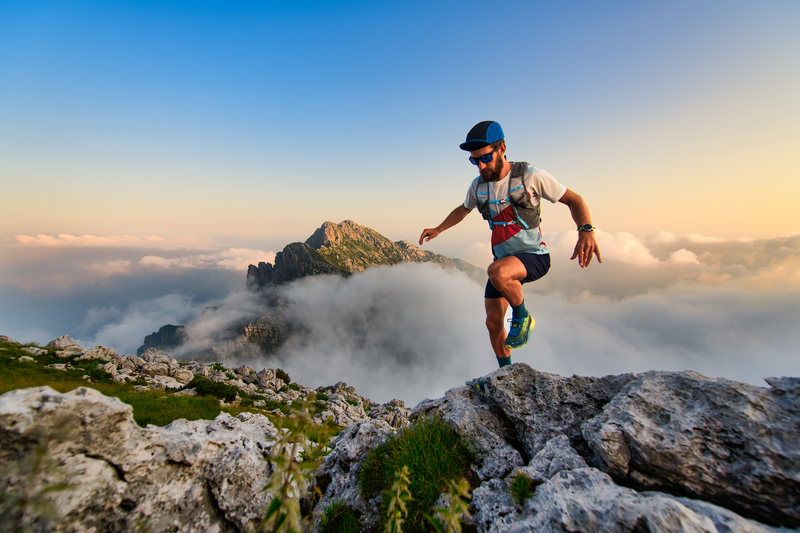
95% of researchers rate our articles as excellent or good
Learn more about the work of our research integrity team to safeguard the quality of each article we publish.
Find out more
SYSTEMATIC REVIEW article
Front. Oncol. , 16 December 2024
Sec. Cancer Epidemiology and Prevention
Volume 14 - 2024 | https://doi.org/10.3389/fonc.2024.1510578
This article is part of the Research Topic Living With and Beyond Cancer Across the Lifespan View all 9 articles
Objective: Cancer survivors often face significant health-related quality of life (HRQoL) challenges. Although exercise has been proven to improve HRQoL in cancer survivors, the optimal dose and intensity of exercise for this population has not been fully determined. Adherence to exercise may vary based on exercise intensity, affecting results. This study explored the dose-response relationship of different exercise types and intensities to better understand their impact on HRQoL in cancer survivors.
Methods: We searched five databases—PubMed, Embase, the Cochrane Library, Web of Science, and Scopus—from their inception until 1 December 2023. Data analysis was performed using R software with the MBNMA and RJAGS packages. Due to combining data from different scales, effect sizes were reported as standardized mean differences (SMD) with 95% credible intervals (95% CrI). The risk of bias was assessed independently by three reviewers using the RoB2 tool.
Results: A total of 48 studies involving 3050 cancer survivors. Across all exercise types, the most beneficial exercise dose was identified to be 850 metabolic equivalents of task (METs)-min/week (SMD: 0.753, 95%Crl: 0.463 to 1.096), with diminishing returns observed beyond 1,100 METs-min/week. Among the various types of exercises, mixed training (MT) emerged as the optimal choice, demonstrating its efficacy at 970 METs-min/week (SMD: 0.883, 95% Crl: 0.455 to 1.345). Aerobic exercise (AE) at a dose of 430 METs-min/week (SMD: 0.681, 95% Crl: 0.206 to 1.099) and resistance training (RT) at 450 METs-min/week (SMD: 0.695, 95% Crl: 0.227 to 1.203) also showed significant benefits. Additionally, mind-body exercises, such as tai chi, qigong, or yoga, exhibited optimal effects at a dose of 390 METs-min/week (SMD: 0.672, 95% Crl: 0.259 to 1.087).
Conclusion: Our study sheds light on the intricate relationship between exercise interventions and health-related quality of life in cancer survivors, as elucidated through a systematic review and Bayesian network meta-analysis. The identified optimal exercise dose of 850 METs-min/week resulted in a significant improvement in health-related quality of life, underscoring the importance of regular exercise in cancer survivorship. MT emerged as the most effective modality, closely followed by RT, AE, and MBE.
Systematic review registration: https://www.crd.york.ac.uk/prospero/display_record.php?RecordID=493328, identifier CRD42024493328.
Cancer is a group of diseases characterized by abnormal cell growth. It is one of the deadliest diseases worldwide, claiming several million lives annually (1, 2). According to research statistics from 185 countries for 36 kinds of cancers in 2020, there were approximately 19.3 million cases of cancer and nearly 10 million cancer-related deaths worldwide, and the incidence rates of cancer in both men and women were two to three times higher in developing countries than in developed countries (3). Because of the strong influence of the demographic shift towards aging on the varying trends in cancer incidence in different regions, the incidence of all cancers is projected to double by 2070 compared with 2020 (4). In addition, the global economic cost of cancer from 2020 to 2050 is estimated to be US$25.2 trillion which is equivalent to an annual tax on the global gross domestic product of 0.55% (5). Although medical treatment has significantly improved the survival rate of cancer patients in recent years, the symptoms that arise after treatment can seriously impact the quality of life of these patients. Therefore, it is crucial from both clinical and public health perspectives to eliminate the negative effects of cancer in all aspects.
