- 1Department of Digestive Tumor, The Second Affiliated Hospital of Fujian Medical University, Quanzhou, China
- 2Department of Gynecology and Obstetrics, The Second Affiliated Hospital of Fujian Medical University, Quanzhou, China
- 3Department of Oncology, The Second Affiliated Hospital of Fujian Medical University, Quanzhou, China
Purpose: This study aimed to develop and validate a model for accurately assessing the risk of distant metastases in patients with gastric cancer (GC).
Methods: A total of 301 patients (training cohort, n = 210; testing cohort, n = 91) with GC were retrospectively collected. Relevant clinical predictors were determined through the application of univariate and multivariate logistic regression analyses. Then the clinical model was established. Venous phase computed tomography (VPCT) images were utilized to extract radiomic features, and relevant features were selected using univariate analysis, Spearman correlation coefficient, and the least absolute shrinkage and selection operator (Lasso) regression. Subsequently, radiomics scores were calculated based on the selected features. Radiomics models were constructed using five machine learning algorithms according to the screened features. Furthermore, separate joint models incorporating radiomic features and clinically independent predictors were established using traditional logistic regression algorithms and machine learning algorithms, respectively. All models were comprehensively assessed through discrimination, calibration, reclassification, and clinical benefit analysis.
Results: The multivariate logistic regression analysis revealed that age, histological grade, and N stage were independent predictors of distant metastases. The radiomics score was derived from 15 selected features out of a total of 944 radiomic features. The predictive performance of the joint model 1 [AUC (95% CI) 0.880 (0.811-0.949)] constructed using logistic regression is superior to that of the joint model 2 [AUC (95% CI) 0.834 (0.736-0.931)] constructed using SVM algorithm. The joint model 1 [AUC(95% CI) 0.880(0.811-0.949)], demonstrated superior performance compared to the clinical model [AUC(95% CI) 0.781(0.689-0.873)] and radiomics model [AUC(95% CI) 0.740(0.626-0.855), using LR algorithm]. The NRI and IDI values for the joint model 1 and clinical model were 0.115 (95% CI 0.014 -0.216) and 0.132 (95% CI 0.093-0.171), respectively; whereas for the joint model 1 and LR model, they were found to be 0.130 (95% CI 0.018-0.243) and 0.116 (95% CI 0.072-0.160), respectively. Decision curve analysis indicated that the joint model 1 exhibited a higher clinical net benefit than other models.
Conclusions: The nomogram of the joint model, integrating radiomic features and clinically independent predictors, exhibits robust predictive capability for early identification of high-risk patients with a propensity for distant metastases of GC.
1 Introduction
Gastric cancer (GC) is a prevalent malignancy globally, ranking fifth in terms of its incidence and fourth in terms of its mortality rate (1). It is well-established that the prognosis of GC varies significantly depending on the treatment approach employed at different stages (2). Studies have reported that at initial diagnosis, distant metastases are observed in approximately 21.9-44.6% of patients with GC (3, 4), leading to an unfavorable prognosis with a median overall survival of merely 5 months and a 5-year survival rate as low as 3.9% (5). Therefore, early identification and effective intervention targeting high-risk patients with distant metastases can substantially enhance patient outcomes.
The primary modality for identifying distant metastases in clinical practice is computed tomography (CT) examination, which offers advantages such as high spatial resolution, strong non-invasiveness, and robust technical support for image processing. However, the CT diagnosis of distant metastasis in GC exhibits characteristics of high specificity but low sensitivity (6). MRI and PET-CT serve as adjunctive modalities for detecting distant metastasis in conjunction with CT, yet their sensitivity remains limited (6). Moreover, existing imaging techniques can only identify present distant metastases and cannot predict the risk of future occurrences. Therefore, clinicians encounter considerable difficulty in precisely identifying distant metastases, highlighting the pressing requirement for the development of an innovative and accurate predictive approach that can serve as a supplementary diagnostic tool in detecting distant metastases among recently diagnosed GC patients.
Radiomics, an increasingly prominent field in recent years, employs high-throughput extraction of quantitative features to convert medical images into high-dimensional and minable data, followed by comprehensive data analysis for decision support (7). By comprehensively extracting quantitative features from regions of interest, radiomics can discern subtle differences in medical images that may elude human perception and quantify the extent of heterogeneity observed in neoplasms (8). Moreover, the quantitative features obtained from images can provide insights into biological aspects including cellular morphology, gene expression, and molecular properties (9). These features are relatively independent yet interconnected with traditional clinical and molecular attributes, thereby enhancing the accuracy of evidence-based medicine (10, 11). Prior research has showcased the resilience of radiomics models that employ CT scans to effectively forecast lymph node metastasis, assess ovarian metastasis, and detect peritoneal and omental metastasis among patients with GC (12–15). These findings offer promising prospects for utilizing radiomic features and clinical data to prognosticate the risk of distant metastases in individuals with GC.
Hence, we hypothesize that radiomics could be a valuable asset in forecasting the risk of distant metastases in GC. The primary aim of this study was to establish a predictive model for distant metastases using CT-based radiomic features and clinical data, with the ultimate goal of developing an individualized nomogram to offer an influential instrument for personalized therapy of patients with GC.
2 Materials and methods
2.1 Patients
The present study retrospectively screened all cases of GC initially diagnosed at the Second Affiliated Hospital of Fujian Medical University from January 2018 to December 2022, as retrieved from the database.
The stated criteria were utilized for the purpose of inclusion: (1) histologically confirmed primary GC; (2) comprehensive evaluation of distant metastases using whole-body 18F-FDG-PET-CT or contrast-enhanced CT scans of the chest, abdomen, and pelvis, MRI of the brain, and radionuclide bone imaging prior to treatment initiation. Exclusion criteria included: (1) coexistence with other primary malignant tumors; (2) Siewert type I esophagogastric junction tumors; (3) insufficient clinical data; and (4) obvious artifacts or poor gastric distension on CT images. A total of 301 patients meeting these criteria were randomly allocated to either a training cohort or a testing cohort, with a distribution ratio of 7:3.
This study received ethical approval from the Ethics Committee of the Second Affiliated Hospital of Fujian Medical University, and the need for obtaining informed consent was waived. The research flowchart is illustrated in Figure 1.
