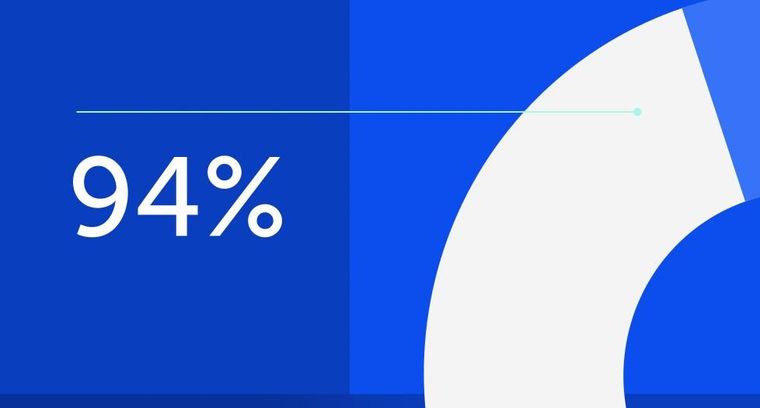
94% of researchers rate our articles as excellent or good
Learn more about the work of our research integrity team to safeguard the quality of each article we publish.
Find out more
SYSTEMATIC REVIEW article
Front. Oncol., 15 January 2025
Sec. Cancer Epidemiology and Prevention
Volume 14 - 2024 | https://doi.org/10.3389/fonc.2024.1506366
Objective: The prevalence of long COVID among cancer patients remains unknown. This study aimed to determine the prevalence of long COVID and explore potential risk factors among cancer patients.
Methods: A systematic search was performed on PubMed, Web of Science, and Embase from database inception until 21 March 2024, to identify studies that reported long COVID in cancer patients. Two investigators independently screened the studies and extracted all information about long COVID in cancer patients for subsequent analysis. Methodological quality was assessed using the “Joannagen Briggs Institute (JBI) Critical Appraisal Checklist for Studies Reporting Prevalence Data”.
Results: A total of 13 studies involving 6,653 patients were included. The pooled prevalence of long COVID was 23.52% [95% confidence interval (CI), 12.14% to 40.64%] among cancer patients reported experiencing long COVID after acute severe acute respiratory syndrome coronavirus 2 (SARS-CoV-2) infection. The pooled prevalence of any long COVID in cancer patients was 20.51% (95% CI, 15.91% to 26.03%), 15.79% (95% CI, 11.39% to 21.47%), and 12.54% (95% CI, 6.38% to 23.18%) in 3, 6, and 12 months follow-up duration. Fatigue was the most common symptom, followed by respiratory symptoms, myalgia, and sleep disturbance. Patients with comorbidities had a significantly higher risk of experiencing long COVID [odds ratio (OR) = 1.72; 95% CI, 1.09 to 2.70; p = 0.019]. No statistically significant differences in sex, primary tumor, or tumor stage were detected.
Conclusion: Nearly a quarter of cancer patients will experience long COVID after surviving from SARS-CoV-2 infection, and this would even last for 1 year or longer. Fatigue, respiratory symptoms, myalgia, and sleep disturbance need to be more addressed and managed to reduce symptom burden on cancer patients and improve quality of life. Patients with comorbidities are at a high risk of developing long COVID. Further randomized controlled trials with rigorous methodological designs and large sample sizes are needed for future validation.
Systematic review registration: https://www.crd.york.ac.uk/PROSPERO/, identifier CRD42023456665.
Severe acute respiratory syndrome coronavirus 2 (SARS-CoV-2) continues to spread rapidly worldwide. According to the World Health Organization (WHO) Coronavirus (COVID-19) Dashboard, there have been over 772 million confirmed cases of COVID-19 worldwide, and nearly 7 million people have died from it (1). After recovery from acute infection, many patients will still experience persistent symptoms such as cough, dyspnea, and fatigue (2–4), which is known as long COVID. The prevalence of long COVID-19 is high (5, 6), independent of the severity of initial COVID-19 presentation (7). However, the definition of long COVID has not been universally agreed upon. “Long COVID” (includes both ongoing symptomatic COVID-19 from 4 to 12 weeks) was named by the UK National Institute for Health and Care Excellence (NICE) (8), “post-COVID-19 condition” (3 months from the onset of COVID-19 and with symptoms that last for at least 2 months and cannot be explained by an alternative diagnosis) was named by the WHO (9), and “Long COVID” (at least 4 weeks after infection is the start of when long COVID can first be identified) was named by the U.S. Centers for Disease Control and Prevention (CDC) (10), and approximately 65% of the studies do not use any of the three definitions (11).
Although 10%–30% of cancer patients die from SARS-CoV-2 infection (12–15), the majority of cancer patients recover from acute infection and are at risk of long COVID. One study revealed that compared with individuals not infected with COVID-19, patients with long COVID had greater mortality and healthcare utilization at the 1-year follow-up (16). Another study also revealed an association between long COVID and poorer survival outcomes in cancer patients (17), which may be related to providing less treatment, delaying treatment initiation, or promoting cancer progression (18). Understanding the long-term effects of COVID-19 in cancer patients is fundamental to trying to protect cancer patients from long COVID and the adverse events it may cause. However, because of the limited sample size and the wide variation in the reported prevalence of long COVID in cancer patients, no systematic reviews or meta-analyses have been published to address this issue.
