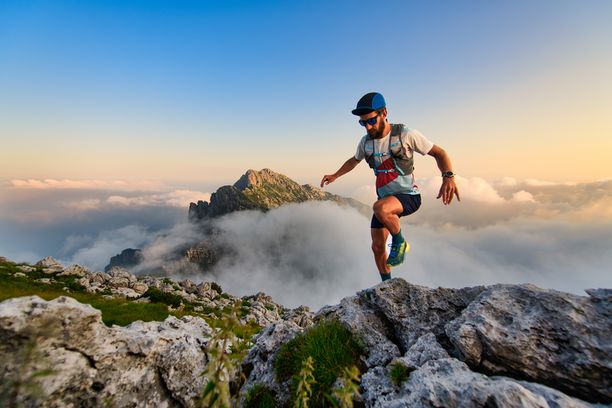
94% of researchers rate our articles as excellent or good
Learn more about the work of our research integrity team to safeguard the quality of each article we publish.
Find out more
REVIEW article
Front. Oncol., 04 November 2024
Sec. Skin Cancer
Volume 14 - 2024 | https://doi.org/10.3389/fonc.2024.1500300
Non-melanoma skin cancer (NMSC) mainly includes basal cell carcinoma, cutaneous squamous cell carcinoma, and Merkel cell carcinoma, showing a low mortality rate but the highest incidence worldwide. In recent decades, research has focused on understanding the pathogenesis and clinical treatments of NMSC, leading to significant advances in our knowledge of these diseases and the development of novel therapies, including immunotherapy. Nevertheless, the low to moderate objective response rate, high recurrence, and therapeutic resistance remain persistent challenges, which are partly attributable to the intratumoral heterogeneity. This heterogeneity indicates that tumor cells, immune cells, and stromal cells in the tumor microenvironment can be reshaped to a series of phenotypic and transcriptional cell states that vary in invasiveness and treatment responsiveness. The advent of single-cell RNA sequencing (scRNA-seq) has enabled the comprehensive profiling of gene expression heterogeneity at the single-cell level, which has been applied to NMSC to quantify cell compositions, define states, understand tumor evolution, and discern drug resistance. In this review, we highlight the key findings, with a focus on intratumoral heterogeneity and the mechanism of drug resistance in NMSC, as revealed by scRNA-seq. Furthermore, we propose potential avenues for future research in NMSC using scRNA-seq.
Non-melanoma skin cancer (NMSC) is the most prevalent malignant skin tumor, accounting for 99% of all skin cancers, with basal cell carcinoma (BCC) and cutaneous squamous cell carcinoma (cSCC) being the most two common types (1). Other less common types of NMSC encompass Merkel cell carcinoma (MCC), extramammary Paget’s disease (EMPD), and various skin adnexal carcinomas, including sebaceous carcinoma, apocrine adenocarcinoma, among others (2). The latest data shows that the worldwide incidence of new NMSC cases increased from 4.9 million in 2010 to 6.4 million in 2019, representing a 30.6% increase (3). This is attributable to a confluence of factors including increased life expectancy, heightened exposure to ultraviolet radiation (UVR), and advancements in data collection and diagnostic technologies (4). However, given the low mortality rate of most NMSCs and the accessibility of medical services in developing and underdeveloped countries (5), the global incidence of NMSC may be severely underestimated. Consequently, NMSC has now become a substantial economic burden (6).
With the development and innovation of next-generation sequencing (NGS) technologies, numerous dysregulated genes associated with their mutation, expression, and epigenetic modification during NMSC progression have been sought out (7–10). Bulk RNA-sequencing (RNA-seq) is a powerful tool for identifying tumor suppressor genes and oncogenes, showing advantages of low signal-to-noise ratio, and cost-effectiveness (11). Given that heterogeneity is the most defining feature of tumors (12), it is important to note that the limitations of this approach should be considered when applied to cancer biology, given its tissue-based nature. Firstly, bulk RNA-seq averages the gene expression across a pooled population of cells within the examined tissue, leading to a potential risk of masking the gene expression changes of rare cell populations. Furthermore, accurate cell composition cannot be obtained, despite the development of various deconvolution methods to estimate the cell composition from bulk RNA-seq data (13). The cell heterogeneity is evident not only in the variety of cell types and their respective states, but also in the dynamic plasticity during tumor progression and the drug resistance that develops after therapeutic interventions (14). Additionally, the evaluation of cell-to-cell complex crosstalk among the tumor microenvironment (TME) is also inaccessible by bulk RNA-seq. Fortunately, these difficulties are being increasingly resolved by the single-cell approach (15).
Single-cell RNA sequencing (scRNA-seq) is a novel approach that enables high-throughput transcriptome sequencing at the single-cell level, effectively tackling the challenge of transcriptomic heterogeneity among cells within a sample (Figure 1A). The technology of scRNA-seq can be used for cell composition evaluation, copy number variation (CNV) inference of tumor cells, pseudotime analysis for different cell clusters, and cell communications (Figure 1B). Since Tang et al. published the first scRNA-seq technology in 2009 (15), the field has undergone rapid development and widespread application in the life sciences, including tumor biology (16), development (17), immunology (18), neuroscience (19), and drug development (20). However, while scRNA-seq has revolutionized our understanding of cellular heterogeneity and function, some limitations are not obviously neglectable. The technique is subject to technical biases, such as doublets or drop-out events where true gene expression levels are not captured, leading to incomplete and inaccurate datasets (21, 22). Additionally, the low throughput of some single-cell platforms can limit the number of cells that can be analyzed in a single experiment, potentially missing rare cell types. The high cost and complexity of the technology can also be barriers to its widespread adoption. Furthermore, the interpretation of single-cell data requires sophisticated bioinformatics tools and expertise, which may introduce errors or biases in the analysis (23). Therefore, the results explored from the scRNA-seq technology should be confirmed further by various biological experiment. Actually, some reviews have discussed the application and advancement of scRNA-seq in skin diseases (24, 25) and skin cancer (26, 27), with a particular focus on melanoma (28). Here, we primarily focused on the application of scRNA-seq in dissecting intratumoral heterogeneity, drug resistance, and the specific mechanism of cell-cell interactions in the progression of NMSC.
Figure 1. General workflow of scRNA-seq experiments from sequencing to data analysis. (A) General workflow of scRNA-seq experiments in NMSCs. (B) General analyses for scRNA-seq data. Data was downloaded and analyzed with the accession of GSE130973, GSE1993304, GSE144236, GSE200334, and GSE218170 from the Gene Expression Omnibus (GEO) database. NMSC, non-melanoma skin cancer; scRNA-seq, single-cell RNA sequencing; UMAP, uniform manifold approximation and projection; CNV, copy number variation.