2.2 Data and images collection
The collected data encompassed clinical characteristics and VPCT images. Clinical characteristics of patients were extracted from the medical record system, including age, gender, tumor location, tumor size, histological classification and grade, T stage, N stage, M stage, CEA and CA19-9 levels, neutrophil-to-lymphocyte ratio (NLR), platelet-to-lymphocyte ratio (PLR), as well as metastatic site information. Distant metastasis is defined as non-regional lymph node metastasis or distant organ metastasis confirmed by biopsy. For gastric cancer patients initially diagnosed with distant metastasis via computed tomography (CT), a subset may forgo biopsy. However, the presence of metastatic disease can be confirmed if there is a change in size of the lesions during post-treatment follow-up, leading to classification as stage M1.
The CT images are acquired from the radiology department and saved in the Digital Imaging and Communication in Medicine (DICOM) format. All subjects underwent comprehensive multi-phase contrast-enhanced CT examinations, including arterial, venous, and delayed phases, in addition to non-enhanced CT scans, prior to their respective treatments. The CT examination was performed using a 128-slice Philips Brilliance iCT and a Siemens dual-source CT scanner. Other scanning parameters involved setting the tube voltage at 120 kV, adjusting the tube current within the range of 180 to 540 mA, maintaining a slice thickness of 2 mm, utilizing an image matrix size measuring 512×512, and determining pixel spacing as 0.765625×0.765625.
2.3 Image segmentation and radiomic features extraction
Image segmentation was performed using ITK-SNAP software (version 3.6.0; http://www.itksnap.org). A medical oncologist with 5 years of expertise in oncology manually segmented the regions of interest (ROI) for all subjects’ lesions, which were subsequently reviewed by a radiologist possessing a decade of professional expertise. Both doctors were blinded to the patients’ clinical conditions. The segmented CT image files were saved in Neuroimaging Informatics Technology Initiative(NIFTI) format. When conducting feature extraction, the ROI images underwent normalization and resampling to achieve a pixel spacing of 2.0mm×2.0mm×2.0mm, thereby ensuring accurate pixel size and slice thickness. Pyradiomics (16), an open-source Python package accessible at the following link (https://pyradiomics.readthedocs.io/en/latest/), was used for feature extraction.
Feature extraction was facilitated by employing Laplacian of Gaussian (LoG) and wavelet filters, which are renowned for their efficacy in enhancing relevant information and reducing noise within digital image processing. The ROIs can yield five distinct types of radiomic features: (1) first-order statistical features, (2) shape and size features, (3) texture features, (4) LoG features, and finally, (5) wavelet features.
To guarantee the reproducibility and reliability of radiomics features, 30 cases were randomly selected. One month after the initial segmentation, the same oncologist (Observer 1) and the same radiologist (Observer 2) resegmented the ROI to assess the intra- and inter-observer reproducibility of the extracted features. Only features with an intra-class correlation coefficient (ICC) greater than 0.75 for both inter- and intra-observer agreements were considered stable and selected for further analysis.
2.4 Feature selection and radiomics score construction
In the feature selection process, we employed univariate analysis to eliminate irrelevant features. The Spearman correlation coefficient was utilized to identify feature pairs exhibiting a high degree of correlation. Feature pairs characterized by a correlation coefficient absolute value exceeding 0.9 were deemed to have a strong correlation and thus, only one of the two feature was retained. The remaining features were standardized using the formula (X − μ)/σ, where μ is the mean and σ is the standard deviation of each feature. Subsequently, the least absolute shrinkage and selection operator regression (Lasso) was applied to identify radiomic features that exhibit the strongest correlation with distant metastasis in GC. The model’s correlation coefficients and constants were calculated, leading to derivation of the radiomics scoring formula. Figure 2 illustrates the methodology for creating the radiomics score (radscore).
2.5 Clinical model construction
We employed univariate and multivariate logistic regression analyses in order to ascertain the clinically independent predictors. Subsequently, we constructed clinical nomograms incorporating these independent predictors.
2.6 Radiomics models construction
We utilize selected radiomic features to construct radiomics models employing various machine learning algorithms, including support vector machines (SVM), logistic regression (LR), random forests (RF), naive Bayes (NB), and extreme gradient boosting models (XGB). We then compare the effectiveness of these models to determine the optimal algorithm.
2.7 Radiomics-clinical model construction
Relevant clinical predictors that showed statistical significance in the univariate regression analysis were incorporated into a logistic multivariate regression analysis along with radscore. Afterwards, we developed the joint model 1 by including variables with a P-value below 0.05. Furthermore, we incorporated both clinical features and radiomic features into the feature selection process, identifying the clinical and radiomic features most strongly associated with distant metastasis of GC through feature selection. Subsequently, we constructed joint models using the aforementioned five machine learning algorithms and determined the optimal machine learning joint model 2 by comparing the effectiveness of these models. Finally, by comparing the predictive performance of joint model 1 and joint model 2, we identified the best joint model and compared it with the clinical model and the best radiomics model.
2.8 Model evaluation
The effectiveness of the models was gauged through the AUC, indicating the area under the receiver operating characteristic (ROC) curve. To compare the AUC values across different models, we employed the DeLong test. The calibration of the best joint model was evaluated using a calibration curve and Hosmer-Lemeshow test. Net reclassification improvement (NRI) and integrated discrimination improvement (IDI) were computed to compare performance across various models. Additionally, decision curve analysis (DCA) was conducted to evaluate the practical value of our models by quantifying net benefit at various threshold probabilities. Ultimately, the sensitivity and specificity of the best joint model were determined and compared with those of conventional computed tomography (CT) for diagnostic accuracy.
2.9 Statistical analysis
The statistical analysis was conducted using IBM SPSS software (version 27.0), Python software (version 3.11.4), and R software (version 4.4.0; https://www.r-project.org). Clinical baseline characteristics were compared between the training cohort and testing cohort, employing the Chi-square test for categorical variables and the Mann-Whitney test for continuous variables. Additionally, the Mann-Whitney test was utilized to assess radscore consistency between the training and testing cohort. A statistical significance was determined when the p-value was less than 0.05 on both sides.
3 Results
3.1 Clinical characteristics
Among the 301 eligible cases, 202 presented no distant metastasis while 99 exhibited distant metastasis at initial diagnosis, resulting in an incidence rate of 32.9%. Peritoneal dissemination emerged as the predominant location for distant metastasis (42.4%), closely trailed by hepatic involvement (39.4%) and non-regional lymph node infiltration (30.3%). Less frequent sites of metastasis included the lung (14.1%), adrenal glands (6.1%), bone (5.1%), pancreas (5.1%), spleen (2.0%), abdominal wall (2.0%), ovary (2.0%), and brain (1.0%). The distribution of metastases was characterized by 57 cases (57.6%) with involvement of a single organ and 43 cases (43.4%) with involvement of multiple organs, with non-regional lymph nodes being counted as one organ.