Exercise is increasingly recognized as a non-pharmacological intervention with profound implications for improving the quality of life across a spectrum of chronic diseases, including metabolic syndrome-related disorders, cardiovascular and pulmonary diseases, muscular and joint disorders, and cancer (6, 7). In the case of exercise in oncology, a multitude of preclinical studies have consistently demonstrated the anti-tumor effects of different types of exercise across various cancer models (8, 9). Furthermore, adding to the body of evidence, a review published in the Journal of Natural Metabolism systematically explored the pathogenic factors of cancer and proposed a reprogramming strategy involving the immune and metabolic networks stimulated by exercise. This not only reinforces the connection between exercise and the suppression of tumorigenesis but also underscores the logical coherence underlying the role of exercise in combating cancer (10).
Health-related quality of life (HRQoL) serves as the primary endpoint for evaluating the well-being of cancer survivors, encompassing the impact of the disease and its treatments on physical, psychological, and social dimensions (11). Consequently, a growing number of researchers are increasingly directing their attention toward understanding and enhancing HRQoL among cancer survivors. For example, in a previous study investigating the efficacy of exercise in improving overall HRQoL among adult cancer survivors post-treatment, it was concluded that exercise indeed had a positive impact, particularly evident in addressing cancer-specific concerns (12). Furthermore, a recent network meta-analysis encompassing 93 studies with 7,435 cancer patients further reinforced these findings. It demonstrated that a combination of aerobic and resistance exercises significantly enhances HRQoL in both the during and post-treatment phases, highlighting its clinical significance (13).
There is evidence of the effectiveness of exercise in improving the HRQoL of cancer patients, and studies have confirmed the effectiveness of specific exercise forms. However, there are still research gaps regarding the optimal exercise dose. To improve precision exercise interventions for cancer survivors’ HRQoL, we used metabolic equivalents of task (METs) for different exercise doses (14) and explored the optimal dose-response relationship between exercise and HRQoL.
Our study is a systematic review and used a new dose-response Bayesian model network meta-analysis (MBNMA) method (15). The aim was to quantify the available evidence from randomized controlled trials that exercise improves HRQoL in cancer survivors. We used the new method to not only explore the optimal mode of exercise to improve the quality of life of cancer survivors but also identify the optimal exercise dose that improves cancer survivors’ HRQoL.
This systematic review and network meta-analysis was registered with the International Prospective Register of Systematic Reviews (PROSPERO: CRD 42024493328) and this NMA followed the Preferred Reporting Items for Systematic Review and Meta-analysis protocols statement extension (PRISMA-NMA) checklist (16).
A systematic search for studies was carried out in the PubMed, Embase, the Cochrane Library, Web of Science, and Scopus databases from their inception to 1 December 2023. The search strategy was structured according to the Peer Review of Electronic Search Strategies 2015 guidelines (17). We provide a complete list of search times, search entries, and search results in the Supplementary Material (Supplementary Table 1). Two investigators (ZX and YY) independently screened the title/abstract and full text of the articles, with any disagreements resolved by discussion or adjudication by a third author (YL).
According to the principles of the “PICOS” inclusion criteria (18), the included studies comprised the following criteria: (a) Population: cancer survivors over 18 years of age; (b) Intervention: Any form of exercise; (c) Outcome: Measure the health-related quality of life in cancer survivors [ex: Functional Assessment of Cancer Therapy-General (Fact-General) (19); 36-Item Short Form Health Survey (SF-36) (20); European Organization for Research and Treatment of Cancer Quality of Life Questionnaire-Core 30 (EORTC QLQ-C30) (21); World Health Organization Quality of Life-BREF (WHOQOL-BREF) (22)]; (d) Study design: Must be randomized controlled trial (RCT); (e): Comparison: Controls could be daily care, physical health education, or other forms of exercise.
The exclusion criteria were as follows: (a) studies on children with cancer and cancer patients undergoing treatment; (b) intervention studies combining exercise with cognitive therapy, physiotherapy, massaging, diet, or medication; (c) studies that excluded trials with no control group; (d) endpoints that reported cancer-specific scales and excluded non-cancer survivors’ HRQoL scales; and (e) individuals with major depression, cognitive impairment or other diseases that may affect exercise ability and quality of life.
Two authors independently extracted data from the included studies (ZX and YY), and all authors resolved disagreements by consensus. First, the following data from each included study were extracted: first author and year of publication, sample (sample size), region (study country), age (patient age), cancer type, intervention characteristics (intervention duration/time, type of exercise), and HRQoL-related outcome indicators.
Second, data were extracted as means and standard deviations (SD) of the HRQoL-related outcome indicators before and after the intervention, based on the Cochrane Handbook for Systematic Evaluation of Intervention (23). To meet the data analysis requirements of the dose-response network meta-analysis package in the R program, we also converted the standard errors (SE). SE=SD/SQRT (Sample size/N) (24). The relevant data are provided in Supplementary File 3. When it was not possible to retrieve the minimum required data from a published report for the dose-response meta-analysis, we contacted the authors and invited them to provide additional data.
First, we coded the interventions in a first level of categorization to classify all the interventions as equivalent to analyze the dose-response effect of overall exercise on the HRQoL of cancer survivors. Second, to analyze the optimal dose and the different forms of exercise, a second level of classification coding was performed on the interventions: Aerobic exercise (AE) (treadmill training, dance; stationary bike, etc.), mind-body exercise (MBE) (yoga; taiji; qigong);, mixed training (MT) (combined aerobic exercise and resistance exercise), resistance training (RT), and control (Placebo).