Basal cell carcinoma (BCC) is the most common type of skin cancer, accounting for approximately 75% of all NMSC cases (29). BCC originates from epidermal cells, frequently occurs on the face, and some types of BCC, such as infiltrative BCC, often shows strong local invasiveness. The primary risk factor for BCC is UVR, with light-skinned individuals being more susceptible (30). Mutations in the Hedgehog (HH) signaling pathway (80%) and TP53 (60%) were predominantly found in BCC (31), and the aberrant activation of the HH signaling pathway has been proven to play a critical role in the majority of cases, making it a promising candidate for targeted therapies (32). However, only a 30–43% response rate was observed with HH signaling pathway inhibitors (Vismodegib or Sonidegib) (32) or PD-1 inhibitors (33), for metastatic BCC or with locally-advanced BCC, indicating a potential for acquired or intrinsic drug resistance (34, 35).
cSCC is the second most common NMSC, frequently occurring on the head and face of the elderly. Substantial evidence implicates that UVR and other non-UVR risk factors include immunosuppression, infections, specific drugs, and exogenous chemical mutagens involved in cSCC initiation (36). Furthermore, actinic keratosis (AK), as a precancerous lesion of cSCC, is closely related to the risk of cSCC. A cohort study involving more than 200,000 cases of AK showed that up to 1.92% of AK progressed to cSCC (37). Another multicenter clinical trial reported that the progression rate of severe AK (Olsen grade 3) to invasive cSCC was as high as 20.9% (38). Although the majority of cSCC can be treated by surgical resection or topically treated with imiquimod or 5-fluorouracil (39), a small proportion will progress into metastases with significant morbidity and mortality (40). Consequently, for multiple or locally advanced, metastatic cSCC, immune checkpoint inhibitors (ICI) can be applied, with an average objective response rate of 42–58% for anti-PD-1 antibodies (Cemiplimab, Pembrolizumab, Nivolumab) (41–44).
Merkel cell carcinoma (MCC) is a rare but highly aggressive neuroendocrine cancer of the skin, that has quadrupled in incidence over the past two decades (45). The development of MCC is linked to two primary factors: the integration of Merkel cell polyomavirus (MCPyV) into the host genome, which leads to the persistent expression of viral early genes, including small and large T antigens, or the mutagenic effects of UVR (46). Surgery remains the most common method by which primary MCC tumors are removed. For advanced MCC, Immunotherapy has now become the standard treatment, leading to a 31.8–68% objective response for different ICI agents (47, 48). In addition to BCC, cSCC, and MCC, there are several other less common types of NMSC, such as EMPD, sebaceous carcinoma, apocrine adenocarcinoma, and various other skin adnexal carcinomas (2).
BCC is the most common NMSC and numerous studies have demonstrated the existence of intra-tumoral heterogeneity (ITH) within BCC, encompassing tumor cells, immune cells, and stromal cells of BCC (49–52). For example, Huang et al. extensively depicted the dynamic cell heterogeneity among tumor epithelial cells, immune cells, myeloid cells, endothelial cells, and stromal cells of infiltrative BCC and revealed the inflammatory characteristics and CD4+ Treg-derived impaired immunity of BCC by scRNA-seq (49). Ganier and colleagues also conducted a comprehensive comparison of the cell composition differences between BCC healthy skin from different anatomical sites and found that there were significant expansions of RGS5+ pericytes and POSTN+ cancer-associated fibroblasts (CAFs) in BCC (52), the latter was in accord with the result of Chen et al. (49). Another prominent finding was that their data suggest that malignant cells of BCC could originate from cells of the inner and outer hair follicle bulb, given that they analyzed keratinocytes in the interfollicular epidermis and in follicular structures using scRNA-seq (52). However, due to differences in classification criteria or the selection of canonical marker genes, the heterogeneity of some cells cannot be compared across studies, such as fibroblasts (49, 51). In such instances, the sharing and integration of scRNA-seq data become of even greater importance.
The complicated cell-to-cell communication within the TME plays a pivotal role in regulating tumor progression. Through integrating single-cell and spatial transcriptomics, Yerly et al. uncovered the epithelial collective migration characteristics of tumor cells and extracellular matrix (ECM) remodeling features of CAFs from the invasive niche of invasive BCC, and Activin A likely promotes tumor cell collective migration by activating fibroblasts to remodel the ECM (50). Furthermore, this large degree of ECM remodeling in BCC is likely driven by the expression of collagen- and metalloproteinase-coding genes (51). Additionally, they also found tumor cells responded to the sudden burst of WNT5A-drived CAFs-specific inflammatory signaling pathways by producing heat shock proteins (51). In addition to the crosstalk between tumor cells and CAFs, a strong crosstalk between ANGPT2+ lymphatic endothelial cells and leukocytes was observed, demonstrating a strong ability to promote leukocyte migration and activation of this lymphatic endothelial cell (49).
Advanced BCCs exhibit a high mutational burden and ITH, and typically occur drug resistance or relapse after Smoothened (SMO) inhibitors or ICI treatments (31, 53). Yao and colleagues employed scRNA-seq to determine the molecular characteristics of SMO inhibitor-resistant tumor cells, which showed high expression of LY6D, LYPD3, and TACSTD2 (54). Mechanically, AP-1 and TGF-ß cooperativity drive the nuclear myocardin-related transcription factor (nMRTF) resistance pathway, which amplifies non-canonical GLI1 activity in the LY6D+ resistant tumor cells (54). Indeed, the plasticity of tumor cells represents a significant contributing factor to drug resistance. BCC transdifferentiation into squamous cell carcinoma is a distinct and important form of resistance for BCC, discarding the dependency on the HH pathway (55). Interestingly, the LY6D+ SMO inhibitor-resistant tumor cells, extensively cover the morphological and transcriptional cell states of basal to squamous cell carcinoma transition (56). With the transition processing, the expressions of LYPD3, TACSTD2, LY6D, CD44, and MUC1 were increased, while the expression of GLI1 was decreased, indicating the occurrence of drug resistance (56, 57). As a supplement, the same research team also demonstrated the mechanism regarding LY6D– tumor epithelial proliferation. A TREM2+ VCAM1+ macrophage population was identified and spatially and functionally defined by integrating the scRNA-seq, the co-detection by indexing (CODEX) multiplex system, and the cytometry by time of flight (CyTOF) approach, which was found to be adjacent to the highly proliferative LY6D– lower tumor epithelium area and promoted LY6D– tumor epithelial proliferation via secretion of the ligand oncostatin-M (OSM) (58). In addition to the resistance to the SMO inhibitor, Pich-Bavastro et al. also found that Activin A secreted by tumor cells would mediate the polarization of CAFs and macrophages, thereby facilitating the resistance of tumor cells to ICI by scRNA-seq (59).