The entire cohort was divided into two cohorts, namely a training cohort (n=210) and a testing cohort (n=91), using a random allocation method with a ratio of 7:3. In the training cohort, distant metastasis was identified in only 69 cases (32.9%), whereas 141 cases (67.1%), exhibited no indications of distant metastasis. Similarly, within the testing cohort, there were 30 cases (33.0%) with distant metastasis and 61 cases (67.0%) without distant metastasis observed. Comparison of clinical characteristics between these two cohorts revealed no significant differences in baseline characteristics (Table 1), indicating satisfactory data division outcomes.
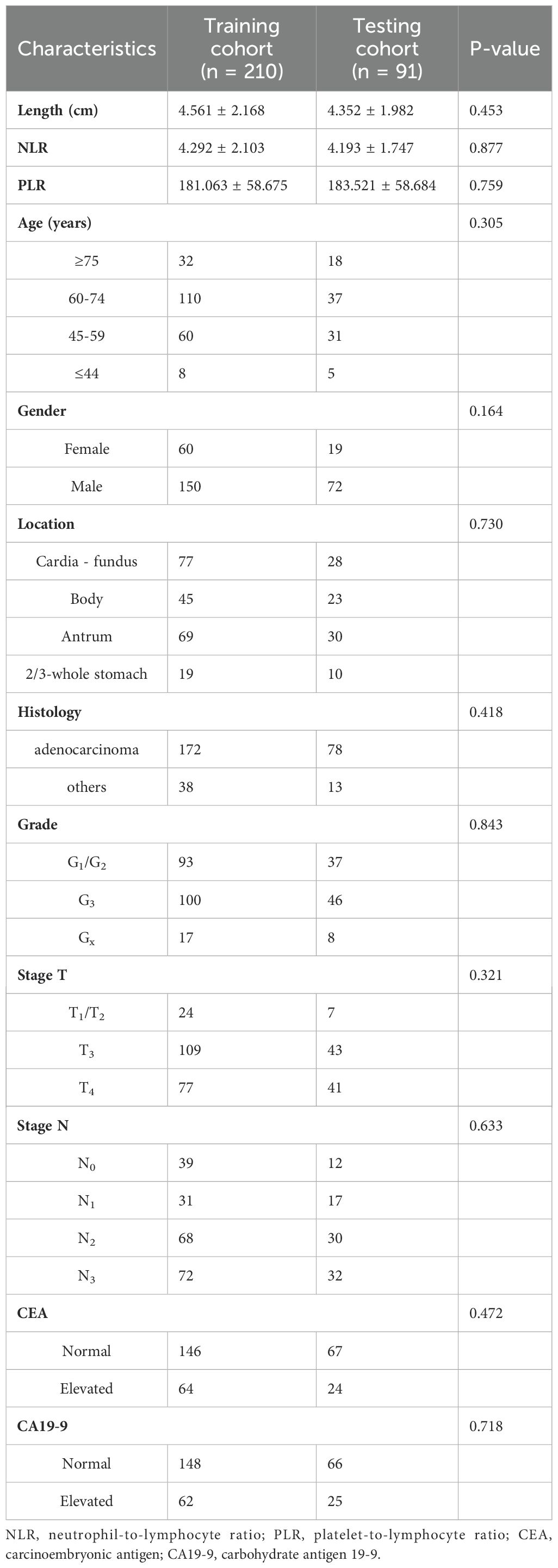
Table 1. Comparison of baseline characteristics between the training and testing cohorts in patients with gastric cancer.
3.2 Features selection and radiomics score
A total of 944 radiomic features were extracted from the ROIs, and after conducting ICC, univariate analysis, Spearman correlation coefficient, and applying the Lasso regression algorithm, we ultimately selected 15 features that exhibited the strongest association with distant metastasis of GC. The calculation formula for radscore is illustrated in Figure 2.
In the training cohort, there was a significant difference in radscores between patients with and without distant metastasis (mean ± SD: 0.445 ± 0.141 vs 0.272 ± 0.145; z = -7.216, P < 0.001). A similar trend was observed in the testing cohort where patients with distant metastasis had higher radscores compared to those without (mean ± SD: 0.429 ± 0.173 vs 0.294 ± 0.163; z = -3.672, P < 0.001). Furthermore, the radscore of the training cohort was 0.329 ± 0.165, while the radscore of the testing cohort was 0.338 ± 0.177 (z = -1.001, P = 0.317), indicating satisfactory data division outcomes.
3.3 Development and validation of the clinical model
The univariate logistic regression analysis revealed significant differences in age, histological grade, T stage, N stage, CEA, and CA19-9 (Table 2). Subsequently, a multivariate logistic regression analysis was conducted on the aforementioned independent predictors. The results demonstrated that age, histological grade, and N stage were clinically independent predictors (P<0.05).
Based on these predictors obtained from the final screening, we developed a clinical prediction model. The AUC of the training cohort was found to be 0.797 (95% CI 0.738-0.856). Furthermore, the AUC of the testing cohort was found to be 0.781 (95% CI 0.689-0.873). Figure 3 presents the nomogram along with ROC and calibration curves illustrating our clinical prediction model.
