- 1Princess Máxima Center for Pediatric Oncology, Oncode Institute, Utrecht, Netherlands
- 2Division Cell Biology, Metabolism & Cancer, Department Biomolecular Health Sciences, Faculty of Veterinary Medicine, Utrecht University, Utrecht, Netherlands
Metabolic rewiring is a defining characteristic of cancer cells, driving their ability to proliferate. Leveraging these metabolic vulnerabilities for therapeutic purposes has a long and impactful history, with the advent of antimetabolites marking a significant breakthrough in cancer treatment. Despite this, only a few in vitro metabolic discoveries have been successfully translated into effective clinical therapies. This limited translatability is partially due to the use of simplistic in vitro models that do not accurately reflect the tumor microenvironment. This Review examines the effects of current cell culture practices on cancer cell metabolism and highlights recent advancements in establishing more physiologically relevant in vitro culture conditions and technologies, such as organoids. Applying these improvements may bridge the gap between in vitro and in vivo findings, facilitating the development of innovative metabolic therapies for cancer.
1 Introduction
Cancer cells can autonomously rewire their metabolic pathway activity to meet their increased bioenergetic, biosynthetic, and redox needs (1). These reprogramming activities are observed ubiquitously across many cancer types and are therefore considered a hallmark of cancer (2, 3). Established in the 1920s with Otto Warburg’s pioneering work on aerobic glycolysis (4), the study of cancer metabolism represents one of the oldest areas of research in cancer biology (1).
The concept of exploiting metabolic vulnerabilities as a cancer therapy is longstanding (5), with the first antimetabolite therapy dating back to 1948 (6). At that time, Farber and colleagues demonstrated that aminopterin, a folate analogue blocking de novo nucleotide biosynthesis, could halt tumor progression in children with acute lymphoblastic leukemia (ALL) (6). Since then, several other antimetabolites including methotrexate, 6-mercaptopurine and 5-fluorouracil (5-FU) have found their way into the clinic and are now being extensively used in various cancer treatment regimens (7–9).
Despite these advancements, only a limited number of identified metabolic vulnerabilities have been successfully translated into effective (targeted) therapies to date. This is, at least in part, due to the use of reductionist in vitro models in preclinical studies that fail to recapitulate the complex microenvironment that defines the heterogeneous metabolic landscape of human tumors (10, 11). Furthermore, it has become increasingly appreciated that the artificial environment of cell culture systems dictates the metabolic state of cancer cells and that minor adjustments in cancer modeling (i.e., cell culture architecture and microenvironmental interactions), biochemical (i.e., nutrients, cell culture media) and physicochemical (i.e., oxygen levels, pH) factors could easily alter metabolic pathway activity, thereby influencing metabolic readouts (12, 13).
In this Review, we discuss the impact of current cancer models, biochemical and physiochemical conditions in standard cell culture practices on cancer cell metabolism. Furthermore, recent efforts to improve the modeling capacity of in vitro systems to better recapitulate physiologic conditions are discussed, including their strengths and current limitations.
2 Modeling of tumor tissue architecture and microenvironmental interactions enhances the metabolic fidelity of in vitro cancer models
Conventional cancer cell metabolism studies have predominantly been conducted using two-dimensional (2D) cell cultures. However, cells grown as a monolayer do not accurately replicate the three-dimensional (3D) growth dynamics of a tumor (14). Moreover, gaining comprehensive insights into cancer metabolism requires models that facilitate the study of intra- and intercellular communication and tumor-microenvironment (TME) interactions. 3D cell culture platforms, including tumor spheroids and organoids, replicate many of the pathophysiological features of solid tumors, such as cell-cell contacts as well as pH, oxygen and nutrient gradients (15). Such models are increasingly favored to study tumor biological processes in vitro (16), but also exhibit several drawbacks. Currently used 3D cultures lack many components of the TME that shape the unique metabolic landscape of patient tumors, such as infiltrating stromal and immune cells, vasculature and the tumor interstitial fluid (17). Below, we discuss recent efforts in optimizing cell culture dimensionality (e.g., 3D cultures) and complexity (e.g., co-cultures, tissue explants, microfluidics) that have led to in vitro tumor models that better recapitulate the metabolic landscape of tumors.
2.1 Cell culture architecture: 2D versus 3D
3D cell culture models are a promising tool to mitigate the gap between 2D culture systems and cancer tissues to study the metabolic complexity of cancer (18). Various studies tried to assess how the metabolic profile of 3D models compares to that of 2D cultures and are reviewed in Tables 1, 2. Most of these studies report an increase in the glycolytic- (19, 20, 42, 43) and oxidative capacities (20, 21, 42) of 3D cultures. Yet, several studies report the opposite, with diminished glycolysis (21) or oxidative metabolism (19, 43) present in 3D models. Moreover, 3D models showed a higher maintenance of ATP production (20, 42, 44, 45), redox balance (42, 44), and biomass synthesis, including nucleotides (42, 44), amino acids (21, 42, 44), lipids (22, 23, 42–45) and NADPH (42, 44). Nevertheless, reductions in amino acid (43) and especially de novo nucleotide synthesis (24, 43, 45) were also reported for 3D models compared to their 2D counterparts. Because these comparative studies were conducted in models of various cancer types, it is believed that these contradictory results can be partially attributed to the metabolic variation across tumor entities. Although a considerable number of studies have addressed the metabolic differences between 2D and 3D cultures, fewer studies make the comparison between these in vitro systems and the metabolic profiles found in primary tumor tissues. Nevertheless, studies that do investigate this indicate a closer resemblance of 3D models to cancer tissues compared to either the traditional 2D cultures (44) or normal, non-cancerous tissue (45).
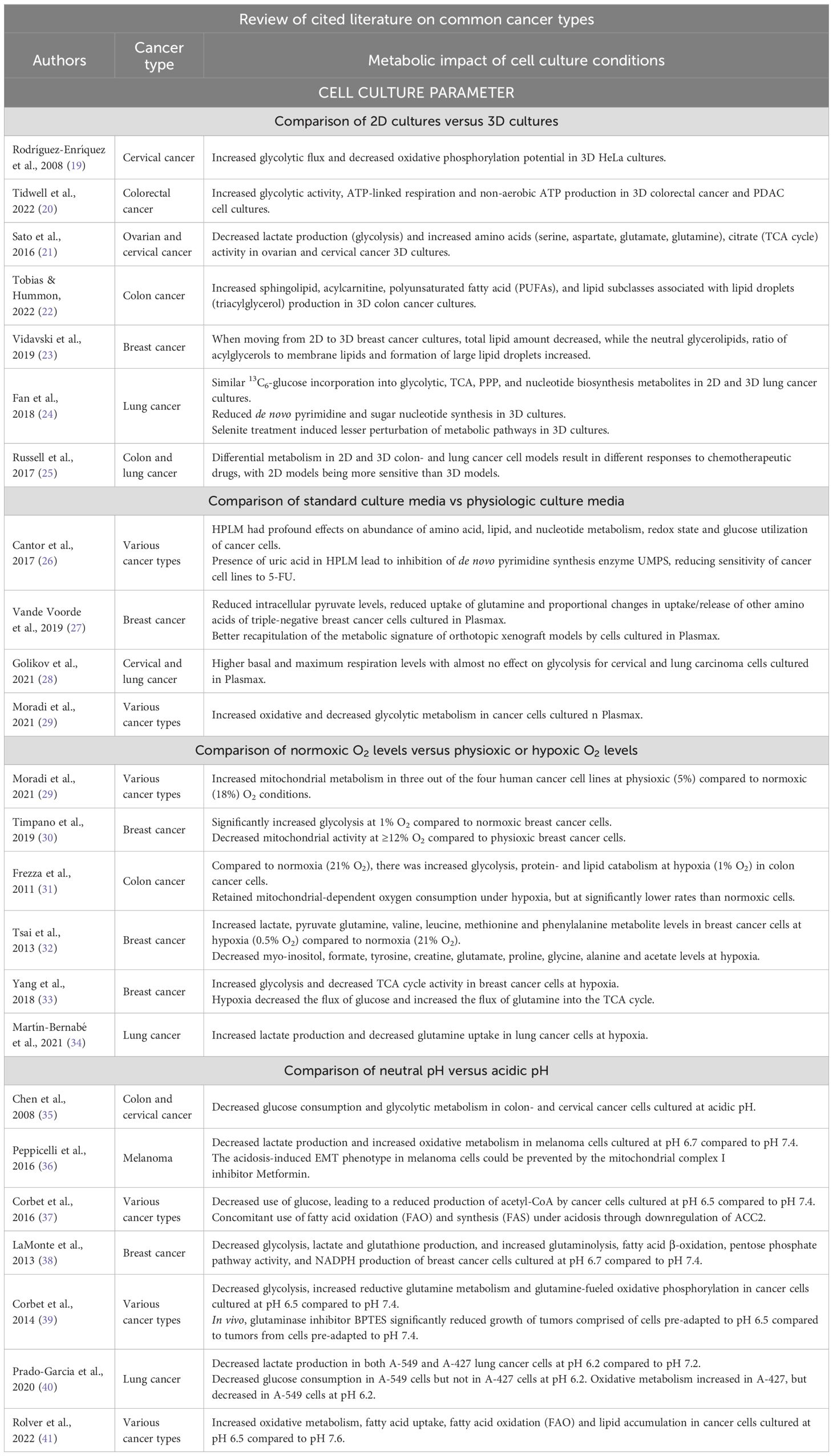
Table 1. Cited literature on more common cancer types that review the impact of cell culture conditions on cancer cell metabolism in vitro.
