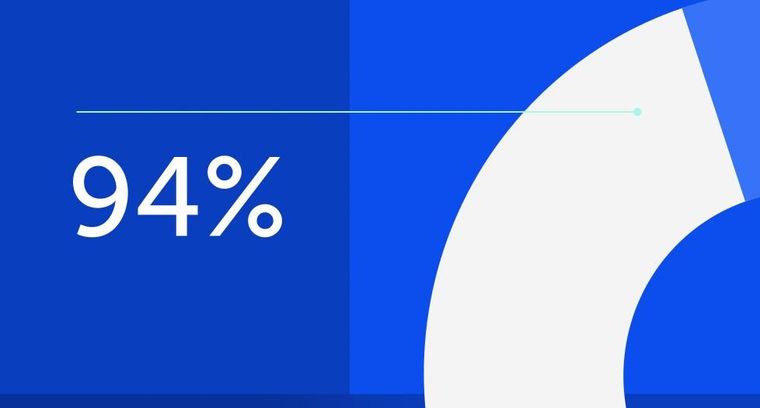
94% of researchers rate our articles as excellent or good
Learn more about the work of our research integrity team to safeguard the quality of each article we publish.
Find out more
ORIGINAL RESEARCH article
Front. Oncol., 22 July 2024
Sec. Molecular and Cellular Oncology
Volume 14 - 2024 | https://doi.org/10.3389/fonc.2024.1432333
This article is part of the Research TopicNew Insights on Sialic Acid and Sialylated Glycans in the Tumorigenic ProcessView all 6 articles
Introduction: Ovarian and other peritoneal cancers have a strong tendency to metastasize into the surrounding adipose tissue. This study describes an effect of the adipose microenvironment on upregulation of sialic acid-containing glycans in ovarian cancer (OC). Heterogeneous populations of glycosylated OC tumors converged to a highly sialylated cell state that regulates tumorigenesis in an immune-dependent manner.
Methods: We modeled the adipose microenvironment by conditioning growth media with human patient-derived adipose tissue. OC cell lines grown in the presence vs. absence of adipose conditioned media (ACM) were characterized by transcriptomics, western blotting, and chemical biology glycan labeling methods. Fluorescence-activated cell sorting was used to separate adipose-driven upregulation of hypersialylated (“SNA-high”) vs. hyposialylated (“SNA-low”) OC subpopulations. The two subpopulations were characterized by further transcriptomic and quantitative polymerase chain reaction analyses, then injected into a syngeneic mouse model. Immune system involvement was implicated using wild type and athymic nude mice with a primary endpoint of overall survival.
Results: Adipose conditioning resulted in upregulation of sialyltransferases ST3GAL1, ST6GAL1, ST6GALNAC3, and ST8Sia1. In culture, OC cells displayed two distinct sialylated subpopulations that were stable for up to 9 passages, suggesting inherent heterogeneity in sialylation that is maintained throughout cell division and media changes. OC tumors that implanted in the omental adipose tissue exclusively reprogrammed to the highly sialylated subpopulation. In wild type C57BL/6 mice, only the hypersialylated SNA-high subpopulation implanted in the adipose, whereas the hyposialylated SNA-low subpopulation failed to be tumorigenic (p=0.023, n=5). In the single case where SNA-low established a tumor, post-mortem analysis revealed reprogramming of the tumor to the SNA-high state in vivo. In athymic nude mice, both subpopulations rapidly formed tumors, implicating a role of the adaptive immune system.
Conclusions: These findings suggest a model of glycan-dependent tumor evolution wherein the adipose microenvironment reprograms OC to a tumorigenic state that resists the adaptive immune system. Mechanistically, adipose factors upregulate sialyltransferases. To our knowledge, this is the first demonstration of the effect of adipose microenvironment on OC tumor sialylation. Our results set the stage for translational applications targeting sialic acid pathways in OC and other peritoneal cancer tumorigenesis and metastasis.
Sialylation, the addition of negatively charged sialic acid sugars on terminal ends of glycans, is upregulated in most cancers and implicated across nearly all phases of cancer progression (1). Sialic acids are 9-carbon hexosamine sugars that cap the ends of glycan chains on proteins and lipids, with roles in altered adhesion and invasion, resistance to apoptosis and immune evasion (2–4). Sialic acids are added to growing glycan chains by twenty different sialyltransferase (STase) enzymes, which fall under one of four groups: ST3GAL and ST6GAL add sialic acid to galactose; while ST6GALNAC and ST8SIA add sialic acid to N-acetylgalactosamine or sialic acid, respectively (5). STases add sialic acids either through an α-2,3 (in the case of ST3GAL), α-2,6 (in the case of ST6GAL and ST6GALNAC), or α-2,8 (in case ST8SIA) linkage, which refers to the stereochemistry and position of sialic acid relative to the preceding sugar residue. Upregulated expression of STases in cancers have been shown to occur through DNA hypomethylation, gene amplification or as a result of oncogene activity (5). Hypersialylation has been correlated with pro-tumor functions in various cancer types (6–8). A current gap in knowledge is the lack of clear understanding on how the tumor microenvironment regulates cancer cell sialylation (9).
Peritoneal cancers such as pancreas, colon, gastric, and ovarian exhibit strong predilection to adipose rich-niches in the peritoneal cavity (10–13). These sites include the adipose-rich omentum as well as the mesenteric and perigonadal adipose (14). Adipose tissues not only serve as an energy depot that can sustain the energy requirements of rapidly growing cancer cells, but can also exert paracrine and endocrine effects by secreting adipokines, cytokines, and chemokines that can support cancer cell migration and invasion (15).
Ovarian cancer (OC), by mortality rate, is the deadliest of all gynecological cancers (16, 17). A key driver of mortality is that OC is often diagnosed at a late, already metastatic, stage (16, 17). The adipose-rich omentum is an early and primary site of OC metastasis (18–21). The chemokine interleukin 8 (IL-8) is secreted by adipocytes and has been shown to chemoattract OC cells very early in the process of metastasis formation (21). Within the adipose niche, cross talk between adipocytes and OC cells leads to metabolic reprogramming in both cell types, which provide OC cells the required energy to sustain rapid cancer growth. Moreover, the adipose microenvironment has been shown to confer chemoresistance through Akt (22) and Bclxl signaling pathways (23). Following treatment, the adipose microenvironment is also a frequent site of residual and recurrent OC (24–26). The importance of the adipose microenvironment in OC progression is underscored in studies demonstrating that the extent of tumor debulking in the adipose-rich omentum (24). The response of adipose-associated metastatic disease to chemotherapy is directly proportional to patient survival (27).
In this study, we demonstrate that the adipose microenvironment is a critical regulator of OC cell sialylation. Using in vitro and in vivo assays and both human and mouse models of OC. We showed that secrete factors from omental cultures upregulated several STases and hence reprogrammed overall OC cell sialylation. Further, we demonstrate enhanced tumor establishment by hypersialylated OC cells in an immune dependent manner, with different tumor growth kinetics and overall survival changes in immune-competent vs. immune-incompetent animals. Our results demonstrate that adipose-induced sialylation reprogramming has significant clinical implications in the targeting of sialylation as therapy for OC and other peritoneal cancers.
Human subject research was reviewed by Wayne State University IRB and found to not meet the definition of Human Participant Research and therefore exempted from IRB oversight. Samples were collected after obtaining informed consent and de-identified by the Karmanos Cancer Institute Biobanking and Correlative Sciences Core. Omentum samples were consecutively collected from patients undergoing laparoscopic or open surgery for a benign or malignant gynecological condition irrespective of diagnosis or age.