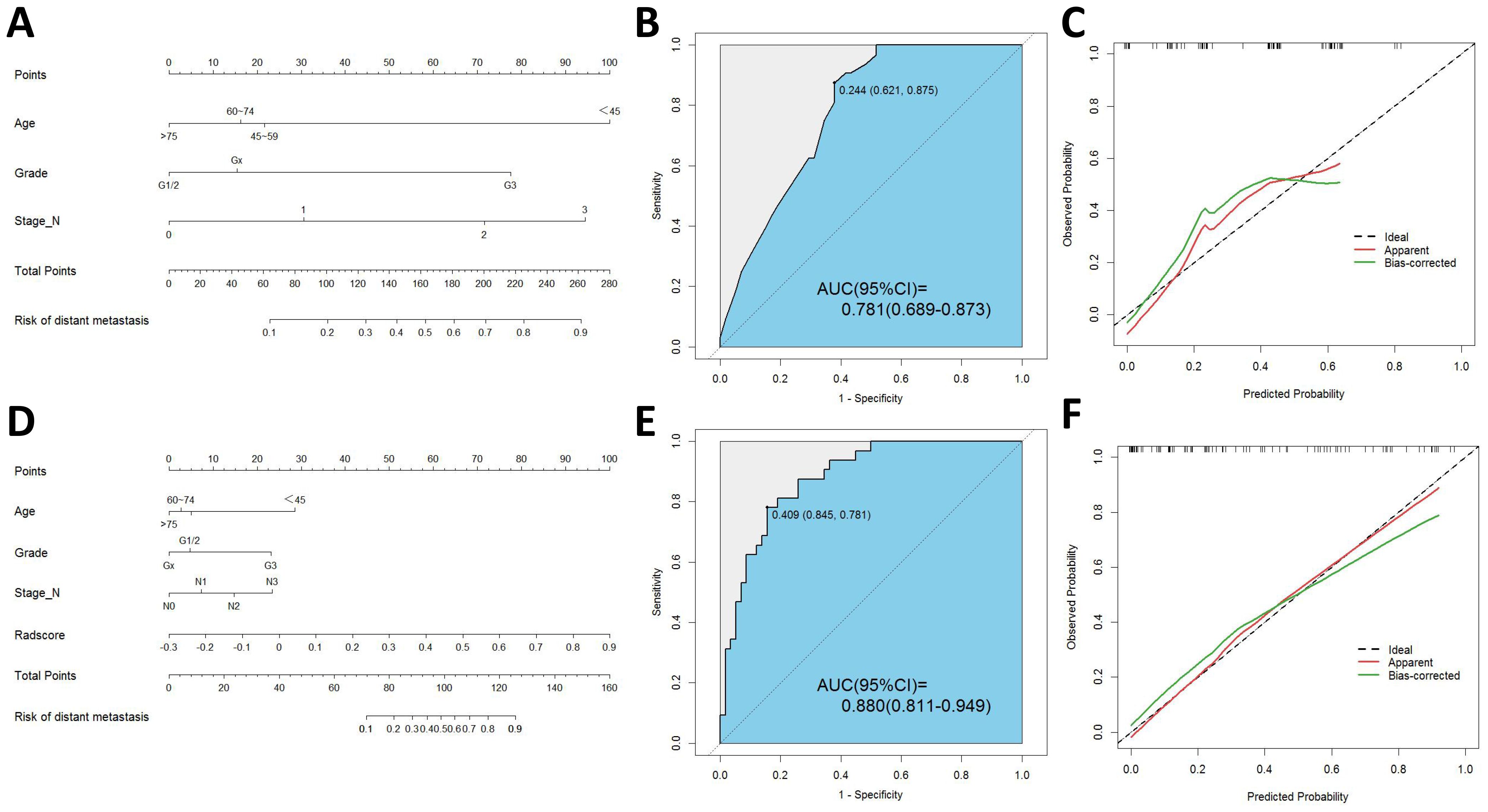
Figure 3. (A) Nomogram of clinical model. (B) ROC curve of clinical model. (C) Calibration curve of clinical model. (D) Nomogram of radiomics-clinical model. (E) ROC curve of radiomics-clinical model. (F) Calibration curve of radiomics-clinical model.
3.4 Development and validation of the radiomics models
Different machine learning algorithms, including support vector machine (SVM), logistic regression (LR), Random forest (RF), Naive Bayes (NB), and extreme gradient enhancement model (XGB), were employed to develop the radiomics models. Figure 4A presents the AUC results of all models in the testing cohort except for the machine learning joint models, revealing that the LR model exhibits the best performance among the radiomics models.
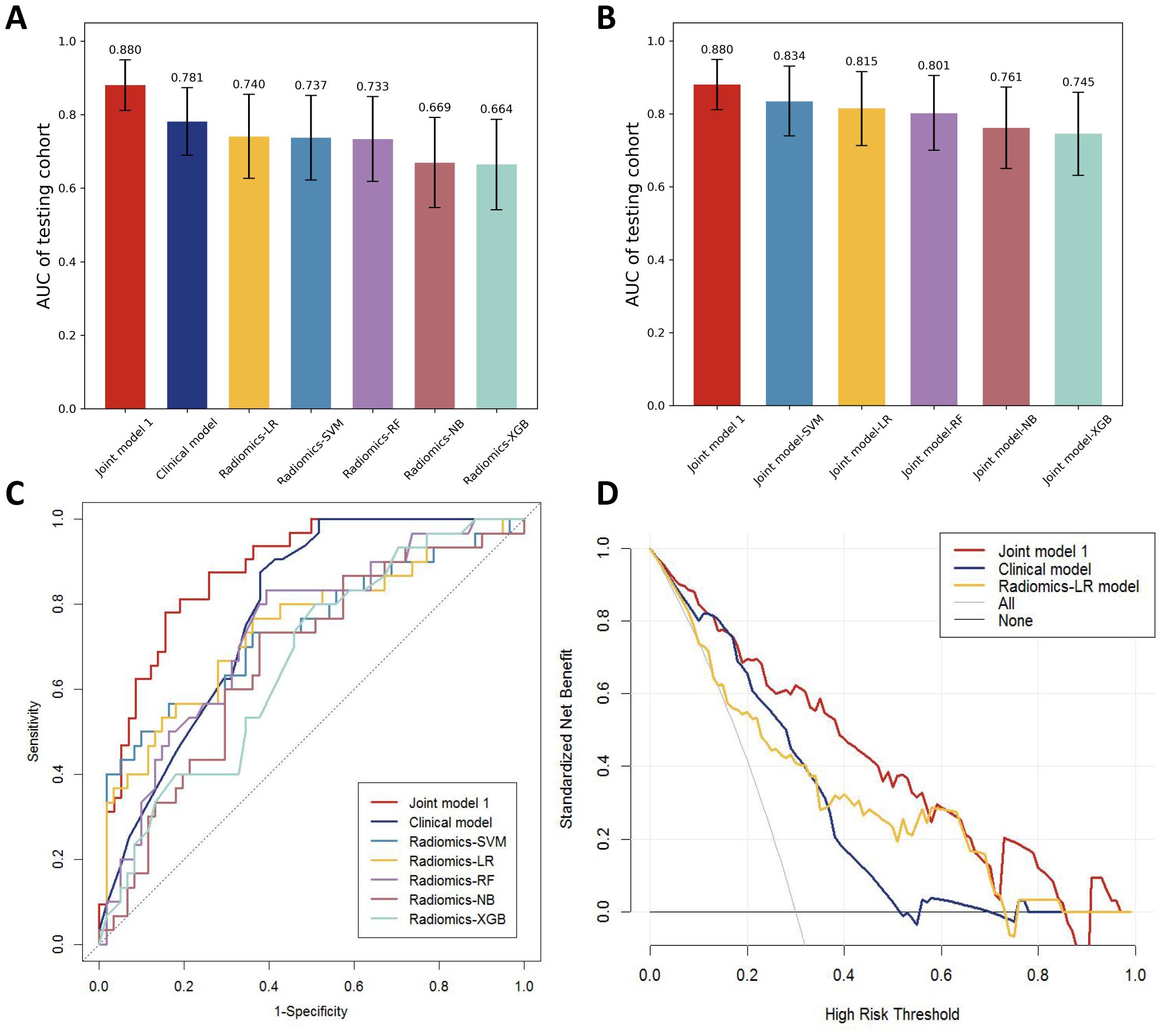
Figure 4. (A) AUC comparison of testing cohorts for all models. (B) AUC comparison of testing cohorts for all joint models. (C) ROC comparison of testing cohorts for all models. (D) DCA curve.