Collectively, most studies focused on the infiltrative BCC and identified high heterogeneity within BCC, including tumor cells and immune cells, indicating the complexity of BCC (Table 1). For this reason, with the increase in the number of cells, a large number of cell subpopulations have been identified, but their exact functions in promoting or inhibiting cancer require further investigation. The investigation of drug resistance mechanisms in BCC is a highly active area of research, with notable progress having been made in understanding the role of LY6D+/– tumor cells in BCC resistance (Table 1). However, the comparability of cell subpopulations across different studies needs to be further clarified, either by using identical marker genes, or the same classification criteria or by integrating and reanalyzing different datasets. Moreover, the multi-omics approach is gradually demonstrating its unique potential in deciphering the heterogeneity and drug resistance of BCC (52).
cSCC is the second most common NMSC after BCC. As the first scRNA-seq study in cSCC, Ji et al. extensively dissected the ITH of cSCC using 10 tumors and matched adjacent tissues, and identified a cluster of tumor-specific keratinocytes (TSKs) (60). The TSKs exhibited high expression of MMP10, PTHLH, LAMC2, and SLITRK, localizing to a fibrovascular niche at the leading edge of the tumor as their adjacent cells were enriched for CAFs and endothelial cells. Furthermore, they also exhibited an abundant epithelial-mesenchymal transition (EMT) signature which serves as a hub for intercellular communication with stromal and immune cells, contributing to cSCC progression, immunosuppression, and ITH. Compared with the 10× Genomics Chromium platform, the Smart-seq2 strategy shows the capacity to detect a greater number of genes within each cell. Based on this, Yan et al. obtained 350 single cells from six primary UV-induced cSCC and determined the gene expression and chromosomal copy number variation pattern in cSCC (61).
In addition to focusing on cSCC itself, the recurrence of cSCC also warrants attention. The treatment of recurrent cSCC is usually more challenging, therefore exploring the recurrence mechanisms of cSCC can help prevent its recurrence and facilitate its treatment. Recurrent cSCC trended to exhibit worse immunosuppressed microenvironment featured by T cell exclusion, CD8+ T cell exhaustion, and enrichment of pro-tumor SPP1+ tumor-associated macrophages (TAMs) (62). Interestingly, a cluster of TSKs identified by Ji et al. in the primary cSCC (60), was also studied in the recurrent cSCC. Although the total number of cell communication events was reduced in the recurrent cSCC (possibly due to the smaller cell number in the recurrent cSCC), TSKs still demonstrated a robust communication with inflammatory CAFs (iCAFs) and IL7R+ CAFs which may be associated with cSCC recurrence (62). Actually, IL7R+ CAFs may be a subtype of iCAFs, as they expressed high levels of IL6, CXCL1, CXCL8, and other cytokines (62). Those studies highlight the ITH of cSCC and also emphasize the pivotal role of TSKs in cSCC progression and recurrence, through extensive communication with different kinds of CAFs.
cSCC can progress from normal skin under persistent UVR, or from AK, a precancerous lesion, or the in situ carcinoma, Bowen’s disease (BD). In recent years, there has been a growing body of research investigating this progression spectrum of cSCC, attempting to prevent and intercept at the early stages of cSCC. Generally, there was no significant difference in the proportion of different kinds of keratinocytes (63), or other immune and stromal cells between AK and normal skin (64), indicating a small change during the progression from normal skin to AK. Even so, some AK-specific keratinocytes or genes were still identified including ALDH3A1, IGFBP2 from Zou et al. (63), and IL1R2, WFDC2 from Li et al. (64). Similarly, frequent cell communications between AK-specific keratinocytes and papillary fibroblasts were observed in AK, especially through the ANGPTL4–ITGA5 interaction, indicating the central role of the ANGPTL pathway in AK development (64). Additionally, the number of proliferative basal cells was found to be significantly increased in BD, compared with AK (63). During the progression of cSCC, malignant cells activate fibroblasts into tumor-promoting CAFs. Thus, Schütz et al. demonstrated the heterogeneity of CAFs between BD and cSCC (65) and found that iCAFs and myofibroblastic CAFs (myCAFs) were dominantly present in BD and cSCC, respectively, in contrast to their absence in AK. Further analyses showed that iCAFs may derive from pro-inflammatory FBs playing immunoregulatory function, while myCAFs may originate from mesenchymal FBs which are associated with ECM organization (65). However, although most studies emphasize the importance of different types of CAFs during cSCC progression and recurrence, It remains an unsolved mystery regarding fibroblasts transform into CAFs at which stage during the progression of cSCC, and through what mechanisms.
Immunosuppression is a key risk for cSCC. Frazzette et al. found that Immunocompromised organ transplant recipients exhibited reduced proportions of cytotoxic and naïve tumor-infiltrating lymphocytes through scRNA-seq, which may be the cause of increased cSCC risk (66). Immunotherapy has been widely used in the treatment of high-risk cSCC. Adoptive cytotoxic T cell transfer-based immunotherapy (ACT) has a good initial therapeutic effect on cSCC, but there is a high probability of recurrence. Using cSCC mouse models, Miao et al. found that tumor-initiating stem cells selectively express CD80, which inhibits T cell activity upon engagement with cytotoxic T lymphocyte antigen 4 (CTLA4), suggesting that blockade of these cells may repress cSCC recurrence (67). Additionally, using scRNA-seq and scTCR-seq, Yost et al. found that cSCC and BCC that respond to anti-PD-1 therapy are derived from their own characteristics, continuously recruiting new T cells, rather than reinvigorating pre-existing tumor-infiltrating T cells through scRNA-seq (68).
Taken together, similar to BCC, the heterogeneity was uncovered first in cSCC and highlighted the important role of TSKs in cSCC progression and recurrence (Table 2). Additionally, developing novel immunotherapeutic strategies that target the immunosuppressive microenvironment and prevent recurrence will be essential to improve patient outcomes. In recent years, an increasing number of studies have focused on the progression from normal skin to cSCC. The transition from AK to cSCC is marked by the activation of fibroblasts into tumor-promoting CAFs. However, the transformation of fibroblasts into CAFs during cSCC progression remains unclear.