Therefore, we conducted systematic reviews and meta-analyses of the existing evidence on long COVID, to determine the prevalence of long COVID and explore potential risk factors. The results of this study will help fill a significant knowledge gap about the lingering effects of COVID-19 on cancer patient outcomes.
This systematic review was performed according to the Preferred Reporting Items for Systematic Review and Meta-Analysis (PRISMA) (19), and the protocol was registered at PROSPERO (No. CRD42023456665).
Studies that assessed the long COVID among cancer patients were systematically searched in PubMed, Embase, and Web of Science from database inception to 21 March 2024. We used the following search terms: “COVID-19”, “Long COVID”, “Symptom”, and “Cancer”. The detailed search strategy is provided in Supplementary Table S1. The reference lists of the included studies were manually searched for additional studies that met the inclusion criteria.
The inclusion criteria were as follows (1): include cancer patients infected with SARS-CoV-2; (2) measure long COVID symptoms; (3) assess symptoms at least 4 weeks after initial COVID-19 infection; and (4) provide data consisting entirely of cancer patients.
The exclusion criteria were as follows: (1) the study was a review, case report, conference abstract, or protocol; (2) the publications were repeated; (3) data or full texts were unavailable; and (4) non-English language publications.
Two investigators independently screened the titles and abstracts and removed irrelevant studies according to the inclusion and exclusion criteria. Two investigators extracted the following data: the characteristics of cancer patients, the test used for SARS-CoV-2 infection diagnosis, follow-up time, sample size, and outcomes. Any inconsistencies were resolved by discussion with a third investigator.
Two investigators independently assessed methodological quality using the “Joannagen Briggs Institute (JBI) Critical Appraisal Checklist for Studies Reporting Prevalence Data” (20). The checklist consists of nine questions (1): Was the sample frame appropriate to address the target population? (2) Were study participants recruited in an appropriate way? (3) Was the sample size adequate? (4) Were the study subjects and setting described in detail? (5) Was data analysis conducted with sufficient coverage of the identified sample? (6) Were valid methods used for the identification of the condition? (7) Was the condition measured in a standard, reliable way for all participants? (8) Was there appropriate statistical analysis? and (9) Was the response rate adequate, and if not, was the low response rate managed appropriately? Each item has four options: yes, no, unclear, or not applicable. An overall score was used to reflect the number of questions with an option of “yes”.
We only used the study with the largest sample size for each meta-analysis if certain patients could be included in several studies. The long COVID ratio was used as the original proportion data. The Shapiro−Wilk normality test was employed to determine whether the data fit a normal distribution. If the measurement data conformed to a normal distribution, the original proportion was adopted for analysis; otherwise, the Freeman–Tukey double arcsine transformation was used to adjust the data. τ2 was used to estimate the between-study variance. The I2 value was used to assess heterogeneity (21). If I2 ≥ 50%, a random-effects model was used; otherwise, a fixed-effects model was used (22). The odds ratio (OR) and 95% confidence interval (CI) were used to pool dichotomous variables. Publication bias was examined using Egger’s test, and subgroup analyses were performed based on follow-up duration. Meta-analyses were pooled using the R Software (version 4.3.2 R Project for Statistical Computing, Vienna, Austria) and the “meta” R package (23). p-value < 0.05 was considered statistically significant.
For the analysis of each symptom, we found that most of the studies reported only the symptoms with a higher prevalence instead of all data on symptoms they investigate. Because it is inappropriate to combine these data directly, which might bias results, we used a systematic review to summarize symptoms with higher frequency.
A total of 1,570 records were identified from the initial database search, and 254 duplicate papers were removed. Of these, 1,316 records remained, and 1,274 records were excluded after screening the titles or abstracts. Furthermore, 42 records were needed to read the full text, and ultimately, a total of 13 studies (17, 24–35), including 6,653 cancer patients, were included in the meta-analysis. The study selection flowchart is shown in Figure 1.
These studies were conducted in two studies each in the USA (24, 25), the UK (28, 32), France (27, 34), and Spain (33, 35), and one each in China (29), India (26), and Italy (30). Two studies (17, 31) both used cross-sectional data from the OnCoVID study, which is an active European registry study enrolling consecutive patients from Belgium, France, Germany, Italy, Spain, and the UK (17). All the patients were followed up for more than 4 weeks, and the follow-up duration ranged from 28 days to 14 months. Only 10 studies (17, 24, 25, 27–32, 35) used long COVID in cancer patients as an outcome measure; others (26, 33, 34) just reported the prevalence of long COVID in cancer patients. One study (32) included 94 hematology patients, 90 of whom had a hematological malignancy, and the remain 4 had non-malignant hematological diseases. Six studies (28, 30, 32–35) included patients with a diagnosis of COVID-19 and did not explicitly mention how to test for a COVID-19 diagnosis. The characteristics of the included studies are presented in Table 1.