3.5 Development and validation of the radiomics–clinical model
The univariate logistic regression analysis revealed a statistically significant difference in radscore between patients with distant metastasis and those without (P < 0.001). The logistic multivariate regression analysis incorporated the radscore and clinical characteristics that demonstrated statistical significance in the univariate regression analysis. The results revealed significant statistical associations (P < 0.05) between age, histological grade, N stage, and radscore (Table 2), leading to the establishment of the joint model 1. In the training cohort, the AUC was 0.865 (95% CI 0.812-0.918); while in the testing cohort, the AUC was 0.880(95% CI 0.811-0.949).
By incorporating both clinical and radiomic features into the feature selection process, we identified 22 features with the strongest association with distant metastasis of GC after the selection. The clinical features included age, histological grade, N stage, and CEA, while the radiomic features included original_glcm_Imc2, log-sigma-3-0-mm-3D_firstorder_Median, log-sigma-3-0-mm-3D_glcm_MCC, log-sigma-3-0-mm-3D_glrlm_ShortRunLowGrayLevelEmphasis, log-sigma-3-0-mm-3D_glszm_HighGrayLevelZoneEmphasis, log-sigma-3-0-mm-3D_glszm_SmallAreaLowGrayLevelEmphasis, wavelet-LLH_firstorder_Median, wavelet-LLH_glrlm_LongRunHighGrayLevelEmphasis, wavelet-LLH_glszm_GrayLevelVariance, wavelet-LLH_glszm_HighGrayLevelZoneEmphasis, wavelet-LLH_glszm_SmallAreaEmphasis, wavelet-LHL_firstorder_Median, wavelet-LHL_glszm_ZoneVariance, wavelet-HLH_glszm_SmallAreaHighGrayLevelEmphasis, wavelet-HHL_glcm_Imc1, wavelet-LLL_firstorder_TotalEnergy, wavelet-LLL_firstorder_Variance, and wavelet-LLL_glcm_InverseVariance. Using the aforementioned features, we constructed five machine learning joint models. Figure 4B presents the AUC of joint model 1 and all machine learning joint models in the testing cohort, demonstrating that the SVM algorithm-built joint model 2 performed the best. In the training cohort, the AUC was 0.842 (95% CI 0.780-0.904); in the testing cohort, the AUC was 0.834 (95% CI 0.736-0.931). The DeLong test indicated that, compared to joint model 2, joint model 1 had superior discriminative performance (P < 0.05). Figure 3 presents the nomogram along with ROC and calibration curves illustrating our radiomics-clinical prediction model 1.
3.6 Evaluation of models
The performance of the clinical model, radiomics models, and joint model 1 was compared in Figure 4C. The DeLong test demonstrated that the joint model 1 exhibited significantly better discriminatory capability in comparison to both the clinical model and radiomics models (P < 0.05). The calibration curve indicated a good agreement between predicted and observed values for the joint model 1. Furthermore, the Hosmer-Lemeshow test did not find any statistically significant difference for the joint model 1 (p = 0.5419), suggesting a well-fitted joint model. The NRI and IDI values for the joint model 1 and clinical model were 0.115 (95% CI 0.014 -0.216) and 0.132 (95% CI 0.093-0.171), respectively; whereas for the joint model 1 and LR model, they were found to be 0.130 (95% CI 0.018-0.243) and 0.116 (95% CI 0.072-0.160), respectively. These results indicate a significant improvement in predictive power when comparing the joint model 1 to both the clinical model and LR model. The decision curve analysis depicted in Figure 4D demonstrates that across various threshold probabilities, net benefit is better for the joint model 1 than for other models. The sensitivity and specificity of CT for the diagnosis of distant metastasis are detailed in Table 3. The sensitivity of CT is 65.7%, with a specificity of 98.5%. In contrast, the sensitivity of the joint model 1 reaches 78.1%, and its specificity is 84.5%.

Table 3. The sensitivity and specificity of M staging by CT using histological examination as the reference standard.
4 Discussion
Through integration of clinical data and radiomic features, we have successfully developed and validated a nomogram model to precisely forecast the occurrence of distant metastases in gastric cancer. This constructed nomogram can serve as a user-friendly and non-invasive tool for individualized treatment of GC patients.
To construct the most precise prediction model, we developed a diverse range of models including clinical model, radiomics models, and joint model. The clinical model exhibited strong performance (AUC = 0.781). Out of the different machine learning models assessed, the LR model demonstrated exceptional performance with an AUC score of 0.740, slightly lower than that of the clinical model; however, statistical analysis using Delong test revealed no significant difference in AUC between these two models (p > 0.05). Among the joint models 2 constructed using machine learning algorithms, the SVM algorithm demonstrated the best performance. However, compared to joint model 2, joint model 1 achieved superior performance (AUC = 0.880, Delong test, p < 0.05). Furthermore, compared to radiomic models built using machine learning algorithms, the predictive performance of the machine learning joint models was enhanced. These results indicate that integrating radiomics with clinical features enhances diagnostic efficiency within this prediction model framework. Furthermore, we employed DCA curves along with NRI and IDI metrics to comprehensively evaluate various models’ performances. While ROC curves evaluate predictive accuracy solely based on discrimination ability, DCA curves offer valuable insights into the potential drawbacks and advantages associated with false negatives and false positives (17). NRI quantifies differences in correct classification rates between two classifiers by measuring variations in sensitivity and specificity sums (18). Similarly to NRI, IDI measures gaps in prediction probabilities (19). Both indicators are suitable for comparative analyses among different models. In clinical practice, the principal modality for detecting distant metastasis in gastric cancer patients is CT examination. However, studies by Leeman, Feng, Pan, et al. (20–22), have reported that the overall sensitivity of CT in diagnosing distant metastasis varies from 14.3 to 59.1%, with a specificity ranging from 89.6 to 99.8%. In the present study, the sensitivity and specificity of CT for diagnosing distant metastasis were 65.7% and 98.5%, respectively, whereas our joint model exhibited a sensitivity of 78.1% and a specificity of 84.5%. The sensitivity of our model in diagnosing distant metastasis is significantly higher than that of CT scans, which implies a substantial reduction in the rate of missed diagnoses in clinical practice. The specificity of our model is only slightly lower than that of CT scans, suggesting its potential as an auxiliary diagnostic tool to complement CT scans and reduce the rate of false positives.
