- 1Department of Radiology, Nanxishan Hospital of Guangxi Zhuang Autonomous Region, Guilin, China
- 2Graduate School, Guilin Medical University, Guilin, China
- 3Philips (China) Investment Co., Ltd., Guangzhou Branch, Guangzhou, China
- 4Department of Radiology, Affiliated Hospital of Youjiang Medical University for Nationalities, Baise, China
- 5Life Science and clinical Medicine Research Center, Affiliated Hospital of Youjiang Medical University for Nationalities, Baise, China
Background: In recent years, studies have confirmed the predictive capability of spectral computer tomography (CT) in determining microvascular invasion (MVI) in patients with hepatocellular carcinoma (HCC). Discrepancies in the predicted MVI values between conventional CT imaging features and spectral CT parameters necessitate additional validation.
Methods: In this retrospective study, 105 cases of small HCC were reviewed, and participants were divided into MVI-negative (n=53, Male:48 (90.57%); mean age, 59.40 ± 11.79 years) and MVI-positive (n=52, Male:50(96.15%); mean age, 58.74 ± 9.21 years) groups using pathological results. Imaging features and iodine density (ID) obtained from three-phase enhancement spectral CT scans were gathered from all participants. The study evaluated differences in imaging features and ID values of HCC between two groups, assessing their diagnostic accuracy in predicting MVI occurrence in HCC patients. Furthermore, the diagnostic efficacy of imaging features and ID in predicting MVI was compared.
Results: Significant differences were noted in the presence of mosaic architecture, nodule-in-nodule architecture, and corona enhancement between the groups, all with p-values < 0.001. There were also notable disparities in IDs between the two groups across the arterial phase, portal phase, and delayed phases, all with p-values < 0.001. The imaging features of nodule-in-nodule architecture, corona enhancement, and nonsmooth tumor margin demonstrate significant diagnostic efficacy, with area under the curve (AUC) of 0.761, 0.742, and 0.752, respectively. In spectral CT analysis, the arterial, portal, and delayed phase IDs exhibit remarkable diagnostic accuracy in detecting MVI, with AUCs of 0.821, 0.832, and 0.802, respectively. Furthermore, the combined models of imaging features, ID, and imaging features with ID reveal substantial predictive capabilities, with AUCs of 0.846, 0.872, and 0.904, respectively. DeLong test results indicated no statistically significant differences between imaging features and IDs.
Conclusions: Substantial differences were noted in imaging features and ID between the MVI-negative and MVI-positive groups in this study. The ID and imaging features exhibited a robust diagnostic precision in predicting MVI. Additionally, our results suggest that both imaging features and ID showed similar predictive efficacy for MVI.
Introduction
The landscape of hepatocellular carcinoma (HCC) epidemiology is evolving with the surge in alcohol consumption, the escalating rates of obesity, and advancements in the treatment of hepatitis B virus and hepatitis C virus (1). Nevertheless, the implications of these shifts on the worldwide burden of HCC are not yet fully understood (1). HCC exhibits considerable heterogeneity and a grim prognosis, positioning it as the third most prevalent cause of cancer-related mortality globally (2). Surgical resection and liver transplantation remain the most effective treatments; nevertheless, recurrence stands out as a significant factor impacting the long-term survival of patients post-surgery (3).Tumor microvascular invasion (MVI) is a pivotal factor contributing to recurrence and poor prognosis post-HCC surgery, significantly affecting patient outcomes. For instance, in HCC patients with a tumor diameter greater than 2 cm and complicated by MVI, simply increasing the resection margin is insufficient to enhance overall survival rates (4). Despite efforts to widen the resection margin, long-term prognosis remains bleak, necessitating the implementation of additional comprehensive treatments to combat tumor recurrence and metastasis (4, 5). Therefore, the crucial assessment for MVI before cancer resection surgery profoundly influences the surgical approach, prognosis, and postoperative management. Consequently, the accurate evaluation of MVI in patients with primary small HCC has increasingly captured attention (6).
MVI is currently defined as a pathological condition distinguished by the presence of tumor cell nests along the walls of vascular structures, including endothelial cells within the portal and hepatic venous systems (7). To date, the conventional approach for preoperatively diagnosing HCC with MVI is through a pathological needle biopsy. However, needle biopsy presents several drawbacks, including invasiveness, a high risk of metastasis, a significant rate of false negatives, and challenges in acquiring adequate material, rendering it scarcely utilized in clinical settings (8). In recent years, numerous investigations have explored the association between laboratory markers, imaging features, and other parameters with MVI, yielding promising outcomes (9). Tumor imaging features, such as include non-smooth tumor margins, peritumoral enhancement and corona enhancement, have been extensively researched and utilized for predictive MVI due to their ability to reflect aspects of the tumor’s aggressive biological behavior (10). However, preoperative MVI diagnosis is challenging, and the imaging findings and definition are subjective and highly readerdependent. In recent years, studies have confirmed the predictive capability of spectral CT in determining MVI in patients with HCC (11–14). A study believed that conventional CT imaging features and spectral CT quantitative parameters exhibited almost identical predictive capabilities in anticipating MVI (11). Another study advocated that spectral CT offered more quantitative parameters compared to conventional CT, enhancing the differentiation between small HCC with and without MVI (12). Discrepancies in the predicted MVI values between conventional CT imaging features and spectral CT parameters necessitate additional validation.
This study aim to compare the diagnostic efficacy of imaging features and iodine density (ID) value, a parameter derived from spectral CT, for predicting MVI in HCC. In this study, 105 cases of small HCC were retrospectively reviewed, and subjects were categorized into MVI-negative and MVI-positive groups based on pathological findings. Imaging features and ID derived from three phase enhancement spectral CT scans were collected from all subjects. In this study, we assessed the discrepancies in imaging features and ID values of HCC between groups with and without MVI. We also explored the diagnostic accuracy of these imaging features and ID values in predicting the occurrence of MVI in HCC patients. Additionally, the diagnostic efficacy of imaging features and ID for predicting MVI were compared.
Methods
This is a retrospective study conducted at Nanxishan Hospital of Guangxi Zhuang Autonomous Region. Between June 2020 and July 2023, a total of 105 cases of HCC were collected, confirmed through pathological examination with surgical specimens in a consecutive manner. All patients had undergone spectral CT examination within 1 week before surgery. The inclusion and exclusion criteria for this study were detailed in Figure 1. The study was conducted in accordance with the Declaration of Helsinki (as revised in 2013). This study has been approved by the Ethics Committee of Nanxi Mountain Hospital, Guangxi Zhuang Autonomous Region [NO.2020 (KY-E-37)]. Due to its retrospective study, the requirement for informed consent is waived.