MCC is an aggressive skin cancer typically caused by the MCPyV and UVR. Similar to other NMSC, the prominent ITH was observed in MCC. Das et al. identified two distinct MCC tumor cell states: a “mesenchymal-like” state characterized by an inflammatory phenotype and a “well-differentiated neuroepithelial” state, which is associated with acquired resistance to ICIs (69). Additionally, the study demonstrated that manipulation of the intrinsic tumor cell into a “mesenchymal-like” state could lead to increased sensitivity to PI3K inhibitors, suggesting a potential non-genomic resistance mechanism (69). Furthermore, it seems that the heterogeneity of MCC cells was not due to their viral status, as one study reported the co-clustering of MCC cells from virus-positive and virus-negative tumor samples (70). Specifically, MCC cells can be classified into two subtypes according to the expression of neuroendocrine (NE) related genes, and YAP1 suppresses cell-cycle progression in the MCC cells with high expression of NE genes in part through indirect downregulation of MCPyV large T antigen expression (70). The similarities and differences in gene signature between the “mesenchymal-like” and “high NE” states of MCC cells should be further investigated. Another study also reported the plasticity of MCC cells and they found that the inhibition of MCPyV T antigen expression could revert MCC cells to neuron-like cells revealing the potential conversion of an aggressive cancer phenotype to a differentiated state (71).
The immune cells and fibroblast states have also been dissected by scRNA-seq. Gherardin et al. identified a cluster of γδ T cells in MCC, exhibiting proinflammatory phenotype, which may be important effector cells and may serve as prognostic biomarkers and potential therapeutic targets for MCC (72). Previous studies have characterized tumor-specific T cell responses to MCPyV-positive MCC, which have typically been CD8+ T cells (73), but little is known about the T cell response to MCPyV-negative MCC. Therefore, Church et al. identified and characterized tumor-specific Th1-skewed CD4+ T cells targeting multiple neoantigens in a MCPyV-negative MCC patient who achieved a profound and durable partial response to anti-PD-L1 therapy (74). Fan et al. demonstrates that MCC-derived exosome-shuttle miR-375 induces fibroblast polarization by inhibiting RBPJ and TP53 expression, leading to a pro-tumorigenic microenvironment (75).
Therapeutic resistance has been studied by many studies. MHC class I molecules (MHC-I), encoded by the human leukocyte antigen (HLA-A, -B, and -C) genes, are the primary molecules for presenting endogenous tumor antigens to CD8+ T cells. Genetic loss of a single HLA allele has been proven to be associated with ICI resistance in MCC (76), as it results in an inability to effectively present tumor antigens, preventing CD8+ T cells from recognizing and killing tumor cells. Intriguingly, Paulson et al. found that tumor cells evaded recognition by CD8+ T cells by selectively downregulating the expression of specific HLA genes, resulting in acquired adoptive cellular immunotherapy and ICI therapy resistance, through scRNA-seq in two metastatic MCC patients (77), while the histone deacetylase inhibitor domatinostat can reverse this effect by restoring HLA gene expression on MCC cells (78), and cause decreased cell viability and apoptosis through suppressing the transcription factor HES1 (79). Meanwhile, the tumor-associated macrophages (TAMs) were the predominant myeloid subset in MCC, exhibiting an immunosuppressive gene signature, and were also associated with ICI resistance (80). Furthermore, Pulliam et al. found that the frequency of tumor-specific CD8+ T cells in the blood was positively correlated with ICI response and exhibited a senescent phenotype, while tumor-specific CD8+ T cells in the tumor showed an exhausted phenotype (73); and it was pointed out that the resistance to anti-PD-L1 originates from the decrease of HLA-I on tumor cells (73), which was consistent with the result of Paulson et al. (77). This suggests that ICI resistance at treatment re-initiation was not due to a shortage of tumor-specific T cells in the blood, but rather to the downregulation of HLA-I on tumor cells.
Overall, the ITH of MCC including MCPyV-positive and MCPyV-negative has been investigated through scRNA-seq technology (Table 3). At least two states of MCC tumor cells have been identified, and one of them is sensitive to therapeutic treatments. The reversion or conversion of different subtypes indicates the plasticity of MCC cells. Furthermore, the mechanism of ICI resistance should be paid more attention in the future.
EMPD is one rare type of NMSC, mainly localizing to the vulvar, perianal, scrotal, penile, and axillary regions. To date, only one scRNA-seq study has been performed in EMPD. Song et al. revealed significant epithelial and immune cell heterogeneity using tumor and peri-tumoral skin samples from one scrotal EMPD patient through scRNA-seq (81). Furthermore, this study found that MSI1, an RNA-binding protein, is overexpressed in the basal epithelial cells of human EMPD skin, and ectopic overexpression of Msi1 in the epidermal basal layer of mice results in a phenotype resembling human EMPD at histopathological, single-cell, and molecular levels (81), which supports the in situ transformation theory, i.e. keratinocyte-to-Paget-like cell conversion of disease pathogenesis. Mechanically, Msi1 activates the Her2-mTOR signaling pathway by directly suppressing Cmtm5 and Msi1 can also directly repress Pten to activate mTOR signaling. In conclusion, this study provides compelling evidence that the Msi1-mTOR signaling pathway plays a critical role in the pathogenesis of EMPD and that targeting mTOR signaling may offer a novel therapeutic approach for treating this disease. Although only one scRNA-seq study was carried out because of the rarity of EMPD, it is valuable to explore the recurrence mechanism of EMPD. Similarly, multi-omics at the single-cell level should be considered to uncover the pathogenies of EMPD.
A large number of scRNA-seq studies have been applied to NMSC (Figure 2), evolving from the initial revelation of cellular composition to the analysis of specific cellular subpopulations. The characterization of the diversity of functional states in tumor cells and immune cells of NMSC not only expanded our understanding of how tumors evolve and respond to ICI treatments, but also provided potential therapeutic targets to overcome treatment resistance. For example, identifying effectors that regulate BCC transdifferentiation into squamous cell carcinoma or mechanisms underlying tumor cell state transitions may present opportunities to reverse or prevent the emergence of treatment-resistant cell states. Regarding cSCC, The transition from AK to cSCC is marked by the activation of fibroblasts into CAFs, while this transformation during cSCC progression remains unclear. However, the incomplete transcriptomic information, the miss of rare cell types, sophisticated bioinformatics analyses, and the lack of spatial information also should be noted in scRNA-seq. Thus, Further research should consider some issues for scRNA-seq in NMSC. 1) More refined sampling methods of scRNA-seq or integrated spatial transcriptomic sequencing techniques are necessary to explore ITH in different tumor niches; 2) A unified naming system for cell subpopulations should be proposed to facilitate the comparison between similar studies conducted by different research teams, such as CAFs; 3) multi-omics approaches at single-cell level should be applied to comprehensively explore the pathogenesis, recurrence, and drug resistance in NMSC.