Radiomics has found extensive application in the investigation of malignancy, including lung cancer, colorectal cancer, thyroid cancer, and gastric cancer (23–26). Moreover, at the microscopic scale, radiomics is employed to evaluate the immune environment and immunotherapy response of tumors (27). In previous studies on gastric cancer, radiomics has been applied to predict lymph node metastasis, peritoneal metastasis, response to neoadjuvant chemotherapy, and long-term survival (12, 14, 28, 29). Gao developed a CT-based radiomic model for predicting lymph node metastasis in gastric cancer with excellent discriminative ability (AUC = 0.89) (12). Dong successfully employed CT radiomics to accurately identify occult peritoneal metastases in advanced gastric cancer patients both in the training set (AUC = 0.958) and test set (AUC = 0.941) (14). Radiomics has also been employed to forecast neoadjuvant chemotherapy response in locally advanced gastric cancer (AUC = 0.736) (28). These results emphasize the considerable potential of radiomics in the development of predictive models. Similarly, our study shows that the joint model achieves excellent accuracy in predicting distant metastases in GC patients, with an AUC of 0.865 for the training cohort and 0.880 for the testing cohort.
Utilizing multivariate logistic regression analysis, we identified age, histological grade, and N stage as independent clinical predictors for distant metastasis in GC patients. Earlier research has documented an increased prevalence of lymph node metastasis among gastric cancer patients in the younger age group (30, 31), suggesting that increased lymph node metastasis in younger patients may contribute to distant metastasis. Furthermore, our findings indicate that patients with poorly differentiated gastric cancer are more prone to developing distant metastases compared to those with moderately and highly differentiated tumors, potentially due to the enhanced growth capacity and invasive potential of poorly differentiated gastric cancer tissues in infiltrating surrounding tissues, capillaries, and lymphatics. Our study incorporated systemic immunoinflammatory markers, including NLR and PLR, as clinically independent predictors for distant metastases. This is due to the strong correlation between tumor development and advancement and inflammation, wherein inflammatory cells facilitate cancer cell proliferation, angiogenesis, and invasion (32). Neutrophils modify the tumor microenvironment and secrete inflammatory mediators to promote tumor cell proliferation, invasion, and metastasis (33). Platelet activation acts as a chemical attractant inducing cancer cell metastasis (34). Lymphocytes have a significant impact on suppressing the growth and spread of cancer cells by employing cytokine-induced cytotoxicity, thereby contributing to effective immune responses against malignancies (35). According to Osama et al., NLR and PLR are linked to gastric cancer distant metastasis (36); however, our study did not identify them as independent predictors of distant metastasis. This discrepancy may be attributed to an inadequate sample size.
To enhance the richness of image information acquisition, this study employs a Laplacian of Gaussian (LoG) filter and a wavelet filter in the feature extraction process. The LoG filter, a second-order derivative filter, is primarily utilized for edge detection and feature point recognition (37). Conversely, the wavelet filter offers multi-scale and multi-directional analytical capabilities, and is extensively applied in denoising and image enhancement techniques (38). It is evident that the majority of the 15 selected features are derived from these two filters. Moreover, the Spearman correlation coefficient is applied to eliminate features with high inter-correlation, which aids in reducing the complexity of the model and mitigating the risk of overfitting. Subsequently, the radiomics models are established using machine learning algorithms such as SVM, LR, RF, NB, and XGB, based on the selected features. The findings suggest that LR, SVM, and RF-based radiomics models demonstrate comparable predictive efficacy as clinical features, thereby establishing themselves as dependable biomarkers for forecasting GC metastasis.
To the best of our knowledge, this study represents the first attempt to develop and validate a radiomics-based nomogram for predicting the risk of distant metastases in patients with GC. The utility of this approach lies in its utilization of readily available CT imaging technology and a well-established methodology to forecast the risk of distant metastases, without incurring additional financial burden for patients. Furthermore, the presentation of the model in a nomogram format allows for a more graphic representation of how various parameters influence the outcomes, thus facilitating its clinical application. However, several limitations of our research should be acknowledged. Firstly, despite the robust performance of the model, it requires a necessary suite of external validations to corroborate its generalizability. Consequently, future studies should incorporate a diverse patient population from different centers for validation purposes. Secondly, the current study is retrospective in nature and hence necessitates confirmation through additional prospective investigations. Lastly, given our selection of venous phase CT images for ROI segmentation, the predictive capabilities of extracting features from arterial phase and delayed phase CT images require additional verification.
5 Conclusions
Radiomics of venous phase CT images prior to treatment holds promise as a potential biomarker for predicting the occurrence of distant metastases in individuals diagnosed with gastric cancer. The radiomics-clinical model exhibits a remarkable predictive capability, offering significant value in the early identification of high-risk patients with a propensity for distant metastases, thereby providing a broad application potential in clinical decision-making.
Data availability statement
The raw data supporting the conclusions of this article will be made available by the authors, without undue reservation. Requests to access these datasets should be directed to H-BX, 1344206467@qq.com.
Ethics statement
The studies involving humans were approved by Ethics Committee of The Second Affiliated Hospital of Fujian Medical University. The studies were conducted in accordance with the local legislation and institutional requirements. Written informed consent for participation was not required from the participants or the participants’ legal guardians/next of kin in accordance with the national legislation and institutional requirements.