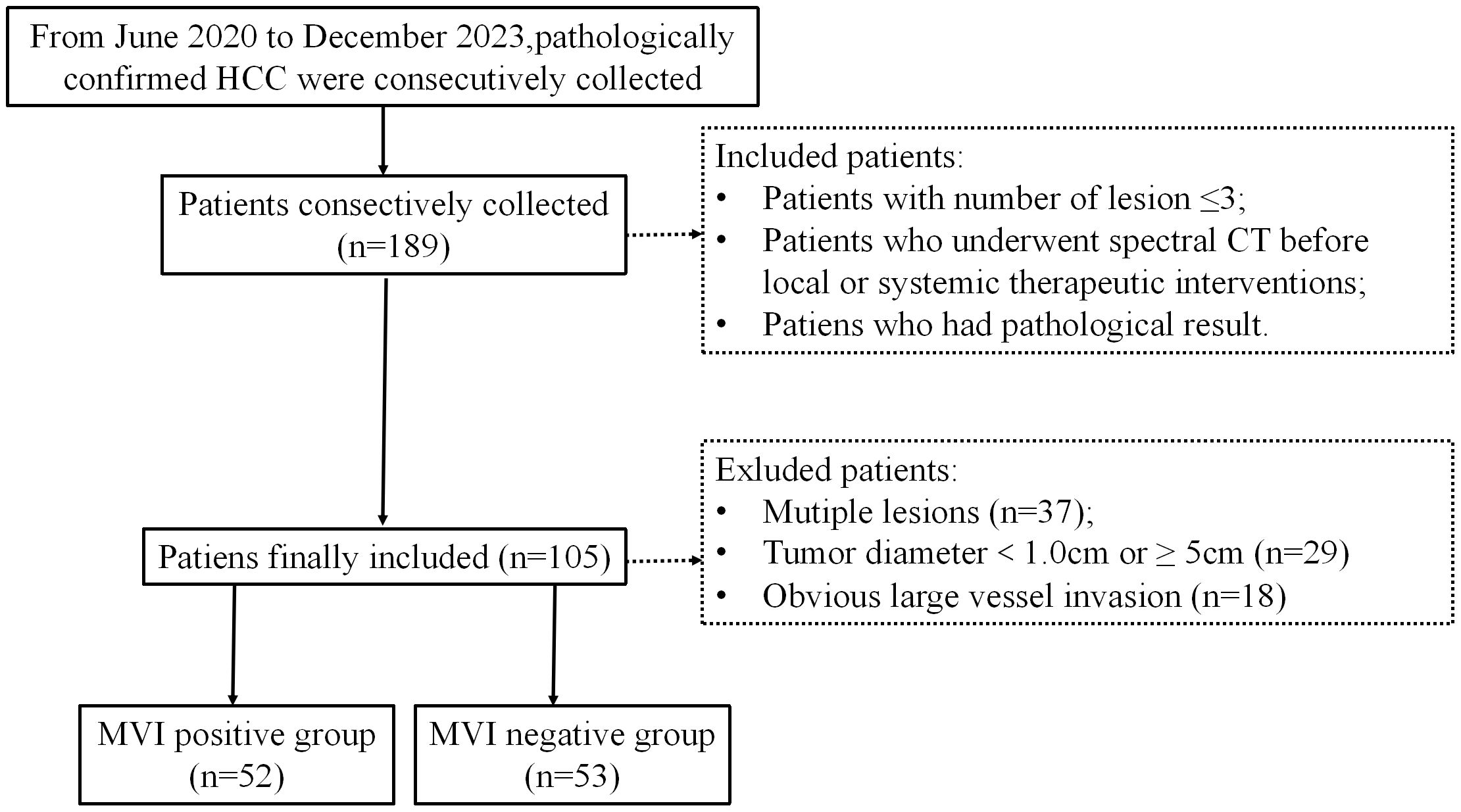
Figure 1. Flowchart shows inclusion and exclusion process of the subjects. HCC, hepatocellular cancer; MVI, microvascular invasion.
Protocol of CT examination
CT scans were performed at a spectral CT scanner (IQon, Philips Healthcare, Amsterdam, The Netherlands). All scans included conventional CT, and contrast-enhanced scans (including arterial, portal and delayed phase). All patients drink about 250ml water before the inspection. The contrast agent (320mg/ml, 70 mL, iopromide; Beijing Beilu Pharmaceutical Co., Ltd., Beijing, China) was administered via an antecubital vein at an intended flow rate of 2.0~3.0ml/s. Scans were performed using the bolus chase method. The region of interest (ROI) was located at the same level of descending aorta. The arterial phase scan was performed after a 6S delay when the CT value exceeded the threshold of 180HU. A portal phase scan was performed after the arterial phase at the 33S. Delayed phase scanning was performed 101s after the arterial period. The same scanning protocol was repeated once during follow-up after TACE treatment in HCC patients. Protocols and parameters of the CT examination were shown as follows. Various phases of the CT liver examination were conducted using the following parameters. Common technical settings across all phases included a pitch of 1.234, rotation time of 0.27s, collimation size of 64×0.625, a field of view (FOV) of 345mm×345mm, a matrix size of 512×512, reconstruction slice and interval of 1mm, and employed the iDose4 level 4 reconstruction mode. Spectral parameters specific to Arterial, Portal and Delayed phases utilized Spectral-Based Imaging (SBI) spectral parameters in analysis.
Imaging features analysis
Preoperative images were obtained from a picture archiving and communication system and independently reviewed by two faculty abdominal radiologists with over 10 (JL) and 5 years (RM) of experience. In cases of inter-reviewer discrepancies, a third observer (QX, with 15 years of experience) facilitated consensus. Though informed of the HCC diagnosis, all three readers remained blinded to clinical history and the pathological assessment of MVI. Following the principles of major and ancillary LI-RADS categorization, the identification of HCC high-risk patients involved assessing specific imaging features (15), including (a) lesion major diameter, (b) nonrim arterial phase hyperenhancement (nonrim APHE), (c) nonperipheral “washout,” (d) enhancing capsule, (e) mosaic architecture, (f) nodule-in-nodule architecture, (h) corona enhancement, and two non-LIRADS features: tumor nonsmooth tumor margin and intratumor necrosis (11, 16, 17). A detailed summary of CT imaging features is provided in Table 1.
ID analysis
Two radiologists (JL and XQ, with over 10 and 15 years of experience in abdominal CT diagnosis, respectively), blinded to patients’ clinical data and pathological results, processed and measured all spectral CT images using the Philips Spectral Diagnostic Suite 9.0 workstation (Philips Healthcare). Three consecutive image slices, encompassing the maximum cross-section of the tumor and its upper and lower layers, were selected for region of interest (ROI) delineation and parameter measurement (Figure 2). The ROIs were drawn as large as possible to cover the entire cross-section of the tumor. Initially, the ROI was outlined on the arterial phase image of the spectral CT, and then it was transferred to the portal and delayed phase images of the spectral CT. ID values were automatically generated. Care was taken to place the ROI on solid tumor regions, avoiding areas with vessels, calcification, or cystic/necrotic changes.