Previous studies have demonstrated the good efficacy of metabolic equivalents of task (METs) in evaluating exercise intensity (25, 26). Thus, in our study, the term “dose” referred to energy expenditure expressed in METs-min/week. We used the method validated by Ainsworth et al. (14) to calculate the different doses associated with each intervention in our study and to calculate the product of the duration of the exercise intervention, the number of sessions, and the metabolic equivalent of the task (METs-min/week). In order to better promote the connectivity of the network, we used an approximate value of 250, 500, 750, 1000, or 1500 METs-min/week for the exercise dose, which was adopted from previous studies (27).
We used the Bayesian-Model of network meta-analysis package (MBNMA) (28), and “rjags” package (29) in R version 4.0.3. (R Foundation for Statistical Computing, Vienna, Austria). We conducted the network meta-analysis to compare the overall relative effectiveness and different dose responses of the interventions under investigation. We checked the connectivity of the motion pattern grid for different motion doses based on the dose approximation (30) and we obtained agreement between the two models by comparing them with an uncorrelated mean-effects model (UME) (Supplementary File 4.1) (31). Finally, we assessed transitivity using a node-splitting approach (Supplementary File 4.2) (32). There were no parametric results that indicated a violation of the above key assumptions.
Regarding the dose-response models, firstly, to identify the observed effect of the overall exercise dose and different exercise doses on HRQoL, we plotted a confirmation of the non-linear relationship between overall exercise dose and cancer survivors’ HRQoL improvement (Supplementary File 5 Figures 1, 2).
To summarize the dose-response relationship between exercise and cancer survivors’ HRQoL, we compared dose-response models (Emax, Exponential, Restricted cubic spline, non-parametric, and linear models) regarding fit indices, including the deviance information criterion (DIC), between-study standard deviation, the number of parameters in the models, and the residual values. Ultimately, we opted for the random effect of restricted cubic splines to evaluate the non-linear dose-response association, as detailed in Supplementary File 5 Table 5.1.
In order to visualize our study data and model-fitting results, deviance plots were used to confirm the robustness of our model selection (Supplementary File 5 Figures 3, 4) (33). We employed a Markov Chain Monte Carlo (MCMC) model, utilizing three chains with 20,000 iterations each (the first 10,000 discarded) and a thinning factor of 10. Through this model, we leveraged Beta coefficients derived from restricted cubic spline curves to estimate both the overall and varying effects of exercise doses on HRQoL, identifying the exercise dose associated with the optimal effect. Additionally, we placed nodes at the 10th, 50th, and 90th percentiles of the exercise dose to effectively visualize the results of our model fitting process (15). Our study considered different outcome measures. Therefore, we chose the standardized mean difference (SMD) (34) as the effect size for our study. We used a 95% credible interval (95% CrI) to provide a range of values within which we are confident the true effect size lies (35). The code to reproduce the results presented in this paper can be requested by contacting the first author’s e-mail address.
It was essential to identify and address the fundamental assumptions of heterogeneity, inconsistency, and consistency. Therefore, we first used the tau-squared (τ²) test and I² statistic to evaluate the statistical heterogeneity between the studies. Moreover, we assessed global inconsistency statistically using the design-by-treatment interaction test. We separated indirect from direct evidence using the SIDE (Separating Indirect from Direct Evidence) test using the “netmeta” package. Additionally, we compared adjusted funnel plots to assess the risk of publication bias under specific circumstances. Egger’s test indicated bias when p<0.05.
Additionally, the risk of bias was assessed according to the second version of the Cochrane risk-of-bias tool for randomized trials (RoB 2) (36). Three reviewers (BQ, YB, and TW) assessed the study. The assessment items included randomized sequence generation, bias due to deviation from the intended intervention, incomplete data, bias in measurements, and selective bias in reporting results. And conducted a risk assessment of our research based on the following items:
● Random sequence generation. This domain assesses whether the process of randomizing participants was adequately described and whether the random sequence generation method was likely to produce comparable groups. Studies were classified as having low, unclear, or high risk of bias based on the transparency and appropriateness of the randomization process.
● Bias due to deviations from intended interventions. This domain assesses whether participants and personnel were blinded to the interventions and whether deviations from the intended interventions could have affected the study results. Risks were categorized based on the degree of blinding and the handling of deviations from the intervention protocol.
● Incomplete data. This domain considers whether the study had complete outcome data or if there were substantial follow-up losses that could affect the reliability of the results. Risks were assessed based on how missing data were handled and the impact on the study conclusions.
● Bias in outcome measurement. This domain assesses whether the methods used to measure the outcomes were appropriate and whether there was a risk of bias in the measurement process. Studies were rated based on whether the outcome measurements were objectively consistent or if there was a risk of bias due to subjective assessment methods.
● Selective reporting bias. This domain assesses whether there was evidence of the selective reporting of outcomes, such as reporting only some outcomes and omitting others. Studies were rated based on whether all prespecified outcomes were reported and whether there was any evidence of selective reporting.
Disagreements between reviewers were resolved by the third author (JW), who was responsible for re-examining controversial assessments and discussing with other reviewers to reach a consensus, ensuring the accuracy and consistency of the assessment results.
To assess the potential impact of high risk of bias on our findings, we performed sensitivity analyses in which we excluded studies assessed to be at high risk of bias and reanalyzed the dose-response data.