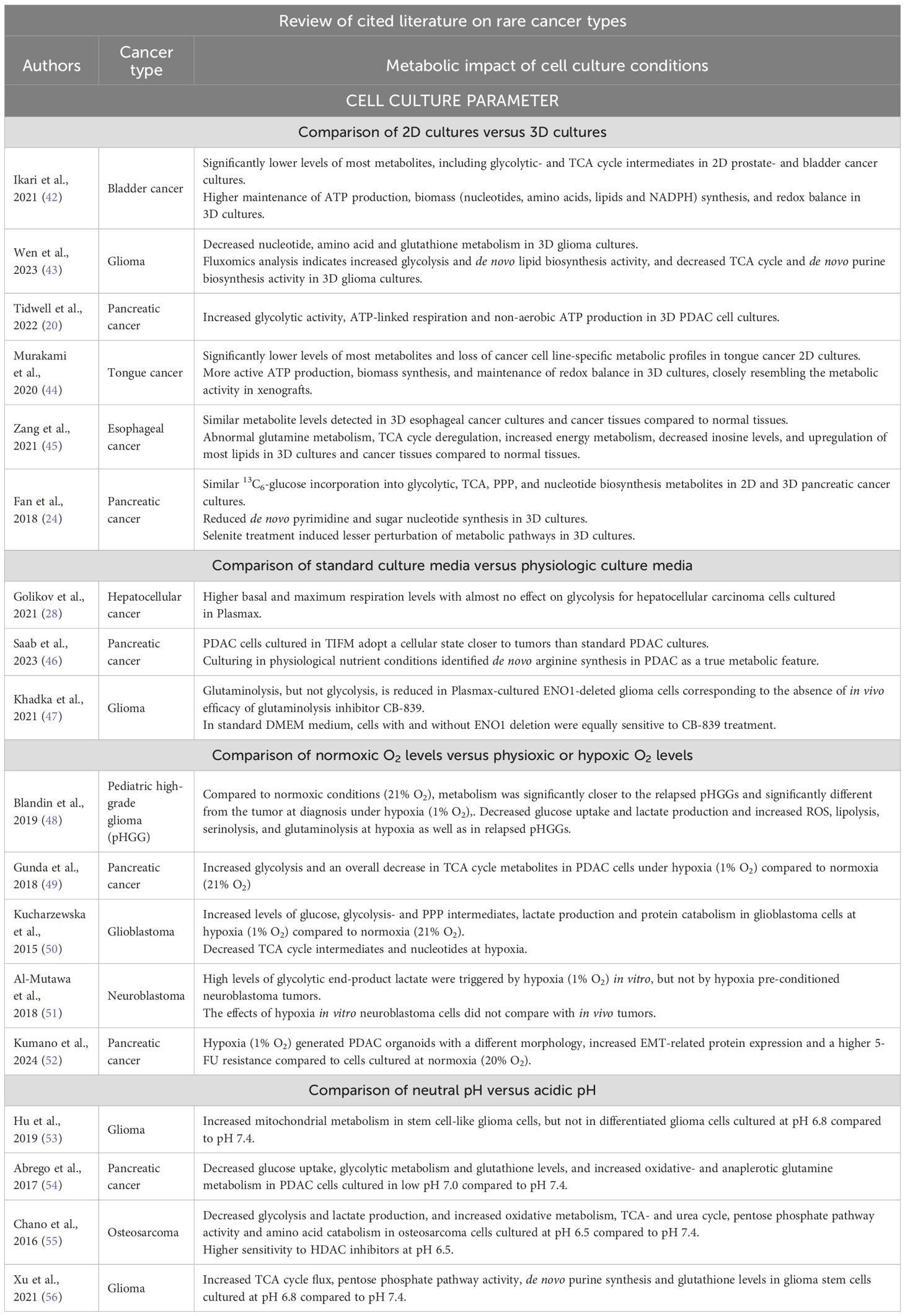
Table 2. Cited literature on more rare cancer types that review the impact of cell culture conditions on cancer cell metabolism in vitro.
Given the metabolic differences identified between 2D and 3D culture conditions, it is not surprising that these models show altered sensitivities to commonly used therapeutic agents. Several findings highlight the importance of considering 3D over or next to 2D models in pre-clinical studies evaluating cancer metabolism and responses to anti-cancer drugs, with numerous studies indicating that 3D models exhibit greater resistance to chemotherapeutics compared to 2D models (24, 25, 57–59), thereby more accurately mimicking drug responses observed in vivo.
A burgeoning number of studies combines 3D organoid models such as patient-derived tumor organoids (PDTO) with metabolomics and stable-isotope tracing approaches to study cancer cell metabolism (60–64). Several reports have demonstrated that this approach could facilitate the assessment of metabolic responses to treatment and aid in the development of novel metabolic treatment strategies. For instance, Neef and colleagues (63) observed dose-dependent alterations in the metabolic profiles of patient-derived colorectal cancer (CRC) organoids subjected to 5-FU treatment. Importantly, the metabolites that exhibited significant changes were primarily associated with purine and pyrimidine metabolism, consistent with the known mechanism of action of 5-FU (63). Furthermore, Ludikhuize et al. (64) investigated the 5-FU response in PDTO models mimicking the different CRC stages. They found that 5-FU induces DNA damage and cell death in p53-deficient CRC organoids due to pyrimidine imbalance, with enhanced toxicity observed in KRASG12D glycolytic CRC organoids when targeting the Warburg effect (64). Together, these studies illustrate the valuable role of 3D organoids in monitoring drug-induced metabolic changes and identifying tumor-specific metabolic sensitivities in vitro.
2.2 Tumor microenvironment interactions
The advent of co-culture systems has made it possible to incorporate multiple cell types to study metabolic cell-cell communication. These in vitro co-culture techniques have provided fundamental insights into the metabolic crosstalk between tumor cells and stromal cells, encompassing adipocytes (65–68), endothelial cells (69, 70) and fibroblasts (71, 72), as well as immune cells such as macrophages (73–75) and T lymphocytes (76–78). However, such systems still lack the cellular diversity as well as the matrix and vascular compartments found within the TME. To address this issue, several next-generation culture platforms have been developed. For example, several groups have set out to establish patient-derived explants (PDEs) to investigate tumor cell metabolism. PDEs are generated by directly culturing fresh, non-dissociated tumor tissue slices in vitro, thereby preserving native tissue architecture, TME, cell-cell interactions and metabolic crosstalk of the in vivo situation (79). In the past, PDEs have been used for metabolic studies (80, 81), but due to their relatively short-term viability and the lack of consistent PDE culturing methodologies their use remains limited (16).