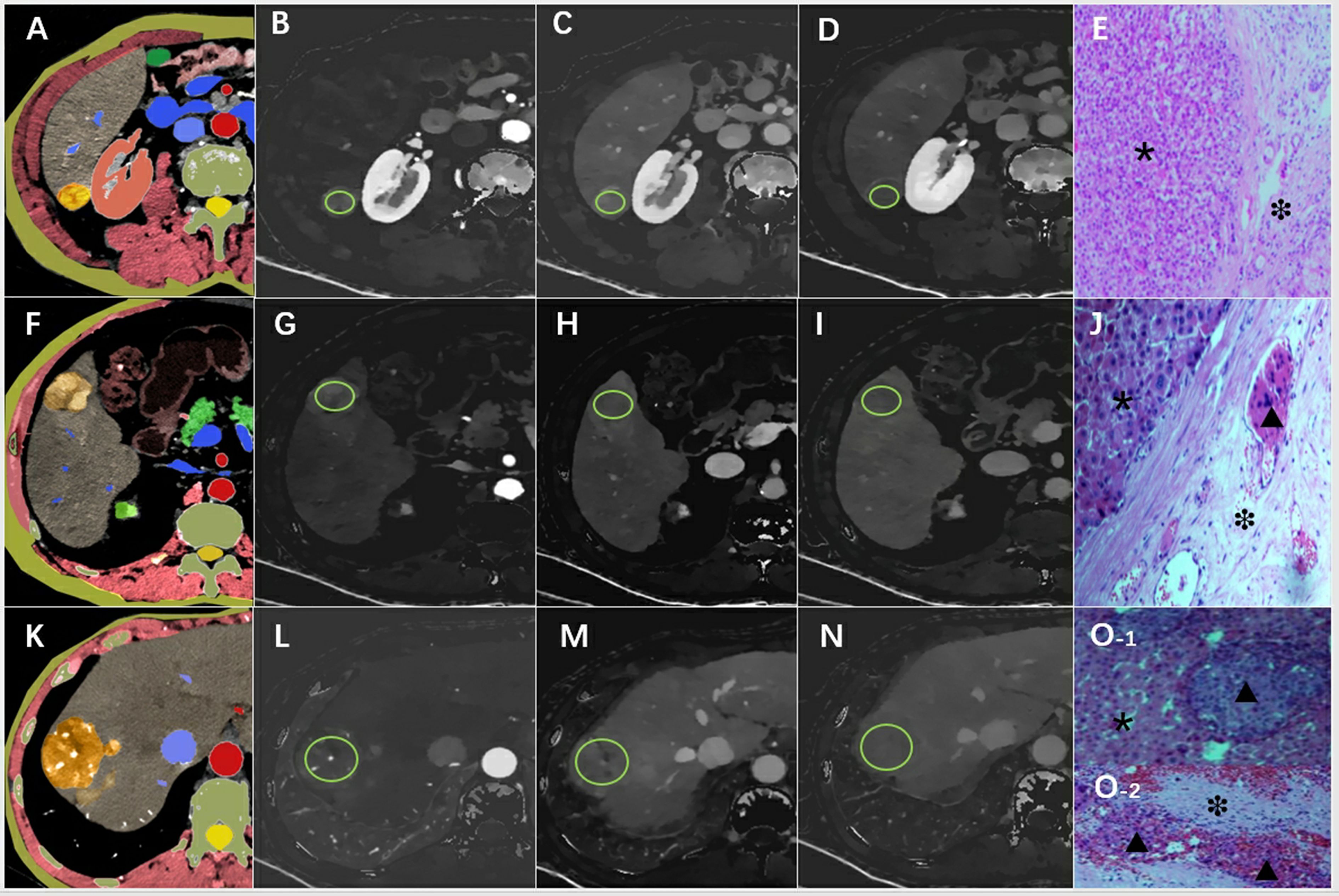
Figure 2. An example of the definition of the regions of interest. Examples of the definition of the regions of interest (ROIs) (light green circles) for quantitative analyses. (A) Schematic diagram of hepatocellular carcinoma with smooth tumor margin, (B) ID-A=1.02 mg/ml, (C) ID-P=1.23 mg/ml, (D) ID-D=1.05 mg/ml, (E) pathological pictures (×200), the tumor area (*) and MVI-negative in the normal area (❉) around the tumor were marked. (F) Schematic diagram of hepatocellular carcinoma with nodule-in-nodule architecture, (G) ID-A=1.87 mg/ml, (H) ID-P=1.58 mg/ml, (I) ID-D=1.32 mg/ml, (J) pathological pictures (×200) showed the tumor area (*) and MVI-positive(▴) in the normal area(❉) around the tumor. (K) Schematic diagram of hepatocellular carcinoma with corona enhancement, (L) ID-A=1.64 mg/ml, (M) ID-P=1.43 mg/ml, (N) ID-D=1.02 mg/ml, (O) pathological pictures (×200), O-1 shoued MVI-positive (▴) in the tumor (*), and also combined with MVI-positive (▴) in normal area (❉) in the O-2. ID-A, iodine density in hepatic arterial phase; ID-P, iodine density in portal-venous phase; ID-D, iodine density in delayed phase.
MVI examination
All surgical specimens were examined by one pathologist (L.Z., with 18 years of experience) who was blinded to the clinical and imaging data. A tissue sample containing the suspected tumor and surrounding tissue is acquired via biopsy or surgical resection and then fixed in formalin to maintain its structural integrity. Subsequently, the fixed tissue is dehydrated, embedded in paraffin, and sectioned thinly for microscopic examination. Following staining with hematoxylin and eosin (H&E) to visualize cellular details, all specimen sections are reviewed by a single experienced pathologist blinded to imaging and clinical data. Microscopic examination is conducted to detect any signs of microvascular invasion, characterized by tumor cells infiltrating small blood vessels or lymphatic channels near the tumor (Figure 2). The pathologist documents the presence, extent, and specifics of MVI in the pathology report and integrates these findings with other histopathological features to offer an accurate diagnosis and prognosis (18). Stringent quality control procedures, including potential review by multiple pathologists, are implemented to ensure the precision and reliability of the pathological assessments. The pathological assessment and diagnostic criteria for MVI in this study align with the Chinese Guidelines for the Diagnosis and Treatment of Hepatocellular Carcinoma (18).
Statistical analysis
Statistical analyses were conducted using SPSS 26.0 (IBM Corp., Armonk, NY, USA). A bilateral p-value of less than 0.05 was considered indicative of statistical significance. The intraclass coefficient coefficient (ICC) test was utilized to assess the consistency of the ROI measurements made by both radiologists. Intraobserver agreements of imaging features were evaluated using Cohen κ-statistics. The strength of agreement via ICC and κ values < 0.4, 0.4–0.6, 0.6–0.8, and > 0.8 were categorized poor, moderate, good, and excellent agreement, respectively (13). The means of the variables measured by the two radiologists were utilized for statistical analysis. Normality and homogeneity of variance were tested using the Shapiro-Wilk test. Count data were presented as cases, and differences between groups were assessed using the chi-square test. Measurement data with a normal distribution were expressed as mean ± standard deviation and were compared between groups using an independent samples t-test, with post-hoc tests corrected using Bonferroni adjustment. Binary logistic regression analysis was employed to investigate the association between baseline spectral CT parameters and the presence of MVI. Initially, variables showing statistical associations were identified through binary logistic regression. Subsequently, variables with a p-value less than 0.05 were included in the multivariable logistic regression model to determine combined predictive parameters. Three prediction models were developed based on these combined parameters: Model I using imaging features, Model II based on spectral CT variables (including ID values on arterial, portal, and delayed phase images), and Model III incorporating both imaging features and spectral CT parameters. The diagnostic performance of spectral parameters and the three combined models was assessed using the receiver operating characteristic curve (ROC). Area under the curve (AUC) values, cutoff values, sensitivity, and specificity were calculated based on the optimal Youden index. Additionally, the DeLong test was employed to compare the AUC differences among the variables and models.
Results
Patient characteristics
Finally, a total of 105 patients with HCC were included in this study. Based on the presence or absence of MVI in the pathological results, all subjects were categorized into either the MVI-positive or MVI-negative group. The MVI-positive group comprised 53 subjects with a mean age of 59.40 ± 11.79 years and was predominantly male (50 individuals, 96.15%). In contrast, the MVI-negative group consisted of 52 subjects with a mean age of 58.74 ± 9.21 years and was also predominately male (48 individuals, 90.57%). There was no statistical difference in gender or age between the two groups. The baseline characteristics of patients are listed in Table 1.
Reliability of measurements
The measurements obtained by both observers exhibited high consistency (P>0.05). The ICC values for the ID in the arterial, portal, and delayed phases were determined to be 0.803, 0.891, and 0.817, respectively. In imaging features, interobserver agreements were good to excellent, respectively (κ values range from 0.674 to 0.817).
Comparison of variables between two groups
A detailed comparison of the imaging features between the two groups is provided in Table 1 and Figure 3. The CT imaging features included the major diameter in centimeters with a mean ± SD of 5.94 ± 4.59 and 8.70 ± 4.22 for the MVI-negative and MVI-positive groups, respectively. The difference was statistically significant (p = 0.002). Other features such as nonrim APHE, nonperipheral washout, and enhancing capsule showed varying percentages without significant differences. Notable differences were observed in the presence of mosaic architecture, nodule-in-nodule architecture, corona enhancement, nonsmooth tumor margin, and intratumor necrosis between the groups, all with p-values < 0.001. However, conventional CT values in the arterial phase, portal venous phase, and delayed phase did not exhibit significant differences between the groups.