Nine studies (17, 24, 25, 27–29, 31, 32) with scores above 7 points indicated that most studies were of a high quality. One study (30) scored 6 points, two studies (33, 34) scored 4 points, and one (26) scored 2 points. Four studies (26, 27, 30, 33) did not report the number of patients lost to follow-up, and two retrospective studies (17, 31) were not applicable, which is the main problem for quality assessment. Second, five studies (24, 26, 28, 30, 32) did not have sufficient sample size. Four studies (26, 29, 33, 34) did not report the demographic information of cancer patients in detail. The detailed assessment results are shown in Supplementary Table S2.
Because two studies (17, 31) used a portion of data from the OnCoVID study, only the study with a larger sample size was included in the meta-analysis to prevent duplication of patients. We performed a pooled analysis of 12 studies comprising 6,467 patients. The results of the random-effects model (I² = 98.3%) showed that approximately 23.52% (95% CI, 12.14% to 40.64%) of cancer patients experienced long COVID after acute SARS-CoV-2 infection (Figure 2).
We conducted a sensitivity analysis by eliminating one study at a time (Figure 3). After the removal of one study (33), heterogeneity was significantly reduced from I² = 98.3% to I² = 96.7%, and the pooled estimated prevalence of long COVID increased from 23.52% to 29.9%. This suggested that the study may have a potential impact on the results. Egger’s tests were performed for the prevalence of long COVID, and the results revealed that no publication bias was observed (p = 0.88).
Five studies (24, 26, 29, 33, 34) reported long COVID in both cancer patients and patients with no cancer diagnosis. The results of the random-effects model (I² = 73.3%) revealed that there was no statistical difference between cancer patients and non-cancer patients with SARS-COV-2 infection (OR = 1.24; 95% CI, 0.78 to 1.97; p = 0.37).
Four studies (24, 30, 32, 35) reported a long-term follow-up of 3 months. The results of the random-effects model (I² = 50.7%) revealed that approximately 20.51% (95% CI, 15.91% to 26.03%) of cancer patients experienced long COVID.
Four studies (24, 27, 31, 35) reported a long-term follow-up of 6 months. The results of the random-effects model (I² = 70%) showed that approximately 15.79% (95% CI, 11.39% to 21.47%) of cancer patients experienced long COVID.
Three studies (24, 29, 31) reported a long-term follow-up of 12 months. The proportion did not satisfy a normal distribution (p = 0.038). After using Freeman–Tukey double-arcsine transformation, the data conformed to a normal distribution. The results of the random-effects model (I² = 79.7%) showed that approximately 12.54% (95% CI, 6.38% to 23.18%) of cancer patients still experienced long COVID. The results of subgroup analysis are shown in the Supplementary Materials.
Six studies (17, 25, 27, 28, 30, 35) involved five risk factors that were reported by more than three studies. Three studies (27, 28, 35) compared patients with comorbidities to patients without comorbidities. The results of the fixed-effects model (I² = 0%) revealed that patients with comorbidities had a significantly higher risk of experiencing long COVID (OR = 1.72; 95% CI, 1.09 to 2.7; p = 0.019). In addition, another study (17) indicated that cancer patients with more than two comorbidities were at a higher risk of experiencing long COVID (p < 0.001). Five studies (17, 25, 27, 28, 35) reported the risk between hospitalized and non-hospitalized patients with cancer. The results of the random-effects model (I² = 88.6%) revealed that no significant difference was observed (OR = 1.84; 95% CI, 0.89 to 3.80; p = 0.102). However, a study (32) involving 94 hematological patients indicated that hospitalization would increase the risk of long COVID. However, there were four patients with non-malignant hematological diseases that could not be eliminated. The study was not included in the combined sample. Furthermore, no significant difference was observed in sex, primary tumor, or tumor stage (Table 2).
Because of the different criteria, we could not perform a meta-analysis of the associations. Five studies (17, 27, 28, 30, 35) contrasted different age intervals, and only one study indicated that there was a statistically significant difference in that cancer patients over the age of 65 years were more likely to have long COVID (p = 0.048) [17]. Different anti-tumor treatment strategies were not statistically associated with long COVID in three studies (17, 27, 35). Two studies revealed that obese patients had a significantly higher risk of experiencing long COVID (27, 30). Pinato et al. (17) reported that patients with long COVID were more likely to have a history of smoking than those with no history (p < 0.001), and reported that the distribution of primary tumor was also significantly different (p = 0.048). Lasagna et al. (30) reported that type 2 diabetes mellitus (T2DM) is associated with long COVID (p = 0.004).