R182 and OCSC1-F2 human OC cell lines were established as previously described (28–37). A2780 (RRID : CVCL_0134) human OC cell line was a kind gift from Dr. TC Hamilton. ovcar3 was grown with 20% fbs but the rest of the media mixture was the same RPMI for the other human cell lines were maintained in Roswell Park Memorial Institute (RMPI 1640) media containing 10% fetal bovine serum (FBS), 1% penicillin-streptomycin, 1% MEM-NEAA, 1% HEPES and 1% sodium pyruvate. Triple knock out (TKO) mouse OC cells were kindly provided by Dr. M. Matzuk (38). TKO cells were obtained from spontaneously formed high-grade serous ovarian tumors in mice with conditional KO of Dicer and PTEN and gain of function p53 mutation (p53LSL-R172H/+Dicerflox/floxPtenflox/flox Amhr2cre/+). TKO cells were cultured in 1:1 Dulbecco’s modified eagle medium (DMEM) and Ham’s F12 (F12) medium containing 10% fetal bovine serum and 1% penicillin-streptomycin. ID8Trp53-/- mouse OC cells (clone F3) were kindly provided by Dr. I. McNeish (39, 40) and maintained in DMEM high Glucose (Thermo Fisher Scientific, Waltham, MA) supplemented with 4% FBS, 1% Penicillin-Streptomycin, 1% Sodium Pyruvate, and 1% Insulin-Transferrin-Selenium. ID8Trp53-/- cells were derived from wild-type ID8 mouse OC cells (RRID : CVCL_IU14) by KO of p53 using CRISPR/Cas9 (39, 40). mCherry fluorescence was stably expressed in OCSC1-F2 and TKO cells using lentivirus as previously described (35). All cells were maintained in standard culture conditions at 37°C with 5% CO2. All cell lines were frequently tested for Mycoplasma and authenticated at least once a year by short tandem repeat (STR) profiling and used within 8 passages for each experiment.
Adipose conditioned media (ACM) were prepared as previously described (23, 41). Briefly, 0.5 g of omentum tissue was minced with sterile razor blades and cultured in 10 mL DMEM/F12 media supplemented with 1% exosome-depleted fetal bovine serum (System Biosciences, Palo Alto, CA). ACM was collected the following day, centrifuged at 1500 RPM for 5 minutes, and stored at -80°C until use.
mRNA-seq primed from the polyA was used to determine expression profiles. Lexogen’s QuantSeq 3’mRNA-seq Library Prep Kit (FWD for Illumina) was utilized for building RNA-seq libraries from 0.1-200 ng of total RNA in 5 µl of nuclease-free ultrapure water. Libraries were quantified on the Qubit and Agilent 2200 Tapestation using the DNA High Sensitivity Screen tape. The electrophoretogram, RNA Integrity Number (RIN), and the ratio of the 28S:18S RNA bands are collectively examined to determine overall quality of the RNA. The barcoded libraries were multiplexed at equimolar concentrations and sequenced with 75 bp reads on an Illumina NovaSeq SP flow cell. Average sequencing depth was 1.7x107 reads per sample. Data was demultiplexed using Illumina’s CASAVA 1.8.2 software. After read quality was assessed (42), reads were aligned to the human genome (Build hg38) (43) and tabulated for each gene region (44). Differential gene expression analysis was used to compare transcriptome changes between conditions using a paired design (45). Significantly altered genes (unadjusted p-value ≤ 0.05) were input in iPathwayGuide (Advaita Bioinformatics, Ann Arbor, MI) to identify differentially regulated Pathways. Significantly impacted pathways were those with combined overrepresentation and pathway perturbation with unadjusted p-value < 0.05. Data generated from RNA sequencing is publicly available in Gene Expression Omnibus at GSE269831.
Whole cell protein lysates were isolated by resuspending cell pellets in 1x Cell lysis buffer (Cell Signaling Technologies) with added Complete™ Protease Inhibitor Cocktail (Millipore Sigma), followed by centrifugation for 20 minutes at 13,000 rpm. Protein lysates were quantified using BCA assay. 50 μg of protein lysate was electrophoresed on 12% SDS-polyacrylamide gels and transferred to PVDF membranes (EMD Millipore). After blocking with 5% milk, membranes were probed overnight with primary antibodies at 4°C and incubated with an appropriate secondary antibody for 1 hour at room temperature. The blots were developed using enhanced chemiluminescence and imaged using GE ImageQuant LAS 500 chemiluminescence (Cytiva Life Sciences). The following antibodies were used: ST3GAL1 (RRID: AB_3096968) and GAPDH (RRID : AB_1078991).
Cells were treated with 50 µM 1,3,4,6-tetraacetyl-N-azidoacetylmannosamine (Ac4ManNAz) for 24 h prior to incubation with 50 µM DBCO-AF488 (Lumiprobe) for 1 hr. Cells were then rinsed with PBS containing 1% FBS prior to imaging using Cytation 5 (Agilent-BioTek). Mean fluorescence was calculated using Gen5 software (RRID : SCR_017317).
Cells from culture were collected by trypsinization. Cells from tumors were dissociated using razor blades and passed through 70μm filter to obtain single cell suspension. 1x106 cells were resuspended in 100 µL FACS buffer (1X PBS + 1% bovine serum albumin + 0.05% sodium azide) and stained for 30 mins on ice with the following lectins at 1:400 dilution: SNA-FITC (Vector Laboratories; RRID : AB_2336719), Mal-I-FITC (Bioworld 21761036), Mal-II-FITC (Bioworld 21511103) and PNA-FITC (RRID : AB_2315097). Pe-Cy7 conjugated anti-CD45 (RRID : AB_312979) was used at 1:100 dilution. For analysis of samples from dissociated tumors, Zombie R718 dye (BioLegend) was used to exclude dead cells. After staining, cells were rinsed 3 times with FACS buffer. Data were acquired using CytoFLEX analyzer (RRID : SCR_019627) and CytExpert (RRID : SCR_017217) acquisition software (Beckman Coulter, Brea, CA). Data were analyzed and histograms were generated using FlowJo (RRID : SCR_008520; Becton, Dickinson and Company, Ashland, OR). Gating strategy for the analysis of samples from dissociated tumors is shown in Supplementary Figure 7. For flow cytometry-assisted cell sorting (FACS) of TKO cells, SNA-stained cells were sorted using SH800S (RRID : SCR_018066; Sony Biotechnology, San Jose, CA). Cells were recovered in FBS-containing media appropriate for each cell type, washed with PBS, and plated into T25 tissue culture flasks for expansion and analysis.
RNA was extracted using RNeasy kit (Qiagen) following manufacturer’s instructions. One μg RNA was converted to cDNA using iScript cDNA synthesis kit (Bio-Rad Laboratories) and 1:10 dilution of cDNA was used for each qPCR reaction. qPCR was performed using TaqPath™ qPCR Master Mix, CG (Thermo Fisher Scientific: A55866) with the following TaqMan primers: St3Gal1 (Thermo Fisher Scientific Assay ID: Mm00501493_m1); St6Gal1 (Thermo Fisher Scientific Assay ID: Mm00486119_m1); St6GalNac3 (Thermo Fisher Scientific Assay ID: Mm01316813_m1); and RPS17 (Thermo Fisher Scientific Assay ID: Mm01314921_g1). qCPR was run on CFX96TM PCR detection system (Bio-Rad) using the following thermocycling parameters: polymerase activation at 95°C for 20 secs followed by 40 cycles of denaturation at 95°C for 15 sec and annealing/extension at 60°C for 1 min. Relative expression was calculated using the comparative ΔΔCT method. No RT samples were used as negative control. All reactions were performed in triplicates.
All the described experiments using mice were approved by Wayne State University Animal Care and Use Committee (IACUC 22–03-4474) and mice were housed at Wayne State University Division of Laboratory Animal Resources. Mouse OC cells were injected intra-peritoneally (i.p.) in 7 week old female C57BL/6 mice (RRID : IMSR_JAX:000664; Jackson Laboratories, strain 000664) at 1×107 or in athymic nude mice (Inotiv (Envigo) Hsd : Athymic nude- Foxn1nu, strain 6905F) at 5×106. mCherry fluorescence was measured by live imaging under isoflurane anesthesia twice weekly using Ami HT Imaging System (Spectral Instruments). Mice were imaged with an Excitation of 570nm, emission of 630nm. Tumor burden was quantified using mCherry region of interest (ROI) using Aura Imaging Software (Spectral Instruments). mCherry ROI area exceeding 3.4x108 photons/sec (for C57BL/6) or 5x108 photons/sec (for athymic nude mice) were considered above background based on imaging of non-tumor bearing mice. Animals were sacrificed when mCherry ROI area exceeded 1×109 photons/second for two consecutive images or when abdominal width reached or exceeded 3.4 cm. All animals were included in the analysis and investigators were not blinded to groupings.