Figure 2. Representative advances of NMSC by scRNA-seq. KCs, Keratinocytes; CAFs, Cancer-associated fibroblasts; TAMs, tumor-associated macrophages; ICI, Immune checkpoint inhibitors; TME, Tumor microenvironment; NMSC, Non-melanoma skin cancer; BCC, Basal cell carcinoma; cSCC, Cutaneous squamous cell carcinoma; MCC, Merkel cell carcinoma; EMPD, Extramammary Paget’s disease.
GY: Conceptualization, Funding acquisition, Visualization, Writing – original draft, Writing – review & editing. XW: Conceptualization, Funding acquisition, Project administration, Supervision, Writing – review & editing. GZ: Conceptualization, Funding acquisition, Project administration, Supervision, Visualization, Writing – review & editing.
The author(s) declare that financial support was received for the research, authorship, and/or publication of this article. This work was supported by the National Natural Science Foundation of China (82303576, 82073013, and 82073016).
The authors declare that the research was conducted in the absence of any commercial or financial relationships that could be construed as a potential conflict of interest.
All claims expressed in this article are solely those of the authors and do not necessarily represent those of their affiliated organizations, or those of the publisher, the editors and the reviewers. Any product that may be evaluated in this article, or claim that may be made by its manufacturer, is not guaranteed or endorsed by the publisher.
1. Katalinic A, Kunze U and Schafer T. Epidemiology of cutaneous melanoma and non-melanoma skin cancer in schleswig-holstein, Germany: Incidence, clinical subtypes, tumour stages and localization (epidemiology of skin cancer). Br J Dermatol. (2003) 149:1200–6. doi: 10.1111/j.1365-2133.2003.05554.x
2. Losquadro WD. Anatomy of the skin and the pathogenesis of nonmelanoma skin cancer. Facial Plast Surg Clin North Am. (2017) 25:283–9. doi: 10.1016/j.fsc.2017.03.001
3. Global Burden of Disease Cancer C, Kocarnik JM, Compton K, Dean FE, Fu W, Gaw BL, et al. Cancer incidence, mortality, years of life lost, years lived with disability, and disability-adjusted life years for 29 cancer groups from 2010 to 2019: A systematic analysis for the global burden of disease study 2019. JAMA Oncol. (2022) 8:420–44. doi: 10.1001/jamaoncol.2021.6987
4. Apalla Z, Lallas A, Sotiriou E, Lazaridou E, Ioannides D. Epidemiological trends in skin cancer. Dermatol Pract Concept. (2017) 7:1–6. doi: 10.5826/dpc.0702a01
5. Access GBDH, Quality C. Measuring performance on the healthcare access and quality index for 195 countries and territories and selected subnational locations: A systematic analysis from the global burden of disease study 2016. Lancet. (2018) 391:2236–71. doi: 10.1016/S0140-6736(18)30994-2
6. Hu W, Fang L, Ni R, Zhang H and Pan G. Changing trends in the disease burden of non-melanoma skin cancer globally from 1990 to 2019 and its predicted level in 25 years. BMC Cancer. (2022) 22:836. doi: 10.1186/s12885-022-09940-3
7. Winge MCG, Kellman LN, Guo K, Tang JY, Swetter SM, Aasi SZ, et al. Advances in cutaneous squamous cell carcinoma. Nat Rev Cancer. (2023) 23:430–49. doi: 10.1038/s41568-023-00583-5
8. Cives M, Mannavola F, Lospalluti L, Sergi MC, Cazzato G, Filoni E, et al. Non-melanoma skin cancers: Biological and clinical features. Int J Mol Sci. (2020) 21:5394. doi: 10.3390/ijms21155394
9. Lazar AD, Dinescu S and Costache M. Deciphering the molecular landscape of cutaneous squamous cell carcinoma for better diagnosis and treatment. J Clin Med. (2020) 9:2228. doi: 10.3390/jcm9072228
10. Dika E, Scarfi F, Ferracin M, Broseghini E, Marcelli E, Bortolani B, et al. Basal cell carcinoma: A comprehensive review. Int J Mol Sci. (2020) 21:5572. doi: 10.3390/ijms21155572
11. Stark R, Grzelak M and Hadfield J. Rna sequencing: The teenage years. Nat Rev Genet. (2019) 20:631–56. doi: 10.1038/s41576-019-0150-2
12. Hanahan D. Hallmarks of cancer: New dimensions. Cancer Discovery. (2022) 12:31–46. doi: 10.1158/2159-8290.CD-21-1059
13. Becht E, Giraldo NA, Lacroix L, Buttard B, Elarouci N, Petitprez F, et al. Estimating the population abundance of tissue-infiltrating immune and stromal cell populations using gene expression. Genome Biol. (2016) 17:218. doi: 10.1186/s13059-016-1070-5
14. Lytle NK, Barber AG and Reya T. Stem cell fate in cancer growth, progression and therapy resistance. Nat Rev Cancer. (2018) 18:669–80. doi: 10.1038/s41568-018-0056-x
15. Tang F, Barbacioru C, Wang Y, Nordman E, Lee C, Xu N, et al. Mrna-seq whole-transcriptome analysis of a single cell. Nat Methods. (2009) 6:377–82. doi: 10.1038/nmeth.1315
16. Gohil SH, Iorgulescu JB, Braun DA, Keskin DB and Livak KJ. Applying high-dimensional single-cell technologies to the analysis of cancer immunotherapy. Nat Rev Clin Oncol. (2021) 18:244–56. doi: 10.1038/s41571-020-00449-x
17. Xu Y, Zhang T, Zhou Q, Hu M, Qi Y, Xue Y, et al. A single-cell transcriptome atlas profiles early organogenesis in human embryos. Nat Cell Biol. (2023) 25:604–15. doi: 10.1038/s41556-023-01108-w
18. Papalexi E, Satija R. Single-cell rna sequencing to explore immune cell heterogeneity. Nat Rev Immunol. (2018) 18:35–45. doi: 10.1038/nri.2017.76
19. Yuste R, Hawrylycz M, Aalling N, Aguilar-Valles A, Arendt D, Armananzas R, et al. A community-based transcriptomics classification and nomenclature of neocortical cell types. Nat Neurosci. (2020) 23:1456–68. doi: 10.1038/s41593-020-0685-8