A total of six studies (17, 25, 27–30) reported the long COVID-specific symptoms with a higher frequency. Fatigue was the most common symptom that occurred in cancer patients after SARS-CoV-2 infection. Respiratory symptoms, myalgia, sleep disturbance, and cognitive impairment were reported in two or more studies. Dyspnea, brain fog, dizziness, loss of taste or smell, weight loss, and gastrointestinal symptoms were also reported in only one study (Table 3).
Only three studies (17, 25, 31) presented outcomes after COVID-19. Dagher et al. (25) reported that no long COVID cancer patient had a higher mortality beyond 30 days of initial COVID-19 diagnosis (p < 0.001). Pinato et al. (17) reported that long COVID may cause more adjustments or discontinuations of systemic anti-cancer therapy (SACT) (p = 0.021) and the discontinuations of SACT were confirmed to be independently associated with an increased risk of death (p = 0.002). However, Cortellini et al. (31) reported that disease progression at 6 months had no significant difference between patients who experienced long COVID and those who did not experience long COVID (p = 0.139).
This systematic review and meta-analysis investigated the prevalence of long COVID in cancer patients. We found that approximately 23.52% of cancer patients reported experiencing long COVID after acute SARS-CoV-2 infection. A recent meta-analysis of 137 studies revealed that the prevalence of any long COVID was more than 40% in healthy adults (36). However, our finding did not observe any significant differences between cancer patients and those with no cancer.
We also found that long COVID symptoms decreased over time as the follow-up duration increased. This is similar to findings from studies of other populations (37). These findings indicate that long COVID may result in complete recovery even if the time until full recovery is long. Notably, approximately 12.54% of cancer patients reported experiencing long COVID after 12 months of being infected with SARS-CoV-2. In a longitudinal cohort study, COVID-19 survivors had a markedly lower health status than the general population at 2 years (38). Long COVID has been shown to affect multiple organ systems. A long-lasting reduction in vascular density, specifically affecting small capillaries, was found in patients with long COVID compared with controls 18 months after infection, with the potential to affect oxygen delivery (39). Compared with non-COVID-19 controls, people with long COVID have been found to have gut dysbiosis lasting at least 14 months (40).
Owing to the lack of complete data on long COVID symptoms in each study, we used a qualitative descriptive approach to summarize symptoms with a higher frequency. Our findings indicated that fatigue is the symptom with the highest frequency, followed by respiratory symptoms, myalgia, and sleep disturbance. A similar meta-analysis investigated the symptoms of post-COVID-19 patients from 3 to 12 months after COVID-19 hospitalization and revealed that more than 40% of patients experienced fatigue and breathlessness, followed by cough, sleep disturbance, depression, and loss of taste or smell. In addition, long COVID can impact patients’ quality of life (41). A meta-analysis indicated that long COVID patients may experience persistent decreases in quality of life up to 6 months after infection (4). Persistent symptoms and decreased quality of life may not be significantly associated with disease severity (42).
Significant differences in long COVID risk between patients with comorbidities and patients with no comorbidities were found in this meta-analysis. A similar phenomenon was observed in a previous cohort study conducted in China (5) that reported that having two or more comorbidities resulted in a higher risk of developing long COVID at the 6-month follow-up. There were studies that showed that obesity or having T2DM was significantly associated with a higher risk of long COVID, as previous meta-analysis research had suggested (43). Obesity and long COVID share a metabolic proinflammatory state that promotes associated signs and symptoms to linger for a prolonged period of time (44). T2DM has a bidirectional relationship with COVID-19, and COVID-19 itself has been postulated to cause diabetes and to worsen glycemic control in pre-existing diabetes (45). A long duration of uncontrolled diabetes causes organ damage, and in particular, microvascular injury may contribute to long COVID (46). In addition, having a history of smoking may be associated with experiencing long COVID. Smoke has been shown to be a significant risk factor for both long COVID and severe acute COVID-19 (47, 48). Cigarette smoke exposure increases ACE2, which may increase the risk of developing severe COVID-19 as well as a higher mortality (49–51). It is still controversial whether older age is an independent risk factor for long COVID in cancer patients. A meta-analysis revealed that individuals 40 to 69 years old and those 70 years or older are at equally high risk of long COVID (43). There is an assumption that it is difficult for older patients who are at high risk of long COVID to survive from acute COVID-19 infection (52). No association was observed between long COVID and sex, which is inconsistent with the findings of previous studies. Sylvester et al. (53) reported that the likelihood of having long COVID syndrome was significantly greater among female individuals. A UK study including more than 480,000 COVID-19 patients indicated that female sex was a risk factor for long COVID (54). Further research is needed to explore whether female sex is a characteristic of long COVID in cancer patients or it is the result of heterogeneity and bias. In addition, we found that there were no significant differences between the difference in primary tumor, anti-tumor treatment strategies, or tumor stage.