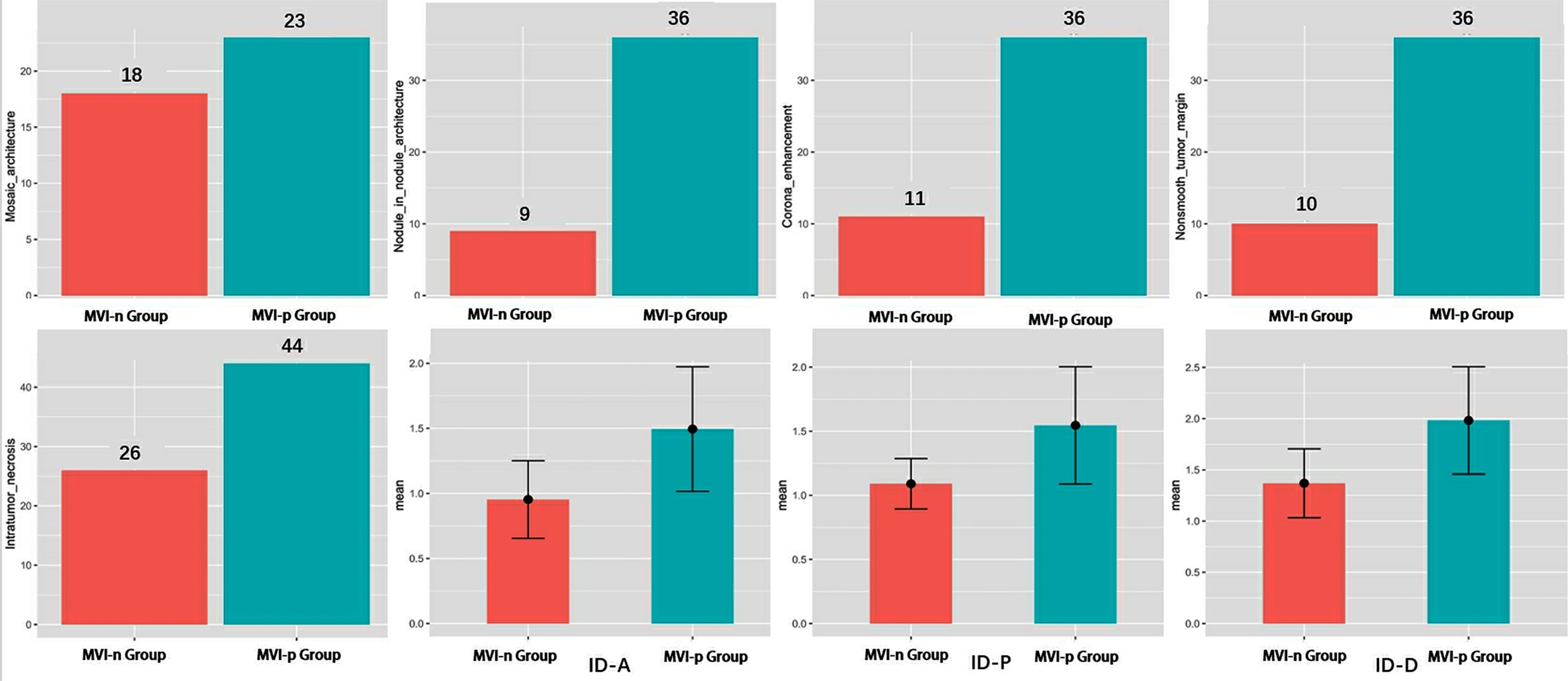
Figure 3. Analysis of differences in variables between two groups. MVI, microvascular invasion; ID-A, iodine density in hepatic arterial phase; ID-P, iodine density in portal-venous phase; ID-D, iodine density in delayed phase.
There was a significant difference in IDs between the two groups across the arterial phase, portal phase, and delayed phases (Table 1 and Figure 3). The mean values of arterial phase ID in MVI-negative group (0.95 ± 0.30 mg/ml3) was significantly lower than that of MVI-positive group (1.49 ± 0.48 mg/ml3) (p<0.001). Similarly, the mean values of portal phase ID in MVI-negative group (1.37 ± 0.34 mg/ml3) was significantly lower than that of MVI-positive group (1.98 ± 0.52 mg/ml3) (p<0.001). Furthermore, the mean values of delayed phase ID in MVI-negative group (1.09 ± 0.20 mg/ml3) was significantly lower than that of MVI-positive group (1.55 ± 0.46 mg/ml3) (p=<0.001).
The association between variables and MVI in HCC patients
Among the imaging features analyzed, nodule-in-nodule architecture, non-smooth tumor margin and corona enhancement demonstrated significant associations with the evaluation of MVI (see Table 2 and Figure 4). The regression coefficient for nodule-in-nodule architecture was 1.434 (p=0.013), with an odds ratio (OR) of 4.197 (95% CI: 1.347-13.081) In contrast, the regression coefficient for corona enhancement was 1.235 (p=0.024), with an OR of 3.438 (95% CI: 1.180-10.021). Conversely, non-smooth tumor margin exhibited a regression coefficient of 1.156 (p=0.042), with an OR of 3.176 (95% CI: 1.045-9.652). Notably, other imaging features such as major diameter, mosaic architecture, and intratumor necrosis did not show a significant association with MVI.
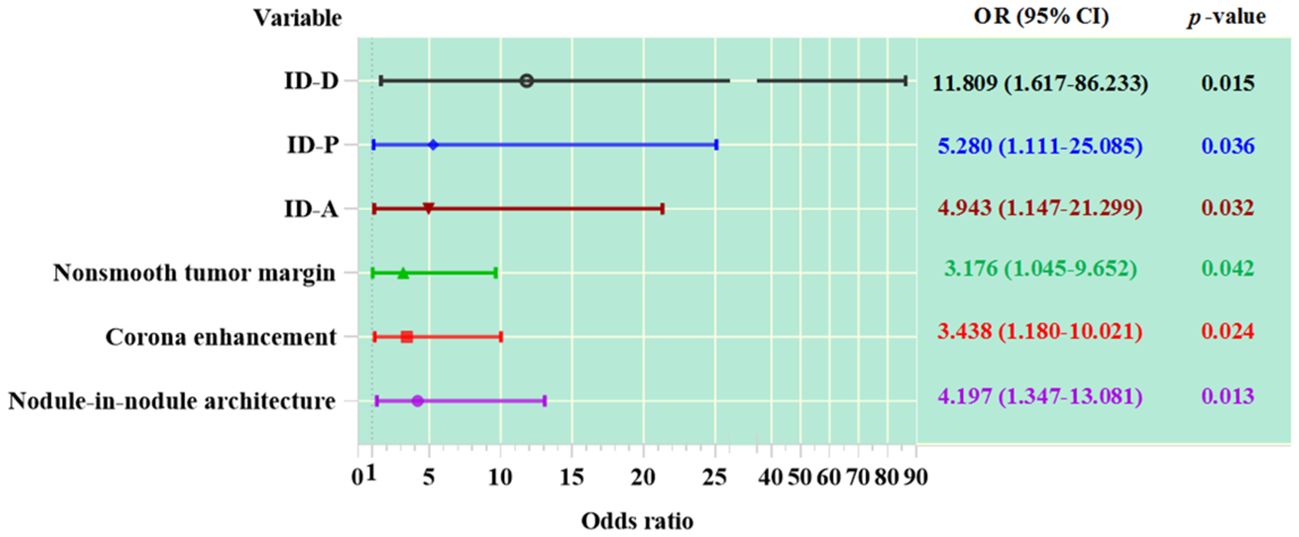
Figure 4. The association between variables and MVI in HCC patients. ID-A, iodine density in hepatic arterial phase; ID-P, iodine density in portal-venous phase; ID-D, iodine density in delayed phase.