Unpaired two-tailed Student t tests, assuming Gaussian distribution, or one-way or two-way analysis of variance (ANOVA) with multiple comparisons were used for comparison between different groups. Log-rank (Mantel-Cox) test was used for survival analysis. P values of 0.05 or less were considered statistically significant. Statistical analysis was performed, and all data were graphed, using GraphPad Prism v9.3.1 (San Diego, CA; RRID : SCR_002798). Data are presented as mean ± SEM.
Given the significance of the adipose microenvironment in OC progression (21, 22, 41, 46, 47) we set to identify mechanisms induced by chronic exposure of OC cells to adipose secreted factors. Since adipocytes represent the primary cell type in the omentum, we obtained adipose-conditioned media (ACM) from dissociated human omentum (23, 41), treated human A2780 OC cells for 7 days with ACM, and performed transcriptomic analysis. Of the 27,162 measured genes, we observed 593 differentially expressed genes (DEGs; p<0.05; fold-changed (FC)>0.6) relative to non-ACM-treated control cells (Figure 1A). Pathway Enrichment and Pathway Impact analyses showed 11 differentially regulated pathways (Table 1, Figure 1B) and one of them was the glycosaminoglycan biosynthesis pathway (p=0.036; Figure 1B, yellow dot). In the glycosaminoglycan biosynthesis pathway, two genes were significantly upregulated in ACM-treated cells: B3GNT7 (p=0.039), which encodes β1-3- N-acetylglucosaminyltransferase and ST3GAL1 (p=0.034), which encodes a STase (Figure 1C). The increase in ST3GAL1, one of several STases that catalyze the addition of sialic acid to terminal ends of glycans, was validated at the protein level using two different patient omenta (Figure 1D; Supplementary Figure 1). These results suggested that the adipose environment can elevate sialylation in OC cells.
Figure 1 Adipose-conditioned media (ACM) upregulate sialyltransferases in human ovarian cancer cells. A2780 human OC cells were treated with ACM for 7 days prior to RNA sequencing. Control cells were maintained in growth media. (A) Volcano plot of differentially expressed genes (DEGs;p<0.05 and fold-change>0.6) comparing Control vs ACM-treated cells; position of ST3GAL1 and B3GNT7 are shown; (B) Differentially regulated pathways showing both Pathway impact (pORA) and Pathway enrichment (pAcc); red dots are differentially regulated and Pathway names are shown in Table 1; yellow dot corresponds to Glycosaminoglycan biosynthesis pathway (p=0.036); (C) DEGs within Glycosaminoglycan pathway: B3GNT7 (p=0.039) and ST3GAL1 (p=0.034); (D) Western blot analysis of human R182 ovarian cancer cells treated with ACM from either patient W or patient Y showing upregulation of ST3GAL1. NT, no treatment control.
Table 1 Differentially regulated pathways in ovarian cancer cells treated with adipose-conditioned media.
To determine if adipose-induced upregulation of STases leads to a measurable increase in cell surface sialylation, we used copper-independent and strain-promoted azide-alkyne click chemistry (SPAAC) to directly label sialic acid sugars. SPAAC reactions connect an azide species and alkyne via a bioorthogonal cycloaddition reaction (48–50). The azide component was installed on sialic sugars through feeding tetraacetylated N-azidoacetyl-mannosamine (ManNAz), which is metabolized and converted to sialic acid azide in cells and added to terminal ends of sialoglycans. The strained alkyne component we used was dibenzocyclooctyne (DBCO) coupled to AF488 fluorophore (DBCO-AF488). When DBCO-AF488 was added to cells that had metabolically incorporated azides on cell surface sialic acids, the reaction resulted in a covalent bond that linked the fluorophore to cell surface sialic acids (Figure 2A). We first determined basal sialic acid expression. Click chemistry performed on mCherry+ TKO mouse OC cells showed membranal green staining demonstrating cell surface sialylation (Figure 2B). To quantitate the effect of adipose conditioning on sialic acid expression, we treated R182 human OC cells with ACM for 72 h and ManNAz was added during the last 24 h of treatment (Figure 2Ci). At the end of the treatment, cells were incubated with DBCO-AF488. Quantification of fluorophore signal showed significant upregulation of cell surface sialic acid expression with ACM compared to ManNaz only control (Figure 2Cii). These results showed that OC cells expressed basal cell surface sialoglycans, which were significantly enhanced by adipose.
Figure 2 Adipose-conditioned media (ACM) upregulate cell surface sialylation in human and mouse ovarian cancer cells. (A) Diagram of click chemistry detailed in text; (B) mCherry+ TKO mouse OC cells were treated with 50 μM ManNAz everyday for 3 days followed by treatment with DBCO-FITC. Microscopy analysis shows basal expression of cell surface sialoglycans; (C) i, treatment protocol with ACM prior to click chemistry; ii, R182 human OC cells were treated as in Ci and mean intensity of AF488 was quantified. Note basal sialylation, which is upregulated by ACM treatment. Data are presented as mean ± SEM (n=3); ** p = 0.0062 by One-Way ANOVA with post-hoc multiple comparison analysis.
For a more comprehensive characterization of cell surface sialylation, we used a panel of four fluorophore-tagged lectins: Sambucus nigra lectin (SNA), Maackia amurensis Lectin I (MAL I), Maackia amurensis Lectin II (MAL II), and peanut agglutinin (PNA). Lectins are sugar binding proteins isolated from plants and animals and are classically used to evaluate glycan structures. SNA preferentially binds α-2,6-linked sialic acids and is a good indicator of ST6GAL1 activity (51). MAL -I and Mal-II preferentially bind` α-2,3-linked sialic acids, the enzymatic products of ST3GAL1 (52–54). Finally, PNA detects non-sialylated galactose, which is one of the required precursors to sialic acid modification on cell surface glycans (55). We first characterized basal cell surface sialylation and used these lectins to stain a panel of human (OCSC1-F2, R182, OVCAR3 and OVCA432; Supplementary Figure 2) and mouse (TKO and ID8p53KO; Supplementary Figure 3) OC cell lines. All human cell lines showed positive staining for SNA and PNA, although with varying intensity (Supplementary Figure 2). Human cell lines with higher staining for SNA (i.e. R182 > OCSC1-F2) showed lower staining for PNA, as expected. MAL-I staining was only observed in OVCAR3 and MAL-II staining was observed in R182, OVCAR3 and OVCA432 but not in OCSC1-F2. Sialylation pattern on the two mouse cell lines tested was also variable. Both mouse cell lines showed positive staining for SNA and PNA (Supplementary Figure 3). Only TKO showed positive staining for MAL-II. Neither of the mouse cell lines stained positively for MAL-I.
Having characterized basal cell surface sialylation, we then utilized the OCSC1-F2 human OC cells to determine the effect of adipose on specific sialic acid linkages. Thus, OCSC1-F2 cells were treated for 7 days with ACM obtained from three different patient omenta prior to lectin staining. Compared to control cultures, OCSC1-F2 cells treated with ACM showed a trend of increased cell surface expression of α-2,6- and α-2,3-linked sialic acids, as detected by SNA and MAL-I staining, respectively. Despite this trend, statistical significance was not reached (Figures 3A, B), suggesting that the effects had relatively high variability. Minimal increase in MAL-II staining and a decrease in PNA staining were observed in ACM-treated cells but the difference from control cells was also not statistically significant (Figures 3C, D).
Figure 3 Adipose-conditioned media (ACM) upregulate α2,6 and α2,3 sialic acids in human ovarian cancer cells. OCSC1-F2 human OC cells were treated with ACM from three different patients (ACM 22, ACM24, ACM 26) for 7 days prior to staining with (A) FITC-tagged SNA; (B) FITC-tagged MAL-I; (C) FITC-tagged MAL-II; (D) FITC-tagged PNA. Histograms show results from ACM24. Graphs show mean ± SEM. n.s. not significant (p > 0.05).