A total of 18,571 study records were found. After removing duplicates and literature that did not fit the study by title, abstract, etc., we considered 215 full-text articles that were then screened for eligibility. Figure 1 shows a flow diagram of the study selection process. Finally, 48 studies involving 3050 participants were included in our network meta-analysis. The patients included in the studies had different types of cancer and were aged between 44 to 72 years old. The basic characteristics of the included population are given in Table 1.
Whether connectivity was met is the basis of NMA. A lack of connectivity can lead to low statistical power and misleading results when a direct comparison is not possible (32). The analysis confirmed that there was no connectivity deficit in the two networks, ensuring accurate results (Figure 2).
Figure 2. Treatment-level (A) and agent-level network plots (B) for specific interventions. AE, aerobic exercise; RT, resistance training; MBE, mind-body exercise; MT, mixed training (aerobic + resistance training); CON, control group. Exercise dose corresponds to different exercise types (METs-min/week).
Figure 3 shows the non-linear dose-response relationship between overall exercise dose and cancer survivors’ HRQoL. Notably, we found an inverted U-shape between the overall exercise dose and cancer survivors’ HRQoL, and an optimal peak dose for improving HRQoL existed. Overall exercise was found to be effective until 1100 METs-min/week (SMD: 0.716, 95% Crl: 0.169 to 1.280) but the optimal overall exercise dose was found to be 850 METs-min/week (SMD: 0.753, 95% Crl: 0.463 to 1.096).
Figure 3. Dose-response relationship between overall exercise dose and change in the HRQoL of cancer survivors.
Figure 4 shows the non-linear dose-response relationship between different exercise doses and cancer survivors’ HRQoL in 18 studies involving 657 cancer survivors who participated in MT. The graphs show a trend of corresponding improvement in HRQoL with increasing MT dose until 1200 METs-min/week. The optimal MT dose was found to be 970 METs-min/week (SMD: 0.883, 95% Crl: 0.455 to 1.345).
Figure 4. Dose-response relationship between different exercise doses and change in the HRQoL of cancer survivors. AE, aerobic exercise; RT, resistance training; MBE, mind-body exercise; MT, mixed training (aerobic + resistance Training).
Furthermore, in 16 studies involving 369 cancer survivors who participated in AE, in 7 studies involving 288 cancer survivors who participated in RT, and in 13 studies involving 311 cancer survivors who participated in MBE, we found the inverted U-shape for the AE, MBE, and RT intervention doses and cancer survivors’ HRQoL. None of these exercise doses exceeded 750 METs-min/week. AE was effective until 720 METs-min/week, and the optimal AE dose was found to be 430 METs-min/week (SMD: 0.681, 95% Crl: 0.206 to 1.099). Additionally, MBE was effective until 610 METs-min/week and the optimal MBE dose was found to be 390 METs-min/week (SMD: 0.672, 95% Crl: 0.259 to 1.087). The RT was effective until 660 METs-min/week, and the optimal RT dose was found to be 450 METs-min/week (SMD: 0.695, 95% Crl: 0.227 to 1.203).
In total, 22 studies (46%) were found to have a low risk of bias, 15 studies (31%) were found to have an unclear risk of bias, and 11 studies (23%) were found to have a high risk of bias. Figure 5 shows the result of the Cochrane Risk of Bias Tool and the study-level risk of bias assessments are presented in Supplementary File 6.
The heterogeneity of HRQoL was moderate [I^2 = 58.9% (43.0%; 70.4%)] and the SIDE test of all outcomes showed that there was a percentage of comparisons with evidence of inconsistency (Supplementary File 7). At the same time, our funnel plot results showed that there was no publication bias and the funnel plot was symmetrical (Figure 6). In addition, we used Egger’s test to assess publication bias more formally. As shown in the figure, our included studies did not violate these two points.
Figure 6. Funnel plot of the publication bias among the comparison groups. The funnel plot shows the relationship between the effect size and standard error of different exercise methods (AE, MBE, CON, RT, MT). The Egger’s test result was 0.485, indicating that the possibility of publication bias was small. AE, aerobic exercise; RT, resistance training; MBE, mind-body exercise; MT, mixed training (aerobic + resistance Training); CON, control.
We excluded 11 articles with a high risk of bias and reanalyzed the data. The results showed that the optimal dose of overall exercise was estimated to be 730 METs-min/week (SMD:0.727, 95%Crl: 0.461 to 1.038), 890 METs-min/week for MT (SMD:0.8418, 95%Crl: 0.226 to 1.306), 410 METs-min/week for AE (SMD:0.914, 95%Crl: 0.32 to 1.506), 410 METs-min/week for MBE (SMD:0.625, 95%Crl: 0.219 to 1.071), and 630 METs-min/week for RT (SMD:0.588, 95%Crl: 0.301 to 1.195). Additionally, these results of sensitive analysis confirmed the robustness of our study results. We provide visualization curve results in Supplementary Figures 1, 2 in Supplementary File 9.
Our systematic review and network meta-analysis included 48 randomized controlled trials involving 3050 cancer survivors. We determined the optimal exercise dose for overall exercise for improving cancer patients’ HRQoL, and as the dose increased, the effect took on an inverted U shape. We found that the optimal overall exercise dose to alter HRQoL in cancer survivors was 850 METs-min/week. Furthermore, in terms of different exercises, we found MT to be the best form of exercise to improve HRQoL, and we also found the optimal dose of MT (970 METs-min/week). Finally, we found that the optimal dose for improving HRQoL was 430 METs-min/week, 390 METs-min/week, and 450 METs-min/week for AE, MBE, and RT, respectively.