This systematic review and meta-analysis has several strengths. First, this study is the first study to evaluate the association between long COVID and cancer. Second, we also performed subgroup analysis according to the follow-up time and detected publication bias. Third, the only study that included both patients with risk factors and patients without risk factors was included in the analysis to decrease the heterogeneity of the different population. However, certain limitations still exist in our study. First, the included studies and sample size were limited. Although cancer patients should be more focused on and protected during the COVID-19 pandemic, few studies have reported the symptoms of long COVID among cancer patients. Second, there was considerable heterogeneity among the studies, possibly caused by differences in strain, race, geographic location, time of onset of illness, and measurement tools. Third, most studies did not show all of the symptoms they investigated, making it difficult to analyze. The full results of the studies should be encouraged to be indicated. Fourth, few studies have focused on the risk factors for long COVID in cancer patients or the disease outcomes after infection.
In our findings, nearly a quarter of cancer patients will experience long COVID after surviving from SARS-CoV-2 infection, and this may even last for 1 year or longer. Fatigue, myalgia, cough, and sleep disturbance need to be more addressed and managed to reduce the symptom burden in cancer patients and improve their quality of life. Patients with comorbidities are at a high risk of developing long COVID. However, owing to the above limitations, our results need to be further verified by more studies with higher methodological quality.
The original contributions presented in the study are included in the article/Supplementary Material. Further inquiries can be directed to the corresponding author.
HX: Conceptualization, Data curation, Formal analysis, Investigation, Methodology, Project administration, Resources, Software, Supervision, Validation, Visualization, Writing – original draft, Writing – review & editing. TL: Conceptualization, Data curation, Formal analysis, Investigation, Methodology, Project administration, Resources, Supervision, Validation, Visualization, Writing – original draft, Writing – review & editing. YL: Data curation, Funding acquisition, Resources, Writing – review & editing. JY: Data curation, Funding acquisition, Methodology, Writing – review & editing. SR: Writing – review & editing, Data curation, Funding acquisition, Investigation. BH: Writing – review & editing, Funding acquisition, Investigation. HL: Conceptualization, Formal analysis, Methodology, Writing – review & editing, Software. LG: Conceptualization, Data curation, Formal analysis, Investigation, Methodology, Project administration, Resources, Validation, Writing – review & editing. JL: Conceptualization, Data curation, Formal analysis, Funding acquisition, Investigation, Methodology, Project administration, Resources, Software, Supervision, Validation, Visualization, Writing – review & editing.
The author(s) declare financial support was received for the research, authorship, and/or publication of this article. This work was supported by the Scientific and Technological Innovation Project of China Academy of Chinese Medical Sciences (CI2022C004) and the Fundamental Research Funds for the Central public welfare research institutes (ZZ15-WT-05).
The authors sincerely thank the support of Beijing University of Chinese Medicine and Guang’anmen Hospital.
The authors declare that the research was conducted in the absence of any commercial or financial relationships that could be construed as a potential conflict of interest.
The author(s) declare that no Generative AI was used in the creation of this manuscript.
All claims expressed in this article are solely those of the authors and do not necessarily represent those of their affiliated organizations, or those of the publisher, the editors and the reviewers. Any product that may be evaluated in this article, or claim that may be made by its manufacturer, is not guaranteed or endorsed by the publisher.
The Supplementary Material for this article can be found online at: https://www.frontiersin.org/articles/10.3389/fonc.2024.1506366/full#supplementary-material
1. World Health Organization. Available online at: https://www.who.int/emergencies/diseases/novel-coronavirus-2019 (Accessed Match 21st, 2024).
2. Daher A, Balfanz P, Cornelissen C, Müller A, Bergs I, Marx N, et al. Follow up of patients with severe coronavirus disease 2019 (COVID-19): Pulmonary and extrapulmonary disease sequelae. Resp Med. (2020) 174:106197. doi: 10.1016/j.rmed.2020.106197
3. Jacobs L, Gourna E, Lesky-Di D, Nyirenda T, Friedman T, Gupta A, et al. Persistence of symptoms and quality of life at 35 days after hospitalization for COVID-19 infection. PloS One. (2020) 15:e0243882. doi: 10.1371/journal.pone.0243882
4. Sanchez-Ramirez D, Normand K, Zhaoyun Y, Torres-Castro R. Long-term impact of COVID-19: A systematic review of the literature and meta-analysis. Biomedicines. (2021) 9:8. doi: 10.3390/biomedicines9080900
5. Huang C, Huang L, Wang Y, Li X, Ren L, Gu X, et al. 6-month consequences of COVID-19 in patients discharged from hospital: a cohort study. Lancet (London England). (2023) 401:e21–33. doi: 10.1016/S0140-6736(23)00810-3
6. Hayes L, Ingram J, Sculthorpe N. More than 100 persistent symptoms of SARS-coV-2 (Long COVID): A scoping review. Front Med. (2021) 8:750378. doi: 10.3389/fmed.2021.750378
7. Xie Y, Xu E, Bowe B, Al-Aly Z. Long-term cardiovascular outcomes of COVID-19. Nat Med. (2022) 28:583–90. doi: 10.1038/s41591-022-01689-3
8. National Institute for Health and Care Excellence. COVID-19 rapid guideline: managing the long-term effects of COVID-19 (2024). Available online at: https://www.nice.org.uk/guidance/ng188 (Accessed Match 21st, 2024).