We noted in our lectin staining panels that TKO mouse OC cells reproducibly generated two distinct SNA-staining populations in vitro (Supplementary Figure 3). Over time, the percentage of cells in these two sub-populations fluctuated between ca. 20-60%, but the two distinct populations were persistent and were maintained in culture when followed until 9 passages (Supplementary Figure 4). Flow cytometry assisted cell sorting (FACS) allowed us to further interrogate sialylation on these two cell subpopulations. After authentication through STR profiling (Supplementary Figure 5), lectin staining comparing TKOSNAhigh and TKOSNAlow cells showed that these cultures were only different in SNA staining for α-2,6-linked sialic acids and demonstrated comparable staining for α-2,3-linked sialic acids via MAL-I and MAL-II (Supplementary Figure 6). TKOSNAhigh cells showed slightly lower PNA staining compared to TKOSNAlow cells (Supplementary Figure 6). SNA staining remained stable in both TKOSNAhigh and TKOSNAlow cells when followed through different passages (Figure 4B). To further demonstrate the effect of adipose secreted factors, we treated TKOSNAlow cells with ACM. We noted an increase in SNA and MAL-I in ACM-treated TKOSNAlow cells (Figure 5), which parallels what was observed with ACM-treated OCSC1-F2 human OC cells (Figure 3). In TKOSNAlow cells, we also noted a decrease in PNA staining upon treatment with ACM (Figure 5). No changes were observed with MAL-II staining. Taken together, these results demonstrate that adipose secreted factors can upregulate both α-2,6- and α-2,3-linked sialic acids in both human and mouse ovarian cancer cells.
Figure 4 Heterogeneity of sialylation in TKO mouse OC cultures. (A) Gating strategy for FACS to isolate TKOSNAhigh and TKOSNAlow cells from parental TKO cultures; (B) Heatmap of 30 sialylation-related genes from RNA sequencing performed on TKOSNAhigh and TKOSNAlow cells. * denotes genes that are statistically significant (FDR<0.05). Note upregulation of St6Gal1 (FDR=5x10-5) and St6GalNac3 (FDR=0.0007). Increase in St6Gal1 (C) and St6GalNac3 (D) mRNA was validated by RT- qPCR. Data are presented as mean ± SEM (n=3); * p<0.05; ** p<0.01, **** p<0.0001; † 10-fold increase; †† 14-fold increase.
Figure 5 Adipose-conditioned media (ACM) upregulate α2,6 and α2,3 sialic acids in mouse OC cells. TKOSNAlow cells were sorted by FACS and treated with ACM24 for 7 days prior to lectin staining.
To determine if the adipose-induced increase in sialylation observed in vitro is recapitulated in vivo, we established i.p tumors from parental mCherry+ TKO mouse OC cells in C57BL/6 mice. We previously reported the characterization of i.p. ovarian tumors formed by this model and showed its preferential seeding to omentum, pelvic fat, and mesenteric adipose (51, 56). Necropsy showed omental implants (Figure 6) as previously reported (51, 56). We then compared cell surface sialic acid expression between TKO cancer cells in culture (Figure 6, top panel) and dissociated TKO cancer cells from the omentum implants (Figure 6, bottom panel). Interestingly, we observed sialylation reprogramming upon in vivo engraftment. Unlike TKO cells in culture, which showed two peaks for SNA staining, TKO cells from dissociated omental tumors showed a single SNA peak, which matched the staining intensity observed in the TKOSNAhigh cell population (Figure 6). In addition, TKO cells from dissociated tumors showed increase in both MAL-I and MAL-II staining and decrease in PNA compared to TKO cells in culture (Figure 6; Supplementary Figure 7). These results demonstrate that in vivo engraftment may favor or select TKOSNAhigh cells. Additionally, these data demonstrate a broad reprogramming of sialylation in ovarian tumors with increase in both α-2,6- and α-2,3-sialic acid linkages.
Figure 6 In vivo engraftment upregulates general sialylation. top panel, Cell surface sialic acid expression in mCherry+ TKO mouse OC cells in culture as detected by SNA, MAL-I, MAL-II and PNA; bottom panel, mCherry+ TKO mouse OC cells were injected i.p. in C57BL/6 mice and omental tumors were dissociated, stained with anti-CD45 and FITC-tagged lectins (n=5). Histograms show FITC staining from CD45-negative population. Note loss of SNA- low population and increase in Mal-I and Mal-II upon in vivo tumor formation. Histograms show data from one mouse. Similar results were observed in other mice. Double sided arrow shows SNA levels in tumors is comparable to SNA levels in TKOSNAhigh cells.
The finding in TKO mouse OC cultures of two subpopulations of cells based on SNA staining is in line with previous reports of heterogeneity in expression of α-2,6 sialic acids in breast and lung cancer cultures (57–59). We further confirmed that these cells have differential surface sialic acid levels by treating TKOSNAhigh and TKOSNAlow cells with neuraminidase. Neuraminidase removes all cell surface sialic acids and exposes the underlying galactose, which can be bound by PNA (Supplementary Figure 8). Indeed, baseline PNA staining showed that TKOSNAhigh cells had lower PNA staining compared to TKOSNAlow cells (Supplementary Figure 8) and thus suggests higher cell surface sialic acid in TKOSNAhigh cells. After neuraminidase treatment however, both cell populations showed comparable PNA staining further proving initial difference in cell surface sialic acid levels between the two cell subpopulations.
We then further characterized these two cell subpopulations (Figure 4A) and performed RNA sequencing to identify key genes that may regulate the sialylation differences. We focused on the expression of 30 sialylation-associated genes (60) and found significant difference in expression of St3gal1 (FDR=0.0003), St3gal5 (FDR=5x10-5), St6gal1 (FDR=1x10-125), St6galnac3 (FDR=0.0007), St8sia1 (FDR=0.042), and Slc35a1 (FDR=0.004). Of these DEGs, St6gal1 and St6galnac3 demonstrated the highest fold increase in TKOSNAhigh compared to TKOSNAlow cells of up to 14-fold and 10-fold increase, respectively (Figure 4B). The upregulation in both St6gal1 and St6galnac3 was further validated by RT-qPCR (Figures 4C, D). Taken together, these data are consistent with a heterogeneous pool of sialylated OC cells in culture.
The observation that parental TKO OC cells formed i.p. tumors that consisted of only TKOSNAhigh cells (Figure 5) suggested that TKOSNAlow cells are not tumorigenic. To test this hypothesis, we injected each subpopulation (Supplementary Figure 9) i.p. in immune-competent C57BL/6 mice. We observed tumor formation only in mice administered TKOSNAhigh cells. Logarithmic tumor growth was seen in these mice beginning at day 30 (Figures 7A, B). In contrast, mice injected with TKOSNAlow cells demonstrated measurable disease only immediately after injection (day 3), after which point the signal dropped and all but one mouse remained disease-free until day 70 (Figures 7A, B). As such, tumor growth rate was significantly different in TKOSNAhigh group compared to TKOSNAlow group (p<0.0001; Figure 7A) and mice in TKOSNAhigh group showed significantly shorter overall survival (p=0.035; Figure 7C). These results show that SNA/sialic acid enriches for OC cells that are tumorigenic in immune- competent mice.
Figure 7 TKOSNAlow cells do not form tumors in immune- competent mice. 1x107 TKOSNAhigh or TKOSNAlow cells were injected i.p. in female C57BL/6 mice (n=5). mCherry fluorescence was acquired every 3-4 days and mCherry ROI area was quantified as measure of i.p. tumor burden. (A) Tumor growth curves showing significant difference in measured mCherry ROI between groups (p<0.0001 by Two-Way ANOVA). Dashed line shows threshold for mCherry signal; (B) Representative images obtained from live imaging; (C) Kaplan-Meir survival curve (Log-rank test) showing significantly shorter overall survival in mice injected with TKOSNAhigh cells (p=0.023).