First, we demonstrated that there is an optimal overall exercise dose. This is consistent with previous research that showed that exercise, no matter what it is, may not only be “healthy” but may actually have therapeutic effects (37). It was also well established in a cohort study that cancer survivors who engaged in 15 MET-hours of physical activity per week had a 27% lower risk of cancer-related mortality (38).
Notably, research suggests that exercise may exert its beneficial effects on cancer through various mechanisms, such as the alteration of circulating factors such as myokines and hormones released by skeletal muscle (39). One study in particular delved into these mechanisms, shedding light on how exercise can inhibit cancer cell proliferation, induce apoptosis, regulate metabolism, and enhance the immune environment (40). In our study, we determined the effective and safe value range of the overall exercise dose, which is undoubtedly a highlight for exercise and tumor research.
Furthermore, we found that the optimal form was MT (combined aerobic exercise and resistance training) for improving HRQoL in cancer survivors. The optimal MT dose was 970 METs-min/week. This finding not only aligns with previous analyses that unanimously recognize MT as the most effective form of exercise for enhancing HRQoL but also corresponds with international statements regarding exercise guidelines for cancer survivors (13, 41). In the included studies, MT consists of aerobic exercise and resistance training. In our study, we determined the optimal dose for resistance training to be 450 METs-min/week. Previous studies have indicated that resistance training correlates with a decreased risk of all-cause mortality, cardiovascular disease, and cancer-specific mortality (42). Furthermore, recent reviews of study outcomes suggest that non-traditional resistance training can yield equivalent or greater improvements in muscle strength, hypertrophy, body function, and composition compared to traditional methods. Studies on eccentric, cluster, and blood flow restriction training in older adults and other clinical populations provide robust evidence supporting its potential application in cancer research (43). This underscores the significance of integrating resistance exercise into the treatment regimen for cancer patients, as our study unequivocally confirms its efficacy in enhancing HRQoL within this demographic. Notably, our research identified the optimal intensity for resistance exercise, marking a substantial advancement over prior studies. Moreover, the optimal aerobic exercise dose to improve HRQoL was found to be 430 METs-min/week. We explored the main potential mechanism: an animal study has demonstrated that exercise is linked to the mobilization and redistribution of natural killer (NK) cells through adrenaline and interleukin-6 (IL-6), ultimately regulating tumorigenesis. This research result suggests that aerobic exercise can reduce the risk of cancer and disease recurrence by over 60% (44). Our research categorizes tai chi, qigong, and yoga as MBE (45). We found that the optimal dose of MBE was 390 METs-min/week. A previous study confirmed an improvement in the HRQoL of cancer patients through tai chi and qigong (46). On this basis, we determined the dose of MBE. However, the underlying mechanisms by which mind-body exercise improves symptoms in cancer patients are uncertain.
In addition, we conducted heterogeneity, inconsistency, and publication bias tests through network meta-analysis. Our results did not find any statistical differences in our data. We found that the heterogeneity was moderate and used a random effects model to merge the data. These key steps also indirectly boosted the credibility of our research from statistics to practice.
While our study provides valuable insights, it is important to acknowledge its limitations. First, we did not conduct meticulous statistical heterogeneity analysis to explore possible heterogeneity among the studies.
Furthermore, in the preliminary analysis, we found that MT was the most effective in improving the HRQoL of cancer survivors. However, after sensitivity analysis, AE showed a more significant effect after excluding the studies with a high risk of bias. This change in results may reflect the impact of the risk of bias in different studies on the preliminary results. Specifically, some studies with high bias may have overestimated the effect of MT, causing the preliminary analysis results to favor MT.
This suggests that in high-quality, low-bias studies, AE may have an advantage in improving health-related quality of life. This finding reminds us that study quality and risk of bias should be considered when selecting interventions, and suggests that the relative effectiveness of different types of exercise may need to be re-evaluated in different research scenarios.
Nevertheless, this does not negate the potential value of MT, especially in specific patient groups. However, based on the results of the sensitivity analysis, AE may be a more robust and widely applicable option. Future studies should further explore the effects of these interventions in different cancer survivor groups to determine the optimal treatment plan.
Additionally, we did not report basic information regarding patient medication use, and it is plausible that patients received treatment not solely due to exercise-related improvements in outcomes. Moreover, our findings focused exclusively on the HRQoL of cancer patients and may not extend to all symptoms associated with cancer. We also note that the generalizability of our results may be limited as we observed an insufficient number of randomized controlled studies conducted in Asian populations. Although most exercise doses were reported following extensive deliberations between two reviewers, there remains the possibility of some misclassification at the dose level. Therefore, approximations were employed for dose characterization. Another potential limitation is the inclusion of cancer survivors with comorbidities (e.g., major depression or cognitive impairment) that may have underlying factors that may affect quality of life. These comorbidities may have had an impact on the intervention effect and may have confounded the relationship between exercise dose and HRQoL improvement to some extent. Although excluding these individuals would have resulted in a more homogeneous sample and a clearer understanding of the optimal exercise dose, we chose to include them in the study to increase the external validity of the study. Many cancer survivors face multiple health challenges simultaneously, and including these individuals made our findings more generalizable. Future studies may consider stratifying participants according to specific comorbidities or controlling for these variables in the study design to more precisely assess the effects of the exercise intervention. Finally, there may be possible differences in diagnosis stage and cancer type due to a higher proportion of female participants in the sample as certain cancer types (such as breast cancer) are more common in women, resulting in a relatively large number of female cancer survivors. This gender imbalance may have had an impact on the research results as a result of differences in physiology, psychology, and response to exercise intervention. Therefore, the inconsistent diagnosis of cancer patients in our study and the mixture of various cancer types may lead to doubts about the reliability of our results. In future RCT experiments, we should actively verify the true and effective value of our core research results, and provide recommended guidelines for exercise prescription for the next RCT experiment.