9. Soriano J, Murthy S, Marshall J, Relan P, Diaz J. A clinical case definition of post-COVID-19 condition by a Delphi consensus. Lancet Infect Dis. (2022) 22:e102–7. doi: 10.1016/S1473-3099(21)00703-9
10. Centers for Disease Control and Prevention. Long COVID or post-COVID conditions (2022). Available online at: https://archive.cdc.gov/#/details?url=https://www.cdc.gov/coronavirus/2019-ncov/long-term-effects/index.html (Accessed Match 21st, 2024).
11. Chaichana U, Man K, Chen A, Wong I, George J, Wilson P, et al. Definition of post-COVID-19 condition among published research studies. JAMA network Open. (2023) 6:e235856. doi: 10.1001/jamanetworkopen.2023.5856
12. Lee L, Cazier J, Angelis V, Arnold R, Bisht V, Campton N, et al. COVID-19 mortality in patients with cancer on chemotherapy or other anticancer treatments: a prospective cohort study. Lancet (London England). (2020) 395:1919–26. doi: 10.1016/S0140-6736(20)31173-9
13. Garassino M, Whisenant J, Huang L, Trama A, Torri V, Agustoni F, et al. COVID-19 in patients with thoracic Malignancies (TERAVOLT): first results of an international, registry-based, cohort study. Lancet Oncol. (2020) 21:914–22. doi: 10.1016/S1470-2045(20)30314-4
14. Lee L, Cazier J, Starkey T, Briggs S, Arnold R, Bisht V, et al. COVID-19 prevalence and mortality in patients with cancer and the effect of primary tumour subtype and patient demographics: a prospective cohort study. Lancet Oncol. (2020) 21:1309–16. doi: 10.1016/S1470-2045(20)30442-3
15. Kuderer N, Choueiri T, Shah D, Shyr Y, Rubinstein S, Rivera D, et al. Clinical impact of COVID-19 on patients with cancer (CCC19): a cohort study. Lancet (London England). (2020) 395:1907–18. doi: 10.1016/S0140-6736(20)31187-9
16. Devries A, Shambhu S, Sloop S, Overhage J. One-year adverse outcomes among US adults with post-COVID-19 condition vs those without COVID-19 in a large commercial insurance database. JAMA Health Forum. (2023) 4:e230010. doi: 10.1001/jamahealthforum.2023.0010
17. Pinato D, Tabernero J, Bower M, Scotti L, Patel M, Colomba E, et al. Prevalence and impact of COVID-19 sequelae on treatment and survival of patients with cancer who recovered from SARS-CoV-2 infection: evidence from the OnCovid retrospective, multicentre registry study. Lancet Oncol. (2021) 22:1669–80. doi: 10.1016/S1470-2045(21)00573-8
18. Clark J, Dwyer D, Pinwill N, Clark P, Johnson P, Hackshaw A. The effect of clinical decision making for initiation of systemic anticancer treatments in response to the COVID-19 pandemic in England: a retrospective analysis. Lancet Oncol. (2021) 22:66–73. doi: 10.1016/S1470-2045(20)30619-7
19. Page M, Mckenzie J, Bossuyt P, Boutron I, Hoffmann T, Mulrow C, et al. The PRISMA 2020 statement: an updated guideline for reporting systematic reviews. BMJ (Clinical Res ed.). (2021) 372:n71. doi: 10.1136/bmj.n71
20. Munn Z, Moola S, Lisy K, Riitano D, Tufanaru C. Methodological guidance for systematic reviews of observational epidemiological studies reporting prevalence and cumulative incidence data. Int J Evid Based Healthc. (2015) 13:147–53. doi: 10.1097/XEB.0000000000000054
21. Rücker G, Schwarzer G, Carpenter J, Schumacher M. Undue reliance on I(2) in assessing heterogeneity may mislead. BMC Med Res Methodol. (2008) 8:79. doi: 10.1186/1471-2288-8-79
22. Borenstein M, Hedges L, Higgins J, Rothstein H. A basic introduction to fixed-effect and random-effects models for meta-analysis. Res Synth Methods. (2010) 1:97–111. doi: 10.1002/jrsm.12
23. Viechtbauer W. Conducting meta-analyses in R with the metafor package. J Stat Software. (2010) 36:1–48. doi: 10.18637/jss.v036.i03
24. Fankuchen O, Lau J, Rajan M, Swed B, Martin P, Hidalgo M, et al. Long COVID in cancer: A matched cohort study of 1-year mortality and long COVID prevalence among patients with cancer who survived an initial severe SARS-coV-2 infection. Am J Clin Oncol. (2023) 46:300–5. doi: 10.1097/COC.0000000000001005
25. Dagher H, Chaftari A, Subbiah I, Malek A, Jiang Y, Lamie P, et al. Long COVID in cancer patients: preponderance of symptoms in majority of patients over long time period. Elife. (2023) 12:e81182. doi: 10.7554/eLife.81182