The observed difference in tumorigenic potential between TKOSNAhigh and TKOSNAlow cells in immune competent mice may be due to cell-intrinsic mechanisms or these differences may be immune related. Analysis of cell growth in culture showed comparable growth rate for the two cell populations (Supplementary Figure 10). To determine the contribution of the immune system we injected each cell population in athymic nude mice lacking T cells. Interestingly, in the absence of T cells, TKOSNAlow cells were able to form tumors, albeit with slower kinetics (Figures 8A, B). Mice injected TKOSNAlow cells showed significant delay in tumor formation (p=0.04) but there was no significant difference in overall survival (Figures 8A, C). Taken together, our results demonstrate that the tumorigenic capacity of hyposialylated OC cells can be fully inhibited by the adaptive immune system. In contrast, the innate immune system can only delay but not fully prevent its tumorigenic potential.
Figure 8 TKOSNAlow cells undergo sialylation reprogramming and form tumors in immune-compromised mice. 5x106 TKOSNAhigh or TKOSNAlow cells were injected i.p. in female athymic nude mice (n=5). mCherry fluorescence was acquired every 3-4 days and mCherry ROI area was quantified as measure of i.p. tumor burden. (A) Tumor growth curves showing significant difference in measured mCherry ROI between groups (p=0.04 by Two-Way ANOVA). Dashed line shows threshold for mCherry signal; (B) Representative images obtained from live imaging; (C) Kaplan-Meir survival curve (Log-rank test) showing no significant difference in overall survival between groups (p=0.11, ns); (D) Necropsy shows omentum as primary location of i.p. tumors. Omental tumors were dissociated and cultured as explants for 2 passages prior to lectin staining; (E) RT-qPCR for St3Gal1, St6Gal1, and St6GalNac3. Data are presented as mean ± SEM (n=3); ** p <0.01, *** p < 0.001, ****p<0.0001 by One-Way ANOVA. n.s. not significant (p > 0.05).
The observation that TKOSNAlow cells form tumors in immune deficient mice provided a platform to further validate in vivo sialylation reprogramming. Thus, we characterized the tumors formed by both the TKOSNAhigh and TKOSNAlow cells in athymic nude mice. Necropsy showed that majority of the i.p. tumors were seeded in the omentum (Figure 8D). We then established cultures from dissociated omental explants and characterized their sialylation levels. SNA staining showed comparably high SNA intensity between explants from TKOSNAhigh and TKOSNAlow tumors demonstrating that TKOSNAlow cells are re-programmed in vivo to gain α-2,6-sialylation (Figure 8D). In addition to equivalent SNA staining, explants from both groups showed positive staining for Mal-II demonstrating gain in α-2,3- sialylation as well (Figure 8D). Finally, we measured the levels of St3Gal1, St6Gal1, and St6GalNac3 in the tumor explants. qPCR data showed significant increase in all STases in the tumor explants compared to TKOSNAlow cells grown in culture (Figure 8E). This data replicates what was found in vitro ACM treatment (Figure 5). In both in vitro ACM treatment (Figure 5) and in vivo tumor implantation (Figure 8D), OC cells showed increased sialylation. Taken together, our results demonstrated that adipose factors reprogrammed OC to a hypersialylated state, and that hypersialylation in OC cells resulted in immune system avoidance and a decrease in OC survival.
We demonstrate in this study that the adipose microenvironment is a critical regulator of OC cell sialylation. We first took a broad approach to how secreted factors from adipose-rich omentum impacted the OC cell transcriptome. We discovered a significant effect on the STase, ST3GAL1, and using human and mouse models of OC further characterized adipose-induced sialylation. Upon adipose conditioning in vitro, both human and mouse OC cells increased sialylation for both α-2,3 and α-2,6 linked sialic acids. Further, in vivo engraftment, which for OC typically occurs in the omentum, also lead to increased overall sialylation. Mechanistically, we observed increased expression of not only ST3GAL1, but also ST6GAL1 and ST6GALNAC3 upon in vivo engraftment. Intriguingly, we discovered two distinct subpopulations of OC cells with low or high α-2,6-sialic acid levels. When separately injected into immune-competent mice, these subpopulations had altered tumorigenicity and only the hypersialylated, but not the hyposialylated, OC cells exhibited consistent tumorigenic potential. Interestingly, the lack of T cells in athymic nude mice allowed tumor formation of hyposialylated OC cells. Nevertheless, upon successful tumor formation, both hypersialylated and hyposiaylated OC cells displayed increased overall sialylation.
Like most solid tumors in the peritoneal cavity, OC preferentially metastasizes to the adipose-rich omentum (61). Upon establishment in this niche, studies have shown that OC cells undergo metabolic reprogramming characterized by upregulation of fatty acid intake, shift to β-oxidation, and diversion of glucose towards glycerol-3-phosphate (19, 21, 62, 63). To our knowledge however, this is the first time that the effect of adipose on cancer cell sialylation has been reported. Sialic acid patterns on cell surfaces are regulated stochastically by the levels of individual STase (64, 65). There are 20 known STases, many of which are conserved in mice and humans (66). Previous reports have shown that high-grade serous OC with high expression of sialic acid-related genes demonstrate worse overall survival (60). Further, ST3GAL3 was identified as most predictive of prognosis (60). Our data showed that ST6GAL1 and ST6GALNAC3 are upregulated in the highly sialylated mouse OC cell subpopulations and in addition, that exposure to factors secreted by adipose tissue upregulates at least three STases, ST3GAL1, ST6GAL1, and ST6GALNAC3. The exact mechanism by which adipose secreted factors upregulate STases and thereby increase overall sialylation is under investigation in our lab. Given the known metabolic reprogramming that occurs in OC cells in the adipose niche, we speculate that the changes in nutrient flux and metabolic signaling, which are also major determinants of glycosylation patterns in cells (67, 68), could play a role.
Cell surface sialic acids are ligands for Siglec receptors on immune cells. Siglecs have differential expression on various types of immune cells and most are immunosuppressive via a cytosolic immunoreceptor tyrosine-based inhibitor motif (ITIM) domain (69). Sialic-acid/Siglec binding has been shown to lead to failed maturation of macrophages, generation of myeloid-derived suppressor cells (MDSC), promotion of T regulatory cells, and inactivation of natural killer cells (60, 70, 71). There are 15 Siglecs in humans and 9 in mice and each bind specific sialic acid linkages on glycans. The demonstration that adipose upregulates several STases and increases both α-2,3 and α-2,6 linked sialic acids is critical because these linkages control which Siglec receptor is activated and suggests that adipose-induced sialylation can potentially lead to activation of more than one Siglec receptor consequently promoting an immunosuppressive, pro-tumor immune microenvironment.
An interesting finding is that the tumorigenic capacity of hyposialylated OC cells was curtailed by the presence of T cells. Tumors formed by TKOSNAlow cells in athymic nude mice, still however, grew significantly slower compared to tumors from TKOSNAhigh cells. These results demonstrated that although immune cells such as NK cells, B cells, and macrophages, for instance, can significantly delay the growth of TKOSNAlowcells in vivo, T cells are required to fully prevent their tumorigenic capacity. Moreover, the presence or absence of T cells seemed to be the switch that dictates whether tumors will form or whether hyposialylated OC cells will be reprogrammed to be hypersialylated thus leading to successful tumor formation.
In conclusion, we put forth a proposed model for adipose -induced sialic acid reprogramming (Figure 9). In our model, hypersialylated OC cells that migrated to adipose-rich sites such as the omentum readily established tumors in this niche and upregulated STase expression and overall cell surface sialylation. In contrast, hyposialylated OC cells became targets of the immune system. In the absence of T cells however, hyposialylated OC cells undergo sialylation reprogramming, which further selects for highly sialylated OC cells as a “feed-forward” effect, which can contribute to an immunosuppressive tumor microenvironment. Implications of targeting sialic acid reprogramming factors may thus complement clinical strategies that directly target tumor sialyation patterns (72) for enhanced treatment options.
Figure 9 Proposed model of sialylation reprogramming by adipose microenvironment. (A) Working model for OC sialic acid reprograming in adipose-rich niches involving upregulation of several STase. (B) OC cell sialylation dictates tumor formation in an immune-dependent manner. Absence of T cells allow tumor formation and sialylation reprogramming in hyposialylated OC cells.