20. Van de Sande B, Lee JS, Mutasa-Gottgens E, Naughton B, Bacon W, Manning J, et al. Applications of single-cell rna sequencing in drug discovery and development. Nat Rev Drug Discovery. (2023) 22:496–520. doi: 10.1038/s41573-023-00688-4
21. McGinnis CS, Murrow LM and Gartner ZJ. Doubletfinder: Doublet detection in single-cell rna sequencing data using artificial nearest neighbors. Cell Syst. (2019) 8:329–337 e4. doi: 10.1016/j.cels.2019.03.003
22. Ran D, Zhang S, Lytal N and An L. Scdoc: Correcting drop-out events in single-cell rna-seq data. Bioinformatics. (2020) 36:4233–9. doi: 10.1093/bioinformatics/btaa283
23. Wu Y, Zhang K. Tools for the analysis of high-dimensional single-cell rna sequencing data. Nat Rev Nephrol. (2020) 16:408–21. doi: 10.1038/s41581-020-0262-0
24. Xia D, Wang Y, Xiao Y and Li W. Applications of single-cell rna sequencing in atopic dermatitis and psoriasis. Front Immunol. (2022) 13:1038744. doi: 10.3389/fimmu.2022.1038744
25. Zou D, Qi J, Wu W, Xu D, Tu Y, Liu T, et al. Applications of single-cell sequencing in dermatology. Med Sci Monit. (2021) 27:e931862. doi: 10.12659/MSM.931862
26. Lichtenberger BM, Kasper M. Cellular heterogeneity and microenvironmental control of skin cancer. J Intern Med. (2021) 289:614–28. doi: 10.1111/joim.13177
27. Srivastava A, Bencomo T, Das I and Lee CS. Unravelling the landscape of skin cancer through single-cell transcriptomics. Transl Oncol. (2023) 27:101557. doi: 10.1016/j.tranon.2022.101557
28. Lim SY, Rizos H. Single-cell rna sequencing in melanoma: What have we learned so far? EBioMedicine. (2024) 100:104969. doi: 10.1016/j.ebiom.2024.104969
29. Nikanjam M, Cohen PR, Kato S, Sicklick JK and Kurzrock R. Advanced basal cell cancer: Concise review of molecular characteristics and novel targeted and immune therapeutics. Ann Oncol. (2018) 29:2192–9. doi: 10.1093/annonc/mdy412
30. Wu S, Han J, Li WQ, Li T and Qureshi AA. Basal-cell carcinoma incidence and associated risk factors in u.S. Women and men. Am J Epidemiol. (2013) 178:890–7. doi: 10.1093/aje/kwt073
31. Bonilla X, Parmentier L, King B, Bezrukov F, Kaya G, Zoete V, et al. Genomic analysis identifies new drivers and progression pathways in skin basal cell carcinoma. Nat Genet. (2016) 48:398–406. doi: 10.1038/ng.3525
32. Sekulic A, Von Hoff D. Hedgehog pathway inhibition. Cell. (2016) 164:831. doi: 10.1016/j.cell.2016.02.021
33. In GK, Nallagangula A, Choi JS, Tachiki L, Blackburn MJ, Capone S, et al. Clinical activity of pd-1 inhibition in the treatment of locally advanced or metastatic basal cell carcinoma. J Immunother Cancer. (2022) 10:e004839. doi: 10.1136/jitc-2022-004839
34. Sharpe HJ, Pau G, Dijkgraaf GJ, Basset-Seguin N, Modrusan Z, Januario T, et al. Genomic analysis of smoothened inhibitor resistance in basal cell carcinoma. Cancer Cell. (2015) 27:327–41. doi: 10.1016/j.ccell.2015.02.001
35. Whitson RJ, Lee A, Urman NM, Mirza A, Yao CY, Brown AS, et al. Noncanonical hedgehog pathway activation through srf-mkl1 promotes drug resistance in basal cell carcinomas. Nat Med. (2018) 24:271–81. doi: 10.1038/nm.4476
36. Que SKT, Zwald FO and Schmults CD. Cutaneous squamous cell carcinoma: Incidence, risk factors, diagnosis, and staging. J Am Acad Dermatol. (2018) 78:237–47. doi: 10.1016/j.jaad.2017.08.059
37. Madani S, Marwaha S, Dusendang JR, Alexeeff S, Pham N, Chen EH, et al. Ten-year follow-up of persons with sun-damaged skin associated with subsequent development of cutaneous squamous cell carcinoma. JAMA Dermatol. (2021) 157:559–65. doi: 10.1001/jamadermatol.2021.0372
38. Ahmady S, Jansen MHE, Nelemans PJ, Kessels J, Arits A, de Rooij MJM, et al. Risk of invasive cutaneous squamous cell carcinoma after different treatments for actinic keratosis: A secondary analysis of a randomized clinical trial. JAMA Dermatol. (2022) 158:634–40. doi: 10.1001/jamadermatol.2022.1034
39. Work G, Invited R, Kim JYS, Kozlow JH, Mittal B, Moyer J, et al. Guidelines of care for the management of cutaneous squamous cell carcinoma. J Am Acad Dermatol. (2018) 78:560–78. doi: 10.1016/j.jaad.2017.10.007
40. Karia PS, Han J, Schmults CD. Cutaneous squamous cell carcinoma: Estimated incidence of disease, nodal metastasis, and deaths from disease in the United States, 2012. J Am Acad Dermatol. (2013) 68:957–66. doi: 10.1016/j.jaad.2012.11.037
41. Gross ND, Miller DM, Khushalani NI, Divi V, Ruiz ES, Lipson EJ, et al. Neoadjuvant cemiplimab for stage ii to iv cutaneous squamous-cell carcinoma. N Engl J Med. (2022) 387:1557–68. doi: 10.1056/NEJMoa2209813
42. Gross ND, Miller DM, Khushalani NI, Divi V, Ruiz ES, Lipson EJ, et al. Neoadjuvant cemiplimab and surgery for stage ii-iv cutaneous squamous-cell carcinoma: Follow-up and survival outcomes of a single-arm, multicentre, phase 2 study. Lancet Oncol. (2023) 24:1196–205. doi: 10.1016/S1470-2045(23)00459-X
43. Munhoz RR, Nader-Marta G, de Camargo VP, Queiroz MM, Cury-Martins J, Ricci H, et al. A phase 2 study of first-line nivolumab in patients with locally advanced or metastatic cutaneous squamous-cell carcinoma. Cancer. (2022) 128:4223–31. doi: 10.1002/cncr.34463