In patients with HCC, the values of ID in the arterial phase, portal phase, and delayed phase are significantly correlated with MVI (see Table 2 and Figure 4). The regression coefficient for arterial phase ID was 1.598 (p=0.032), with an OR of 4.943 (95% CI: 1.147-21.299). Similarly, the regression coefficient for portal phase ID was 1.664 (p=0.036), with an OR of 5.280 (95% CI: 1.111-25.085). The delayed phase ID had a regression coefficient of 2.469 (p=0.015).
The diagnostic efficacy of spectral CT parameters predicting MVI
Valuable insights from the analysis reveal that nodule-in-nodule architecture, corona enhancement, and nonsmooth tumor margin, as part of the general imaging features, exhibit significant diagnostic efficacy, boasting respective AUC of 0.761, 0.742, and 0.752. These imaging feature variables demonstrate notable sensitivity and specificity levels alongside significant p-values. Specifically, the diagnostic sensitivity for nodule-in-nodule architecture, corona enhancement, and nonsmooth tumor margin was 0.692, 0.692, and 0.692, respectively, while their diagnostic specificity values were 0.830, 0.792, and 0.811 correspondingly.
In spectral CT analysis, the identifiers for arterial, portal, and delayed phase ID demonstrate significant diagnostic accuracy in detecting MVI. The arterial phase ID, at a cut-off value of 0.599 mg/ml3, yielded an AUC of 0.821 (95% CI: 0.734-0.907) with sensitivity and specificity values of 76.9% and 83.0%, respectively. Similarly, the portal phase ID, at a cut-off value of 0.616 mg/ml3, achieved an AUC of 0.832 (95% CI: 0.751-0.913) with sensitivity and specificity rates of 67.3% and 94.3%. Moreover, the delayed phase imaging contrast, with a cut-off value of 0.600 mg/ml3, demonstrated an AUC of 0.802 (95% CI: 0.713-0.891) coupled with sensitivity and specificity values of 78.8% and 81.1% respectively (Table 3 and Figure 5).
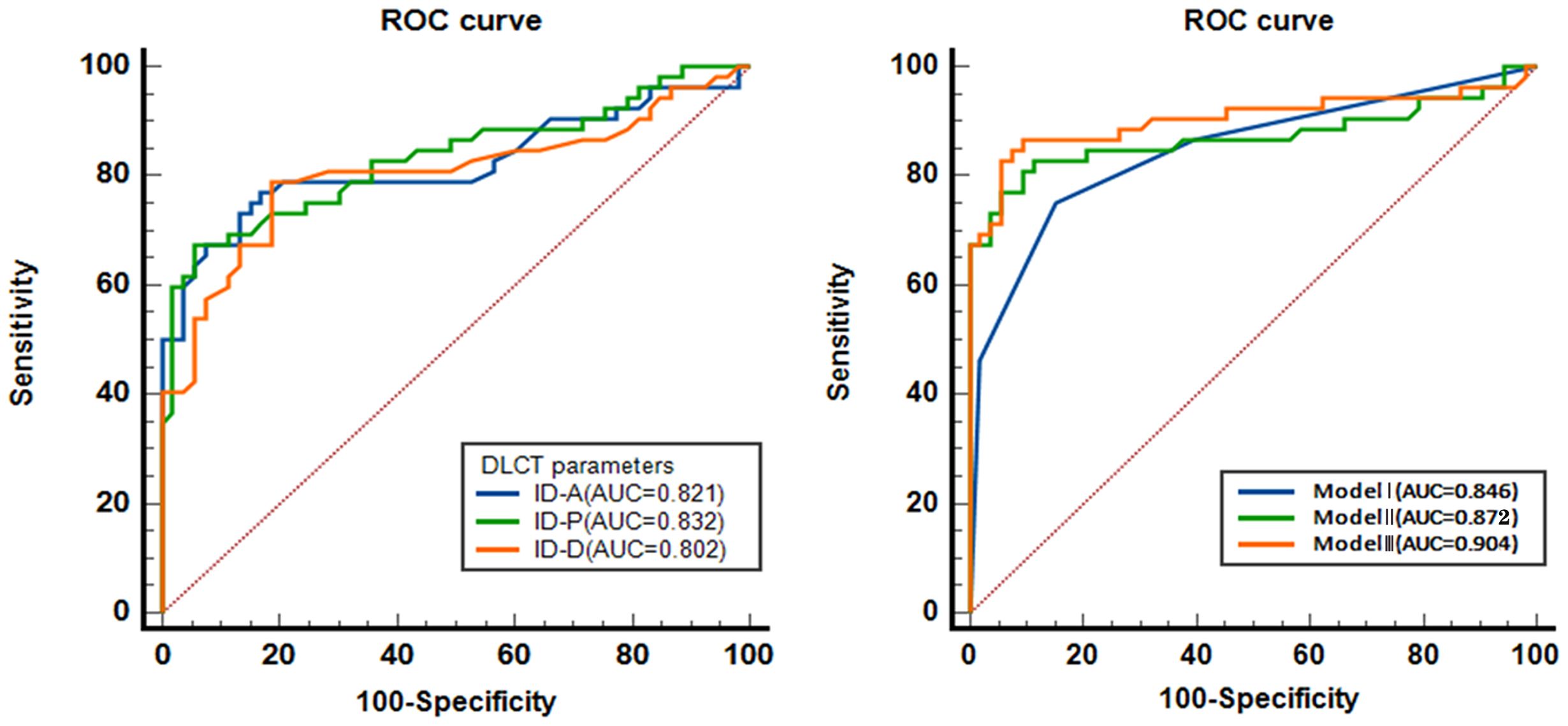
Figure 5. The diagnostic efficacy of spectral CT parameters predicting MVI. ROC, receiver operating characteristic curve. AUC, area under the curve; ID-A, iodine density in hepatic arterial phase; ID-P, iodine density in portal-venous phase; ID-D, iodine density in delayed phase. Model I, combination of the variables of General CT characteristics; Model II, combination of the variables of ID; Model III, combination of Model I and Model II.
Moreover, the combined models, including Model I, Model II, and Model III, show excellent AUC values, strong sensitivity and specificity, and significant predictive capabilities. The AUC of combined model I was 0.846 (95% CI: 0.769 ~ 0.923), with a sensitivity and specificity of 75.0% and 84.9%, respectively. The AUC of combined model II was 0.872 (95% CI: 0.795 ~ 0.949), with a sensitivity and specificity of 82.7% and 88.7%, respectively. The AUC of combined model III was 0.904 (95% CI: 0.836 ~ 0.973), with a sensitivity and specificity of 86.5% and 92.5%, respectively (Table 3 and Figure 5).
DeLong test between AUCs
A comparison of the DeLong test among the AUCs was outlined in Table 4. The DeLong test results revealed statistically significant differences between nodule-in-nodule architecture and Model I (p=0.0061), corona enhancement and Model I (p=0.0019), nonsmooth tumor margin and Model I (p=0.0029), as well as between Model III and Model I (p=0.0459).
Discussion
In this study, we found that both ID values and imaging features of HCC exhibited a robust diagnostic accuracy in predicting MVI. Interestingly, no significant disparity was observed in the diagnostic efficacy of ID values and imaging features for predicting MVI.