The datasets presented in this study can be found in online repositories. The names of the repository/repositories and accession number(s) can be found in the article/Supplementary Material.
Ethical approval was not required for the studies on humans in accordance with the local legislation and institutional requirements because only commercially available established cell lines were used. The animal study was approved by Institutional Animal Care and Use Committee (IACUC) for Wayne State University. The study was conducted in accordance with the local legislation and institutional requirements.
AF: Investigation, Methodology, Validation, Writing – review & editing. GL: Investigation, Methodology, Validation, Writing – original draft. NA: Data curation, Formal analysis, Writing – review & editing. TW: Resources, Investigation, Writing – review & editing. RT: Investigation, Writing – review & editing. SS: Investigation, Writing – review & editing. RG: Resources, Writing – review & editing. RM: Resources, Funding acquisition, Writing – review & editing. GM: Funding acquisition, Writing – review & editing. CF: Conceptualization, Funding acquisition, Supervision, Writing – review & editing. AA: Conceptualization, Funding acquisition, Supervision, Project administration, Writing – review & editing.
The author(s) declare financial support was received for the research, authorship, and/or publication of this article. This study was supported in part by the National Institutes of Health – National Institute of General Medical Sciences (NIH/NIGMS) grant R35GM142637 to CF. NIGMS grants T32GM139807 and Administrative Supplement for Diversity R35GM142637-03S1 to CF supporting GL. The Janet Burros Memorial Foundation and Karmanos Cancer Institute SRIG grant to AA.
The authors acknowledge The Microscopy, Imaging and Cytometry Resources Core and The Biobank and Correlative Sciences Core at Karmanos Cancer Institute (supported in part by NIH Center grant P30 CA22453 to the Karmanos Cancer Institute). The authors also wish to thank Grace Swanson for assistance in depositing datasets and the patients and clinical staff for providing resources used in this study.
The authors declare that the research was conducted in the absence of any commercial or financial relationships that could be construed as a potential conflict of interest.
The author(s) declared that they were an editorial board member of Frontiers, at the time of submission. This had no impact on the peer review process and the final decision.
All claims expressed in this article are solely those of the authors and do not necessarily represent those of their affiliated organizations, or those of the publisher, the editors and the reviewers. Any product that may be evaluated in this article, or claim that may be made by its manufacturer, is not guaranteed or endorsed by the publisher.
The Supplementary Material for this article can be found online at: https://www.frontiersin.org/articles/10.3389/fonc.2024.1432333/full#supplementary-material
1. Dobie C, Skropeta D. Insights into the role of sialylation in cancer progression and metastasis. Br J Cancer. (2021) 124:76–90. doi: 10.1038/s41416-020-01126-7
2. Adams OJ, Stanczak MA, von Gunten S, Läubli H. Targeting sialic acid–Siglec interactions to reverse immune suppression in cancer. Glycobiology. (2018) 28:640–7. doi: 10.1016/j.tranon.2019.12.010
3. Barrueto L, Caminero F, Cash L, Makris C, Lamichhane P, Deshmukh RR. Resistance to checkpoint inhibition in cancer immunotherapy. Transl Oncol. (2020) 13:100738. doi: 10.1016/j.tranon.2019.12.010
4. van de Wall S, Santegoets KCM, van Houtum EJH, Büll C, Adema GJ. Sialoglycans and siglecs can shape the tumor immune microenvironment. Trends Immunol. (2020) 41:274–85. doi: 10.1016/j.it.2020.02.001
5. Hugonnet M, Singh P, Haas Q, von Gunten S. The distinct roles of sialyltransferases in cancer biology and onco-immunology. Front Immunol. (2021) 12. doi: 10.3389/fimmu.2021.799861
6. Büll C, Boltje TJ, van Dinther EAW, Peters T, de Graaf AMA, Leusen JHW, et al. Targeted delivery of a sialic acid-blocking glycomimetic to cancer cells inhibits metastatic spread. ACS Nano. (2015) 9:733–45. doi: 10.1021/nn5061964
7. Cui H, Lin Y, Yue L, Zhao X, Liu J. Differential expression of the α2, 3-sialic acid residues in breast cancer is associated with metastatic potential. Oncol Rep. (2011) 25:1365–71. doi: 10.3892/or.2011.1192
8. Berghuis AY, Pijnenborg JF, Boltje TJ, Pijnenborg JM. Sialic acids in gynecological cancer development and progression: Impact on diagnosis and treatment. Int J Cancer. (2022) 150:678–87. doi: 10.1002/ijc.33866
9. van Houtum EJH, Bull C, Cornelissen LAM, Adema GJ. Siglec signaling in the tumor microenvironment. Front Immunol. (2021) 12:790317. doi: 10.3389/fimmu.2021.790317
10. Avula LR, Hagerty B, Alewine C. Molecular mediators of peritoneal metastasis in pancreatic cancer. Cancer Metastasis Rev. (2020) 39:1223–43. doi: 10.1007/s10555-020-09924-4
11. Xiang W, Shi R, Zhang D, Kang X, Zhang L, Yuan J, et al. Dietary fats suppress the peritoneal seeding of colorectal cancer cells through the TLR4/Cxcl10 axis in adipose tissue macrophages. Signal Transduction Targeted Ther. (2020) 5:239. doi: 10.1038/s41392-020-00327-z
12. Natsume M, Shimura T, Iwasaki H, Okuda Y, Hayashi K, Takahashi S, et al. Omental adipocytes promote peritoneal metastasis of gastric cancer through the CXCL2–VEGFA axis. Br J Cancer. (2020) 123:459–70. doi: 10.1038/s41416-020-0898-3
13. Shea AA, Heffron CL, Grieco JP, Roberts PC, Schmelz EM. Obesity modulates the cellular and molecular microenvironment in the peritoneal cavity: implication for ovarian cancer risk. Front Immunol. (2024) 14. doi: 10.3389/fimmu.2023.1323399
14. Chung EM, Biko DM, Arzamendi AM, Meldrum JT, Stocker JT. Solid tumors of the peritoneum, omentum, and mesentery in children: radiologic-pathologic correlation: from the radiologic pathology archives. RadioGraphics. (2015) 35:521–46. doi: 10.1148/rg.352140273
15. Coelho M, Oliveira T, Fernandes R. Biochemistry of adipose tissue: an endocrine organ. Arch Med Sci. (2013) 9:191–200. doi: 10.5114/aoms.2013.33181
16. Peres LC, Cushing-Haugen KL, Köbel M, Harris HR, Berchuck A, Rossing MA, et al. Invasive epithelial ovarian cancer survival by histotype and disease stage. JNCI: J Natl Cancer Institute. (2019) 111:60–8. doi: 10.1093/jnci/djy071
17. SEER Cancer Stat Facts. Ovarian cancer. Bethesda, MD: National Cancer Institute. (2024). Available at: https://seer.cancer.gov/statfacts/html/ovary.html.