In addition to PDEs, cancer-on-chip (CoC) platforms are emerging as advanced 3D approaches. A CoC is a micro-fluidic-based device that usually hosts multiple cell types in a more in vivo-like microenvironment where mechanical stimuli, flow, and rate of chemical release can be controlled (82, 83). Sensors can also be integrated to perform real-time monitoring of physicochemical cues, such as pH and O2 levels (84). Recently, Dornhof et al. (85) integrated biosensors to measure oxygen, lactate, and glucose into a microfluidic CoC platform, allowing precise and reproducible on-chip multi-analyte metabolite monitoring in real-time. Even more advanced is the work of Kalfe et al. (86), who embedded a microfluidic tube containing tumor spheroids directly into a miniaturized NMR metabolomics detector, allowing them to monitor 23 metabolites. Moreover, Chen et al. (87) developed a CoC integrated with electrospray ionization mass spectrometry, enabling the simultaneous measurement of drug-induced apoptosis and metabolites with high stability, sensitivity and repeatability. Indeed, microfluidic systems have been demonstrated to be able to mimic the in vivo tumor conditions better than traditional 2D systems (88). Especially with the integration of primary, PDTO cultures, a superior reproduction of the in vivo conditions could be obtained. Still, one important limitation of CoC systems is their simplicity, as these devices currently only incorporate the essential components (89).
3 Culture medium composition has a profound impact on cancer cell metabolism
Metabolic pathway activity is dynamically regulated in a context-dependent manner to balance the anabolic and catabolic needs within a cell. Cancer cells, which frequently encounter nutrient-poor, acidic microenvironments with restricted oxygen availability, must undergo metabolic reprogramming to adapt to and thrive under these nutritional fluctuations (90, 91). Modeling cancer cells under variable lactate and nutrient concentrations that mimic the cancer microenvironment may therefore enhance our understanding of cancer metabolism in vivo.
Recognition of the impact of cell culture medium composition on the transcriptomic, epigenetic and metabolic profiles of cells has grown considerably over the past years (13, 92, 93). Still, much of our current knowledge on cancer metabolism predominantly stems from studies using cells cultured in standard, nutrient-rich media. Frequently, these standard media include a largely undefined serum component (e.g., fetal bovine serum (FBS)) in conjunction with one of the several defined basal media (e.g., Minimal Essential Medium (MEM), Dulbecco’s Modified Eagle Medium (DMEM), and RPMI 1640). Such standard media were originally designed to promote the proliferation of specific cell types without the need for constant refeeding, rather than to accurately mimic the in vivo metabolic environment (12).
Media formulations mimicking the nutrient concentrations in plasma or the direct microenvironment can improve the biological relevance of in vitro cancer modeling and aid in addressing the metabolic discrepancies between in vivo and in vitro systems. In 2017 and 2019, two physiological media that more accurately represent the metabolic profile of human plasma were formulated, termed human plasma-like medium (HPLM) (26) and Plasmax (27). Multiple studies that used these media formulations (see Tables 1, 2) have indicated a decreased use of glucose and reduced glycolytic activity of cancer cells cultured in physiologic medium, while the use of oxidative metabolism was shown to be increased (26, 28, 29). Profound effects on amino acid, lipid, and nucleotide metabolism have also been reported (26). Furthermore, several studies have demonstrated that culturing of cancer cells in physiological media results in metabolic profiles that more closely resemble the metabolic state of tumors (27, 46), and could lead to a better assessment of the effectiveness of antitumor drugs in vivo (26, 47). For example, Vande Voorde and colleagues (27) compared the metabolic profiles of CAL-120 breast cancer cells cultured in DMEM-F12 and Plasmax, both as 2D monolayers and 3D spheroids, with CAL-120-derived mammary tumors. They found that 3D spheroids cultured in Plasmax had a metabolic profile closest to that of the tumors, suggesting that 3D culture in a physiological medium better approximates the tumor’s metabolic phenotype (27). In addition, Cantor et al. (26) showed that the physiologic uric acid levels present in HPLM directly inhibit uridine monophosphate synthase (UMPS), thereby reducing the sensitivity of cancer cells to antimetabolite 5-fluorouracil.
Tumor cells are not directly exposed to nutrients in circulating plasma, but rather to nutrients present in the extracellular fluid that perfuses the tissue, so-called tumor interstitial fluid (TIF) (94). By extracting both plasma and TIF from murine lung- and pancreatic adenocarcinoma (PDAC) models, Sullivan et al. (95) revealed that the nutrients available in TIF differ from those present in plasma. Building on these findings, TIF medium (TIFM), containing nutrient levels representative of the PDAC microenvironment, was developed by the same group (46). PDAC cells cultured in TIFM more closely resembled the metabolic state of PDAC tumors compared to standard cell culture models. In addition, these TIFM-cultured PDAC models revealed high de novo arginine synthesis activity to be a specific metabolic feature of PDAC tumors (46). Nevertheless, it remains to be determined whether the nutrient concentrations observed in murine TIF are comparable to those in human tumors.
While these advancements in media formulation enhance the metabolic fidelity of cell culture models, physiological media are susceptible to rapid nutrient depletion (96), indicating the necessity of daily media replacement in such cultures. However, the daily renewal of nutrients and growth factors could lead to cyclic metabolic activation of cells and therefore affect experimental readouts. Instead, continuous perfusion of cells with physiologic media using a fluidic system, for example, could at least partially solve this (10).
4 Physicochemical culture properties influence cancer cell metabolism
Both in vitro and in the human body, the physicochemical environment (i.e., temperature, pH, O2 and CO2 levels) is tightly controlled, but not necessarily the same. Under normal physiological conditions, the pH of blood and tissues is tightly regulated to be at pH 7.4 (97). Similarly, a stable pH range of 7.2-7.4 is often maintained in cell culture media by the addition of buffers, such as sodium bicarbonate (NaHCO3) or HEPES (98). In contrast, most conventional cell culture incubators do not regulate O2 levels, resulting in atmospheric O2 concentrations around 18-21% (99), while much lower oxygen levels are found in human tissues, ranging from 3-7.4% (physioxia) (100).
In the microenvironment of human tumors, pH and oxygen levels are subjected to further change. In solid tumors, the microenvironment is frequently acidified due to elevated levels of acidic metabolites, such as lactate, and the secretion of protons (H+) through specific pumps and transporters (101). The latter processes result in a local extracellular pH ranging from 5.5 to 7.0 (102, 103). Extracellular acidosis is therefore considered a hallmark of most human tumors, strongly linked to malignancy, aggressiveness (36) and/or stemness (53) of tumors. Oxygenation in tumors frequently drops to 0.3-4.2% oxygen (hypoxia) (100). Yet, most in vitro cancer studies have been performed under hyperoxic conditions, with 18–21% O2 now frequently referred to as ‘normoxia’ in literature (104). Thus, adjusting O2 levels and pH to more physiologically relevant conditions, as reviewed below (see also Figure 1 and Tables 1, 2), can influence cancer cell metabolism in vitro to provide a more accurate representation of the in vivo tumor milieu.