44. Maubec E, Boubaya M, Petrow P, Beylot-Barry M, Basset-Seguin N, Deschamps L, et al. Phase ii study of pembrolizumab as first-line, single-drug therapy for patients with unresectable cutaneous squamous cell carcinomas. J Clin Oncol. (2020) 38:3051–61. doi: 10.1200/JCO.19.03357
45. Becker JC, Stang A, DeCaprio JA, Cerroni L, Lebbe C, Veness M, et al. Merkel cell carcinoma. Nat Rev Dis Primers. (2017) 3:17077. doi: 10.1038/nrdp.2017.77
46. Hernandez LE, Mohsin N, Yaghi M, Frech FS, Dreyfuss I and Nouri K. Merkel cell carcinoma: An updated review of pathogenesis, diagnosis, and treatment options. Dermatol Ther. (2022) 35:e15292. doi: 10.1111/dth.15292
47. Kaufman HL, Russell J, Hamid O, Bhatia S, Terheyden P, D’Angelo SP, et al. Avelumab in patients with chemotherapy-refractory metastatic merkel cell carcinoma: A multicentre, single-group, open-label, phase 2 trial. Lancet Oncol. (2016) 17:1374–85. doi: 10.1016/S1470-2045(16)30364-3
48. Nghiem P, Bhatia S, Lipson EJ, Sharfman WH, KudChadkar RR, Brohl AS, et al. Three-year survival, correlates and salvage therapies in patients receiving first-line pembrolizumab for advanced merkel cell carcinoma. J Immunother Cancer. (2021) 9:e002478. doi: 10.1136/jitc-2021-002478
49. Huang L, Wang X, Pei S, Li X, Dong L, Bian X, et al. Single-cell profiling reveals sustained immune infiltration, surveillance, and tumor heterogeneity in infiltrative basal cell carcinoma. J Invest Dermatol. (2023) 143:2283–2294 e17. doi: 10.1016/j.jid.2023.04.020
50. Yerly L, Pich-Bavastro C, Di Domizio J, Wyss T, Tissot-Renaud S, Cangkrama M, et al. Integrated multi-omics reveals cellular and molecular interactions governing the invasive niche of basal cell carcinoma. Nat Commun. (2022) 13:4897. doi: 10.1038/s41467-022-32670-w
51. Guerrero-Juarez CF, Lee GH, Liu Y, Wang S, Karikomi M, Sha Y, et al. Single-cell analysis of human basal cell carcinoma reveals novel regulators of tumor growth and the tumor microenvironment. Sci Adv. (2022) 8:eabm7981. doi: 10.1126/sciadv.abm7981
52. Ganier C, Mazin P, Herrera-Oropeza G, Du-Harpur X, Blakeley M, Gabriel J, et al. Multiscale spatial mapping of cell populations across anatomical sites in healthy human skin and basal cell carcinoma. Proc Natl Acad Sci U.S.A. (2024) 121:e231332612: doi: 10.1073/pnas.2313326120
53. Sekulic A, Migden MR, Oro AE, Dirix L, Lewis KD, Hainsworth JD, et al. Efficacy and safety of vismodegib in advanced basal-cell carcinoma. N Engl J Med. (2012) 366:2171–9. doi: 10.1056/NEJMoa1113713
54. Yao CD, Haensel D, Gaddam S, Patel T, Atwood SX, Sarin KY, et al. Ap-1 and TGFß cooperativity drives non-canonical hedgehog signaling in resistant basal cell carcinoma. Nat Commun. (2020) 11:5079. doi: 10.1038/s41467-020-18762-5
55. Ransohoff KJ, Tang JY and Sarin KY. Squamous change in basal-cell carcinoma with drug resistance. N Engl J Med. (2015) 373:1079–82. doi: 10.1056/NEJMc1504261
56. Kuonen F, Li NY, Haensel D, Patel T, Gaddam S, Yerly L, et al. C-fos drives reversible basal to squamous cell carcinoma transition. Cell Rep. (2021) 37:109774. doi: 10.1016/j.celrep.2021.109774
57. Haensel D, Gaddam S, Li NY, Gonzalez F, Patel T, Cloutier JM, et al. Ly6d marks pre-existing resistant basosquamous tumor subpopulations. Nat Commun. (2022) 13:7520. doi: 10.1038/s41467-022-35020-y
58. Haensel D, Daniel B, Gaddam S, Pan C, Fabo T, Bjelajac J, et al. Skin basal cell carcinomas assemble a pro-tumorigenic spatially organized and self-propagating trem2+ myeloid niche. Nat Commun. (2023) 14:2685. doi: 10.1038/s41467-023-37993-w
59. Pich-Bavastro C, Yerly L, Di Domizio J, Tissot-Renaud S, Gilliet M and Kuonen F. Activin a-mediated polarization of cancer-associated fibroblasts and macrophages confers resistance to checkpoint immunotherapy in skin cancer. Clin Cancer Res. (2023) 29:3498–513. doi: 10.1158/1078-0432.CCR-23-0219
60. Ji AL, Rubin AJ, Thrane K, Jiang S, Reynolds DL, Meyers RM, et al. Multimodal analysis of composition and spatial architecture in human squamous cell carcinoma. Cell. (2020) 182:497–514 e22. doi: 10.1016/j.cell.2020.05.039
61. Yan G, Li L, Zhu S, Wu Y, Liu Y, Zhu L, et al. Single-cell transcriptomic analysis reveals the critical molecular pattern of uv-induced cutaneous squamous cell carcinoma. Cell Death Dis. (2021) 13:23. doi: 10.1038/s41419-021-04477-y
62. Li X, Zhao S, Bian X, Zhang L, Lu L, Pei S, et al. Signatures of emt, immunosuppression, and inflammation in primary and recurrent human cutaneous squamous cell carcinoma at single-cell resolution. Theranostics. (2022) 12:7532–49. doi: 10.7150/thno.77528
63. Zou DD, Sun YZ, Li XJ, Wu WJ, Xu D, He YT, et al. Single-cell sequencing highlights heterogeneity and Malignant progression in actinic keratosis and cutaneous squamous cell carcinoma. Elife. (2023) 12:e85270. doi: 10.7554/eLife.85270
64. Li J, Xia Y, Kong S, Yang K, Chen H, Zhang Y, et al. Single-cell rna-seq reveals actinic keratosis-specific keratinocyte subgroups and their crosstalk with secretory-papillary fibroblasts. J Eur Acad Dermatol Venereol. (2023) 37:2273–83. doi: 10.1111/jdv.19289
65. Schutz S, Sole-Boldo L, Lucena-Porcel C, Hoffmann J, Brobeil A, Lonsdorf AS, et al. Functionally distinct cancer-associated fibroblast subpopulations establish a tumor promoting environment in squamous cell carcinoma. Nat Commun. (2023) 14:5413. doi: 10.1038/s41467-023-41141-9