18. Ritch SJ, Telleria CM. The transcoelomic ecosystem and epithelial ovarian cancer dissemination. Front Endocrinol (Lausanne). (2022) 13:886533. doi: 10.3389/fendo.2022.886533
19. Mukherjee A, Chiang CY, Daifotis HA, Nieman KM, Fahrmann JF, Lastra RR, et al. Adipocyte-induced FABP4 expression in ovarian cancer cells promotes metastasis and mediates carboplatin resistance. Cancer Res. (2020) 80:1748–61. doi: 10.1158/0008-5472.CAN-19-1999
20. Arie AB, McNally L, Kapp DS, Teng NN. The omentum and omentectomy in epithelial ovarian cancer: a reappraisal: part II–The role of omentectomy in the staging and treatment of apparent early stage epithelial ovarian cancer. Gynecol Oncol. (2013) 131:784–90. doi: 10.1016/j.ygyno.2013.09.013
21. Nieman KM, Kenny HA, Penicka CV, Ladanyi A, Buell-Gutbrod R, Zillhardt MR, et al. Adipocytes promote ovarian cancer metastasis and provide energy for rapid tumor growth. Nat Med. (2011) 17:1498–503. doi: 10.1038/nm.2492
22. Yang J, Zaman MM, Vlasakov I, Roy R, Huang L, Martin CR, et al. Adipocytes promote ovarian cancer chemoresistance. Sci Rep. (2019) 9:13316. doi: 10.1038/s41598-019-49649-1
23. Cardenas C, Montagna MK, Pitruzzello M, Lima E, Mor G, Alvero AB. Adipocyte microenvironment promotes Bclxl expression and confers chemoresistance in ovarian cancer cells. Apoptosis. (2017) 22:558–69. doi: 10.1007/s10495-016-1339-x
24. Spiliotis JD, Iavazzo C, Kopanakis ND, Christopoulou A. Secondary debulking for ovarian carcinoma relapse: The R-R dilemma - is the prognosis different for residual or recurrent disease? J Turk Ger Gynecol Assoc. (2019) 20:213–7. doi: 10.4274/jtgga.galenos.2019.2018.0165
25. Lengyel E. Ovarian cancer development and metastasis. Am J Pathol. (2010) 177:1053–64. doi: 10.2353/ajpath.2010.100105
26. Bjorndal B, Burri L, Staalesen V, Skorve J, Berge RK. Different adipose depots: their role in the development of metabolic syndrome and mitochondrial response to hypolipidemic agents. J Obes. (2011) 2011:490650. doi: 10.1155/2011/490650
27. Santoro A, Angelico G, Piermattei A, Inzani F, Valente M, Arciuolo D, et al. Pathological chemotherapy response score in patients affected by high grade serous ovarian carcinoma: the prognostic role of omental and ovarian residual disease. Front Oncol. (2019) 9:778. doi: 10.3389/fonc.2019.00778
28. Kamsteeg M, Rutherford T, Sapi E, Hanczaruk B, Shahabi S, Flick M, et al. Phenoxodiol-an isoflavon analogue-induces apoptosis in chemo-resistant ovarain cancer cells. Oncogene. (2003) 22:2611–20. doi: 10.1038/sj.onc.1206422
29. Flick MB, O’Malley D, Rutherford T, Rodov S, Kamsteeg M, Hao XY, et al. Apoptosis-based evaluation of chemosensitivity in ovarian cancer patients. J Soc Gynecol Investig. (2004) 11:252–9. doi: 10.1016/j.jsgi.2003.11.003
30. Alvero AB, O’Malley D, Brown D, Kelly G, Garg M, Chen W, et al. Molecular mechanism of phenoxodiol-induced apoptosis in ovarian carcinoma cells. Cancer. (2006) 106:599–608. doi: 10.1002/cncr.21633
31. Kelly MG, Alvero AB, Chen R, Silasi DA, Abrahams VM, Chan S, et al. TLR-4 signaling promotes tumor growth and paclitaxel chemoresistance in ovarian cancer. Cancer Res. (2006) 66:3859–68. doi: 10.1158/0008-5472.CAN-05-3948
32. Alvero AB, Chen R, Fu HH, Montagna M, Schwartz PE, Rutherford T, et al. Molecular phenotyping of human ovarian cancer stem cells unravels the mechanisms for repair and chemoresistance. Cell Cycle. (2009) 8:158–66. doi: 10.4161/cc.8.1.7533
33. Alvero AB, Fu HH, Holmberg J, Visintin I, Mor L, Marquina CC, et al. Stem-like ovarian cancer cells can serve as tumor vascular progenitors. Stem Cells. (2009) 27:2405–13. doi: 10.1002/stem.191
34. Pizzonia J, Holmberg J, Orton S, Alvero A, Viteri O, McLaughlin W, et al. Multimodality animal rotation imaging system (Mars) for in vivo detection of intraperitoneal tumors. Am J Reprod Immunol. (2012) 67:84–90. doi: 10.1111/j.1600-0897.2011.01070.x
35. Craveiro V, Yang-Hartwich Y, Holmberg JC, Joo WD, Sumi NJ, Pizzonia J, et al. Phenotypic modifications in ovarian cancer stem cells following Paclitaxel treatment. Cancer Med. (2013) 2:751–62. doi: 10.1002/cam4.115
36. Sumi NJ, Lima E, Pizzonia J, Orton SP, Craveiro V, Joo W, et al. Murine model for non-invasive imaging to detect and monitor ovarian cancer recurrence. J visualized experiments: JoVE. (2014) 93:e51815. doi: 10.3791/51815
37. Alvero AB, Kim D, Lima E, Sumi NJ, Lee JS, Cardenas C, et al. Novel approach for the detection of intraperitoneal micrometastasis using an ovarian cancer mouse model. Sci Rep. (2017) 7:40989. doi: 10.1038/srep40989
38. Kim J, Coffey DM, Ma L, Matzuk MM. The ovary is an alternative site of origin for high-grade serous ovarian cancer in mice. Endocrinology. (2015) 156:1975–81. doi: 10.1210/en.2014-1977
39. Walton J, Blagih J, Ennis D, Leung E, Dowson S, Farquharson M, et al. CRISPR/cas9-mediated trp53 and brca2 knockout to generate improved murine models of ovarian high-grade serous carcinoma. Cancer Res. (2016) 76:6118–29. doi: 10.1158/0008-5472.CAN-16-1272
40. Walton JB, Farquharson M, Mason S, Port J, Kruspig B, Dowson S, et al. CRISPR/Cas9-derived models of ovarian high grade serous carcinoma targeting Brca1, Pten and Nf1, and correlation with platinum sensitivity. Sci Rep. (2017) 7:16827. doi: 10.1038/s41598-017-17119-1
41. Zhang Y, Tedja R, Millman M, Wong T, Fox A, Chehade H, et al. Adipose-derived exosomal miR-421 targets CBX7 and promotes metastatic potential in ovarian cancer cells. J Ovarian Res. (2023) 16:233. doi: 10.1186/s13048-023-01312-0
42. Andrews S. FastQC: a quality control tool for high throughput sequence data. (2010). Available at: http://www.bioinformatics.babraham.ac.uk/projects/fastqc.