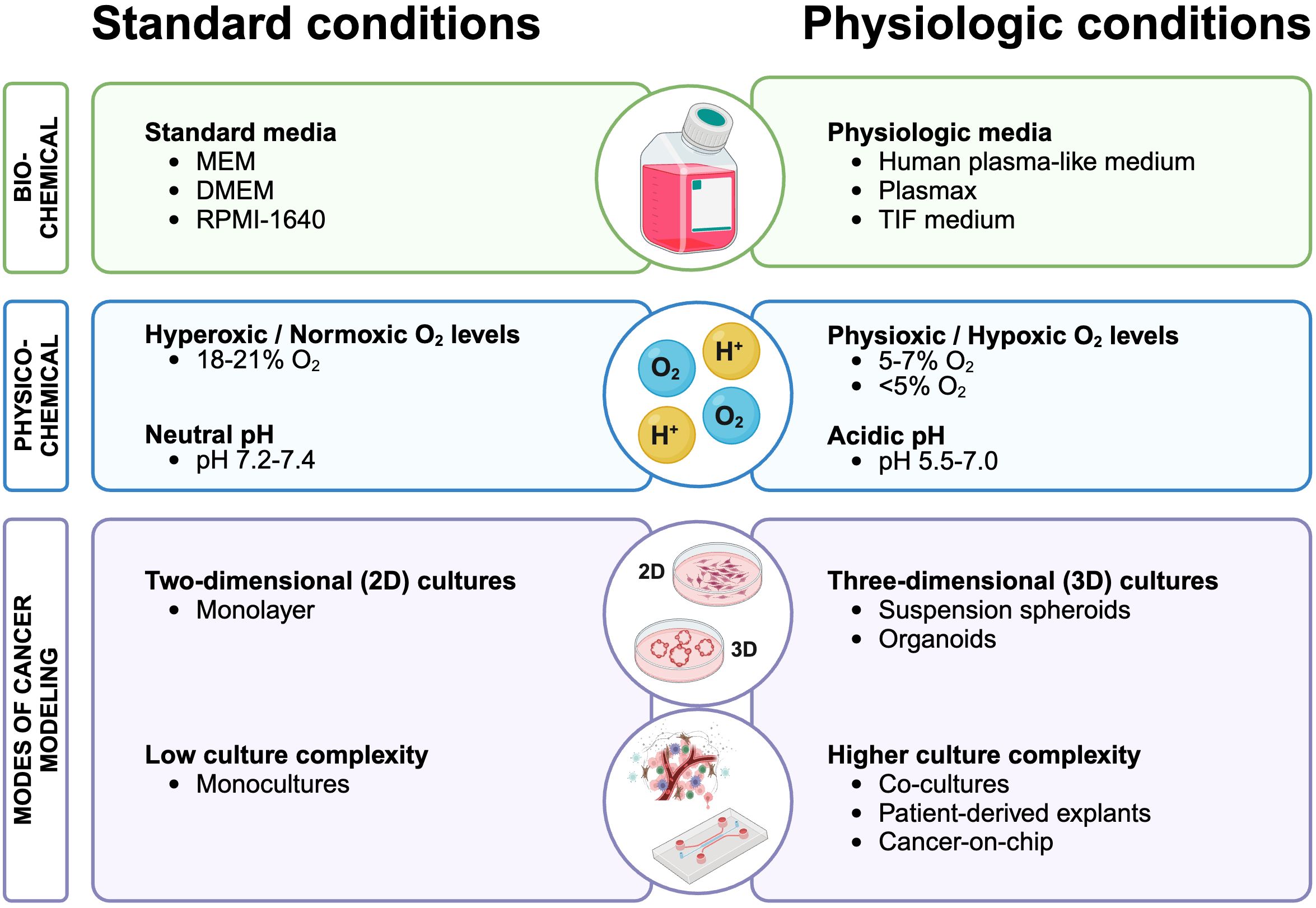
Figure 1. Revising cancer cell culture conditions: comparing standard practices with physiologically relevant conditions for enhanced cancer metabolism modeling. Standard cell culture practices (left) that could be improved to more accurately reflect human physiology (right) to enhance our understanding of metabolic processes in cancer. Created with BioRender.com.
4.1 Oxygen levels: normoxia versus physioxia and hypoxia
The supraphysiological O2 levels used in cell culture systems greatly impact cancer cell metabolism, and could confound the metabolic findings between in vitro and in vivo settings (99). These findings could have important implications when studying various cancer drugs that target energy metabolism. Several studies report higher mitochondrial activity at physioxia than at normoxia (29, 30, 48), suggesting that the standard O2 cell culture conditions suppress the actual oxidative capacity of cancer cells in vitro. Hypoxic conditions, on the other hand, have been shown to induce glycolysis (30–34, 49–51) and decrease the overall levels of TCA cycle metabolites and oxidative metabolism as compared to normoxia (33, 49, 50). Although diminished, mitochondrial-dependent metabolism did remain active at hypoxia (31, 33). For instance, Yang and colleagues (33) observed that exposing MDA-MB-231 breast cancer cells to hypoxia decreased the flux of glucose yet increased glutamine flux into the TCA cycle by enhancing glutaminolysis to compensate for the reduced mitochondrial metabolism under hypoxia. In addition to changes in energy metabolism, several studies report increased protein- (31, 33) and lipid catabolism (31, 48) under hypoxic conditions (1% O2). These increased catabolic reactions likely occur to compensate for the impaired mitochondrial activity that could not be corrected by increasing the glycolytic flux (31).
Subjecting cancer cells to physiologically relevant O2 levels might result in metabolic profiles that more accurately reflect tumor metabolism in vivo (48). For example, Blandin and colleagues demonstrated that the hypoxia-induced metabolic switch in cultured pediatric high-grade glioma (pHGG) cells was similar to the metabolic profile of matched relapsed pHGG tumors, indicating that culturing pHGG cells at 1% O2 more closely reflects patient tumor metabolism than cells cultured at normoxia (48). By subjecting identical PDAC surgical samples to 20% or 1% O2, Kumano et al. (52) found that hypoxia generated PDAC organoids with a different morphology, increased EMT-related protein expression and a higher 5-FU resistance. Their results suggest that hypoxia selects for PDAC cells with malignant traits, aiding in the development of effective anticancer treatments.
4.2 pH: neutral versus acidic
As with oxygen, culturing cancer cells at a tumor-like acidic pH reprograms their metabolism, revealing vulnerabilities that could improve the prediction of therapeutic effectiveness in vivo. Various studies compared the energy metabolism of cancer cells cultured at physiologic pH 7.4 or acidic pH ranging from 6.0 to 7.0. These revealed that acidosis reduced the general glucose uptake in tumor cells (35, 37, 54), and redirected glucose away from glycolysis and lactate production (35–40, 54) towards oxidative metabolism (36, 39, 53–56). Furthermore, several studies report an increased concomitant use of fatty acid (FA) breakdown and synthesis in cancer cells at acidic pH (37, 41). The latter process endows acid-adapted cancer cells with an increased capacity for utilizing FA for metabolic needs, while limiting glycolysis (41). Moreover, cells experiencing acidosis shift their metabolism towards the pentose phosphate pathway (PPP) (55, 56), important for the production of NADPH. NADPH is crucial for antioxidant defense, and acts in part by recycling glutathione (GSH) to counteract reactive oxygen species (ROS) (38, 105). Several papers report diminished GSH synthesis at low pH (38, 54), thereby increasing the demand for PPP-derived NADPH to recycle existing GSH pools (38). These findings indicating that low environmental pH affects both energy and redox metabolism to maintain homeostasis under acidosis-induced oxidative stress.
Acidosis-induced metabolic rewiring of cancer cells results in novel metabolic vulnerabilities that could potentially be exploited. As shown by Peppicelli et al. (36), treating acid-exposed melanoma cells with the mitochondrial complex I inhibitor Metformin inhibited the acidosis-induced oxidative metabolism and reduced the proliferation and epithelial-to-mesenchymal transition (EMT) of invasive melanoma cells. Chano et al. (55) showed a higher sensitivity of osteosarcoma cells to HDAC inhibitors at pH 6.5, suggesting that acidosis promotes metabolic profiles that contribute to epigenetic maintenance. Lastly, Corbet et al. (39) showed that targeting acid-driven glutamine metabolism in vivo with the glutaminase inhibitor BPTES significantly reduced the growth of tumors comprised of cells pre-adapted to pH 6.5, compared to tumors from cells adapted to neutral pH 7.4.
5 Discussion
Most of our current knowledge on cancer cell metabolism stems from reductionist in vitro models grown in a highly artificial environment. In this review, we present how current standard cell culture practices markedly influence cancer cell metabolism and how the use of more physiologically relevant culture conditions could mitigate discrepancies between in vitro and in vivo findings. The main findings of cited studies are compiled in Table 1. Summarized by us in Figure 1 are the current recommendations for standard cell culture practices that could improve the metabolic fidelity of in vitro modeling systems.