Imaging features in MVI prediction
In the present study, the incidence of imaging features such as nodule-in-nodule architecture, corona enhancement, and nonsmooth tumor margin was lower in the MVI-negative group than the MVI-positive group. These imaging characteristics mirror, to some extent, the molecular biological characteristics and aggressiveness of liver cancer. Corona enhancement, indicative of the tumor’s elevated blood flow perfusion, is commonly associated with increased arterial input and venous output surrounding the tumor (19). The existence of nodule-in-nodule structures offers compelling evidence of the multistep progression in hepatocarcinogenesis, and indicating a transformation in HCC biology (20). The imaging feature of nonsmooth tumor margin can be regarded as indicative of HCC’s aggressive biological tendencies to invade the tumor capsule and protrude into the non-tumoral parenchyma (21). These imaging feature probably relates to the known hypothesis of tumor aggressive characteristics that aid in identifying MVI. Previous studies have established a positive correlation between corona enhancement and MVI. Lee and colleagues demonstrated that corona enhancement serves as an imaging biomarker for predicting the occurrence of MVI (22). Yang et al. reported that, as the preoperative biomarkers for predicting MVI, corona enhancement demonstrates the highest accuracy, surpassing both internal arteries and tumor size (23. A non-smooth tumor margin is a promising indicator of preoperative MVI assessment. Li et al. reported the non-smooth tumor margin and tumor size were significantly associated with MVI (24). Jiang et al. discovered that a non-smooth tumor margin exhibits a stronger association with MVI when compared to other imaging features, including internal artery, hepatobiliary phase peritumoral hypointensity, and tumor multifocality (25). In a meta-analysis of non-smooth tumor margin predicting MVI, the pooled AUC, sensitivity and specificity were 0.79, 35% and 79%, respectively (21). A nodule-in-nodule appearance in early-stage HCC could be interpreted as a morphologic marker of dedifferentiation of early HCC (26). However, this imaging feature is controversial when link it to MVI. Sheng et al. found that the imaging feature of nodule-in-nodule architecture showed a significant association with MVI, with a magnitude of association comparable to that of the internal artery (27). The study by Wang et al. found that there is no significant correlation between the imaging feature of nodule-in-nodule and MVI (28). Moreover, another study reported that absence of nodule-in-nodule architecture were independent predictors of MVI, and the association with MVI was significantly higher than tumor size (29). Although the diagnostic efficacy of imaging features in predicting MVI is well-established, concerns persist regarding the predictability and repeatability of these features. Moreover, there is still controversy surrounding their positive predictive values (30). Several factors may contribute to the inconsistency. Firstly, the efficacy of these imaging features in predicting MVI can vary across different studies due to factors such as sample sizes and patient demographics (31). Secondly, precise identification and interpretation of imaging features are essential for ensuring repeatability since image interpretation hinges on both the quality of the device and the proficiency of the operator. For instance, corona enhancement may be mistaken for a peritumoral capsule due to its appearance, and likewise, mosaic architecture could be misconstrued as necrosis (32). A sensitivity of only 54% is observed in imaging diagnosis of non-smooth tumor margins compared to pathological diagnosis (33). In future study, a third radiologist was consulted to obtain consensus evaluation may reduce the probability of imaging feature misinterpretation.
Spectral CT metrics in MVI prediction
In this study, we found the disparity of ID values between groups and validated that ID values have a strong diagnostic accuracy in predicting MVI. Spectral CT parameters have been validated as a surrogate method for assessing perfusion abnormalities in organs, facilitating the evaluation of tumor grading, pathological classification, and invasiveness by detecting changes in tumor perfusion (34–37). ID plays a pivotal role in predicting the aggressiveness and tumor characteristics of HCC. This metric provides valuable information about vascular permeability, tumor perfusion, and tissue iodine uptake, offering insights into the underlying pathophysiological mechanisms driving tumor behavior. Lewin et al. discovered that the spectral CT parameters model successfully predicted intratumoral and peritumoral MVI in HCC (14). Mulé et al. reported that in advanced HCC lesions, late-arterial ID is strongly related to both blood flow and blood volume, while portal ID mainly reflects blood volume, offering ID the ability to evaluate both morphological and perfusion changes (38). Zhu et al. employed spectral CT parameters to predict MVI in solitary AFP-negative HCC measuring ≤ 5 cm, achieving an AUC of 0.755 for arterial ID and 0.683 for delayed ID (11). In another study, Yang et al. employed spectral CT for evaluating MVI in small HCC ≤ 3 cm, reporting an AUC of 0.853, a sensitivity of 87.50%, and a specificity of 79.60% (12). Kim et al. evaluated the diagnostic performance of normalized intratumoral and peritumoral IDs in predicting MVI. Their findings revealed that the ID in outer layer 1 demonstrated the highest AUC of 0.747 (13). Our findings are in line with the above conclusions, demonstrating the consistent stability and repeatability of spectral CT parameters in predicting MVI.
Comparison of imaging features and spectral CT parameters in predicting MVI
Another result of this study revealed no significant difference in the diagnostic efficacy between ID values and imaging features for predicting MVI in HCC. Moreover, the incorporation of both protocols did not improve the predictive accuracy of spectral CT. Zhu et al. observed that conventional CT imaging features and spectral CT quantitative parameters demonstrated nearly identical predictive abilities in predicting MVI, with AUCs of 0.848 and 0.849, respectively. Yet, the combination of imaging features and spectral CT parameters notably enhanced the diagnostic efficacy (11). In a study by Yang et al., spectral CT was found to offer more quantitative parameters compared to conventional CT, enhancing the differentiation between small HCC with and without MVI (12). Imaging feature has also been confirmed to improve the diagnostic efficacy of imaging omics in predicting MVI (23). The results of the aforementioned studies do not align with our findings. Further validation is needed to evaluate the supplementary predictive benefit of spectral CT parameters over conventional CT imaging features. In addition to its recognized superior predictive capability for MVI, spectral CT is acknowledged to provide further advantages in MVI prognostication. These benefits encompass heightened lesion detection, enhanced lesion characterization, improved imaging features interpretation, inherently robust imaging protocols for pathological explication, and reduced radiation exposure (39).
Limitation
This study had several potential limitations. Firstly, this was a single center, single scanner, and single vendor retrospective study, and the sample size of this study is insufficient, The results of this study need to be verified by more diversity of this study. Secondly, this study is a retrospective study, and the study efficacy may receive the effect of case selection. Thirdly, In this study, we only used ID to predict MVI. The first reason is that spectral parameters are derived by the same decomposition model, other parameters does not provide additional lesion information rather than the ID (34). The second reason is that the risk of multicollinearity may increase using multiple spectral parameters derived from the same set of images in the same statistical model. Finally, in addition to imaging features and spectral CT, various protocols such as radiomics models, quantitative parameters of magnetic resonance imaging, and molecular imaging have shown promising predictive abilities for MVI (40–42). This study did not include a comparison with these models. Conducting further comparisons among different imaging schemes to predict MVI will be beneficial for clinicians in making informed decisions.
Conclusion
Substantial differences were noted in imaging features and ID between the MVI-negative and MVI-positive groups in this study. The ID and imaging features exhibited a robust diagnostic precision in predicting MVI. Additionally, our results suggest that both imaging features and ID showed similar predictive efficacy for MVI. Additional validation is essential to ascertain the differences in predictive accuracy between imaging characteristics and spectral CT for MVI.
Data availability statement
The raw data supporting the conclusions of this article will be made available by the authors, without undue reservation.
Ethics statement
The studies involving humans were approved by the Ethics Committee of Nanxi Mountain Hospital, Guangxi Zhuang Autonomous Region. The studies were conducted in accordance with the local legislation and institutional requirements. The ethics committee/institutional review board waived the requirement of written informed consent for participation from the participants or the participants’ legal guardians/next of kin because Due to its retrospective study, the requirement for informed consent is waived.
Author contributions
XZ: Writing – original draft, Writing – review & editing. JL: Writing – original draft, Writing – review & editing. XL: Writing – original draft, Writing – review & editing. RM: Writing – original draft, Writing – review & editing. WZ: Writing – original draft, Writing – review & editing. PY: Writing – original draft, Writing – review & editing. BH: Writing – original draft, Writing – review & editing. FL: Writing – original draft, Writing – review & editing. XML: Writing – original draft, Writing – review & editing. ZS: Writing – original draft, Writing – review & editing. XQ: Writing – original draft, Writing – review & editing.