Author contributions
H-BX: Writing – original draft, Writing – review & editing, Conceptualization. M-LL: Software, Writing – original draft, Writing – review & editing. H-ZX: Formal analysis, Writing – review & editing. C-YW: Data curation, Writing – review & editing. T-WX: Funding acquisition, Supervision, Writing – review & editing. A-YZ: Methodology, Supervision, Writing – review & editing.
Funding
The author(s) declare financial support was received for the research, authorship, and/or publication of this article. This study was supported by Research Projects Directed by Doctoral Supervisors at the Second Affiliated Hospital of Fujian Medical University (Grant No. 2021GCC15).
Conflict of interest
The authors declare that the research was conducted in the absence of any commercial or financial relationships that could be construed as a potential conflict of interest.
Publisher’s note
All claims expressed in this article are solely those of the authors and do not necessarily represent those of their affiliated organizations, or those of the publisher, the editors and the reviewers. Any product that may be evaluated in this article, or claim that may be made by its manufacturer, is not guaranteed or endorsed by the publisher.
Supplementary material
The Supplementary Material for this article can be found online at: https://www.frontiersin.org/articles/10.3389/fonc.2024.1476340/full#supplementary-material
References
1. Sung H, Ferlay J, Siegel RL, Laversanne M, Soerjomataram I, Jemal A, et al. Global cancer statistics 2020: GLOBOCAN estimates of incidence and mortality worldwide for 36 cancers in 185 countries. CA Cancer J Clin. (2021) 71:209–49. doi: 10.3322/caac.21660
2. Ajani JA, D'amico TA, Bentrem DJ, Chao J, Cooke D, Corvera C, et al. Gastric cancer, version 2.2022, NCCN clinical practice guidelines in oncology. J Natl Compr Canc Netw. (2022) 20:167–92. doi: 10.6004/jnccn.2022.0008
3. Liu J, Sun R, Cai K, Xu Y, Yuan W. A nomogram combining neutrophil to lymphocyte ratio (NLR) and prognostic nutritional index (PNI) to predict distant metastasis in gastric cancer. Sci Rep. (2024) 14:15391. doi: 10.1038/s41598-024-65307-7
4. Zhang X, Wang X, Li W, Sun T, Diao D, Dang C. Predictive value of neutrophil-to-lymphocyte ratio for distant metastasis in gastric cancer patients. Sci Rep. (2022) 12:10269. doi: 10.1038/s41598-022-14379-4
5. Zhang Y, Lin Y, Duan J, Xu K, Mao M, Wang X. A population-based analysis of distant metastasis in stage IV gastric cancer. Med Sci Monitor. (2020) 26:e923867. doi: 10.12659/MSM.923867
6. Kwee RM. Modern imaging techniques for preoperative detection of distant metastases in gastric cancer. World J Gastroenterol. (2015) 21(37):10502–9. doi: 10.3748/wjg.v21.i37.10502
7. Gillies RJ, Kinahan PE, Hricak H. Radiomics: images are more than pictures, they are data. Radiology. (2016) 278:563–77. doi: 10.1148/radiol.2015151169
8. Ligero M, Garcia-Ruiz A, Viaplana C, Villacampa G, Raciti MV, Landa J, et al. A CT-based radiomics signature is associated with response to immune checkpoint inhibitors in advanced solid tumors. Radiology. (2021) 299:109–19. doi: 10.1148/radiol.2021200928
9. Aerts HJ, Velazquez ER, Leijenaar RT, Parmar C, Grossmann P, Carvalho S, et al. Decoding tumour phenotype by noninvasive imaging using a quantitative radiomics approach. Nat Commun. (2014) 5:4006. doi: 10.1038/ncomms5006
10. Aerts HJ. The potential of radiomic-based phenotyping in precision medicine: A review. JAMA Oncol. (2016) 2:1636–42. doi: 10.1001/jamaoncol.2016.2631
11. Lambin P, Leijenaar RTH, Deist TM, Peerlings J, de Jong EEC, van Timmeren J, et al. Radiomics: the bridge between medical imaging and personalized medicine. Nat Rev Clin Oncol. (2017) 14:749–62. doi: 10.1038/nrclinonc.2017.141
12. Gao X, Ma T, Cui J, Zhang Y, Wang L, Li H, et al. A CT-based radiomics model for prediction of lymph node metastasis in early stage gastric cancer. Acad Radiol. (2021) 28:e155–e64. doi: 10.1016/j.acra.2020.03.045
13. Zhang QW, Yang PP, Gao YJ, Li ZH, Yuan Y, Li SJ, et al. Assessing synchronous ovarian metastasis in gastric cancer patients using a clinical-radiomics nomogram based on baseline abdominal contrast-enhanced CT: a two-center study. Cancer Imaging. (2023) 23:71. doi: 10.1186/s40644-023-00584-5
14. Dong D, Tang L, Li ZY, Fang MJ, Gao JB, Shan XH, et al. Development and validation of an individualized nomogram to identify occult peritoneal metastasis in patients with advanced gastric cancer. Ann Oncol. (2019) 30:431–8. doi: 10.1093/annonc/mdz001
15. Wu A, Wu C, Zeng Q, Cao Y, Shu X, Luo L, et al. Development and validation of a CT radiomics and clinical feature model to predict omental metastases for locally advanced gastric cancer. Sci Rep. (2023) 13(1):8442. doi: 10.1038/s41598-023-35155-y
16. Van Griethuysen JJM, Fedorov A, Parmar C, Hosny A, Aucoin N, Narayan V, et al. Computational radiomics system to decode the radiographic phenotype. Cancer Res. (2017) 77:e104–e7. doi: 10.1158/0008-5472.CAN-17-0339
17. Van Calster B, Wynants L, Verbeek JFM, Verbakel JY, Christodoulou E, Vickers AJ, et al. Reporting and interpreting decision curve analysis: A guide for investigators. Eur Urol. (2018) 74:796–804. doi: 10.1016/j.eururo.2018.08.038
18. Barbour SJ, Coppo R, Zhang H, Liu ZH, Suzuki Y, Matsuzaki K, et al. Evaluating a new international risk-prediction tool in igA nephropathy. JAMA Intern Med. (2019) 179:942–52. doi: 10.1001/jamainternmed.2019.0600
19. Zhou ZR, Wang WW, Li Y, Jin KR, Wang XY, Wang ZW, et al. In-depth mining of clinical data: the construction of clinical prediction model with R. Ann Transl Med. (2019) 7:796. doi: 10.21037/atm.2019.08.63