However, not all factors described are easily implementable. For example, measuring metabolic activity under low O2 conditions requires the use of expensive hypoxic chambers and incubators that are not routinely used in most laboratories. Furthermore, not all cell culture models can currently be grown or maintained in a 3D setting. Finally, the development of the more complex CoC models requires interdisciplinary knowledge, ranging from biology to microfluidic chip engineering.
Still, tremendous progress has been made in optimizing the culture conditions and models to more accurately study cancer metabolism in vitro. Nevertheless, it is expected that our increasing knowledge on TME physiology as well as continuing technological advances will ultimately result in more representative models to study cancer metabolism. Presumably, these improvements will generate novel and meaningful insights into cancer metabolism that will be more effectively translated into successful anti-cancer therapies.
Author contributions
MK: Conceptualization, Investigation, Validation, Visualization, Writing – original draft, Writing – review & editing. CB: Conceptualization, Funding acquisition, Supervision, Validation, Writing – review & editing. JD: Conceptualization, Funding acquisition, Supervision, Validation, Writing – review & editing.
Funding
The author(s) declare financial support was received for the research, authorship, and/or publication of this article. We are grateful for support from the European Research Council (ERC) starting Grant (#850571) and the Children Cancer-free Foundation (KiKa #377).
Acknowledgments
We would like to thank J. DeMartino and M. Houweling for critical reading of the manuscript. We regret that due to space limitation, we were unable to cite many other studies relevant to the subject of this review.
Conflict of interest
The authors declare that the research was conducted in the absence of any commercial or financial relationships that could be construed as a potential conflict of interest.
Publisher’s note
All claims expressed in this article are solely those of the authors and do not necessarily represent those of their affiliated organizations, or those of the publisher, the editors and the reviewers. Any product that may be evaluated in this article, or claim that may be made by its manufacturer, is not guaranteed or endorsed by the publisher.
References
1. De Berardinis RJ, Chandel NS. Fundamentals of cancer metabolism. Sci Adv. (2016) 2. doi: 10.1126/SCIADV.160020
2. Hanahan D, Weinberg RA. Hallmarks of cancer: the next generation. Cell. (2011) 144:646–74. doi: 10.1016/J.CELL.2011.02.013
3. Pavlova NN, Thompson CB. The emerging hallmarks of cancer metabolism. Cell Metab. (2016) 23:27–47. doi: 10.1016/J.CMET.2015.12.006
4. Warburg O. On the origin of cancer cells. Science. (1956) 123:309–14. doi: 10.1126/SCIENCE.123.3191.309
5. Wolpaw AJ, Dang CV. Exploiting metabolic vulnerabilities of cancer with precision and accuracy. Trends Cell Biol. (2018) 28:201–12. doi: 10.1016/J.TCB.2017.11.006
6. Diamond LK, Mercer RD, Sylvester RF, Wolff JA. Temporary remissions in acute leukemia in children produced by folic acid antagonist, 4-aminopteroyl-glutamic acid. N Engl J Med. (1948) 238:787–93. doi: 10.1056/NEJM194806032382301
7. Danzi F, Pacchiana R, Mafficini A, Scupoli MT, Scarpa A, Donadelli M, et al. To metabolomics and beyond: a technological portfolio to investigate cancer metabolism. Signal Transduction Targeted Ther. (2023) 8:1–22. doi: 10.1038/s41392-023-01380-0
8. Lambie DG, Johnson RH. Drugs and folate metabolism. Drugs. (1985) 30:145–55. doi: 10.2165/00003495-198530020-00003/METRICS
9. Kaye SB. New antimetabolites in cancer chemotherapy and their clinical impact. Br J Cancer. (1998) 78:1. doi: 10.1038/BJC.1998.747
10. Dragic H, Chaveroux C, Cosset E, Manie SN. Modelling cancer metabolism in vitro: current improvements and future challenges. FEBS J. (2024) 291:402–11. doi: 10.1111/FEBS.16704
11. Davidson SM, Papagiannakopoulos T, Olenchock BA, Heyman JE, Keibler MA, Luengo A, et al. Environment impacts the metabolic dependencies of ras-driven non-small cell lung cancer. Cell Metab. (2016) 23:517–28. doi: 10.1016/J.CMET.2016.01.007
12. Cantor JR. The rise of physiologic media. Trends Cell Biol. (2019) 29:854. doi: 10.1016/J.TCB.2019.08.009
13. Golikov MV, Valuev-Elliston VT, Smirnova OA, Ivanov AV. Physiological media in studies of cell metabolism. Mol Biol. (2022) 56:629–37. doi: 10.1134/S0026893322050077
14. Kapałczyńska M, Kolenda T, Przybyła W, Zajączkowska M, Teresiak A, Filas V, et al. 2D and 3D cell cultures – a comparison of different types of cancer cell cultures. Arch Med Sci. (2018) 14:910. doi: 10.5114/AOMS.2016.63743
15. Griffith LG, Swartz MA. Capturing complex 3D tissue physiology in vitro. Nat Rev Mol Cell Biol. (2006) 7:211–24. doi: 10.1038/nrm1858
16. Templeton AR, Jeffery PL, Thomas PB, Perera MPJ, Ng G, Calabrese AR, et al. Patient-derived explants as a precision medicine patient-proximal testing platform informing cancer management. Front Oncol. (2021) 11:767697. doi: 10.3389/FONC.2021.767697
17. Polak R, Zhang ET, Kuo CJ. Cancer organoids 2.0: modelling the complexity of the tumour immune microenvironment. Nat Rev Cancer. (2024) 24:523–39. doi: 10.1038/s41568-024-00706-6
18. Pampaloni F, Reynaud EG, Stelzer EHK. The third dimension bridges the gap between cell culture and live tissue. Nat Rev Mol Cell Biol. (2007) 8:839–45. doi: 10.1038/nrm2236
19. Rodríguez-Enríquez S, Gallardo-Pérez JC, Avilés-Salas A, Marín-Hernández A, Carreño-Fuentes L, Maldonado-Lagunas V, et al. Energy metabolism transition in multi-cellular human tumor spheroids. J Cell Physiol. (2008) 216:189–97. doi: 10.1002/JCP.21392
20. Tidwell TR, Røsland GV, Tronstad KJ, Søreide K, Hagland HR. Metabolic flux analysis of 3D spheroids reveals significant differences in glucose metabolism from matched 2D cultures of colorectal cancer and pancreatic ductal adenocarcinoma cell lines. Cancer Metab. (2022) 10:1–16. doi: 10.1186/S40170-022-00285-W
21. Sato M, Kawana K, Adachi K, Fujimoto A, Yoshida M, Nakamura H, et al. Spheroid cancer stem cells display reprogrammed metabolism and obtain energy by actively running the tricarboxylic acid (TCA) cycle. Oncotarget. (2016) 7:33297. doi: 10.18632/ONCOTARGET.8947
22. Tobias F, Hummon AB. Lipidomic comparison of 2D and 3D colon cancer cell culture models. J Mass Spectrom. (2022) 57(8):e4880. doi: 10.1002/JMS.4880
23. Vidavsky N, Kunitake JAMR, Diaz-Rubio ME, Chiou AE, Loh HC, Zhang S, et al. Mapping and profiling lipid distribution in a 3D model of breast cancer progression. ACS Cent Sci. (2019) 5:768–80. doi: 10.1021/ACSCENTSCI.8B00932
24. Fan TWM, El-Amouri SS, Macedo JKA, Wang QJ, Song H, Cassel T, et al. Stable isotope-resolved metabolomics shows metabolic resistance to anti-cancer selenite in 3D spheroids versus 2D cell cultures. Metabolites. (2018) 8(3):40. doi: 10.3390/METABO8030040