Funding
The author(s) declare that no financial support was received for the research, authorship, and/or publication of this article.
Acknowledgments
Special thanks are extended to Dr. Zhao Lei from the Department of Pathology at Nanxishan Hospital of Guangxi Zhuang Autonomous Region for providing valuable assistance in surgical specimen handling.
Conflict of interest
Authors XML and ZS are employed by Philips China Investment Co.
The remaining authors declare that the research was conducted in the absence of any commercial or financial relationships that could be construed as a potential conflict of interest.
Publisher’s note
All claims expressed in this article are solely those of the authors and do not necessarily represent those of their affiliated organizations, or those of the publisher, the editors and the reviewers. Any product that may be evaluated in this article, or claim that may be made by its manufacturer, is not guaranteed or endorsed by the publisher.
References
1. Huang DQ, Singal AG, Kono Y, Tan DJH, El-Serag HB, Loomba R. Changing global epidemiology of liver cancer from 2010 to 2019: NASH is the fastest growing cause of liver cancer. Cell Metab. (2022) 34:969–77. doi: 10.1016/j.cmet.2022.05.003
2. Sung H, Ferlay J, Siegel RL, Laversanne M, Soerjomataram I, Jemal A, et al. Global Cancer Statistics 2020: GLOBOCAN Estimates of Incidence and Mortality Worldwide for 36 Cancers in 185 Countries. CA Cancer J Clin. (2021) 71:209–49. doi: 10.3322/caac.21660
3. Dopazo C, Søreide K, Rangelova E, Mieog S, Carrion-Alvarez L, Diaz-Nieto R, et al. Hepatocellular carcinoma. Eur J Surg Oncol. (2024) 50:107313. doi: 10.1016/j.ejso.2023.107313
4. Jung DH, Hwang S, Lee YJ, Kim KH, Song GW, Ahn CS, et al. Small Hepatocellular Carcinoma With Low Tumor Marker Expression Benefits More From Anatomical Resection Than Tumors With Aggressive Biology. Ann Surg. (2019) 269:511–9. doi: 10.1097/SLA.0000000000002486
5. Wang L, Wang W, Yao X, Rong W, Wu F, Chen B, et al. Postoperative adjuvant radiotherapy is associated with improved survival in hepatocellular carcinoma with microvascular invasion. Oncotarget. (2017) 8:79971–81. doi: 10.18632/oncotarget.v8i45
6. Zhang ZH, Jiang C, Qiang ZY, Zhou YF, Ji J, Zeng Y, et al. Role of microvascular invasion in early recurrence of hepatocellular carcinoma after liver resection: A literature review. Asian J Surg. (2024) 47:2138–43. doi: 10.1016/j.asjsur.2024.02.115
7. Wang W, Guo Y, Zhong J, Wang Q, Wang X, Wei H, et al. The clinical significance of microvascular invasion in the surgical planning and postoperative sequential treatment in hepatocellular carcinoma. Sci Rep. (2021) 11:2415. doi: 10.1038/s41598-021-82058-x
8. Lv K, Cao X, Du P, Fu JY, DY G, Zhang J. Radiomics for the detection of microvascular invasion in hepatocellular carcinoma. World J Gastroenterol. (2022) 28:2176–83. doi: 10.3748/wjg.v28.i20.2176
9. Wang L, Jin YX, Ji YZ, Mu Y, Zhang SC, Pan SY. Development and validation of a prediction model for microvascular invasion in hepatocellular carcinoma. World J Gastroenterol. (2020) 26:1647–59. doi: 10.3748/wjg.v26.i14.1647
10. Renzulli M, Brocchi S, Cucchetti A, Mazzotti F, Mosconi C, Sportoletti C, et al. Can Current Preoperative Imaging Be Used to Detect Microvascular Invasion of Hepatocellular Carcinoma? Radiology. (2016) 279:432–42. doi: 10.1148/radiol.2015150998
11. Zhu Y, Feng B, Cai W, Wang B, Meng X, Wang S, et al. Prediction of Microvascular Invasion in Solitary AFP-Negative Hepatocellular Carcinoma ≤5 cm Using a Combination of Imaging Features and Quantitative Dual-Layer Spectral-Detector CT Parameters. Acad Radiol. (2023) 30 Suppl 1:S104–16. doi: 10.1016/j.acra.2023.02.015
12. Yang CB, Zhang S, Jia YJ, Yu Y, Duan HF, Zhang XR, et al. Dual energy spectral CT imaging for the evaluation of small hepatocellular carcinoma microvascular invasion. Eur J Radiol. (2017) 95:222–7. doi: 10.1016/j.ejrad.2017.08.022
13. Kim TM, Lee JM, Yoon JH, Joo I, Park SJ, Jeon SK, et al. Prediction of microvascular invasion of hepatocellular carcinoma: value of volumetric iodine quantification using preoperative dual-energy computed tomography. Cancer Imaging. (2020) 20:60. doi: 10.1186/s40644-020-00338-7
14. Lewin M, Laurent-Bellue A, Desterke C, Radu A, Feghali JA, Farah J, et al. Evaluation of perfusion CT and dual-energy CT for predicting microvascular invasion of hepatocellular carcinoma. Abdom Radiol (NY). (2022) 47:2115–27. doi: 10.1007/s00261-022-03511-7
15. American college of radiology CT/MRI LI-RADS v2018 . Available online at: http://www.acr.org/Quality-Safety/Resources/LIRADS/ (Accessed November 13, 2019).