43. Dobin A, Davis CA, Schlesinger F, Drenkow J, Zaleski C, Jha S, et al. STAR: ultrafast universal RNA-seq aligner. Bioinformatics. (2013) 29:15–21. doi: 10.1093/bioinformatics/bts635
44. Anders S, Pyl PT, Huber W. HTSeq–a Python framework to work with high-throughput sequencing data. Bioinformatics. (2015) 31:166–9. doi: 10.1093/bioinformatics/btu638
45. Robinson MD, McCarthy DJ, Smyth GK. edgeR: a Bioconductor package for differential expression analysis of digital gene expression data. Bioinformatics. (2010) 26:139–40. doi: 10.1093/bioinformatics/btp616
46. Miranda F, Mannion D, Liu S, Zheng Y, Mangala LS, Redondo C, et al. Salt-inducible kinase 2 couples ovarian cancer cell metabolism with survival at the adipocyte-rich metastatic niche. Cancer Cell. (2016) 30:273–89. doi: 10.1016/j.ccell.2016.06.020
47. Chehade H, Tedja R, Ramos H, Bawa TS, Adzibolosu N, Gogoi R, et al. Regulatory role of the adipose microenvironment on ovarian cancer progression. Cancers. (2022) 14:2267. doi: 10.3390/cancers14092267
48. Beatty KE, Fisk JD, Smart BP, Lu YY, Szychowski J, Hangauer MJ, et al. Live-cell imaging of cellular proteins by a strain-promoted azide-alkyne cycloaddition. Chembiochem. (2010) 11:2092–5. doi: 10.1002/cbic.201000419
49. Chang PV, Prescher JA, Sletten EM, Baskin JM, Miller IA, Agard NJ, et al. Copper-free click chemistry in living animals. Proc Natl Acad Sci U.S.A. (2010) 107:1821–6. doi: 10.1073/pnas.0911116107
50. Srinivasan S, Yee NA, Wu K, Zakharian M, Mahmoodi A, Royzen M, et al. SQ3370 Activates Cytotoxic Drug via Click Chemistry at Tumor and Elicits Sustained Responses in Injected & Non-injected Lesions. Adv Ther (Weinh). (2021) 4:2000243. doi: 10.1002/adtp.202000243
51. Alvero AB, Hanlon D, Pitruzzello M, Filler R, Robinson E, Sobolev O, et al. Transimmunization restores immune surveillance and prevents recurrence in a syngeneic mouse model of ovarian cancer. OncoImmunology. (2020) 9:1758869. doi: 10.1080/2162402X.2020.1758869
52. Zhou X, Yang G, Guan F. Biological functions and analytical strategies of sialic acids in tumor. Cells. (2020) 9:273. doi: 10.3390/cells9020273
53. Shen L, Luo Z, Wu J, Qiu L, Luo M, Ke Q, et al. Enhanced expression of α2, 3-linked sialic acids promotes gastric cancer cell metastasis and correlates with poor prognosis. Int J Oncol. (2017) 50:1201–10. doi: 10.3892/ijo.2017.3882
54. Putthisen S, Silsirivanit A, Panawan O, Niibori-Nambu A, Nishiyama-Ikeda Y, Ma-In P, et al. Targeting alpha2, 3-sialylated glycan in glioma stem-like cells by Maackia amurensis lectin-II: A promising strategy for glioma treatment. Exp Cell Res. (2022) 410:112949. doi: 10.1016/j.yexcr.2021.112949
55. Pereira ME, Kabat EA, Lotan R, Sharon N. Immunochemical studies on the specificity of the peanut (Arachis hypogaea) agglutinin. Carbohydr Res. (1976) 51:107–18. doi: 10.1016/S0008-6215(00)84040-9
56. Alvero AB, Fox A, Madina BR, Krady MM, Gogoi R, Chehade H, et al. Immune modulation of innate and adaptive responses restores immune surveillance and establishes antitumor immunologic memory. Cancer Immunol Res. (2024) 12:261–74. doi: 10.1158/2326-6066.CIR-23-0127
57. Pally D, Pramanik D, Hussain S, Verma S, Srinivas A, Kumar RV, et al. Heterogeneity in 2,6-linked sialic acids potentiates invasion of breast cancer epithelia. ACS Cent Sci. (2021) 7:110–25. doi: 10.1021/acscentsci.0c00601
58. Fernandes JP, Cristi F, Eaton HE, Chen P, Haeflinger S, Bernard I, et al. Breast Tumor-Associated Metalloproteases Restrict Reovirus Oncolysis by Cleaving the sigma1 Cell Attachment Protein and Can Be Overcome by Mutation of sigma1. J Virol. (2019) 93:e01380. doi: 10.1128/JVI.01380-19
59. Chen JY, Tang YA, Huang SM, Juan HF, Wu LW, Sun YC, et al. A novel sialyltransferase inhibitor suppresses FAK/paxillin signaling and cancer angiogenesis and metastasis pathways. Cancer Res. (2011) 71:473–83. doi: 10.1158/0008-5472.CAN-10-1303
60. Cao K, Zhang G, Yang M, Wang Y, He M, Zhang C, et al. Attenuation of sialylation augments antitumor immunity and improves response to immunotherapy in ovarian cancer. Cancer Res. (2023) 83:2171–86. doi: 10.1158/0008-5472.CAN-22-3260
61. Peters PN, Schryver EM, Lengyel E, Kenny H. Modeling the early steps of ovarian cancer dissemination in an organotypic culture of the human peritoneal cavity. JoVE (Journal Visualized Experiments). (2015) 106:e53541. doi: 10.3791/53541
62. Ladanyi A, Mukherjee A, Kenny HA, Johnson A, Mitra AK, Sundaresan S, et al. Adipocyte-induced CD36 expression drives ovarian cancer progression and metastasis. Oncogene. (2018) 37:2285–301. doi: 10.1038/s41388-017-0093-z
63. Mukherjee A, Bezwada D, Greco F, Zandbergen M, Shen T, Chiang CY, et al. Adipocytes reprogram cancer cell metabolism by diverting glucose towards glycerol-3-phosphate thereby promoting metastasis. Nat Metab. (2023) 5:1563–77. doi: 10.1038/s42255-023-00879-8
64. Pham ND, Pang P-C, Krishnamurthy S, Wands AM, Grassi P, Dell A, et al. Effects of altered sialic acid biosynthesis on N-linked glycan branching and cell surface interactions. J Biol Chem. (2017) 292:9637–51. doi: 10.1074/jbc.M116.764597
65. Zhang X, Nie H, Whited J, Wang D, Li Y, Sun X-L. Recent approaches for directly profiling cell surface sialoform. Glycobiology. (2018) 28:910–24. doi: 10.1093/glycob/cwy046
66. Harduin-Lepers A, Vallejo-Ruiz V, Krzewinski-Recchi MA, Samyn-Petit B, Julien S, Delannoy P. The human sialyltransferase family. Biochimie. (2001) 83:727–37. doi: 10.1016/S0300-9084(01)01301-3
67. Peixoto A, Relvas-Santos M, Azevedo R, Santos LL, Ferreira JA. Protein glycosylation and tumor microenvironment alterations driving cancer hallmarks. Front Oncol. (2019) 9:380. doi: 10.3389/fonc.2019.00380
68. Marshall S, Nadeau O, Yamasaki K. Dynamic actions of glucose and glucosamine on hexosamine biosynthesis in isolated adipocytes: differential effects on glucosaomine 6-phosphate, UDP-N-acetylglucosamine, and ATP levels. J Biol Chem. (2004) 279:35313–9. doi: 10.1074/jbc.M404133200
69. Pillai S, Netravali IA, Cariappa A, Mattoo H. Siglecs and immune regulation. Annu Rev Immunol. (2012) 30:357–92. doi: 10.1146/annurev-immunol-020711-075018
70. Fraschilla I, Pillai S. Viewing Siglecs through the lens of tumor immunology. Immunol Rev. (2017) 276:178–91. doi: 10.1111/imr.12526
71. Lubbers J, Rodriguez E, van Kooyk Y. Modulation of immune tolerance via siglec-sialic acid interactions. Front Immunol. (2018) 9:2807. doi: 10.3389/fimmu.2018.02807
Keywords: ovarian cancer, sialic acid, sialylation, adipose microenvironment, ST3GAL1, ST6GAL1, tumorigenesis, metastasis
Citation: Fox A, Leonard GD, Adzibolosu N, Wong T, Tedja R, Sharma S, Gogoi R, Morris R, Mor G, Fehl C and Alvero AB (2024) Adipose microenvironment promotes hypersialylation of ovarian cancer cells. Front. Oncol. 14:1432333. doi: 10.3389/fonc.2024.1432333
Received: 13 May 2024; Accepted: 28 June 2024;
Published: 22 July 2024.
Edited by:
Rachel A. Willand-Charnley, South Dakota State University, United StatesReviewed by:
Eleni Maniati, Queen Mary University of London, United KingdomCopyright © 2024 Fox, Leonard, Adzibolosu, Wong, Tedja, Sharma, Gogoi, Morris, Mor, Fehl and Alvero. This is an open-access article distributed under the terms of the Creative Commons Attribution License (CC BY). The use, distribution or reproduction in other forums is permitted, provided the original author(s) and the copyright owner(s) are credited and that the original publication in this journal is cited, in accordance with accepted academic practice. No use, distribution or reproduction is permitted which does not comply with these terms.
*Correspondence: Ayesha B. Alvero, YXllc2hhLmFsdmVyb0B3YXluZS5lZHU=; Charlie Fehl, Y2hhcmxpZS5mZWhsQHdheW5lLmVkdQ==
†These authors have contributed equally to this work
Disclaimer: All claims expressed in this article are solely those of the authors and do not necessarily represent those of their affiliated organizations, or those of the publisher, the editors and the reviewers. Any product that may be evaluated in this article or claim that may be made by its manufacturer is not guaranteed or endorsed by the publisher.
Research integrity at Frontiers
Learn more about the work of our research integrity team to safeguard the quality of each article we publish.