26. Nair P, Nair C, Kulirankal K, Corley EM, Edathadathil F, Gutjahr G, et al. Characterization and predictive risk scoring of long COVID in a south Indian cohort after breakthrough COVID infection; a prospective single centre study. BMC Infect Dis. (2023) 23:670. doi: 10.1186/s12879-023-08600-6
27. Hajjaji N, Lepoutre K, Lakhdar S, Bécourt S, Bellier C, Kaczmarek E, et al. 16 months follow up of patients' Behavior and mild COVID-19 patterns in a large cohort of cancer patients during the pandemic. Front Oncol. (2022) 12:901426. doi: 10.3389/fonc.2022.901426
28. Monroy-Iglesias M, Tremble K, Russell B, Moss C, Dolly S, Sita-Lumsden A, et al. Long-term effects of COVID-19 on cancer patients: the experience from Guy's Cancer Centre. Future Oncol (London England). (2022) 18:3585–94. doi: 10.2217/fon-2022-0088
29. Chai C, Feng X, Lu M, Li S, Chen K, Wang H, et al. One-year mortality and consequences of COVID-19 in cancer patients: A cohort study. IUBMB Life. (2021) 73:1244–56. doi: 10.1002/iub.2536
30. Lasagna A, Albi G, Figini S, Basile S, Sacchi P, Bruno R, et al. Long-COVID in patients with cancer previously treated with early anti-SARS-coV-2 therapies in an out-of-hospital setting: A single-center experience. Cancers. (2023) 15:1269. doi: 10.3390/cancers15041269
31. Cortellini A, Salazar R, Gennari A, Aguilar-Company J, Bower M, Bertuzzi A, et al. Persistence of long-term COVID-19 sequelae in patients with cancer: An analysis from the OnCovid registry. Eur J Cancer (Oxford England: 1990). (2022) 170:10–6. doi: 10.1016/j.ejca.2022.03.019
32. Willan J, Agarwal G, Bienz N. Mortality and burden of post-COVID-19 syndrome have reduced with time across SARS-CoV-2 variants in haematology patients. Brit J Haematol. (2023) 201:640–4. doi: 10.1111/bjh.18700
33. de Arriba Fernández A, Alonso Bilbao J, Espiñeira Francés A, Cabeza Mora A, Gutiérrez Pérez Á, Díaz Barreiros M, et al. Assessment of SARS-coV-2 infection according to previous metabolic status and its association with mortality and post-acute COVID-19. Nutrients. (2022) 14. doi: 10.3390/nu14142925
34. Robineau O, Zins M, Touvier M, Wiernik E, Lemogne C, de Lamballerie X, et al. Long-lasting symptoms after an acute COVID-19 infection and factors associated with their resolution. JAMA network Open. (2022) 5:e2240985. doi: 10.1001/jamanetworkopen.2022.40985
35. Martinez-Lopez J, de la Cruz J, Gil-Manso R, Cedillo A, Alegre A, Sillero M, et al. Acute and post-acute COVID-19 severity and mortality in patients with hematologic Malignancies: A population-based registry study. Blood. (2021) 138:186. doi: 10.1182/blood-2021-152539
36. Huang Q, Jia M, Sun Y, Jiang B, Cui D, Feng L, et al. One-year temporal changes in long COVID prevalence and characteristics: A systematic review and meta-analysis. Value health: J Int Soc Pharmacoeconomics Outcomes Res. (2023) 26:934–42. doi: 10.1016/j.jval.2022.11.011
37. Han Q, Zheng B, Daines L, Sheikh A. Long-term sequelae of COVID-19: A systematic review and meta-analysis of one-year follow-up studies on post-COVID symptoms. Pathog (Basel Switzerland). (2022) 11:269. doi: 10.3390/pathogens11020269
38. Huang L, Li X, Gu X, Zhang H, Ren L, Guo L, et al. Health outcomes in people 2 years after surviving hospitalisation with COVID-19: a longitudinal cohort study. Lancet Respir Med. (2022) 10:863–76. doi: 10.1016/S2213-2600(22)00126-6
39. Kubánková M, Hohberger B, Hoffmanns J, Fürst J, Herrmann M, Guck J, et al. Physical phenotype of blood cells is altered in COVID-19. Biophys J. (2021) 120:2838–47. doi: 10.1016/j.bpj.2021.05.025
40. Liu Q, Mak J, Su Q, Yeoh Y, Lui G, Ng S, et al. Gut microbiota dynamics in a prospective cohort of patients with post-acute COVID-19 syndrome. Gut. (2022) 71:544–52. doi: 10.1136/gutjnl-2021-325989
41. Cai J, Lin K, Zhang H, Xue Q, Zhu K, Yuan G, et al. A one-year follow-up study of systematic impact of long COVID symptoms among patients post SARS-CoV-2 omicron variants infection in Shanghai, China. Emerg Microbes Infect. (2023) 12:2220578. doi: 10.1080/22221751.2023.2220578
42. Garrigues E, Janvier P, Kherabi Y, Le Bot A, Hamon A, Gouze H, et al. Post-discharge persistent symptoms and health-related quality of life after hospitalization for COVID-19. J infection. (2020) 81:e4–6. doi: 10.1016/j.jinf.2020.08.029