16. Xu X, Zhang HL, Liu QP, Sun SW, Zhang J, Zhu FP, et al. Radiomic analysis of contrast-enhanced CT predicts microvascular invasion and outcome in hepatocellular carcinoma. J Hepatol. (2019) 70:1133–44. doi: 10.1016/j.jhep.2019.02.023
17. Yang J, Dong X, Wang G, Chen J, Zhang B, Pan W, et al. Preoperative MRI features for characterization of vessels encapsulating tumor clusters and microvascular invasion in hepatocellular carcinoma. Abdom Radiol (NY). (2023) 48:554–66. doi: 10.1007/s00261-022-03740-w
18. Zhou J, Sun H, Wang Z, Cong W, Wang J, Zeng M, et al. Guidelines for the Diagnosis and Treatment of Hepatocellular Carcinoma (2019 Edition). Liver Cancer. (2020) 9:682–720. doi: 10.1159/000509424
19. Ham JH, Yu JS, Choi JM, Cho ES, Kim JH, Chung JJ. Corona enhancement can substitute enhancing capsule in the imaging diagnosis of small (</= 3 cm) HCCs on gadoxetic acid-enhanced MRI. Eur Radiol. (2021) 31:8628–37. doi: 10.1007/s00330-021-07911-z
20. Rudini N, Novello C, Destro A, Riboldi E, Donadon M, Viganò L, et al. Phenotypic and molecular changes in nodule-in-nodule hepatocellular carcinoma with pathogenetic implications. Histopathology. (2018) 73:601–11. doi: 10.1111/his.13659
21. Hu H, Zheng Q, Huang Y, Huang XW, Lai ZC, Liu J, et al. A non-smooth tumor margin on preoperative imaging assesses microvascular invasion of hepatocellular carcinoma: A systematic review and meta-analysis. Sci Rep. (2017) 7:15375. doi: 10.1038/s41598-017-15491-6
22. Lee S, Kim SH, Lee JE, Sinn DH, Park CK. Preoperative gadoxetic acid-enhanced MRI for predicting microvascular invasion in patients with single hepatocellular carcinoma. J Hepatol. (2017) 67:526–34. doi: 10.1016/j.jhep.2017.04.024
23. Yang X, Shao G, Liu J, Liu B, Cai C, Zeng D, et al. Predictive machine learning model for microvascular invasion identification in hepatocellular carcinoma based on the LI-RADS system. Front Oncol. (2022) 12:1021570. doi: 10.3389/fonc.2022.1021570
24. Li H, Wang L, Zhang J, Duan Q, Xu Y, Xue Y. Evaluation of microvascular invasion of hepatocellular carcinoma using whole-lesion histogram analysis with the stretched-exponential diffusion model. Br J Radiol. (2022) 95:20210631. doi: 10.1259/bjr.20210631
25. Jiang H, Wei J, Fu F, Wei H, Qin Y, Duan T, et al. Predicting microvascular invasion in hepatocellular carcinoma: A dual-institution study on gadoxetate disodium-enhanced MRI. Liver Int. (2022) 42:1158–72. doi: 10.1111/liv.15231
26. Kojiro M. 'Nodule-in-nodule' appearance in hepatocellular carcinoma: its significance as a morphologic marker of dedifferentiation. Intervirology. (2004) 47:179–83. doi: 10.1159/000078470
27. Sheng L, Wei H, Yang T, Yang J, Zhang L, Zhu X, et al. Extracellular contrast agent-enhanced MRI is as effective as gadoxetate disodium-enhanced MRI for predicting microvascular invasion in HCC. Eur J Radiol. (2024) 170:111200. doi: 10.1016/j.ejrad.2023.111200
28. Wang Y, Chai S, Cai W, Yu J, Liang P. Prognostic and pathological implications of contrast-enhanced ultrasound features in hepatocellular carcinoma. J Cancer Res Ther. (2023) 19:1040–7. doi: 10.4103/jcrt.jcrt_1155_23
29. Wei H, Jiang H, Liu X, Qin Y, Zheng T, Liu S. Can LI-RADS imaging features at gadoxetic acid-enhanced MRI predict aggressive features on pathology of single hepatocellular carcinoma? Eur J Radiol. (2020) 132:109312. doi: 10.1016/j.ejrad.2020.109312
30. Reginelli A, Vacca G, Segreto T, Picascia R, Clemente A, Urraro F, et al. Can microvascular invasion in hepatocellular carcinoma be predicted by diagnostic imaging? A critical review. Future Oncol. (2018) 14:2985–94. doi: 10.2217/fon-2018-0175
31. Erstad DJ, Tanabe KK. Prognostic and Therapeutic Implications of Microvascular Invasion in Hepatocellular Carcinoma. Ann Surg Oncol. (2019) 26:1474–93. doi: 10.1245/s10434-019-07227-9
32. Li M, Xin Y, Fu S, Liu Z, Li Y, Hu B, et al. Corona Enhancement and Mosaic Architecture for Prognosis and Selection Between of Liver Resection Versus Transcatheter Arterial Chemoembolization in Single Hepatocellular Carcinomas >5 cm Without Extrahepatic Metastases: An Imaging-Based Retrospective Study. Med (Baltimore). (2016) 95:e2458. doi: 10.1097/MD.0000000000002458
33. Yamashita Y, Tsuijita E, Takeishi K, Fujiwara M, Kira S, Mori M, et al. Predictors for microinvasion of small hepatocellular carcinoma ≤ 2 cm. Ann Surg Oncol. (2012) 19:2027–34. doi: 10.1245/s10434-011-2195-0
34. Zheng W, Mu R, Qin X, Li X, Liu F, Zhuang Z, et al. Spectral computed tomography parameters could be surrogate imaging markers to detect early perfusion changes in diabetic kidneys. Quant Imaging Med Surg. (2023) 13:6116–28. doi: 10.21037/qims
35. Mu R, Meng Z, Guo Z, Qin X, Huang G, Yang X, et al. Dual-layer spectral detector computed tomography parameters can improve diagnostic efficiency of lung adenocarcinoma grading. Quant Imaging Med Surg. (2022) 12:4601–11. doi: 10.21037/qims
36. Mu R, Meng Z, Guo Z, Qin X, Huang G, Yang X, et al. Diagnostic value of dual-layer spectral detector CT in differentiating lung adenocarcinoma from squamous cell carcinoma. Front Oncol. (2022) 12:868216. doi: 10.3389/fonc.2022.868216
37. Mu R, Meng Z, Zhang X, Guo Z, Zheng W, Zhuang Z, et al. Parameters of Dual-layer Spectral Detector CT Could be Used to Differentiate Non-Small Cell Lung Cancer from Small Cell Lung Cancer. Curr Med Imaging. (2022) 18:1070–8. doi: 10.2174/1573405618666220308105359
38. Mulé S, Pigneur F, Quelever R, Tenenhaus A, Baranes L, Richard P, et al. Can dual-energy CT replace perfusion CT for the functional evaluation of advanced hepatocellular carcinoma? Eur Radiol. (2018) 28:1977–85. doi: 10.1007/s00330-017-5151-y
39. Sodickson AD, Keraliya A, Czakowski B, Primak A, Wortman J, Uyeda JW. Dual energy CT in clinical routine: how it works and how it adds value. Emerg Radiol. (2021) 28:103–17. doi: 10.1007/s10140-020-01785-2
40. Xia TY, Zhou ZH, Meng XP, Zha JH, Yu Q, Wang WL, et al. Predicting Microvascular Invasion in Hepatocellular Carcinoma Using CT-based Radiomics Model. Radiology. (2023) 307:e222729. doi: 10.1148/radiol.222729
41. Zhao W, Liu W, Liu H, Yi X, Hou J, Pei Y, et al. Preoperative prediction of microvascular invasion of hepatocellular carcinoma with IVIM diffusion-weighted MR imaging and Gd-EOB-DTPA-enhanced MR imaging. PloS One. (2018) 13:e0197488. doi: 10.1371/journal.pone.0197488
42. Mulé S, Chalaye J, Legou F, Tenenhaus A, Calderaro J, Galletto Pregliasco A, et al. Hepatobiliary MR contrast agent uptake as a predictive biomarker of aggressive features on pathology and reduced recurrence-free survival in resectable hepatocellular carcinoma: comparison with dual-tracer 18F-FDG and 18F-FCH PET/CT. Eur Radiol. (2020) 30:5348–57. doi: 10.1007/s00330-020-06923-5
Keywords: hepatocellular carcinoma, microvascular invasion, spectral computer tomography, imaging features, iodine density
Citation: Lv J, Li X, Mu R, Zheng W, Yang P, Huang B, Liu F, Liu X, Song Z, Qin X and Zhu X (2024) Comparison of the diagnostic efficacy between imaging features and iodine density values for predicting microvascular invasion in hepatocellular carcinoma. Front. Oncol. 14:1437347. doi: 10.3389/fonc.2024.1437347
Received: 23 May 2024; Accepted: 09 September 2024;
Published: 14 October 2024.
Edited by:
Liang Zhao, Hubei University of Medicine, ChinaReviewed by:
Carlos Bilreiro, Champalimaud Foundation, PortugalZunyi Zhang, Huazhong University of Science and Technology, China
Copyright © 2024 Lv, Li, Mu, Zheng, Yang, Huang, Liu, Liu, Song, Qin and Zhu. This is an open-access article distributed under the terms of the Creative Commons Attribution License (CC BY). The use, distribution or reproduction in other forums is permitted, provided the original author(s) and the copyright owner(s) are credited and that the original publication in this journal is cited, in accordance with accepted academic practice. No use, distribution or reproduction is permitted which does not comply with these terms.
*Correspondence: Xiaoyan Qin, cWlueGlhb3lhbjIwMTNAMTYzLmNvbQ==; Xiqi Zhu, eGlxaS56aHVAMTYzLmNvbQ==
†These authors have contributed equally to this work