XF, Han J, Wu H, Xing H, Li C, et al. Preoperative prealbumin level as an independent predictor of long-term prognosis after liver resection for hepatocellular carcinoma: a multi-institutional study. HPB (Oxford). (2019) 21:157–66. doi: 10.1016/j.hpb.2018.06.1803
51. Ho SY, Guo HR, Chen HH, Peng CJ. Nutritional predictors of survival in terminally ill cancer patients. J Formos Med Assoc. (2003) 102:544–50.
52. Isono N, Imamura Y, Ohmura K, Ueda N, Kawabata S, Furuse M, et al. Transthyretin concentrations in acute stroke patients predict convalescent rehabilitation. J Stroke Cerebrovasc Dis. (2017) 26:1375–82. doi: 10.1016/j.jstrokecerebrovasdis.2017.02.020
53. Knippel RJ, Drewes JL, Sears CL. The cancer microbiome: recent highlights and knowledge gaps. Cancer Discovery. (2021) 11:2378–95. doi: 10.1158/2159-8290.CD-21-0324
54. Beck FK, Rosenthal TC. Prealbumin: a marker for nutritional evaluation. Am Fam Physician. (2002) 65:1575–8.
55. Bhattacharya N, Yuan R, Prestwood TR, Penny HL, DiMaio MA, Reticker-Flynn NE, et al. Normalizing microbiota-induced retinoic acid deficiency stimulates protective CD8(+) T cell-mediated immunity in colorectal cancer. Immunity. (2016) 45:641–55. doi: 10.1016/j.immuni.2016.08.008
56. Brown G. Targeting the retinoic acid pathway to eradicate cancer stem cells. Int J Mol Sci. (2023) 24. doi: 10.3390/ijms24032373
Keywords: transthyretin, prognosis, colorectal cancer, clinicopathologic feature, cancer-specific survival, nomogram
Citation: Zhang Z, Hu C, Shi F, Zhang L, Wang Y, Zhang Y, Zhang X and She J (2025) Low transthyretin is associated with the poor prognosis of colorectal cancer. Front. Oncol. 15:1397019. doi: 10.3389/fonc.2025.1397019
Received: 06 March 2024; Accepted: 20 January 2025;
Published: 05 February 2025.
Edited by:
Mohamad Sonbol, Mayo Clinic Arizona, United StatesReviewed by:
Yanqiang Li, Boston Children’s Hospital and Harvard Medical School, United StatesMarianna Arsenovna Zolotovskaia, I.M. Sechenov First Moscow State Medical University, Russia
Lei Gao, Lanzhou University Second Hospital, China
Copyright © 2025 Zhang, Hu, Shi, Zhang, Wang, Zhang, Zhang and She. This is an open-access article distributed under the terms of the Creative Commons Attribution License (CC BY). The use, distribution or reproduction in other forums is permitted, provided the original author(s) and the copyright owner(s) are credited and that the original publication in this journal is cited, in accordance with accepted academic practice. No use, distribution or reproduction is permitted which does not comply with these terms.
*Correspondence: Junjun She, anVuanVuc2hlMTk3NUBzaW5hLmNvbQ==