Our systematic review and dose-response network meta-analysis were conducted using the latest Bayesian modeling “MBNMA dose” and “ragjs” packages. We revealed a non-linear dose-response relationship between overall exercise dosage and health-related quality of life enhancement in cancer survivors. Notably, an inverted U-shaped pattern was observed, indicating an optimal peak dosage for HRQoL improvement. Effective exercise dosages ranged up to 1100 METs-min/week, with the optimal dosage identified as 850 METs-min/week. Additionally, MT was the optimal exercise form, and peak HRQoL improvement was observed at 970 METs-min/week. Across various exercise types, including AE, RT, and MBE, an inverted U-shaped relationship with HRQoL was evident, with optimal dosages identified for each modality. These findings provide essential insights for tailoring exercise prescriptions to optimize HRQoL outcomes among cancer survivors.
The original contributions presented in the study are included in the article/Supplementary Material. Further inquiries can be directed to the corresponding author.
ZX: Writing – review & editing, Writing – original draft. YYu: Writing – original draft, Writing – review & editing. YYa: Writing – review & editing. BQ: Writing – review & editing. YB: Writing – review & editing. TW: Writing – review & editing. JW: Writing – review & editing. LZ: Writing – review & editing. YL: Writing – original draft, Writing – review & editing.
The author(s) declare that no financial support was received for the research, authorship, and/or publication of this article.
The authors declare that the research was conducted in the absence of any commercial or financial relationships that could be construed as a potential conflict of interest.
The author(s) declare that no Generative AI was used in the creation of this manuscript.
All claims expressed in this article are solely those of the authors and do not necessarily represent those of their affiliated organizations, or those of the publisher, the editors and the reviewers. Any product that may be evaluated in this article, or claim that may be made by its manufacturer, is not guaranteed or endorsed by the publisher.
The Supplementary Material for this article can be found online at: https://www.frontiersin.org/articles/10.3389/fonc.2024.1510578/full#supplementary-material
RCT, Randomized controlled trials; SMD, Standardized mean difference; SD, Standardized Deviation; SE, Standard errors; AE, Aerobic exercise; MBE, Mind-body exercise; MT, Mixed training; RT, Resistance training; DIC, Eviance information Criterion; UME, Uncorrelated mean-effects model; MCMC, Markov Chain Monte Carlo; HRQoL, Health-Related Quality of Life; MCID, Minimum Clinically Important Difference; 95% CrI, 95% credible intervals.
1. Chhikara BS, Parang K. Global Cancer Statistics 2022: the trends projection analysis. Chem Biol Lett. (2023) 10(1):451.
2. Matthews HK, Bertoli C, de Bruin RAM. Cell cycle control in cancer. Nat Rev Mol Cell Biol. (2022) 23:74–88. doi: 10.1038/s41580-021-00404-3
3. Sung H, Ferlay J, Siegel RL, Laversanne M, Soerjomataram I, Jemal A, et al. Global cancer statistics 2020: globocan estimates of incidence and mortality worldwide for 36 cancers in 185 countries. CA Cancer J Clin. (2021) 71:209–49. doi: 10.3322/caac.21660
4. Soerjomataram I, Bray F. Planning for tomorrow: global cancer incidence and the role of prevention 2020-2070. Nat Rev Clin Oncol. (2021) 18:663–72. doi: 10.1038/s41571-021-00514-z
5. Chen S, Cao Z, Prettner K, Kuhn M, Yang J, Jiao L, et al. Estimates and projections of the global economic cost of 29 cancers in 204 countries and territories from 2020 to 2050. JAMA Oncol. (2023) 9:465–72. doi: 10.1001/jamaoncol.2022.7826
6. Mitchell T, Barlow CE. Review of the role of exercise in improving quality of life in healthy individuals and in those with chronic diseases. Curr Sports Med Rep. (2011) 10:211–6. doi: 10.1249/JSR.0b013e318223cc9e
7. Pedersen BK, Saltin B. Evidence for prescribing exercise as therapy in chronic disease. Scandinavian J Med Sci Sports. (2006) 16:3–63. doi: 10.1111/j.1600-0838.2006.00520.x
8. Koelwyn GJ, Quail DF, Zhang X, White RM, Jones LW. Exercise-dependent regulation of the tumour microenvironment. Nat Rev Cancer. (2017) 17:620–32. doi: 10.1038/nrc.2017.78
9. Ashcraft KA, Peace RM, Betof AS, Dewhirst MW, Jones LW. Efficacy and mechanisms of aerobic exercise on cancer initiation, progression, and metastasis: A critical systematic review of in vivo preclinical data. Cancer Res. (2016) 76:4032–50. doi: 10.1158/0008-5472.Can-16-0887
10. Koelwyn GJ, Zhuang X, Tammela T, Schietinger A, Jones LW. Exercise and immunometabolic regulation in cancer. Nat Metab. (2020) 2:849–57. doi: 10.1038/s42255-020-00277-4
11. Roila F, Cortesi E. Quality of life as a primary end point in oncology. Ann Oncol. (2001) 12 Suppl 3:S3–6. doi: 10.1093/annonc/12.suppl_3.s3
12. Mishra SI, Scherer RW, Geigle PM, Berlanstein DR, Topaloglu O, Gotay CC, et al. Exercise interventions on health-related quality of life for cancer survivors. Cochrane Database Syst Rev. (2012) 2012:Cd007566. doi: 10.1002/14651858.CD007566.pub2
13. Martínez-Vizcaíno V, Cavero-Redondo I, Reina-Gutiérrez S, Gracia-Marco L, Gil-Cosano JJ, Bizzozero-Peroni B, et al. Comparative effects of different types of exercise on health-related quality of life during and after active cancer treatment: A systematic review and network meta-analysis. J Sport Health Sci. (2023) 12:726–38. doi: 10.1016/j.jshs.2023.01.002
14. Ainsworth BE, Haskell WL, Herrmann SD, Meckes N, Bassett DR Jr., Tudor-Locke C, et al. 2011 Compendium of physical activities: A second update of codes and met values. Med Sci Sports Exerc. (2011) 43:1575–81. doi: 10.1249/MSS.0b013e31821ece12
15. Pedder H ed. (2021). MBNMAdose: an R package for incorporating dose-response information into network meta-analysis, In: Evidence Synthesis and Meta-Analysis in R Conference 2021.