25. Russell S, Wojtkowiak J, Neilson A, Gillies RJ. Metabolic Profiling of healthy and cancerous tissues in 2D and 3D. Sci Rep. (2017) 7:1–11. doi: 10.1038/s41598-017-15325-5
26. Cantor JR, Abu-Remaileh M, Kanarek N, Freinkman E, Gao X, Louissaint A, et al. Physiologic medium rewires cellular metabolism and reveals uric acid as an endogenous inhibitor of UMP synthase. Cell. (2017) 169:258–272.e17. doi: 10.1016/J.CELL.2017.03.023
27. Vande Voorde J, Ackermann T, Pfetzer N, Sumpton D, Mackay G, Kalna G, et al. Improving the metabolic fidelity of cancer models with a physiological cell culture medium. Sci Adv. (2019) 5(1):eaau7314. doi: 10.1126/SCIADV.AAU7314
28. Golikov MV, Karpenko IL, Lipatova AV, Ivanova ON, Fedyakina IT, Larichev VF, et al. Cultivation of cells in a physiological plasmax medium increases mitochondrial respiratory capacity and reduces replication levels of RNA viruses. Antioxidants (Basel). (2021) 11(1):97. doi: 10.3390/ANTIOX11010097
29. Moradi F, Moffatt C, Stuart JA. The effect of oxygen and micronutrient composition of cell growth media on cancer cell bioenergetics and mitochondrial networks. Biomolecules. (2021) 11(8):1177. doi: 10.3390/BIOM11081177
30. Timpano S, Guild BD, Specker EJ, Melanson G, Medeiros PJ, Sproul SLJ, et al. Physioxic human cell culture improves viability, metabolism, and mitochondrial morphology while reducing DNA damage. FASEB J. (2019) 33:5716–28. doi: 10.1096/FJ.201802279R
31. Frezza C, Zheng L, Tennant DA, Papkovsky DB, Hedley BA, Kalna G, et al. Metabolic profiling of hypoxic cells revealed a catabolic signature required for cell survival. PloS One. (2011) 6:e24411. doi: 10.1371/JOURNAL.PONE.0024411
32. Tsai IL, Kuo TC, Ho TJ, Harn YC, Wang SY, Fu WM, et al. Metabolomic dynamic analysis of hypoxia in MDA-MB-231 and the comparison with inferred metabolites from transcriptomics data. Cancers (Basel). (2013) 5:491. doi: 10.3390/CANCERS5020491
33. Yang J, Cheng J, Sun B, Li H, Wu S, Dong F, et al. Untargeted and stable isotope-assisted metabolomic analysis of MDA-MB-231 cells under hypoxia. Metabolomics. (2018) 14:1–14. doi: 10.1007/S11306-018-1338-8
34. Martín-Bernabé A, Tarragó-Celada J, Cunin V, Michelland S, Cortés R, Poignant J, et al. Quantitative proteomic approach reveals altered metabolic pathways in response to the inhibition of lysine deacetylases in A549 cells under normoxia and hypoxia. Int J Mol Sci. (2021) 22(7):3378. doi: 10.3390/IJMS22073378
35. Chen JLY, Lucas JE, Schroeder T, Mori S, Wu J, Nevins J, et al. The genomic analysis of lactic acidosis and acidosis response in human cancers. PloS Genet. (2008) 4(12):e1000293. doi: 10.1371/JOURNAL.PGEN.1000293
36. Peppicelli S, Toti A, Giannoni E, Bianchini F, Margheri F, Del Rosso M, et al. Metformin is also effective on lactic acidosis-exposed melanoma cells switched to oxidative phosphorylation. Cell Cycle. (2016) 15:1908. doi: 10.1080/15384101.2016.1191706
37. Corbet C, Pinto A, Martherus R, Santiago de Jesus JP, Polet F, Feron O. Acidosis drives the reprogramming of fatty acid metabolism in cancer cells through changes in mitochondrial and histone acetylation. Cell Metab. (2016) 24:311–23. doi: 10.1016/J.CMET.2016.07.003
38. LaMonte G, Tang X, Chen JL-Y, Wu J, Ding C-KC, Keenan MM, et al. Acidosis induces reprogramming of cellular metabolism to mitigate oxidative stress. Cancer Metab. (2013) 1:23. doi: 10.1186/2049-3002-1-23
39. Corbet C, Draoui N, Polet F, Pinto A, Drozak X, Riant O, et al. The SIRT1/HIF2α axis drives reductive glutamine metabolism under chronic acidosis and alters tumor response to therapy. Cancer Res. (2014) 74:5507–19. doi: 10.1158/0008-5472.CAN-14-0705
40. Prado-Garcia H, Campa-Higareda A, Romero-Garcia S. Lactic acidosis in the presence of glucose diminishes warburg effect in lung adenocarcinoma cells. Front Oncol. (2020) 10:807/BIBTEX. doi: 10.3389/FONC.2020.00807/BIBTEX
41. Rolver MG, Holland LKK, Ponniah M, Prasad NS, Yao J, Schnipper J, et al. Chronic acidosis rewires cancer cell metabolism through PPARα signaling. Int J Cancer. (2023) 152:1668–84. doi: 10.1002/IJC.34404
42. Ikari R, Mukaisho KI, Kageyama S, Nagasawa M, Kubota S, Nakayama T, et al. Differences in the central energy metabolism of cancer cells between conventional 2D and novel 3D culture systems. Int J Mol Sci. (2021) 22:1–13. doi: 10.3390/IJMS22041805
43. Wen S, Tu X, Zang Q, Zhu Y, Li L, Zhang R, et al. Liquid chromatography–mass spectrometry-based metabolomics and fluxomics reveals the metabolic alterations in glioma U87MG multicellular tumor spheroids versus two-dimensional cell cultures. Rapid Commun Mass Spectrometry. (2024) 38:e9670. doi: 10.1002/RCM.9670
44. Murakami S, Tanaka H, Nakayama T, Taniura N, Miyake T, Tani M, et al. Similarities and differences in metabolites of tongue cancer cells among two- and three-dimensional cultures and xenografts. Cancer Sci. (2021) 112:918–31. doi: 10.1111/CAS.14749
45. Zang Q, Sun C, Chu X, Li L, Gan W, Zhao Z, et al. Spatially resolved metabolomics combined with multicellular tumor spheroids to discover cancer tissue relevant metabolic signatures. Anal Chim Acta. (2021) 1155:338342. doi: 10.1016/J.ACA.2021.338342
46. Saab JJA, Dzierozynski LN, Jonker PB, Aminitabrizi R, Shah H, Menjivar RE, et al. Pancreatic tumors exhibit myeloid-driven amino acid stress and upregulate arginine biosynthesis. Elife. (2023) 12:e81289. doi: 10.7554/ELIFE.81289
47. Khadka S, Arthur K, Barekatain Y, Behr E, Washington M, Ackroyd J, et al. Impaired anaplerosis is a major contributor to glycolysis inhibitor toxicity in glioma. Cancer Metab. (2021) 9(1):27. doi: 10.1186/S40170-021-00259-4
48. Blandin AF, Durand A, Litzler M, Tripp A, Guérin É, Ruhland E, et al. Hypoxic environment and paired hierarchical 3D and 2D models of pediatric H3.3-mutated gliomas recreate the patient tumor complexity. Cancers (Basel). (2019) 11(12):1875. doi: 10.3390/CANCERS11121875
49. Gunda V, Kumar S, Dasgupta A, Singh PK. Hypoxia-induced metabolomic alterations in pancreatic cancer cells. Methods Mol Biol. (2018) 1742:95–105. doi: 10.1007/978-1-4939-7665-2_9
50. Kucharzewska P, Christianson HC, Belting M. Global profiling of metabolic adaptation to hypoxic stress in human glioblastoma cells. PloS One. (2015) 10(1):e0116740. doi: 10.1371/JOURNAL.PONE.0116740
51. Al-Mutawa YK, Herrmann A, Corbishley C, Losty PD, Phelan M, Sée V. Effects of hypoxic preconditioning on neuroblastoma tumour oxygenation and metabolic signature in a chick embryo model. Biosci Rep. (2018) 38:20180185. doi: 10.1042/BSR20180185
52. Kumano K, Nakahashi H, Louphrasitthiphol P, Kuroda Y, Miyazaki Y, Shimomura O, et al. Hypoxia at 3D organoid establishment selects essential subclones within heterogenous pancreatic cancer. Front Cell Dev Biol. (2024) 12:1327772.