20. Leeman MF, Patel D, Anderson J, OʼNeill JR, Paterson-Brown S. Multidetector computed tomography versus staging laparoscopy for the detection of peritoneal metastases in esophagogastric junctional and gastric cancer. Surg Laparosc Endosc Percutan Tech. (2017) 27:369–74. doi: 10.1097/SLE.0000000000000451
21. Feng XY, Wang W, Luo GY, Wu J, Zhou ZW, Li W, et al. Comparison of endoscopic ultrasonography and multislice spiral computed tomography for the preoperative staging of gastric cancer - results of a single institution study of 610 Chinese patients. PloS One. (2013) 8:e78846. doi: 10.1371/journal.pone.0078846
22. Pan Z, Zhang H, Yan C, Du L, Ding B, Song Q, et al. Determining gastric cancer resectability by dynamic MDCT. Eur Radiol. (2010) 20:613–20. doi: 10.1007/s00330-009-1576-2
23. Warkentin MT, Al-Sawaihey H, Lam S, Liu G, Diergaarde B, Yuan JM, et al. Radiomics analysis to predict pulmonary nodule Malignancy using machine learning approaches. Thorax. (2024) 79:307–15. doi: 10.1136/thorax-2023-220226
24. Cicalini I, Chiarelli AM, Chiacchiaretta P, Perpetuini D, Rosa C, Mastrodicasa D, et al. Multi-omics staging of locally advanced rectal cancer predicts treatment response: a pilot study. Radiol Med. (2024) 129:712–26. doi: 10.1007/s11547-024-01811-0
25. Feng JW, Liu SQ, Qi GF, Ye J, Hong LZ, Wu WX, et al. Development and validation of clinical-radiomics nomogram for preoperative prediction of central lymph node metastasis in papillary thyroid carcinoma. Acad Radiol. (2024) 31:2292–305. doi: 10.1016/j.acra.2023.12.008
26. Liu S, Deng J, Dong D, Fang M, Ye Z, Hu Y, et al. Deep learning-based radiomics model can predict extranodal soft tissue metastasis in gastric cancer. Med Phys. (2023) 51(1):267–277. doi: 10.1002/mp.16647
27. Sun Z, Zhang T, Ahmad MU, Zhou Z, Qiu L, Zhou K, et al. Comprehensive assessment of immune context and immunotherapy response via noninvasive imaging in gastric cancer. J Clin Invest. (2024) 134(6):e175834. doi: 10.1172/JCI175834
28. Wang W, Peng Y, Feng X, Zhao Y, Seeruttun SR, Zhang J, et al. Development and validation of a computed tomography–based radiomics signature to predict response to neoadjuvant chemotherapy for locally advanced gastric cancer. JAMA Network Open. (2021) 4(8):e2121143. doi: 10.1001/jamanetworkopen.2021.21143
29. Jiang Y, Wang H, Wu J, Chen C, Yuan Q, Huang W, et al. Noninvasive imaging evaluation of tumor immune microenvironment to predict outcomes in gastric cancer. Ann Oncol. (2020) 31:760–8. doi: 10.1016/j.annonc.2020.03.295
30. Ji T, Zhou F, Wang J, Zi L. Risk factors for lymph node metastasis of early gastric cancers in patients younger than 40. Med (Baltimore). (2017) 96:e7874. doi: 10.1097/MD.0000000000007874
31. Takatsu Y, Hiki N, Nunobe S, Ohashi M, Honda M, Yamaguchi T, et al. Clinicopathological features of gastric cancer in young patients. Gastric Cancer. (2016) 19:472–8. doi: 10.1007/s10120-015-0484-1
32. Qian S, Golubnitschaja O, Zhan X. Chronic inflammation: key player and biomarker-set to predict and prevent cancer development and progression based on individualized patient profiles. Epma J. (2019) 10:365–81. doi: 10.1007/s13167-019-00194-x
33. Moses K, Brandau S. Human neutrophils: Their role in cancer and relation to myeloid-derived suppressor cells. Semin Immunol. (2016) 28:187–96. doi: 10.1016/j.smim.2016.03.018
34. Coupland LA, Parish CR. Platelets, selectins, and the control of tumor metastasis. Semin Oncol. (2014) 41:422–34. doi: 10.1053/j.seminoncol.2014.04.003
35. Ray-Coquard I, Cropet C, Van Glabbeke M, Sebban C, Le Cesne A, Judson I, et al. Lymphopenia as a prognostic factor for overall survival in advanced carcinomas, sarcomas, and lymphomas. Cancer Res. (2009) 69:5383–91. doi: 10.1158/0008-5472.CAN-08-3845
36. Abu-Shawer O, Abu-Shawer M, Haimour A, Alhouri A, Alkhatib AA, Abki M, et al. Hematologic markers of distant metastases in gastric cancer. J Gastrointest Oncol. (2019) 10:529–36. doi: 10.21037/jgo.2019.01.12
37. Nonato LG, Do Carmo FP, Silva CT. GLoG: laplacian of gaussian for spatial pattern detection in spatio-temporal data. IEEE Trans Vis Comput Graph. (2021) 27:3481–92. doi: 10.1109/TVCG.2020.2978847
Keywords: gastric cancer, distant metastases, radiomics, nomogram, computed tomography
Citation: Xue H-B, Liang M-L, Xu H-Z, Wang C-Y, Xu T-W and Zhao A-Y (2024) Development and validation of an individualized nomogram for predicting distant metastases in gastric cancer using a CT radiomics-clinical model. Front. Oncol. 14:1476340. doi: 10.3389/fonc.2024.1476340
Received: 05 August 2024; Accepted: 12 November 2024;
Published: 29 November 2024.
Edited by:
Wenle Li, Xiamen University, ChinaReviewed by:
Junmeng Li, Henan Provincial People’s Hospital, ChinaQingwen Zeng, The First Affiliated Hospital of Nanchang University, China
Copyright © 2024 Xue, Liang, Xu, Wang, Xu and Zhao. This is an open-access article distributed under the terms of the Creative Commons Attribution License (CC BY). The use, distribution or reproduction in other forums is permitted, provided the original author(s) and the copyright owner(s) are credited and that the original publication in this journal is cited, in accordance with accepted academic practice. No use, distribution or reproduction is permitted which does not comply with these terms.
*Correspondence: Tian-Wen Xu, eHV0aWFud2VuNTNAMTYzLmNvbQ==; Ai-Yue Zhao, emhhb2FpeXVlLTAwMTFAZmptdS5lZHUuY24=
†These authors have contributed equally to this work and share first authorship