16. Page MJ, Moher D, Bossuyt PM, Boutron I, Hoffmann TC, Mulrow CD, et al. Prisma 2020 explanation and elaboration: updated guidance and exemplars for reporting systematic reviews. bmj. (2021) 372. doi: 10.1136/bmj.n160
17. McGowan J, Sampson M, Salzwedel DM, Cogo E, Foerster V, Lefebvre C. Press peer review of electronic search strategies: 2015 guideline statement. J Clin Epidemiol. (2016) 75:40–6. doi: 10.1016/j.jclinepi.2016.01.021
18. Amir-Behghadami M, Janati A. Population, intervention, comparison, outcomes and study (Picos) design as a framework to formulate eligibility criteria in systematic reviews. Emergency Med J. (2020) 37:387–. doi: 10.1136/emermed-2020-209567
19. Brady MJ, Cella DF, Mo F, Bonomi AE, Tulsky DS, Lloyd SR, et al. Reliability and validity of the functional assessment of cancer therapy-breast quality-of-life instrument. J Clin Oncol. (1997) 15:974–86. doi: 10.1200/jco.1997.15.3.974
20. Ware JE, Sherbourne CD. The mos 36-item short-form health survey (Sf-36).1. Conceptual-framework and item selection. Med Care. (1992) 30:473–83. doi: 10.1097/00005650-199206000-00002
21. Aaronson NK, Ahmedzai S, Bergman B, Bullinger M, Cull A, Duez NJ, et al. The european-organization-for-research-and-treatment-of-cancer qlq-C30 - a quality-of-life instrument for use in international clinical-trials in oncology. J Natl Cancer Institute. (1993) 85:365–76. doi: 10.1093/jnci/85.5.365
22. Harper A, Power M, Grp W. Development of the world health organization whoqol-bref quality of life assessment. psychol Med. (1998) 28:551–8. doi: 10.1017/s0033291798006667
23. Higgins JP. Cochrane Handbook for Systematic Reviews of Interventions Version 5.0. 1. The Cochrane Collaboration (2008). Available at: http://www.cochrane-handbook.org.
24. Watt JA, Del Giovane C, Jackson D, Turner RM, Tricco AC, Mavridis D, et al. Incorporating dose effects in network meta-analysis. Bmj. (2022) 376:e067003. doi: 10.1136/bmj-2021-067003
25. Jetté M, Sidney K, Blümchen G. Metabolic equivalents (Mets) in exercise testing, exercise prescription, and evaluation of functional capacity. Clin Cardiol. (1990) 13:555–65. doi: 10.1002/clc.4960130809
26. Eijsvogels TM, Thompson PD. Exercise is medicine: at any dose? Jama. (2015) 314:1915–6. doi: 10.1001/jama.2015.10858
27. Gallardo-Gómez D, del Pozo-Cruz J, Noetel M, Álvarez-Barbosa F, Alfonso-Rosa RM, del Pozo Cruz B. Optimal dose and type of exercise to improve cognitive function in older adults: A systematic review and bayesian model-based network meta-analysis of rcts. Ageing Res Rev. (2022) 76:101591. doi: 10.1016/j.arr.2022.101591
28. Pedder H, Dias S, Bennetts M, Boucher M, Welton NJ. Joining the dots: linking disconnected networks of evidence using dose-response model-based network meta-analysis. Med Decis Making. (2021) 41:194–208. doi: 10.1177/0272989x20983315
29. Shim SR, Kim SJ, Lee J, Rücker G. Network meta-analysis: application and practice using R software. Epidemiol Health. (2019) 41:e2019013. doi: 10.4178/epih.e2019013
30. Higgins JP, Jackson D, Barrett JK, Lu G, Ades AE, White IR. Consistency and inconsistency in network meta-analysis: concepts and models for multi-arm studies. Res Synth Methods. (2012) 3:98–110. doi: 10.1002/jrsm.1044
31. Wei J, Zeng C, Lei GH. Heterogeneity, consistency and model fit should be assessed in bayesian network meta-analysis. Ann Rheum Dis. (2016) 75:e5. doi: 10.1136/annrheumdis-2015-208658
32. Rouse B, Chaimani A, Li T. Network meta-analysis: an introduction for clinicians. Intern Emerg Med. (2017) 12:103–11. doi: 10.1007/s11739-016-1583-7
33. Hamza T, Cipriani A, Furukawa TA, Egger M, Orsini N, Salanti G. A bayesian dose–response meta-analysis model: A simulations study and application. Stat Methods Med Res. (2021) 30:1358–72. doi: 10.1177/0962280220982643
34. Lin L, Aloe AM. Evaluation of various estimators for standardized mean difference in meta-analysis. Stat Med. (2021) 40:403–26. doi: 10.1002/sim.8781
35. Partlett C, Riley RD. Random effects meta-analysis: coverage performance of 95% Confidence and prediction intervals following reml estimation. Stat Med. (2017) 36:301–17. doi: 10.1002/sim.7140
36. Minozzi S, Cinquini M, Gianola S, Gonzalez-Lorenzo M, Banzi R. The revised cochrane risk of bias tool for randomized trials (Rob 2) showed low interrater reliability and challenges in its application. J Clin Epidemiol. (2020) 126:37–44. doi: 10.1016/j.jclinepi.2020.06.015
37. Idorn M, thor Straten P. Exercise and cancer: from “Healthy” to “Therapeutic”? Cancer Immunology Immunotherapy. (2017) 66:667–71.