53. Degitz C, Reime S, Baumbach CM, Rauschner M, Thews O. Modulation of mitochondrial function by extracellular acidosis in tumor cells and normal fibroblasts: Role of signaling pathways. Neoplasia. (2019) 52:100999. doi: 10.1016/j.neo.2024.100999
54. Abrego J, Gunda V, Vernucci E, Shukla SK, King RJ, Dasgupta A, et al. GOT1-mediated anaplerotic glutamine metabolism regulates chronic acidosis stress in pancreatic cancer cells. Cancer Lett. (2017) 400:37. doi: 10.1016/J.CANLET.2017.04.029
55. Chano T, Avnet S, Kusuzaki K, Bonuccelli G, Sonveaux P, Rotili D, et al. Tumour-specific metabolic adaptation to acidosis is coupled to epigenetic stability in osteosarcoma cells. Am J Cancer Res. (2016) 6:859.
56. Xu X, Wang L, Zang Q, Li S, Li L, Wang Z, et al. Rewiring of purine metabolism in response to acidosis stress in glioma stem cells. Cell Death Dis. (2021) 12(3):277. doi: 10.1038/S41419-021-03543-9
57. Lovitt CJ, Shelper TB, Avery VM. Evaluation of chemotherapeutics in a three-dimensional breast cancer model. J Cancer Res Clin Oncol. (2015) 141:951–9. doi: 10.1007/S00432-015-1950-1
58. Longati P, Jia X, Eimer J, Wagman A, Witt MR, Rehnmark S, et al. 3D pancreatic carcinoma spheroids induce a matrix-rich, chemoresistant phenotype offering a better model for drug testing. BMC Cancer. (2013) 13:1–13. doi: 10.1186/1471-2407-13-95
59. Breslin S, O’Driscoll L. The relevance of using 3D cell cultures, in addition to 2D monolayer cultures, when evaluating breast cancer drug sensitivity and resistance. Oncotarget. (2016) 7:45745. doi: 10.18632/ONCOTARGET.9935
60. Maddocks ODK, Athineos D, Cheung EC, Lee P, Zhang T, Van Den Broek NJF, et al. Modulating the therapeutic response of tumours to dietary serine and glycine starvation. Nature. (2017) 544:372–6. doi: 10.1038/nature22056
61. Yoshizaki H, Ogiso H, Okazaki T, Kiyokawa E. Comparative lipid analysis in the normal and cancerous organoids of MDCK cells. J Biochem. (2016) 159:573–84. doi: 10.1093/JB/MVW001
62. Lindeboom RG, van Voorthuijsen L, Oost KC, Rodríguez-Colman MJ, Luna-Velez MV, Furlan C, et al. Integrative multi-omics analysis of intestinal organoid differentiation. Mol Syst Biol. (2018) 14:8227. doi: 10.15252/MSB.20188227
63. Neef SK, Janssen N, Winter S, Wallisch SK, Hofmann U, Dahlke MH, et al. Metabolic drug response phenotyping in colorectal cancer organoids by LC-QTOF-MS. Metabolites. (2020) 10:494. doi: 10.3390/METABO10120494
64. Ludikhuize MC, Gevers S, Nguyen NTB, Meerlo M, Roudbari SKS, Gulersonmez MC, et al. Rewiring glucose metabolism improves 5-FU efficacy in p53-deficient/KRASG12D glycolytic colorectal tumors. Commun Biol. (2022) 5(1):1159. doi: 10.1038/S42003-022-04055-8
65. Rebeaud M, Bouche C, Dauvillier S, Attané C, Arellano C, Vaysse C, et al. A novel 3D culture model for human primary mammary adipocytes to study their metabolic crosstalk with breast cancer in lean and obese conditions. Sci Rep. (2023) 13:1–12. doi: 10.1038/s41598-023-31673-x
66. Wang YY, Attané C, Milhas D, Dirat B, Dauvillier S, Guerard A, et al. Mammary adipocytes stimulate breast cancer invasion through metabolic remodeling of tumor cells. JCI Insight. (2017) 2. doi: 10.1172/JCI.INSIGHT.87489
67. Olszańska J, Pietraszek-Gremplewicz K, Domagalski M, Nowak D. Mutual impact of adipocytes and colorectal cancer cells growing in co-culture conditions. Cell Communication Signaling. (2023) 21:1–18. doi: 10.1186/S12964-023-01155-8
68. Asante EC, Pallegar NK, Viloria-Petit AM, Christian SL. Three-dimensional co-culture method for studying interactions between adipocytes, extracellular matrix, and cancer cells. Methods Mol Biol. (2022) 2508:69–77. doi: 10.1007/978-1-0716-2376-3_7
69. Acevedo-Acevedo S, Millar DC, Simmons AD, Favreau P, Cobra PF, Skala M, et al. Metabolomics revealed the influence of breast cancer on lymphatic endothelial cell metabolism, metabolic crosstalk, and lymphangiogenic signaling in co-culture. Sci Rep. (2020) 10(1):21244. doi: 10.1038/S41598-020-76394-7
70. Halama A, Guerrouahen BS, Pasquier J, Satheesh NJ, Suhre K, Rafii A. Nesting of colon and ovarian cancer cells in the endothelial niche is associated with alterations in glycan and lipid metabolism. Sci Rep. (2017) 7:1–10. doi: 10.1038/srep39999
71. Strating E, Verhagen MP, Wensink E, Dünnebach E, Wijler L, Aranguren I, et al. Co-cultures of colon cancer cells and cancer-associated fibroblasts recapitulate the aggressive features of mesenchymal-like colon cancer. Front Immunol. (2023) 14:1053920/BIBTEX. doi: 10.3389/FIMMU.2023.1053920
72. Koukourakis MI, Kalamida D, Mitrakas AG, Liousia M, Pouliliou S, Sivridis E, et al. Metabolic cooperation between co-cultured lung cancer cells and lung fibroblasts. Lab Invest. (2017) 97:1321–31. doi: 10.1038/labinvest.2017.79
73. Goossens P, Rodriguez-Vita J, Etzerodt A, Masse M, Rastoin O, Gouirand V, et al. Membrane cholesterol efflux drives tumor-associated macrophage reprogramming and tumor progression. Cell Metab. (2019) 29:1376–1389.e4. doi: 10.1016/J.CMET.2019.02.016
74. Yang P, Qin H, Li Y, Xiao A, Zheng E, Zeng H, et al. CD36-mediated metabolic crosstalk between tumor cells and macrophages affects liver metastasis. Nat Commun. (2022) 13:1–16. doi: 10.1038/s41467-022-33349-y
75. Raffo-Romero A, Ziane-Chaouche L, Salomé-Desnoulez S, Hajjaji N, Fournier I, Salzet M, et al. A co-culture system of macrophages with breast cancer tumoroids to study cell interactions and therapeutic responses. Cell Rep Methods. (2024) 4(6):100792. doi: 10.1016/j.crmeth.2024.100792
76. Chen P, Han Y, Wang L, Zheng Y, Zhu Z, Zhao Y, et al. Spatially resolved metabolomics combined with the 3D tumor-immune cell coculture spheroid highlights metabolic alterations during antitumor immune response. Anal Chem. (2023) 95:15153–61. doi: 10.1021/ACS.ANALCHEM.2C05734
77. Jeong SR, Kang M. Exploring tumor–immune interactions in co-culture models of T cells and tumor organoids derived from patients. Int J Mol Sci. (2023) 24:14609. doi: 10.3390/IJMS241914609
78. Bader JE, Voss K, Rathmell JC. Targeting metabolism to improve the tumor microenvironment for cancer immunotherapy. Mol Cell. (2020) 78:1019. doi: 10.1016/J.MOLCEL.2020.05.034
79. Kenerson HL, Sullivan KM, Seo YD, Stadeli KM, Ussakli C, Yan X, et al. Tumor slice culture as a biologic surrogate of human cancer. Ann Transl Med. (2020) 8:114–4. doi: 10.21037/ATM.2019.12.88