66. Frazzette N, Khodadadi-Jamayran A, Doudican N, Santana A, Felsen D, Pavlick AC, et al. Decreased cytotoxic t cells and tcr clonality in organ transplant recipients with squamous cell carcinoma. NPJ Precis Oncol. (2020) 4:13. doi: 10.1038/s41698-020-0119-9
67. Miao Y, Yang H, Levorse J, Yuan S, Polak L, Sribour M, et al. Adaptive immune resistance emerges from tumor-initiating stem cells. Cell. (2019) 177:1172–1186 e14. doi: 10.1016/j.cell.2019.03.025
68. Yost KE, Satpathy AT, Wells DK, Qi Y, Wang C, Kageyama R, et al. Clonal replacement of tumor-specific t cells following pd-1 blockade. Nat Med. (2019) 25:1251–9. doi: 10.1038/s41591-019-0522-3
69. Das BK, Kannan A, Velasco GJ, Kunika MD, Lambrecht N, Nguyen Q, et al. Single-cell dissection of merkel cell carcinoma heterogeneity unveils transcriptomic plasticity and therapeutic vulnerabilities. Cell Rep Med. (2023) 4:101101. doi: 10.1016/j.xcrm.2023.101101
70. Frost TC, Gartin AK, Liu M, Cheng J, Dharaneeswaran H, Keskin DB, et al. Yap1 and wwtr1 expression inversely correlates with neuroendocrine markers in merkel cell carcinoma. J Clin Invest. (2023) 133:e157171. doi: 10.1172/JCI157171
71. Harold A, Amako Y, Hachisuka J, Bai Y, Li MY, Kubat L, et al. Conversion of sox2-dependent merkel cell carcinoma to a differentiated neuron-like phenotype by t antigen inhibition. Proc Natl Acad Sci U.S.A. (2019) 116:20104–14. doi: 10.1073/pnas.1907154116
72. Gherardin NA, Waldeck K, Caneborg A, Martelotto LG, Balachander S, Zethoven M, et al. Gammadelta t cells in merkel cell carcinomas have a proinflammatory profile prognostic of patient survival. Cancer Immunol Res. (2021) 9:612–23. doi: 10.1158/2326-6066.CIR-20-0817
73. Pulliam T, Jani S, Jing L, Ryu H, Jojic A, Shasha C, et al. Circulating cancer-specific cd8 t cell frequency is associated with response to pd-1 blockade in merkel cell carcinoma. Cell Rep Med. (2024) 5:101412. doi: 10.1016/j.xcrm.2024.101412
74. Church C, Pulliam T, Longino N, Park SY, Smythe KS, Makarov V, et al. Transcriptional and functional analyses of neoantigen-specific cd4 t cells during a profound response to anti-pd-l1 in metastatic merkel cell carcinoma. J Immunother Cancer. (2022) 10:e005328. doi: 10.1136/jitc-2022-005328
75. Fan K, Spassova I, Gravemeyer J, Ritter C, Horny K, Lange A, et al. Merkel cell carcinoma-derived exosome-shuttle mir-375 induces fibroblast polarization by inhibition of rbpj and p53. Oncogene. (2021) 40:980–96. doi: 10.1038/s41388-020-01576-6
76. Chowell D, Morris LGT, Grigg CM, Weber JK, Samstein RM, Makarov V, et al. Patient hla class i genotype influences cancer response to checkpoint blockade immunotherapy. Science. (2018) 359:582–7. doi: 10.1126/science.aao4572
77. Paulson KG, Voillet V, McAfee MS, Hunter DS, Wagener FD, Perdicchio M, et al. Acquired cancer resistance to combination immunotherapy from transcriptional loss of class i hla. Nat Commun. (2018) 9:3868. doi: 10.1038/s41467-018-06300-3
78. Song L, Bretz AC, Gravemeyer J, Spassova I, Muminova S, Gambichler T, et al. The hdac inhibitor domatinostat promotes cell-cycle arrest, induces apoptosis, and increases immunogenicity of merkel cell carcinoma cells. J Invest Dermatol. (2021) 141:903–912 e4. doi: 10.1016/j.jid.2020.08.023
79. Srinivas N, Song L, Lei KC, Gravemeyer J, Furtmann F, Gambichler T, et al. The hdac inhibitor domatinostat induces type i interferon alpha in merkel cell carcinoma by hes1 repression. J Cancer Res Clin Oncol. (2023) 149:8267–77. doi: 10.1007/s00432-023-04733-y
80. Tabachnick-Cherny S, Pulliam T, Rodriguez HJ, Fan X, Hippe DS, Jones DC, et al. Characterization of immunosuppressive myeloid cells in merkel cell carcinoma: Correlation with resistance to pd-1 pathway blockade. Clin Cancer Res. (2024) 30:1189–99. doi: 10.1158/1078-0432.CCR-23-1957
Keywords: non-melanoma skin cancer, single-cell RNA sequencing, scRNA-seq, intratumoral heterogeneity, drug resistance, recurrence
Citation: Yan G, Wang X and Zhang G (2024) Unraveling the landscape of non-melanoma skin cancer through single-cell RNA sequencing technology. Front. Oncol. 14:1500300. doi: 10.3389/fonc.2024.1500300
Received: 23 September 2024; Accepted: 16 October 2024;
Published: 04 November 2024.
Edited by:
Xunwei Wu, Hangzhou Medical College, ChinaReviewed by:
Yi Zhang, Sichuan University, ChinaCopyright © 2024 Yan, Wang and Zhang. This is an open-access article distributed under the terms of the Creative Commons Attribution License (CC BY). The use, distribution or reproduction in other forums is permitted, provided the original author(s) and the copyright owner(s) are credited and that the original publication in this journal is cited, in accordance with accepted academic practice. No use, distribution or reproduction is permitted which does not comply with these terms.
*Correspondence: Xiuli Wang, d2FuZ3hpdWxpXzE0MDAwMjNAdG9uZ2ppLmVkdS5jbg==; Guolong Zhang, Z2x6aGFuZ3RqQHRvbmdqaS5lZHUuY24=
Disclaimer: All claims expressed in this article are solely those of the authors and do not necessarily represent those of their affiliated organizations, or those of the publisher, the editors and the reviewers. Any product that may be evaluated in this article or claim that may be made by its manufacturer is not guaranteed or endorsed by the publisher.
Research integrity at Frontiers
Learn more about the work of our research integrity team to safeguard the quality of each article we publish.