38. Li T, Wei S, Shi Y, Pang S, Qin Q, Yin J, et al. The dose–response effect of physical activity on cancer mortality: findings from 71 prospective cohort studies. Br J sports Med. (2016) 50:339–45. doi: 10.1136/bjsports-2015-094927
39. Bettariga F, Taaffe DR, Galvão DA, Bishop C, Kim JS, Newton RU. Suppressive effects of exercise-conditioned serum on cancer cells: A narrative review of the influence of exercise mode, volume, and intensity. J Sport Health Sci. (2023). doi: 10.1016/j.jshs.2023.12.001
40. Wang Q, Zhou W. Roles and molecular mechanisms of physical exercise in cancer prevention and treatment. J Sport Health Sci. (2021) 10:201–10. doi: 10.1016/j.jshs.2020.07.008
41. Campbell KL, Winters-Stone K, Wiskemann J, May AM, Schwartz AL, Courneya KS, et al. Exercise guidelines for cancer survivors: consensus statement from international multidisciplinary roundtable. Med Sci sports Exercise. (2019) 51:2375. doi: 10.1249/MSS.0000000000002116
42. Shailendra P, Baldock KL, Li LSK, Bennie JA, Boyle T. Resistance training and mortality risk: A systematic review and meta-analysis. Am J Prev Med. (2022) 63:277–85. doi: 10.1016/j.amepre.2022.03.020
43. Bettariga F, Bishop C, Taaffe DR, Galvão DA, Maestroni L, Newton RU. Time to consider the potential role of alternative resistance training methods in cancer management? J Sport Health Sci. (2023) 12:715–25. doi: 10.1016/j.jshs.2023.06.007
44. Pedersen L, Idorn M, Olofsson GH, Lauenborg B, Nookaew I, Hansen RH, et al. Voluntary running suppresses tumor growth through epinephrine- and il-6-dependent nk cell mobilization and redistribution. Cell Metab. (2016) 23:554–62. doi: 10.1016/j.cmet.2016.01.011
45. Ives JC, Sosnoff J. Beyond the mind-body exercise hype. Physician sportsmedicine. (2000) 28:67–81. doi: 10.3810/psm.2000.03.776
Keywords: cancer survivor, exercise, health-related quality of life, dose-response, Bayesian network meta-analysis
Citation: Xiong Z, Yuan Y, Yang Y, Qiu B, Bai Y, Wang T, Wang J, Zhang L and Li Y (2024) Optimal exercise dose-response improves health-related quality of life in cancer survivors: a systematic review and Bayesian network meta-analysis of RCTs. Front. Oncol. 14:1510578. doi: 10.3389/fonc.2024.1510578
Received: 13 October 2024; Accepted: 08 November 2024;
Published: 16 December 2024.
Edited by:
İsmail Toygar, Mugla University, TürkiyeReviewed by:
Nurpudji Astuti Taslim, Hasanuddin University, IndonesiaCopyright © 2024 Xiong, Yuan, Yang, Qiu, Bai, Wang, Wang, Zhang and Li. This is an open-access article distributed under the terms of the Creative Commons Attribution License (CC BY). The use, distribution or reproduction in other forums is permitted, provided the original author(s) and the copyright owner(s) are credited and that the original publication in this journal is cited, in accordance with accepted academic practice. No use, distribution or reproduction is permitted which does not comply with these terms.
*Correspondence: Yawen Li, MzQyOUBlY2p0dS5lZHUuY24=
†These authors have contributed equally to this work
Disclaimer: All claims expressed in this article are solely those of the authors and do not necessarily represent those of their affiliated organizations, or those of the publisher, the editors and the reviewers. Any product that may be evaluated in this article or claim that may be made by its manufacturer is not guaranteed or endorsed by the publisher.
Research integrity at Frontiers
Learn more about the work of our research integrity team to safeguard the quality of each article we publish.