80. Mendes R, Graça G, Silva F, Guerreiro ACL, Gomes-Alves P, Serpa J, et al. Exploring metabolic signatures of ex vivo tumor tissue cultures for prediction of chemosensitivity in ovarian cancer. Cancers (Basel). (2022) 14(18):4460. doi: 10.3390/CANCERS14184460
81. Sellers K, Fox MP, Ii MB, Slone SP, Higashi RM, Miller DM, et al. Pyruvate carboxylase is critical for non-small-cell lung cancer proliferation. J Clin Invest. (2015) 125:687–98. doi: 10.1172/JCI72873
82. Ko J, Park D, Lee S, Gumuscu B, Jeon NL. Engineering organ-on-a-chip to accelerate translational research. Micromachines. (2022) 13:1200. doi: 10.3390/MI13081200
83. Mastrangeli M, Millet S, Van den Eijnden-van Raaij J. Organ-on-chip in development: Towards a roadmap for organs-on-chip. ALTEX. (2019) 36:650–68. doi: 10.14573/ALTEX.1908271
84. Lopez-Muñoz GA, Mugal S, Ramón-Azcón J. Sensors and biosensors in organs-on-a-chip platforms. Adv Exp Med Biol. (2022) 1379:55–80. doi: 10.1007/978-3-031-04039-9_3
85. Dornhof J, Kieninger J, Muralidharan H, Maurer J, Urban GA, Weltin A. Microfluidic organ-on-chip system for multi-analyte monitoring of metabolites in 3D cell cultures. Lab Chip. (2022) 22:225–39. doi: 10.1039/D1LC00689D
86. Kalfe A, Telfah A, Lambert J, Hergenröder R. Looking into living cell systems: planar waveguide microfluidic NMR detector for in vitro metabolomics of tumor spheroids. Anal Chem. (2015) 87:7402–10. doi: 10.1021/ACS.ANALCHEM.5B01603
87. Chen Q, Wu J, Zhang Y, Lin JM. Qualitative and quantitative analysis of tumor cell metabolism via stable isotope labeling assisted microfluidic chip electrospray ionization mass spectrometry. Anal Chem. (2012) 84:1695–701. doi: 10.1021/AC300003K
88. Jeibouei S, Hojat A, Mostafavi E, Aref AR, Kalbasi A, Niazi V, et al. Radiobiological effects of wound fluid on breast cancer cell lines and human-derived tumor spheroids in 2D and microfluidic culture. Sci Rep. (2022) 12:1–21. doi: 10.1038/s41598-022-11023-z
89. Cauli E, Polidoro MA, Marzorati S, Bernardi C, Rasponi M, Lleo A. Cancer-on-chip: a 3D model for the study of the tumor microenvironment. J Biol Eng. (2023) 17:1–25. doi: 10.1186/S13036-023-00372-6
90. Lobel GP, Jiang Y, Simon MC. Tumor microenvironmental nutrients, cellular responses, and cancer. Cell Chem Biol. (2023) 30:1015–32. doi: 10.1016/J.CHEMBIOL.2023.08.011
91. Gkiouli M, Biechl P, Eisenreich W, Otto AM. Diverse roads taken by 13C-glucose-derived metabolites in breast cancer cells exposed to limiting glucose and glutamine conditions. Cells. (2019) 8(10):1113. doi: 10.3390/CELLS8101113
92. Nestor CE, Ottaviano R, Reinhardt D, Cruickshanks HA, Mjoseng HK, McPherson RC, et al. Rapid reprogramming of epigenetic and transcriptional profiles in mammalian culture systems. Genome Biol. (2015) 16:1–17. doi: 10.1186/S13059-014-0576-Y
93. Edgar RD, Perrone F, Foster AR, Payne F, Lewis S, Nayak KM, et al. Culture-associated DNA methylation changes impact on cellular function of human intestinal organoids. Cell Mol Gastroenterol Hepatol. (2022) 14:1295. doi: 10.1016/J.JCMGH.2022.08.008
94. Wiig H, Swartz MA. Interstitial fluid and lymph formation and transport: Physiological regulation and roles in inflammation and cancer. Physiol Rev. (2012) 92:1005–60. doi: 10.1152/PHYSREV.00037.2011
95. Sullivan MR, Danai LV, Lewis CA, Chan SH, Gui DY, Kunchok T, et al. Quantification of microenvironmental metabolites in murine cancers reveals determinants of tumor nutrient availability. Elife. (2019) 8:e44235. doi: 10.7554/ELIFE.44235
96. Gardner GL, Moradi F, Moffatt C, Cliche M, Garlisi B, Gratton J, et al. Rapid nutrient depletion to below the physiological range by cancer cells cultured in Plasmax. Am J Physiol Cell Physiol. (2022) 323:C823–34. doi: 10.1152/AJPCELL.00403.2021
97. Hopkins E, Sanvictores T, Sharma S. Urolithiasis.StatPearls Publishing (Boston, MA: Springer) (2022). 19–22 p. doi: 10.1007/978-1-4899-0873-5_4
98. Segeritz CP, Vallier L. Cell culture: growing cells as model systems in vitro. In: Basic Science Methods for Clinical Researchers (2017). (Academic Press) p. 151. doi: 10.1016/B978-0-12-803077-6.00009-6
99. Alva R, Gardner GL, Liang P, Stuart JA. Supraphysiological oxygen levels in mammalian cell culture: current state and future perspectives. Cells. (2022) 11:3123. doi: 10.3390/CELLS11193123
100. McKeown SR. Defining normoxia, physoxia and hypoxia in tumours-implications for treatment response. Br J Radiol. (2014) 87(1035):20130676. doi: 10.1259/BJR.20130676
101. Kato Y, Ozawa S, Miyamoto C, Maehata Y, Suzuki A, Maeda T, et al. Acidic extracellular microenvironment and cancer. Cancer Cell Int. (2013) 13:89. doi: 10.1186/1475-2867-13-89
102. Vaupel P. Tumor microenvironmental physiology and its implications for radiation oncology. Semin Radiat Oncol. (2004) 14:198–206. doi: 10.1016/J.SEMRADONC.2004.04.008
103. Hao G, Xu ZP, Li L. Manipulating extracellular tumour pH: an effective target for cancer therapy. RSC Adv. (2018) 8:22182–92. doi: 10.1039/C8RA02095G
104. Abbas M, Moradi F, Hu W, Regudo KL, Osborne M, Pettipas J, et al. Vertebrate cell culture as an experimental approach - limitations and solutions. Comp Biochem Physiol B Biochem Mol Biol. (2021) 254:110570. doi: 10.1016/J.CBPB.2021.110570
Keywords: cancer metabolism, cell culture conditions, organoids, tumor microenvironment, physiologic media, oxygen, pH
Citation: Kes MMG, Berkers CR and Drost J (2024) Bridging the gap: advancing cancer cell culture to reveal key metabolic targets. Front. Oncol. 14:1480613. doi: 10.3389/fonc.2024.1480613
Received: 14 August 2024; Accepted: 26 August 2024;
Published: 17 September 2024.
Edited by:
Ewa Krawczyk, Georgetown University, United StatesReviewed by:
Angela M. Otto, Technical University of Munich, GermanyCopyright © 2024 Kes, Berkers and Drost. This is an open-access article distributed under the terms of the Creative Commons Attribution License (CC BY). The use, distribution or reproduction in other forums is permitted, provided the original author(s) and the copyright owner(s) are credited and that the original publication in this journal is cited, in accordance with accepted academic practice. No use, distribution or reproduction is permitted which does not comply with these terms.
*Correspondence: Celia R. Berkers, C.R.Berkers@uu.nl; Jarno Drost, J.Drost@prinsesmaximacentrum.nl