43. Tsampasian V, Elghazaly H, Chattopadhyay R, Debski M, Naing T, Garg P, et al. Risk factors associated with post-COVID-19 condition: A systematic review and meta-analysis. JAMA Intern Med. (2023) 183:566–80. doi: 10.1001/jamainternmed.2023.0750
44. Florencio L, Fernández-De-Las-Peñas C. Long COVID: systemic inflammation and obesity as therapeutic targets. Lancet Respir Med. (2022) 10:726–7. doi: 10.1016/S2213-2600(22)00159-X
45. Unnikrishnan R, Misra A. Diabetes and COVID19: a bidirectional relationship. Nutr Diabetes. (2021) 11:21. doi: 10.1038/s41387-021-00163-2
46. Raveendran A, Misra A. Post COVID-19 syndrome ("Long COVID") and diabetes: challenges in diagnosis and management. Diabetes Metab syndrome. (2021) 15:102235. doi: 10.1016/j.dsx.2021.102235
47. Barthélémy H, Mougenot E, Duracinsky M, Salmon-Ceron D, Bonini J, Péretz F, et al. Smoking increases the risk of post-acute COVID-19 syndrome: Results from a French community-based survey. Tob Induc Dis. (2022) 20:59. doi: 10.18332/tid/150295
48. Reddy RK, Charles WN, Sklavounos A, Dutt A, Seed PT, Khajuria A. The effect of smoking on COVID-19 severity: A systematic review and meta-analysis. J Med Virol. (2021) 93:1045–56. doi: 10.1002/jmv.26389
49. Jacobs M, Van Eeckhoutte H, Wijnant S, Janssens W, Joos G, Brusselle G, et al. Increased expression of ACE2, the SARS-CoV-2 entry receptor, in alveolar and bronchial epithelium of smokers and COPD subjects. Eur Respir J. (2020) 56:2002378. doi: 10.1183/13993003.02378-2020
50. Aloufi N, Traboulsi H, Ding J, Fonseca G, Nair P, Huang S, et al. Angiotensin-converting enzyme 2 expression in COPD and IPF fibroblasts: the forgotten cell in COVID-19. Am J Physiol Lung Cell Mol Physiol. (2021) 320:L152–7. doi: 10.1152/ajplung.00455.2020
51. Bao L, Deng W, Huang B, Gao H, Liu J, Ren L, et al. The pathogenicity of SARS-CoV-2 in hACE2 transgenic mice. Nature. (2020) 583:830–3. doi: 10.1038/s41586-020-2312-y
52. Romero Starke K, Reissig D, Petereit-Haack G, Schmauder S, Nienhaus A, Seidler A. The isolated effect of age on the risk of COVID-19 severe outcomes: a systematic review with meta-analysis. BMJ Global Health. (2021) 6:e006434. doi: 10.1136/bmjgh-2021-006434
53. Sylvester S, Rusu R, Chan B, Bellows M, O'Keefe C, Nicholson S. Sex differences in sequelae from COVID-19 infection and in long COVID syndrome: a review. Curr Med Res Opin. (2022) 38:1391–9. doi: 10.1080/03007995.2022.2081454
Keywords: cancer, COVID-19, long COVID, prevalence, risk factors
Citation: Xu H, Lu T, Liu Y, Yang J, Ren S, Han B, Lai H, Ge L and Liu J (2025) Prevalence and risk factors for long COVID among cancer patients: a systematic review and meta-analysis. Front. Oncol. 14:1506366. doi: 10.3389/fonc.2024.1506366
Received: 05 October 2024; Accepted: 17 December 2024;
Published: 15 January 2025.
Edited by:
Alireza Sadjadi, Shariati Hospital, Tehran University of Medical Sciences, IranReviewed by:
Angioletta Lasagna, San Matteo Hospital Foundation (IRCCS), ItalyCopyright © 2025 Xu, Lu, Liu, Yang, Ren, Han, Lai, Ge and Liu. This is an open-access article distributed under the terms of the Creative Commons Attribution License (CC BY). The use, distribution or reproduction in other forums is permitted, provided the original author(s) and the copyright owner(s) are credited and that the original publication in this journal is cited, in accordance with accepted academic practice. No use, distribution or reproduction is permitted which does not comply with these terms.
*Correspondence: Jie Liu, ZHIubGl1amllQDE2My5jb20=
†These authors have contributed equally to this work and share first authorship
Disclaimer: All claims expressed in this article are solely those of the authors and do not necessarily represent those of their affiliated organizations, or those of the publisher, the editors and the reviewers. Any product that may be evaluated in this article or claim that may be made by its manufacturer is not guaranteed or endorsed by the publisher.
Research integrity at Frontiers
Learn more about the work of our research integrity team to safeguard the quality of each article we publish.